- 1Department of Planning, Aalborg University, Aalborg, Denmark
- 2Technology Assessment Group, Paul Scherrer Institut, Villigen, Switzerland
Given a fixed product system model, with the current computational framework of Life Cycle Assessment (LCA) the potential environmental impacts associated to demanding one thousand units of a product will be one thousand times larger than what results from demanding 1 unit only – a linear relationship. However, due to economies of scale, industrial synergies, efficiency gains, and system design, activities at different scales will perform differently in terms of life cycle impact – in a non-linear way. This study addresses the issue of using the linear framework of LCA to study scalable and emerging technologies, by looking at different examples where technology scale up reflects non-linearly on the impact of a product. First, a computer simulation applied to an entire database is used to quantitatively estimate the effect of assuming activities in a product system are subject to improvements in efficiency. This provides a theoretical but indicative idea of how much uncertainty can be introduced by non-linear relationships between input values and results at the database level. Then the non-linear relations between the environmental burden per tkm of transport on one end, and the cargo mass and range autonomy on the other end is highlighted using a parametrized LCA model for heavy goods vehicles combined with learning scenarios that reflect different load factors and improvement in battery technology. Finally, a last example explores the case of activities related to the mining of the cryptocurrency Bitcoin, an emerging technology, and how the impact of scaling the Bitcoin mining production is affected non-linearly by factors such as increase in mining efficiency and geographical distribution of miners. The paper concludes by discussing the relation between non-linearity and uncertainty and by providing recommendations for accounting for non-linearity in prospective LCA studies.
Introduction
The matrix-based computational structure of Life Cycle Assessment (LCA) is well-described in literature (Heijungs and Suh, 2002) and this framework is often presented as linear, even though the term linear can be interpreted in several ways (Heijungs, 2020). One interpretation is that, mathematically, product systems scale up linearly. This interpretation is based on the fact that, given a fixed product system model, the potential environmental impacts associated to demanding one thousand units of a product are one thousand times larger than results from demanding 1 unit only. The key assumption in such a linear LCA framework is that each activity in the product system will maintain a constant ratio between inputs and outputs, no matter how much product is demanded.
Yet real-world systems are more complex than this and do not follow such a linear trend. Due to economies of scale, industrial synergies, efficiency gains, and system design, activities at different scales and technological maturity will perform differently and display different output to input ratios (Caduff et al., 2011). Their life cycle impact will also be different (Caduff et al., 2012, 2014).
In these cases, the impact per unit of product output will be different between a small-scale system, technology, or facility S and a larger one S′ that is upscaled and can potentially produce a quantity of product that is – for example – thousands of times higher. While the functional unit of the LCA remains the same, as it is always the impact per 1 unit of product that is calculated, the system used to determine the impact associated with this functional unit is different. Mathematically, the LCA matrix algebra remains unchanged, and even in the new upscaled system, S′, calculating the impact of 1,000 units will return a result that is 1,000 times higher than the impact of producing 1 unit. However, the impact of 1 unit produced with this upscaled system S' is not necessarily the same as the impact of producing 1 unit with the original small scale system S. Thus, while non-linearity with respect to the production output is an intrinsic property of the system under analysis, linearity with respect to the functional unit is an intrinsic property of the LCA model used to study this system. This is precisely the mismatch that the present study intends to address.
This mismatch between model and reality becomes critical and potentially problematic in the study of technology upscaling, and particularly in the case of emerging technologies. For example, upscaling effects for green technologies are substantial and well-documented (Grubb, 2004; Piccinno et al., 2016; Nemet et al., 2018) and can lead to increases in efficiency and reduction in the impact per output ratio for a specific activity. It is notably the case with renewable energy systems, where the initial environmental burden of manufacture spreads as the load factor of the plant increases (Padey et al., 2013; Miotti et al., 2017).
This effect is then particularly evident for emerging technologies in which data are available only on a pilot scale, and it is realistic to expect substantial improvements when reaching industrial scale. With emerging technologies, a massive increase in production volumes lead to reduction in the environmental burden thanks to economies of scale and technological learning (Piccinno et al., 2016; Sacchi et al., 2019). Miotti et al. (2017) illustrated such a case, where the environmental impact of hydrogen fuel cell stacks is reduced by two thirds as the production volume increases from 200 units/year in 2014 to 500,000 units in 2030.
It is worth noting that the upscaling challenge and potential gain is highly technology-specific. For example, the upscaling of emerging technologies for the treatment of biomass are challenged by the need to work continually and keep controlled physical-chemical conditions but can benefit from synergies such as for example heat recovery aspects that are not appreciable at pilot scale. Another example is the mass-production of complex new technologies such as fuel cells that depend heavily on automatization and robotics and are substantially different from the manual work of manufacturing these technologies in small quantities.
