- Division of Sustainable Development, College of Science and Engineering, Hamad Bin Khalifa University, Doha, Qatar
Critical infrastructures share the same space and mutual dependencies, and consequently contribute to city's overall resilience. However, each has different assessment methods and metrics, which prevents the integration of the performance results for different infrastructures into a single holistic evaluation index that can reflect the city's overall resilience and expected performance. To address this challenge, we propose the development of a multi-criteria-based integration approach that can allow the combination of different evaluation results from different assessment methods through partial factors. The proposed equation incorporates several factors, which can be divided into four groups: (1) method-efficiency factors, (2) infrastructure importance factors, (3) infrastructures' interdependency factors, and (4) strict limits that ensure that the infrastructures are suitable for hosting mega sports events. Using this approach, we hope to open the way for future research into suitable factors and facilitate resilience-based design in sustainable development and municipal planning.
Introduction
Resilience is a relatively new concept, especially to urban planning and infrastructure development. The roots of resilience can be traced back to ancient Greek, describing metal's ability to “bounce back” to its original shape (Anaut, 2005). However, its first introduction into modern science was by Holling in 1973, describing a biological system's capacity to sustain disturbances and recover within a specific timeframe (Holling, 1973). The concept has become incorporated into engineering domains over the last two decades, with a dramatic increase in interest since 2010 due to the increased uncertainty and emergence of new challenges and threats (Wan et al., 2018; Salimi and Al-Ghamdi, 2020). Threats such as natural hazards strengthened by climate change and intentional attacks (including those in the cyber domain) fueled by political tensions have been among the main challenges for urban infrastructures and their security in recent years (Schleussner et al., 2016; Salimi and Al-Ghamdi, 2020).
Ensuring urban infrastructure resilience is critical for achieving sustainable development goals. Over the past 50 years, an accelerated urbanization trend has spread across the globe. This trend has involved huge investments and the development of extensive infrastructure networks to provide essential services and has eventually led to economic and human capital concentration in urban areas (Addanki and Venkataraman, 2017; The Resilience Shift, 2019). However, the increase in the severity of the aforementioned challenges raised concerns about the efficiency of previous development practices and their ability to withstand disturbances (Mattsson and Jenelius, 2015). Moreover, the integration of technological solutions into infrastructure has increased its complexity and created interdependent links between various critical ones threatening to cause the propagation of disturbance from one infrastructure to another (Ahmed et al., 2019; Ganin et al., 2019). These interdependencies, complexities, and unprecedented threats have steered the design mentality toward ensuring the abilities of critical infrastructures to sustain damage and recover in a suitable timeframe and thus toward reducing the loss of economic, human, and environmental resources, in other words, ensuring their resilience (Salimi and Al-Ghamdi, 2020).
Infrastructure resilience is a combination of several properties that can describe the system, and therefore there is no unified definition for it. While reviewing the literature about resilience, one notices a wide array of definitions varying from one researcher to another (Hosseini et al., 2016; Rus et al., 2018). This variation stems from differences in interests and focus, whether it is impact reduction, recovery, or the system in all stages. Furthermore, most of the definitions emphasize a different combination of properties that characterize the system, mainly reliability, vulnerability, robustness, survivability, and flexibility (Serdar and Al-Ghamdi, 2021). This variation in definitions has hindered the efforts to create a consensus on a single metric or indicator and, consequently, a straightforward assessment method (Hosseini et al., 2016; Huck and Monstadt, 2019).
