- 1Division of Environmental Technology Management, Department of Management and Engineering, Linköping University, Linköping, Sweden
- 2AKNINET, Architecture and Design, Stockholm, Sweden
Product-as-a-service (PaaS) offerings have advantages and potential for transforming societies to a circular economy and for improving environmental performance. Original equipment manufacturers providing PaaS offerings take higher responsibility for product performances in the use phase than those selling products. This responsibility can be supported by digital technologies such as the Internet of Things (IoT) and big data analytics (BDA). However, insights on how data of product designs and in-use services are managed for PaaS offerings in product lifecycle management (PLM) software are scarce. This mini-review first gives an account of extant major research works that successfully applied BDA, a specific technique of artificial intelligence (AI), to cases in industry through a systematic literature review. Then, these works are analyzed to capture requirements for a PLM system that will exploit the IoT and BDA for PaaS offerings. The captured requirements are summarized as (1) facilitate product and service integration, (2) address multiple lifecycles, (3) adopt an ontology approach encompassing several product standards, and (4) include reading data to process in an interoperation layer.
Introduction
Product-as-a-service (PaaS) offerings, a special kind of product/service systems (PSSs) (Tukker, 2015), are provided to product users where the provider gets paid by providing the product functions or performances. Thereby, PaaS providers have incentives so that more products are reused, repaired and remanufactured, and thus new components/parts are often included in a product already in use. Therefore, according to a review (Tukker, 2015), they were heralded to be among the most effective instrument for transforming societies to a circular economy (CE) (Stahel, 2016) and improving environmental performance ( Blüher et al.,2020). To exploit these potentials with PaaS, the provider needs to prepare for more complex product lifecycles (Umeda et al., 2012) than with the traditional “make-sell-use-dispose” linear model. Typically, the provider needs to be able to answer questions such as: Which options are preferred when a PaaS contract ends? Recondition, remanufacture, or material-recycle the product? And: Which options are preferred after a fault is identified during a PaaS contract? Repair or remanufacture the focal product, or take it back to provide a new one? Decisions made by answering these questions significantly impact both economic and environmental performance.
However, data and information management supported by various software applications for product lifecycle management (PLM) in the manufacturing industry at present does not help answer the above-stated questions effectively and efficiently. Now, along with the advent of digitalization, a variety of technologies is increasingly used in the manufacturing industry, for instance, sensor technologies, edge computing, cloud computing, the Internet of Things (IoT), cyber-physical systems, digital twin, and artificial intelligence (AI). Accessing and using useful data/information from products in use is becoming possible to create new opportunities, including tackling CE challenges (Jabbour et al., 2019). A number of companies see the opportunity to connect the usage of data and CE challenges (EMF, 2019). In the last decade, PLM research investigated closed-loop PLM (Kiritsis, 2011), service integration (Yoo et al., 2016) and connectivity for test (Nardone et al., 2020), to name a few. Nonetheless, even the advanced PLM research fails to provide a method to answer the above-mentioned questions. This is the gap to be filled urgently to transform the manufacturing industry to contribute to CE and environmental sustainability.
Therefore, this research aims to advance the knowledge for PLM software that will help the manufacturing industry improve its environmental sustainability. As a first step in this research, this mini-review article has selected one area focusing on big data analytics (BDA) as a kind of AI because of industries' high interest in BDA (Dekhtiar et al., 2018; Gao et al., 2020), which is often supported by the IoT in implementation. The goal of this mini-review is to capture requirements for PLM software that will exploit the IoT and BDA for PaaS offerings, based on systematic literature review, and analysis (Tranfield et al., 2003) of successful BDA applications to cases in industry. An earlier review by the authors' group (Sakao and Neramballi, 2020) informs that not so many successful BDA applications for design with industrial cases were available, and hence the goal is assumed to be a good fit for a mini-review instead of a full one. Another reason for choosing a mini-review is the urgency of the need for the knowledge demanded by industry.
The remainder of this mini-review article is structured as follows: section Methods and Materials shows the methods and materials for the review. Next, section Results presents the results of the review. Section Discussion of the review results then discusses the results, and section Conclusions concludes the review with relevant future research.