Thus, modeling the upscaling of emerging technologies goes beyond the sole use of upscaling relationships. In their recent review Tsoy et al. (2020) list several data estimation methods relevant in this context such as process simulation, manual calculations, molecular structure models (only for chemical technologies), and use of proxies. According to these authors, a framework to create LCAs of new technologies at scale includes collaborating with technology experts to define hypothetical upscaled scenarios. This mirrors previous findings of Arvidsson et al. (2017) who also recommend modeling various scenarios using literature, expert interviews, simulation software, and a combination of these methods.
While current research on the LCA of emerging technologies (Valsasina et al., 2017; Bergerson et al., 2019; Blanco et al., 2020) deals indirectly with non-linear effects, the non-linear relations between technological upscaling and life cycle assessment has not been explicitly covered in the literature.
As already pointed out by Heijungs (2020) the impact of a product system on a small interval looks like a straight line, but on a larger interval it becomes non-linear. What remains unaddressed is on a practical level why, where, and how much this is a problem.
In this context, the main objective of this article is to present and discuss different cases of LCAs of product systems that do not scale up linearly. The study intends to show the diversity of potentially non-linear cases to derive more general considerations on how extensively non-linearity can become a problem in the LCA of scalable and emerging technologies as well as to propose possible ways to address this problem.
Methods
This work is based on the analysis of different case studies. The cases are illustrative and were selected because they allow the problem of non-linearity in scalable and emerging technologies to be addressed specifically. The cases were also chosen in order to cover different levels of complexity and various types of uncertainty. Finally, the cases were chosen pragmatically based on data directly available from previous and current research work of the authors on scalable and emerging technologies. All cases were analyzed using the ecoinvent database (Wernet et al., 2016) with different versions and system models depending on the case, cf. Supplementary Table 3. The impact of the different systems under analysis was characterized using the midpoint impact indicator Global Warming measured in kg of Carbon Dioxide equivalents (kg CO2-eq) using the IPCC 2013 method with a time horizon of 100 years (IPCC, 2013). The analysis was performed using the open source software Brightway2 (Mutel, 2017) unless specifically indicated. Code and data used for the analysis can be openly accessed at an online repository (Pizzol et al., 2020).
Estimating the Effect of Improvements in Technology Efficiency at Database Scale
The first case explores how efficiency gains that are theoretically achievable via technology upscaling result in changes in impacts, by taking a whole life cycle inventory database as unit of analysis. The hypothesis is that data used to build a database bottom-up via industry surveys — like in the case of ecoinvent (Wernet et al., 2016)— may not always reflect technologies at their highest readiness level or technological maturity. In other words, the datasets representing some activities might have been collected from a plant or facility that does not operate at large industrial scale and is therefore far from achieving its potential maximum efficiency. The data supplier might thus have measured an exchange in a process that is far from maximum efficiency.
The challenge in studying the effect of this inaccuracy at database scale is then two-fold. On one hand, it is virtually impossible to know in detail which flows can be improved and where the upscaling uncertainty lies. It then becomes necessary to assume an upscaling uncertainty for each exchange in each activity, intended as a probability of being wrong about the efficiency of this activity regarding the input exchange. On the other hand, it is reasonable to expect that the effect of improving the efficiency of one or more activities in the database would be rather different depending on the activity that is considered for the analysis, meaning the functional unit for which the life cycle impacts are calculated.
To tackle these challenges, a computer simulation was performed where the change in the environmental burden of a sample of activities from the ecoinvent database was measured after increasing the efficiency of a number of other activities, thus simulating an improvement that is potentially achievable via technology upscaling or learning effects. Mathematically, the efficiency improvement in an activity is here achieved via a reduction c in the amount of technosphere or biosphere input x required to obtain the production output y.
The idea was then to simulate this efficiency improvement as realistically as possible at whole database scale to provide a theoretical but indicative idea of how much uncertainty may be introduced by non-linearities associated with technology upscaling.
The ratio r was calculated by defining h as the impact vector, Q as the characterization matrix, B as the intervention matrix, A as the technology matrix, and f as the demand vector (Heijungs and Suh, 2002).
Where h was taken as the “base” value of the impact of an activity before changing its efficiency.
And h′ was obtained by improving the efficiency of specific activities in the database. This is further explained in the following, by using different examples of increasing complexity.
The first simple example measured the effects of progressively increasing the efficiency of coal power plants in the production of electricity. This example allowed working under rather controlled conditions before dealing with the intrinsically high variability of the entire database, due to the fact that the database includes several types of activities.