The variation in definitions of infrastructure resilience is reflected by the variation in metrics and assessment methods. Researchers have suggested many assessment methods for infrastructure resilience, each with specific capabilities and limitations (Liu and Song, 2020). Some methods effectively reflect on previous events using several records sources, leveraging on Big Data applications that have limited ability to predict the future. Simulation approaches can help to predict system behavior under specific scenarios but have many limitations regarding calibration and scalability. Complex networks (graph theory) can effectively assess large infrastructure networks by focusing on connectivity measures but have limitations regarding indicating the physical properties and flows. Physical or flow-based models are applicable when focusing on unique elements, a small network, or an extensive network with low resolution (focusing only on the main points and substations and the flow between them) but become hard to apply when the aim is accurate calculations for large-scale networks (such as city-scale). The list of assessment methods is quite long; however, the reader can refer to Sun et al. (2018) and Liu and Song (2020) for further insights and examples. It is worth noting that each focuses on or uses specific metrics, such as the variation in speed, flow, capacity, supply, or connectivity. This broad set of metrics limit comparability, despite the efforts to reflect the metrics as a system performance change over time and to calculate the “resilience triangle” to compare the different arrangements of the system (Bruneau et al., 2003; Sun et al., 2018).
Mega sports events (MSEs) present both a unique and vital opportunity to boost countries' economies and the host cities' development and a formidable challenge to their resilience. Hosting an MSE is a costly venture but a rewarding one if directed appropriately, reshaping the country's future and contributing as an engine for the economic diversification process (Meza Talavera et al., 2019; The Supreme Committee for Delivery Legacy, 2020). Furthermore, MSEs require the reorganization of the host cities' infrastructures to accommodate the expected spectators and fans and thus impose an opportunity and a challenge to reshape the cities and increase their infrastructures' efficiency and resilience. However, the concept of infrastructure resilience has a different meaning when considering MSEs; the concept is extended to ensure meeting the strict minimum performance requirements necessary for event success. Even the term “success” here has a different meaning and can be considered to be promoting a positive image about the nation's capabilities and potential and securing a permanent boost to the tourism sector (Meza Talavera et al., 2019). In other words, during an MSE, the resilience of some systems should be evaluated simultaneously and at different levels; the evaluation should consider the critical elements and the overall network performance to ensure that the cities' resilience meets a satisfactory level.
Evaluating city resilience by expanding the assessment process to consider multiple interconnected and dependent urban infrastructures is a highly complicated and challenging goal. Urban infrastructures (such as electrical, water supply, and transportation) are essential for the development of any urban settlement in the modern age, and the integration of their services raises their efficiency and is critical for any city to thrive (Johansson et al., 2012; Al-Saidi and Saliba, 2019). However, this integration of an interdependency between various infrastructures also form a source of threats, considering the possibility of failure propagation (Ghalavand et al., 2014; Mohebbi et al., 2020). Furthermore, each critical infrastructure has unique characteristics, suitable metrics, and consequently appropriate assessment approaches. All these variables and challenges mentioned above limit the ability to develop a straightforward holistic assessment method that can be scaled to accommodate different networks simultaneously with reasonable accuracy. To address this challenge, we present our suggested solution based on multi-criteria decision analysis concepts, using partial-factors approaches to integrate the assessment results of different infrastructures into a unified index for MSEs.
Methodology
A city can be seen as “a system of systems,” where various critical and supporting infrastructures contribute to the overall functionality and resilience. Each of the urban infrastructures has its unique nature and serves a different role in the city, contributing to the functionality of other infrastructures either directly or indirectly and forming a complex network of relationships, which characterize modern cities and improves their efficiency (Liu and Song, 2020). Furthermore, in many cases, these infrastructures have physical proximity, and therefore damage or adverse event could simultaneously affect several. This complexity, the interdependencies, and the proximity exploit the vulnerability of infrastructures and facilitate the propagation of failure from one to another, thus limiting the effectiveness of the resilience assessment approaches (Mohebbi et al., 2020). Moreover, the variation in metrics, type of service, and nature makes a direct and unified assessment approach impossible.