Methods and Materials
Methods
A systematic literature review and analysis (Tranfield et al., 2003) was followed. The core idea of this mini-review was to identify the latest BDA applications in industry and analyse how the next generation of PLM software in industry could exploit such BDA applications in PaaS settings, while the materials used were explained in section Materials. As the idea indicates, this mini-review focused on research synthesis, building upon the practical state of the art of the intersection of the two different domains, that is, BDA and PLM software. In analyzing BDA works, the framework used in a previous review by Sakao and Neramballi (2020) was adopted because of its suitability to PaaS settings. In analyzing the exploitability of BDA in PLM software, the information flows described in a seminal work in the PLM software domain (Kiritsis, 2011) were built upon.
Materials
Industrial State of the Art for Product Lifecycle Management (PLM) Software
PLM software solutions have originated from traditional PDM (product data management) systems, where information about products mainly in the product development and production phases of the lifecycle are managed (Sudarsan et al., 2005). Core functionality includes version management of information about the product and the ability to relate the information objects to each other. Current state-of-the-art enterprise PLM systems also include modules for collaborating around data (e.g., with external stakeholders such as part suppliers) and workflows for change and quality management; see examples (Autodesk, 2021; PTC, 2021; Siemens, 2021). A recent review (Corallo et al., 2020) lists six complementary industry standards for PLM: STEP (ISO 10303), Mandate (ISO 15531), PLCS (ISO 10303-239), PLM@XML, IEC 62264, and the ISO 22000 standard. However, these standards are as well focused on the design and engineering phases (not in nor after the use phase) of the product lifecycle (Ebert, 2013; Yoo et al., 2016), and provide opportunities for enhancement relating to the use phase, which BDA often sources big data from, and other phases.
Despite some advanced software packages offered by PLM software vendors for managing product data in the use phase (e.g., SLM, i.e., service lifecycle management), it is fair to say that the data, information, and knowledge flows break down after the product delivery when the product enters the use phase (Kiritsis, 2011). The traditional barriers between product design/manufacturing and sales/service are substantial as well in today's PLM systems.
Research Works Regarding Applying Big Data Analytics (BDA) to Cases in Industry
The other material is scientific literature on research works with BDA for design (in a broader sense) identified in the ISI (Institute for Scientific Information) Web of Science in June 2021 with keywords that meet the goal of this article and are commonly used; no limitation was set to publication years in the search. ISI Web of Science collects publications on journals with higher impacts and is among the most widely used data base for scientific reviews; e.g., (Brambila-Macias et al., 2018). Only journal articles are included in this mini-review, because they are often of higher quality and more originality and this boundary makes the reviewed literature pool to a more manageable size: the boundary is a limitation of this mini-review. The keywords adopted for the database search were (“big data analytics”) AND (“product” OR “service” OR “product service system” OR “product as a service”) AND (“design” OR “development”). These keywords and the scope adopted are practically1 identical to those used in January 2020 (Sakao and Neramballi, 2020), where the search yielded 117 results, out of which 11 articles were found, after screening, to be such research that includes practical applications to cases in industry. The same method of search in June 2021 yielded 199 results, out of which 23 articles were found to be such research after the same screening. The result of the search and screening is summarized in Table 1. Out of the 23 articles, 12 articles were newly found and summarized in Table A2. The 23 articles show the research that was based on application to cases in industry and has shown potential in contribution to design; these are, hence, the literature analyzed further in this mini-review.
It should be noted that research with cases in industry is still a minority, ~1/10th. However, this mini-review focused on this subset because it builds upon applications validated with cases in industry and not upon hypothetical studies. In terms of the years of all publications: those published in or after 2019 dominated this set with a 64% share, and the mode was the year 2020. These indicate the growth observed in January 2020 in terms of the number of publications with this topic is still valid and the timeliness of reviewing the works for analyzing future research opportunities. Another characteristic was the variety of disciplines of the journals, spanning from information management, computer science, communication systems, operations research, engineering, management, healthcare, transport, and material engineering to sustainability.
Results
Applications of Big Data Analytics (BDA) in Industry
Applications of BDA to cases in industry are summarized in Tables A1, A2. The type column shows the pair of data source and design object in applying BDA to design in PaaS settings; for example, PS meaning “source the data of products in use to design service.” The research in this table is ordered according to the type, from PP, PS, and SS to SP. In these applications, both products, and services in the use phase provide data for BDA, contributing to designing both products and services. Only two works out of 23 addressed environmental impacts, showing research opportunities of BDA's contribution to environmental sustainability.