Initially, the improvement was only modeled in terms of a reduction in energy and material inputs. This means that only the values in the technosphere matrix were changed to obtain a new technosphere matrix A′ and calculate h′:
Where the element of A′ was obtained as:
Where aj is any matrix column representing coal-based electricity production, and all coal-based electricity production activities in the database were simultaneously modified. c is the fixed coefficient of efficiency improvement. Note that A is assumed square with diagonal values equal to 1. A more visual example is provided below considering the technology matrix A where each unit process j has production output qjand technosphere inputs xi,j and where both columns aj=1 and aj=2 represent coal power plants:
A′ is obtained by multiplying the off-diagonal values of column aj=1 and aj=2 by the same coefficient of efficiency improvement. In this upscaled system the production output is denoted and technosphere inputs are denoted . The assumption is then that is not just proportional but more efficient than proportional:
This allows to obtain:
This simple example was then extended to additionally consider the improvement in terms of emission reduction together with the reduction in material and energy requirements. This means that h′ values were this time obtained by changing both the values in the technosphere matrix and the values in the intervention matrix.
Where the element of A′ is obtained as in Equation (4.1) and the element of B′ is obtained as:
Where aj and bj are the columns representing coal-based power plants in each matrix. More visually, considering the intervention matrix B where each unit process j has production output qj and environmental exchanges zi,j and where both columns bj=1 and bj=2 represent coal power plants, then:
The improvement is again described as an increase in efficiency:
To obtain the upscaled intervention matrix B′:
The simulation consisted in calculating r values (Equation 2) using Global Warming impact values obtained via Equations (3–5) for 10 randomly selected activities (10 different f) in the database and nine progressively increasing values of c ranging from 0.2 to 1.0 in 0.1 increments to cover a theoretical efficiency increase up to 400%.
This simple example allowed a clear understanding of the relationship between efficiency increase and impact. It did not, however, allow conclusions to be drawn that were generalizable at a whole database level. Thus, a more complex example was introduced by measuring the effects of more random and widespread improvements in the efficiency of different activities in the database.
The approach presented in Equations (3–4.4) was upscaled to database level by simultaneously modifying all transformation activities in the ecoinvent database. In principle, efficiency gains can only be observed in transformation activities, as opposed to market activities which only represent the combined supply of similar products based on trade statistics. Again, at first only the technosphere exchanges were modified and h′ calculated as in Equation (4), but this time was obtained as:
Where aj is any matrix column representing a transformation activity, and all transformation activities in the database were simultaneously modified. Ck is again a coefficient with value between zero and one (0 < Ck < 1) representing efficiency improvements, but as opposed to c that was fixed, Ck was instead randomly sampled from a specific probability distribution.
The following presents a visual example of how the A′ matrix was obtained in this more complex simulation, assuming that columns aj=2 and aj=4 of A are both transformation activities and c1, c2, c3, … are randomly sampled instances of Ck (i.e., randomly sampled coefficients).
Once again, this example was further extended to consider both improvements in terms of reduced material and energy requirements and in terms of emission reductions. Thus, was obtained as in Equation (4.6) and as:
This operation was performed on all bj columns representing transformation activities and using randomly generated coefficients as from Equation (5.5). Thus, the way B′ was obtained in was similar to Equation (4.6) with the only difference that all values in the columns bj=2 and bj=4 are multiplied by a coefficient and not only the off-diagonal ones.
The simulation at entire database level consisted in performing the operations described in Equations (4.5, 5.5) repeatedly 1,000 times, randomly sampling different coefficients at each iteration, and then calculating r values via Equation (2) using Global Warming impact values obtained via Equations (3–5) for 50 randomly selected market activities (fifty different f) in the database. In order to provide clearer results, treatment and waste management activities were excluded from the selection as these can return negative results and complicate the interpretation. The result was a matrix of 1,000 × 50 r values where rows represented iterations and columns represented the market activities under analysis. Moreover, this simulation was performed in four different scenarios obtained by using two versions of the same database and two probability distributions of Ck to appreciate the differences due to modeling choices and assumptions. In particular, both the consequential and cutoff version of the ecoinvent v.3.6 database were used and both the beta distribution Ck ~ Beta (α, β) with α = 5 and β = 1, and an uniform distribution Ck ~ U (0, 1).
The reason for choosing a beta distribution, which resembles an exponential distribution between zero and one, is that it can be considered a suitable model for the random behavior of percentages and proportions. As the specific exchanges that can potentially be improved are unknown to the authors, it was assumed that most exchanges are already close to maximum efficiency. The Beta distribution with the selected values allows modeling this assumption, i.e., the probability to sampling a value close to 1 is higher than the probability of sampling a value close to 0. Using a beta distribution was a middle ground between using the same efficiency gain for all input exchanges of an activity and completely randomly selecting efficiency from a uniform distribution between zero and one — which was also performed for the sake of comparison.
The reason for performing the simulation on two versions of the ecoinvent database was that the consequential system model adopts system expansion as a method to solve multifunctionality, resulting in a number of activities being associated with a (mathematically speaking) negative impact. This produces r values >1 and can skew the distribution of results to the right. The comparison with the cutoff version allowed appreciating this additional factor of variability.