The suggested formula (Equation 1) is based on converting the results of infrastructure resilience assessments into a single index, allowing comparability between different development plans according to policymakers' preferences, thus facilitating the success of MSEs. This conversion is conducted through multiple partial factors that address the variations, such as evaluation methods and metrics, interdependencies, and the evaluation's focus or context.
where
MSERI: normalized Mega Sports Events Resilience Index for the whole city by summing different infrastructures indexes
Mi: resilience assessment method efficiency factor used to evaluate infrastructure i
Ii: infrastructure i importance factor
Ri: normalized infrastructure i resilience assessment result
αij: interdependency factor of infrastructure i due to disturbance in infrastructure j.
Figure 1 shows the complete flow diagram of the process.
Resilience Assessment Method Efficiency Factor (Mi)
The variation in assessment methods and metrics combined with each infrastructure's difference necessitates this conversion. The suggested approach to addressing this issue is based on reflecting on previous events and disasters or simulations to evaluate the efficiency of the method used. The reflection results for multiple events are then used as inputs into Monte Carlo analysis for the whole Equation (1), thus avoiding the trap of generalization. This step ensures the representativeness of the results for the actual city infrastructure performance and facilitates the application of any suitable assessment method and metric based on available data and capacities.
To calculate this factor, we will choose a reference performance metric (Based on expert judgment), such as the percent of the completed travels during a disaster compared to the situation under normal conditions. By reflecting on previous events, we can observe the damaged links and the system resilience according to the reference index; for the sack of clarification, we will consider an event that resulted in a performance reduction equal to 20%. By applying other methods such as complex networks, which use connectivity metrics (such as betweenness), and considering the same event impacts and lost links, we can get a resulting reduction in betweenness, and thus system performance equal to 18%. By comparing the two approaches, we can observe a variation equal to 10%, and thus the efficiency of the complex network method in this event was 90%; this means we need an efficiency factor equal to (1/0.9) to convert the assessment to the reference metric. By repeating this process considering many previous events, we can get many performance efficiency factors (one for each event-method pair), creating a database from which we would pick and apply randomly (for each method) using the Monte-Carlo simulation in the equation. This process will require developing the needed databases beforehand for each infrastructure and the agreement on the reference metric each database will be built on.
Infrastructure Importance Factor (Ii)
This factor is used as a ranking tool for different infrastructures based on policymakers' preference and the technical criteria provided by experts to ensure the success of the MSE. The values of the importance factor can be introduced in two ways: using values between 1 and 0 (based on policymakers' judgment)or through a ranking of the infrastructures, which may necessitate the use of the modified equation shown in Equation (2).
where
Oi: Infrastructure (i) order in an ascending importance ranking chosen by the policymaker or the researcher.
The use of the modified Equation (2) automatically normalizes the importance factors of different infrastructures as a fraction of one.
Using the first approach would assign values that sum into a whole like (0.25, 0.25, 0.3, 0.2). In the second approach, we would order the infrastructures according to their importance ascending and calculate the MSERI using the Equation (2); in this case, if we considered the ascending ranking of the infrastructures as follow (transportation, communication, water supply, electrical network) the (indirectly) resulting importance factors would be (, , , and ), respectively.
Infrastructure Resilience Assessment Result (Ri)
Resilience evaluation results for the infrastructures should be normalized to reflect the system performance based on concepts similar to the “resilience triangle,” but not necessarily the same representation. However, the same representation should be adopted for all infrastructures in order to facilitate comparability. Furthermore, the representation should demonstrate the technical requirements, as shown in Figure 2, to allow the discarding of any solutions that do not meet these requirements and limits.
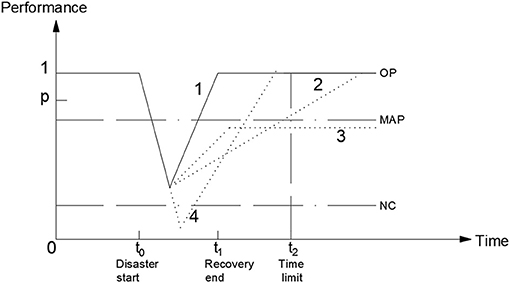
Figure 2. Resilience representation, including technical requirements. P represents the performance; OP is the optimal performance in normal conditions; MAP is the minimum acceptable performance; NC is the necessary remaining capacity limit; t0 is the time at which a disaster starts; t1 is the time at which the recovery process ends; t2 is the time limit for the recovery process as a requirement. There are four performance scenarios in the figure, and only scenario 1 fulfills the acceptance criteria.