The majority of the BDA works analyzed online data (e.g., on social media) to obtain insights of users and predict user behaviors such as purchasing. This type of analytics begins with digital data, where the outputs are applicable to designing products and/or services. However, more relevant to PLM systems is the interaction between physical items (e.g., products and humans) and their digitized data. For instance, conditions of bridges (Kim and Queiroz, 2017), sensor data of athletics goods (Zhan et al., 2018), mechanical part characteristics (Yi and Fones, 2020), car drivers' behaviors (Tofangchi et al., 2021), and transport service users (Lee, 2018) were targeted. The data sources and design objects of relevance to the BDA works are mapped on the scheme for PLM by developing an existing framework (Kiritsis, 2011) as in Figure 1; service design and contexts are newly added to reflect PaaS settings and BDA, respectively. In most of the applications, data sources originate from processes of products or services in the use phase (i.e., the middle of life).
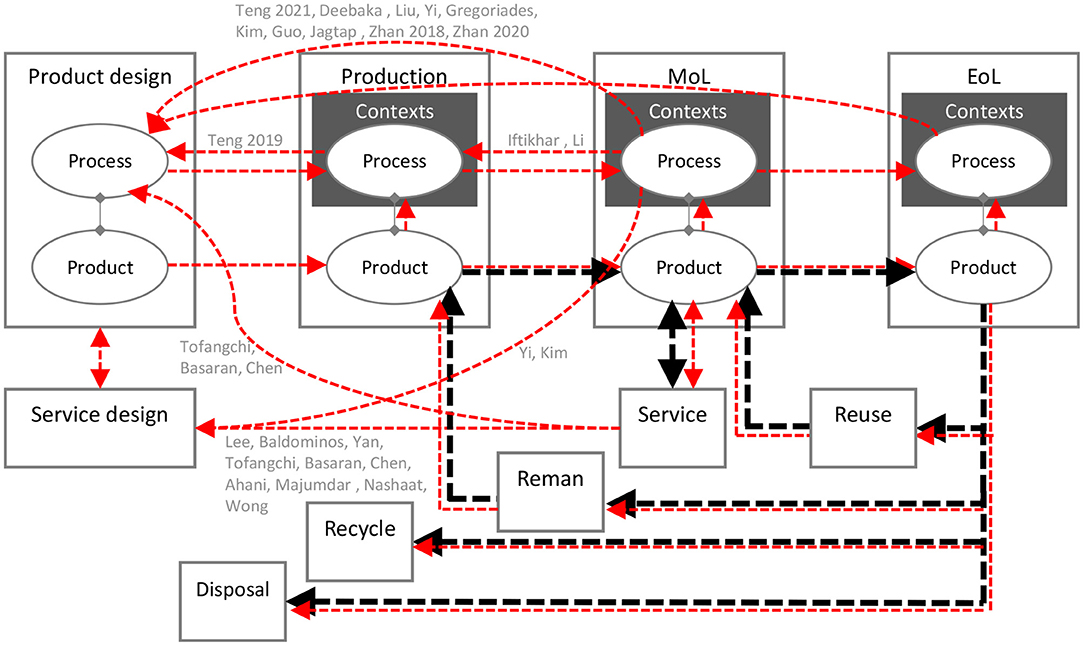
Figure 1. Applications of BDA in industry mapped on PLM information flows. Note: Red (thinner) and black (thicker) arrows denote information and physical flows, respectively. BDA applications in Tables A1, A2 are referred to by their first author names and, if needed, publication years. The PLM flows are developed based on (Kiritsis, 2011). MoL and EoL denote the middle of life and the end of life, respectively.
One important feature observed from analyzing the BDA applications should be mentioned without going to their details in this mini-review. Figure 1 shows that all the information flows representing the BDA applications to design are upstream (from right to left, simply put). This is natural, because design by definition anticipates products or processes to be realized. However, this review showed that such BDA applications executed in real life are reported in scientific publications.
Requirements for Product Lifecycle Management (PLM) Software
This section shows the results of analyzing the current PLM software (section Industrial state of the art for product lifecycle management (PLM) software) in light of the BDA applications (section Applications of big data analytics (BDA) in industry), partly based on earlier PLM software research. The results are presented in the form of requirements (Reqs) for PLM software. A major feature of current PLM software is that the flows of data/information are toward the downstream, i.e., from design to production and, when applicable, to use. This direction is the opposite from information flows in analyzing use cases and then informing to design, which is essential in any engineering design, as pointed out by Sherer (2005). The next generation of PLM systems has to overcome traditional barriers and better integrate product design and product service data (Yoo et al., 2016), especially addressing the information flows toward the upstream. This integration is critical for PaaS and PSS, according to many researchers (Tukker, 2015).