The technical requirements during MSEs depend on the events' nature but can include the maximum transportation time for teams from training fields to the stadiums and the maximum power outage in stadiums based on the capacity of backup systems.
After converting the assessment results and reflecting them on a performance diagram, we calculate the normalized resilience using Equation (3).
where
Pi(t): the performance at time t.
The value of Ri calculated from Equation (3) is then be put directly into Equations (1) or (2). However, in the case of using a qualitative evaluation approach, a scale is defined to translate the values from words into values; for example, a “very good” performance level may be represented by Ri = 0.75. This scale should be built on experts judgment and consider the time inclusively yielding Ri directly, however, we don't recommend following qualitative approaches in the systems assessment.
Interdependency Factor of Infrastructure (αij)
Interdependency is one of the most complicated issues related to resilience due to the complexity of infrastructure relationships and the variation in nature and services among the infrastructures. However, here we suggest an approach that addresses the issue of failure propagation from one infrastructure to another without the need for a combined assessment approach. We calculate the interdependency factor using Equation (4).
where
Pij(t): the performance of infrastructure i as a result of damage in infrastructure j at time t.
The concept used here is that the reduction in the performance of infrastructure i due to damage to infrastructure j is subtracted from the normalized resilience results. This assumption means we only considering the first order of failure propagation from j to i without any reflection to other networks or the source network, as shown in Figure 3, using transportation network as an example.
This simplification allows avoiding iterative cycles of reflected damage between infrastructures, which would require whole city simulation across all infrastructures while considering various metrics and characteristics, a complex simulation that requires extensive resources and calibration process and hard to apply. Our approach aims to avoid such need by hypothesizing that the main damage would be resulting from the first order propagation.
Conclusion
A city can be described as a giant cell supported by a complex network of infrastructures. Critical infrastructures support all activities in cities and ensure that they are habitable and thrive. Simultaneously, these infrastructures are susceptible to many threats and challenges that can disrupt their services and undermine their efficiency, causing substantial economic, social, and environmental losses. The resilience of infrastructures can reduce the impacts of such threats and facilitate fast recovery. However, resilience assessment approaches face many obstacles related to variations in methods, metrics, scale, nature, and interdependencies; and therefore, it is hard to develop a holistic and direct assessment method that can reflect the city's performance under different scenarios.
Mega sports events (MSE) are an important opportunity for any nation to prove its ingenuity and potential and to create a lasting positive legacy for all aspects of sustainable development. An MSE requires considerable investments in order to mobilize the economy, to reorganize or reshape the hosting cities, and to stimulate nationwide development. However, these investments' success requires the resilience of hosting cities' infrastructures to accommodate the influx in demands and to prevent disturbances from interrupting MSE activities.
To address the complex challenge of assessing the resilience of hosting cities' infrastructures, especially during MSEs, we suggest a new equation that uses a multi-criteria approach and partial factors. The proposed equation enables the combination of assessment results for various infrastructures, regardless of their assessment method, by introducing a method-efficiency factor. Furthermore, the method introduces importance factors to allow decision-makers to rank or classify different infrastructures based on their preference. As a procedure to ensure suitability for an MSE, any arrangement or plan that does not meet strict limits is deemed unsuitable and therefore is discarded from the evaluation and requires redesign. Finally, the equation considers the failure propagation under each scenario from one infrastructure to another by subtracting the infrastructures' interdependency factors from the resilience assessment results.