Also, in PaaS offerings, where parts are refurbished or remanufactured after their first life and for their further lives, the PLM system has to support multiple lifecycles. The lack of support for closed-loop manufacturing in current PLM systems has been highlighted in academia (Jun et al., 2007; Kiritsis, 2011). The EU-funded FP6 project PROMISE aimed to provide a means to transfer critical information about a product back to the design phase and forward to appropriate intervention areas (PROMISE, 2008). Another EU-funded project, ResCom, has developed a collection of methodologies and tools for the implementation of closed-loop manufacturing systems supporting multiple lifecycles (ResCom, 2017). Thus, requirements are formulated in a general form as below:
Req 1: Facilitate integration between service and product design.
Req 2: Support management of the product in multiple lifecycles, including traceability.
A common conclusion in both the above-mentioned projects is the importance of product standards (e.g., PLCS, ISO 15926) as key enablers for PLM (see section Industrial state of the art for product lifecycle management (PLM) software). There are several standards available, but most use cases are centered around using standards as exchange formats and not as daily working formats, that is, mostly used at specific times during the engineering workflows (Dekhtiar et al., 2018) as depicted in Figure 1. Moreover, due to differences in the implementations at various organizations, it may be questionable to heavily rely on these formats for analytic purposes. In the same way that a PLM system has to support several product data ontologies, a PLM system has to be able to integrate with various new applications. To support new disruptive technology, an enterprise has to allow for a system-of-systems approach to PLM rather than having a vision that one application can fit it all; in other words, a federated system approach is necessary.
Req 3: Have an ontology approach that can encompass several product standards in daily working formats.
In such an architecture, the PLM system cannot store all data about all products; see the data volumes in the BDA applications (section Applications of big data analytics (BDA) in industry). Because all IoT systems (with products in the use phase) generate lots of data, all cannot be collected to be processed in the PLM mainframe. There is neither a lack of data to collect nor a lack of drawings or documents to read. Rather, the challenge is how to read/collect the data to information and interoperate it to knowledge based on the reviewed successful cases in Tables A1, A2. Integrating only product-generated data but also data generated from other sources, such as human observation, user profile data, weather, and environmental factors will be important; for example, see (Kim and Queiroz, 2017; Tofangchi et al., 2021) in Tables A1, A2.
Big data solutions and cloud platforms can provide infrastructure and tools for processing and analyzing the huge amount of IoT data. However, efficient solutions are still required that can structure, annotate, share and make sense of that data (Yoo et al., 2016). Add to this, that data storage capacity, network traffic, and computation capabilities are all facing an exponential increase, which will demand the manufacturing industry to rethink how engineering data at a large scale is managed (Dekhtiar et al., 2018). The amount of data will not only be too large to be humanly manageable but also bring costs; compare cloud storage cost today at about $0.021 per GB (AWS, 2021).
There is, hence, a need for a middle layer to decide upon what data is to be collected and processed into information, as implied by Figure 1. This means data has to be processed in the edge, and to achieve this efficiently, there will be a need for AI in the edge layer to decide. Many earlier articles are about collecting useful data as pointed out in Fosso Wamba et al. (2015), but the decision of what is useful cannot be made solely by a data scientist or product designer since the data that can be collected is exponentially increasing.
Req 4: The PLM system has to include reading data to process in an interoperation layer before presenting to BDA.
Discussion Of The Review Results
This mini-review contributes to the scientific body of knowledge in two ways. First, an account of applications of BDA to cases in industry for design is updated, building upon the authors' earlier review of the same framework (Sakao and Neramballi, 2020). It is an addition to BDA reviews in smart manufacturing contexts (Cui et al., 2020; Gao et al., 2020). In particular, the account focused on BDA applications in industry, providing useful insights from practice, not only from simulations with hypothetical data. Second, insights into how future PLM software could incorporate BDA and IoT are provided to fill a gap in recent PLM reviews (Sivanathan et al., 2017; Corallo et al., 2020). These insights were represented as four requirements for future PLM software, which were hardly discussed explicitly in earlier research. Also, the presented research complemented information flows essential to PaaS and BDA on the earlier PLM framework (Kiritsis, 2011; Yoo et al., 2016): especially, service design and contexts were added, and addressed in a coherent manner. Importantly, they build upon insights from different topics such as PaaS (Tukker, 2015) and engineering design. Notably, PLM systems often fail in deployment because of their poor alignment or integration with workflows (Ebert, 2013), and the risk of this failure was aimed for minimization in this research.