The proposed equation addresses multiple challenges regarding unifying resilience assessment at the city level. Its applications can be expanded to include the city's development plans outside MSEs; by setting different service limits and goals to correlate with the city's master plan using suitable assessment methods based on the available data and budgets. Such evaluation could also be downscaled to neighborhood level to compare different ones and prioritize developments or infrastructure reinforcement plans.
This paper is expected to open discussions about the need for a holistic resilience assessment approach and pave the way for future studies within the fields of urban planning and metabolism, disaster preparedness and risk reduction, infrastructure engineering, and resilience during MSEs.
Data Availability Statement
The original contributions presented in the study are included in the article further inquiries can be directed to the corresponding author.
Author Contributions
MS and SA-G: conceptualization and methodology. MS: writing—original draft. MK and SA-G: writing—review and editing. SA-G: supervision and funding acquisition. All authors have read and agreed to the published version of the manuscript.
Funding
This publication was made possible by the National Priorities Research Program (NPRP) grant (NPRP12S-0212-190073) from the Qatar National Research Fund (QNRF), a member of Qatar foundation (QF). Any opinions, findings, and conclusions or recommendations expressed in this materials are those of the authors and do not necessarily reflect the views of QNRF or QF. Open Access funding provided by the Qatar National Library.
Conflict of Interest
The authors declare that the research was conducted in the absence of any commercial or financial relationships that could be construed as a potential conflict of interest.
References
Addanki, S. C., and Venkataraman, H. (2017). Greening the economy: a review of urban sustainability measures for developing new cities. Sustain. Cities Soc. 32, 1–8. doi: 10.1016/j.scs.2017.03.009
Ahmed, S., Dey, K., and Fries, R. (2019). Evaluation of transportation system resilience in the presence of connected and automated vehicles. Transp. Res. Rec. J. Transp. Res. Board 2673, 562–574. doi: 10.1177/0361198119848702
Al-Saidi, M., and Saliba, S. (2019). Water, energy, and food supply security in the Gulf Cooperation Council (GCC) countries—a risk perspective. Water 11:455. doi: 10.3390/w11030455
Anaut, M. (2005). Le concept de résilience et ses applications cliniques. Rech. Soins Infirm. 82, 4–11. doi: 10.3917/rsi.082.0004
Bruneau, M., Chang, S. E., Eguchi, R. T., Lee, G. C., O'Rourke, T. D., Reinhorn, A. M., et al. (2003). “A framework to quantitatively assess and enhance the seismic resilience of communities,” in Earthquake Spectra 19, 733–752. doi: 10.1193/1.1623497
Ganin, A. A., Mersky, A. C., Jin, A. S., Kitsak, M., Keisler, J. M., and Linkov, I. (2019). Resilience in Intelligent Transportation Systems (ITS). Transp. Res. Part C Emerg. Technol. 100, 318–329. doi: 10.1016/j.trc.2019.01.014
Ghalavand, Y., Hatamipour, M. S., and Rahimi, A. (2014). A review on energy consumption of desalination processes. Desalin. Water Treat. 54, 1526–1541. doi: 10.1080/19443994.2014.892837
Holling, C. S. (1973). Resilience and stability of ecological systems. Annu. Rev. Ecol. Syst. 4, 1–23. doi: 10.1146/annurev.es.04.110173.000245
Hosseini, S., Barker, K., and Ramirez-Marquez, J. E. (2016). A review of definitions and measures of system resilience. Reliab. Eng. Syst. Saf. 145, 47–61. doi: 10.1016/j.ress.2015.08.006
Huck, A., and Monstadt, J. (2019). Urban and infrastructure resilience: diverging concepts and the need for cross-boundary learning. Environ. Sci. Policy 100, 211–220. doi: 10.1016/j.envsci.2019.05.008
Johansson, J., Hassel, H., Hokstad, P., Utne, I. B., and Vatn, J. (2012). Risk and Interdependencies in Critical Infrastructures: A Guideline for Analysis. London: Springer-Verlag.