One major, inherent challenge in deploying BDA is how to address various types of data, including unstructured, and heterogeneous data. This challenge is more relevant in PLM, covering different phases of the lifecycle. There are no pre-defined base parameters to begin with when implementing BDA or, more generally, AI, nor is any standard available for what to collect or store. Adopting data lakes (Porter and Heppelmann, 2015) is a potential approach, as touched upon in the context of lifecycle engineering (Sakao et al., 2021), but this issue requires further research. Implementing BDA or AI in a PLM system will not be technology-limited; rather, finding the base parameters for use cases in question is perceived as the major challenge.
The practical contribution lies in the advancement of the knowledge for developing future PLM systems, provided from areas beyond PLM. PLM research and development communities can build upon the proposed requirements by further validation with use cases in industry. This interdisciplinary way of work (Sakao and Brambila-Macias, 2018) is critical in digitalization in general, where knowledge from different domains is essential. See other earlier research, for instance, showing the need for domain experts' hand-crafting in applying machine learning (Dekhtiar et al., 2018). Note that the proposed requirements are not exhaustively listed, but the major ones focusing on PaaS and BDA are. Also, part of the information flows enabled by BDA for improving the product lifecycle performance (depicted by Figure 1) may be suitable to be implemented by other types of software than PLM software, for example, production planning based on the information flow from the use phase to production. Thus, a more detailed investigation is necessary for practical implementation as software.
Environmental sustainability is addressed only by a minority of the BDA applications (see Tables A1, A2). A recent review (Jabbour et al., 2019) argues that BDA will contribute to a CE, but more research is needed to support the argument. Earlier PLM research and development also shows the lack of the environmental sustainability aspect and, first of all, the whole lifecycle coverage (see section Industrial state of the art for product lifecycle management (PLM) software). Now, by observing interests by industry in using BDA and AI toward a CE (EMF, 2019), advancing the research and practice for enhancing environmental sustainability is expected.
Conclusions
This mini-review updated an account of applications of big data analytics (BDA) to cases in industry through a systematic literature review, building upon the authors' earlier review with the same framework. Analyzing the found BDA applications and the practical state of the art of product lifecycle management (PLM) software, four requirements were derived, as the main contribution of this paper, for a PLM system that will exploit the Internet of Things (IoT) and BDA for product-as-a-service (PaaS) offerings. It should be noted that a limitation of this mini-review is the boundary for the addressed publications as mentioned in section Materials. Enlarging the boundary for the reviewed literature is of interest as future work. Additional analysis of use cases from industry will enrich the requirements. These requirements are expected to guide the development of the next generations of PLM systems in the future and thereby eventually support manufacturing companies' transition to circular systems and enhance environmental sustainability.
Author Contributions
The first conceptualization and methodology were done by TS; the writing-original draft preparation was done by AKN mainly for the PLM and TS mainly for PaaS and BDA, while the intersection was addressed jointly by AKN and TS; the review was performed by both AKN and TS; and funding acquisition was arranged by TS.
Funding
This research was supported partly by the Adapt 2030 (Adaptive lifecycle design by applying digitalization and AI techniques to production) project (No. 2019-05589), funded by VINNOVA, Sweden's Innovation Agency.
Conflict of Interest
The authors declare that the research was conducted in the absence of any commercial or financial relationships that could be construed as a potential conflict of interest.
Publisher's Note
All claims expressed in this article are solely those of the authors and do not necessarily represent those of their affiliated organizations, or those of the publisher, the editors and the reviewers. Any product that may be evaluated in this article, or claim that may be made by its manufacturer, is not guaranteed or endorsed by the publisher.
Acknowledgments
The authors acknowledge valuable comments to earlier versions of the manuscript from experts in industry, including Torbjörn Holm of Eurostep Group.
Supplementary Material
The Supplementary Material for this article can be found online at: https://www.frontiersin.org/articles/10.3389/frsus.2021.735550/full#supplementary-material
Footnotes
1. ^The only difference is that the 2021 search used (“product” OR “service” OR “product service system” OR “product as a service”) instead of (“product” OR “service” OR “product service system”) as with the 2020 search. However, this difference made no difference in the yielded results.
References
Autodesk (2021). What You Can do With Product Lifecycle Management [Online]. Available online at: https://www.autodesk.com/solutions/plm (accessed June 22, 2021).
AWS (2021). Amazon S3 Pricing [Online]. Available: https://aws.amazon.com/s3/pricing/ (accessed June 22, 2021).
Blüher, T., Riedelsheimer, T., Gogineni, S., Klemichen, A., and Stark, R. (2020). Systematic literature review—effects of PSS on sustainability based on use case assessments. Sustainability 12:12176989. doi: 10.3390/su12176989
Brambila-Macias, S., Sakao, T., and Kowalkowski, C. (2018). Bridging the gap between engineering design and marketing: insights for research and practice in product/service system design. Design Science 4:3. doi: 10.1017/dsj.2018.3
Corallo, A., Latino, M. E., Menegoli, M., and Pontrandolfo, P. (2020). A systematic literature review to explore traceability and lifecycle relationship. Int. J. Product. Res. 58, 4789–4807. doi: 10.1080/00207543.2020.1771455
Cui, Y. S., Kara, S., and Chan, K. C. (2020). Manufacturing big data ecosystem: a systematic literature review. Robot. Comput. Integr. Manuf. 62:101861. doi: 10.1016/j.rcim.2019.101861
Dekhtiar, J., Durupt, A., Bricogne, M., Eynard, B., Rowson, H., and Kiritsis, D. (2018). Deep learning for big data applications in CAD and PLM—Research review, opportunities and case study. Comput. Industr. 100, 227–243. doi: 10.1016/j.compind.2018.04.005
Ebert, C. (2013). Improving engineering efficiency with PLM/ALM. Softw. Syst. Modeling 12, 443–449. doi: 10.1007/s10270-013-0347-3
EMF (2019). Artificial Intelligence and the Circular Economy - AI as a Tool to Accelerate the Transition. Cowes: Ellen MacArthur Foundation.
Fosso Wamba, S., Akter, S., Edwards, A., Chopin, G., and Gnanzou, D. (2015). How ‘big data' can make big impact: findings from a systematic review and a longitudinal case study. Int. J. Product. Econ. 165, 234–246. doi: 10.1016/j.ijpe.2014.12.031
Gao, R. X., Wang, L., Helu, M., and Teti, R. (2020). Big data analytics for smart factories of the future. CIRP Annal. Manufact. Technol. 69, 668–692. doi: 10.1016/j.cirp.2020.05.002
Jabbour, C. J. C., de Sousa Jabbour, A. B. L., Sarkis, J., and Filho, M. G. (2019). Unlocking the circular economy through new business models based on large-scale data: an integrative framework and research agenda. Technol. Forecast. Soc. Change 144, 546–552. doi: 10.1016/j.techfore.2017.09.010
Jun, H. B., Shin, J. H., Kiritsis, D., and Xirouchakis, P. (2007). System architecture for closed-loop PLM. Int. J. Comput. Integr. Manufact. 20, 684–698. doi: 10.1080/09511920701566624
Kim, Y. J., and Queiroz, L. B. (2017). Big Data for condition evaluation of constructed bridges. Eng. Struct. 141, 217–227. doi: 10.1016/j.engstruct.2017.03.028
Kiritsis, D. (2011). Closed-loop PLM for intelligent products in the era of the Internet of things. Comput. Aided Design 43, 479–501. doi: 10.1016/j.cad.2010.03.002
Lee, S. (2018). Night Owl Bus: An ICT-supported public transport option for night time workers and the young in Seoul, South Korea. ITE J. Inst. Transport. Eng. 88, 44–49.
Nardone, R., Marrone, S., Gentile, U., Amato, A., Barberio, G., Benerecetti, M., et al. (2020). An OSLC-based environment for system-level functional testing of ERTMS/ETCS controllers. J. Syst. Softw. 161:478. doi: 10.1016/j.jss.2019.110478
Porter, M. E., and Heppelmann, J. E. (2015). How smart, connected products are transforming companies. Harv. Bus. Rev. 93, 96–114.
PROMISE (2008). Product Lifecycle Management and Information Tracking using Smart Embedded Systems [Online]. Available online at: https://cordis.europa.eu/project/id/507100/reporting (accessed June 21, 2021).