Liu, W., and Song, Z. (2020). Review of studies on the resilience of urban critical infrastructure networks. Reliab. Eng. Syst. Saf. 193:106617. doi: 10.1016/j.ress.2019.106617
Mattsson, L.-G., and Jenelius, E. (2015). Vulnerability and resilience of transport systems—a discussion of recent research. Transp. Res. Part A Policy Pract. 81, 16–34. doi: 10.1016/j.tra.2015.06.002
Meza Talavera, A., Al-Ghamdi, S., and Ko,ç, M. (2019). Sustainability in mega-events: beyond qatar 2022. Sustainability 11:6407. doi: 10.3390/su11226407
Mohebbi, S., Zhang, Q., Christian Wells, E., Zhao, T., Nguyen, H., Li, M., et al. (2020). Cyber-physical-social interdependencies and organizational resilience: a review of water, transportation, and cyber infrastructure systems and processes. Sustain. Cities Soc. 62:102327. doi: 10.1016/j.scs.2020.102327
Rus, K., Kilar, V., and Koren, D. (2018). Resilience assessment of complex urban systems to natural disasters: a new literature review. Int. J. Disaster Risk Reduct. 31, 311–330. doi: 10.1016/j.ijdrr.2018.05.015
Salimi, M., and Al-Ghamdi, S. G. (2020). Climate change impacts on critical urban infrastructure and urban resiliency strategies for the Middle East. Sustain. Cities Soc. 54:101948. doi: 10.1016/j.scs.2019.101948
Schleussner, C.-F., Donges, J. F., Donner, R. V., and Schellnhuber, H. J. (2016). Armed-conflict risks enhanced by climate-related disasters in ethnically fractionalized countries. Proc. Natl. Acad. Sci. U.S.A. 113, 9216–9221. doi: 10.1073/pnas.1601611113
Serdar, M. Z., and Al-Ghamdi, S. G. (2021). “Preparing for the unpredicted: a resiliency approach in energy system,” in Energy Systems Evaluation, Vol. 1, ed J. Ren (New York, NY: Springer International Publishing), 1–19.
Sun, W., Bocchini, P., and Davison, B. D. (2018). Resilience metrics and measurement methods for transportation infrastructure: the state of the art. Sustain. Resilient Infrastruct. 5, 168–199. doi: 10.1080/23789689.2018.1448663
The Resilience Shift (2019). The Resilience Shift Website. FAQ. Available online at: http://resilienceshift.org [accessed November 18, 2019].
The Supreme Committee for Delivery Legacy (2020). The Supreme Committee for Delivery and Legacy (Sustainability Policy). Available online at: https://www.qatar2022.qa/en/about/sustainability (accessed October 10, 2020).
Keywords: resilience assessment, mega sports events, infrastructure interdependency, multi-criteria assessment, urban infrastructure resilience
Citation: Serdar MZ, Koc M and Al-Ghamdi SG (2021) Urban Infrastructure Resilience Assessment During Mega Sport Events Using a Multi-Criteria Approach. Front. Sustain. 2:673797. doi: 10.3389/frsus.2021.673797
Received: 28 February 2021; Accepted: 07 May 2021;
Published: 03 June 2021.
Edited by:
Ruojue Lin, Hong Kong Polytechnic University, Hong KongReviewed by:
Emanuele Pagone, Cranfield University, United KingdomXiaoping Jia, Qingdao University of Science and Technology, China
Copyright © 2021 Serdar, Koc and Al-Ghamdi. This is an open-access article distributed under the terms of the Creative Commons Attribution License (CC BY). The use, distribution or reproduction in other forums is permitted, provided the original author(s) and the copyright owner(s) are credited and that the original publication in this journal is cited, in accordance with accepted academic practice. No use, distribution or reproduction is permitted which does not comply with these terms.
*Correspondence: Sami G. Al-Ghamdi, salghamdi@hbku.edu.qa