PTC (2021). Product Lifecycle Management Capabilities [Online]. Available online at: https://www.ptc.com/en/technologies/plm#what-is-plm (accessed June 22, 2021].
ResCom (2017). Resource Conservative Manufacturing [Online]. Available online at: https://www.rescoms.eu/ (accessed June 21, 2021).
Sakao, T., and Brambila-Macias, S. A. (2018). Do we share an understanding of transdisciplinarity in environmental sustainability research? J. Clean. Prod. 170, 1399–1403. doi: 10.1016/j.jclepro.2017.09.226
Sakao, T., Funk, P., Matschewsky, J., Bengtsson, M., and Ahmed, M. U. (2021). “AI-LCE: Adaptive and intelligent life cycle engineering by applying digitalization and ai methods—an emerging paradigm shift in life cycle engineering,” in: 28th CIRP Conference on Life Cycle Engineering. Amsterdam.
Sakao, T., and Neramballi, A. (2020). A product/service-system design schema: application to big data analytics. Sustainability 12:484. doi: 10.3390/su12083484
Sherer, S. A. (2005). From supply-chain management to value network advocacy: implications for e-supply chains. Supply Chain Manage. Int. J. 10, 77–83. doi: 10.1108/13598540510589151
Siemens (2021). Get Started with PLM Fundamentals [Online]. Available online at: https://www.plm.automation.siemens.com/global/en/products/teamcenter/ (accessed June 22, 2021).
Sivanathan, A., Ritchie, J. M., and Lim, T. (2017). A novel design engineering review system withsearchable content: knowledge engineering viareal-time multimodal recording. J. Eng. Design 28, 681–708. doi: 10.1080/09544828.2017.1393655
Sudarsan, R., Fenves, S. J., Sriram, R. D., and Wang, F. (2005). A product information modeling framework for product lifecycle management. Comput. Aided Design 37, 1399–1411. doi: 10.1016/j.cad.2005.02.010
Tofangchi, S., Hanelt, A., Marz, D., and Kolbe, L. M. (2021). Handling the efficiency–personalization trade-off in service robotics: a machine-learning approach. J. Manage. Inform. Syst. 38, 246–276. doi: 10.1080/07421222.2021.1870391
Tranfield, D., Denyer, D., and Smart, P. (2003). Towards a methodology for developing evidence-informed management knowledge by means of systematic review. Br. J. Manage. 14, 207–222. doi: 10.1111/1467-8551.00375
Tukker, A. (2015). Product services for a resource-efficient and circular economy—a review. J. Clean. Prod. 97, 76–91. doi: 10.1016/j.jclepro.2013.11.049
Umeda, Y., Takata, S., Kimura, F., Tomiyama, T., Sutherland, J. W., Kara, S., et al. (2012). Toward integrated product and process life cycle planning—an environmental perspective. CIRP Annal Manufact. Technol. 61, 681–702. doi: 10.1016/j.cirp.2012.05.004
Yi, S., and Fones, R. (2020). Machine learning framework for predicting reliability of solder joints. Soldering Surf. Mount Technol. 32, 82–92. doi: 10.1108/SSMT-04-2019-0013
Yoo, M.-J., Grozel, C., and Kiritsis, D. (2016). Closed-Loop Lifecycle management of service and product in the internet of things: semantic framework for knowledge integration. Sensors 16:71053. doi: 10.3390/s16071053
Keywords: systematic literature review, product/service system (pss), circular economy, PLM software, big data analytics, context
Citation: Sakao T and Nordholm AK (2021) Requirements for a Product Lifecycle Management System Using Internet of Things and Big Data Analytics for Product-as-a-Service. Front. Sustain. 2:735550. doi: 10.3389/frsus.2021.735550
Received: 02 July 2021; Accepted: 31 July 2021;
Published: 25 August 2021.
Edited by:
Bankole Awuzie, Central University of Technology, South AfricaReviewed by:
Douglas Aghimien, University of Johannesburg, South AfricaTemitope Seun Omotayo, Leeds Beckett University, United Kingdom
Copyright © 2021 Sakao and Nordholm. This is an open-access article distributed under the terms of the Creative Commons Attribution License (CC BY). The use, distribution or reproduction in other forums is permitted, provided the original author(s) and the copyright owner(s) are credited and that the original publication in this journal is cited, in accordance with accepted academic practice. No use, distribution or reproduction is permitted which does not comply with these terms.
*Correspondence: Tomohiko Sakao, tomohiko.sakao@liu.se