Non-linearity in Upscaling Services, the Case of Freight Transportation
The first case was theoretical, and only considered potentially achievable changes in the value of specific flows while not explaining how in detail these changes might manifest.
To show more concretely how a specific technology might display a different behavior at different scales a second case was chosen that considers the transportation of goods. Transportation systems are a case where a non-linear behavior in upscaling can be observed. This can be exemplified with freight transportation where fluctuations of the load factor or fluctuations in the vehicle size and carrying capacity can affect the environmental burden per ton transported in a non-linear manner (Rizet et al., 2012; Pizzol, 2019).
This case provides two examples on how technology upscaling and technology improvement affect the environmental performance of transportation via heavy duty trucks in a non-linear manner. The first example of a 40-ton diesel truck was considered to highlight the non-linear relation between the load factor of the system and its environmental performance per tkm of transportation. The second example of a battery electric truck was used to highlight the non-linear relation between the driving range autonomy and its environmental performance per tkm. These models allow accounting for the variability in the operating conditions of two different transportation technologies at different scales.
The parametrized LCA model for heavy goods vehicles carculator_truck (Sacchi et al., submitted) was used for this case. The tool models trucks of various powertrain types (i.e., internal combustion engine, battery electric, fuel cell electric) and sizes (i.e., from 3.5 to 60 t of gross weight), across time (i.e., from 2000 to 2050), and for different duty cycles (i.e., urban and regional delivery, long haul). Further information is available from the online documentation of the library1 including a detailed description of data sources and modeling assumptions. The library uses the cutoff system model of the ecoinvent v.3.7 database to model the supply of material, services, and energy.
The first example, highlighting the effect of technology upscaling, focused on assessing the non-linear relation between the load factor of a truck and its global warming impact per tkm. To do that, a 40-ton articulated curtainside truck with a diesel engine and a lifetime of 1 million km was modeled with a load factor ranging from 0 to 100% with a 1% increment step. For each increment in the load factor, the vehicle components and drivetrain were sized, after which the tank-to-wheel energy consumption of the vehicle was calculated given a specific driving cycle.
In this case, the driving cycle chosen reflects long haul operations. The tank-to-wheel energy consumption entails the energy needed to overcome different types of resistance, such as the inertia of the vehicle itself, the rolling resistance, the aerodynamic drag, the road gradient, as well as resistance in the transmission shaft and the engine. The curb mass mc [t] of the vehicle was obtained as being the sum of the components' mass, including the energy storage mass me, but excluding passengers and cargo (Equation 6). The available payload mP is the difference between the gross mass mg of the vehicle and its curb mass (Equation 6.1). When the vehicle is “built,” its material and energy inventory is solved. Such inventory contains all the relevant life cycle phases of the vehicle, including its manufacture, maintenance, use and end-of-life. The life cycle Global Warming impact per tkm ht [kg CO2-eq/tkm] is obtained by dividing the total life cycle carbon emissions of the vehicle hl [kg CO2-eq] with the number of kilometers driven l [km] and the payload transported, which is itself the product of the available payload mp and the load factor r [without unit] (Equation 6.2).
A second example, highlighting the effect of technology improvement, focused on assessing the non-linear relation between range autonomy (the distance a truck is required to drive without refueling) and the truck's global warming impact per tkm in 2020 and 2050. The analysis followed a similar approach as in the first simulation but considered a 40-ton articulated truck powered by an electric powertrain instead of a diesel engine. In this case, the energy storage mass me was sized based on the required range autonomy a [km], tank-to-wheel energy consumption of the vehicle Ew [kWh/km], the depth of battery discharge b [without unit] and the energy density of the battery cells d [kWh/kg] (Equation 6.3). As the range autonomy increases, the mass of the energy storage increases as well, reducing the maximum payload available by an equivalent amount. This can however be compensated by an increase in the battery cell energy density. It is worth noting that unlike diesel powertrains, a part of the energy used for braking during downhill or decelerating sections of the driving cycle is recovered here using the electric motor. Also, as a new curb mass is defined, the tank-to-wheel energy consumption of the vehicle needs to be re-calculated, which itself redefines a new energy storage mass and curb mass. Such process stops when the curb mass of the vehicle converges.
The energy storage mass was modeled using lithium-ion batteries based on a nickel manganese cobalt chemistry. It assumed a battery cell energy density of approximately 0.2 kWh per kg of cell today, increasing to 0.5 kWh per kg in 2050 (Ding et al., 2019) and a depth of discharge of 20%. Material and energy inventories were solved for a required range autonomy of 100 to 1,200 km, by increment step of 100 km. As with the diesel truck, the resulting carbon emissions were normalized to a tkm by dividing the overall burden successively by the number of kilometers driven along the use phase of the vehicle and the cargo mass transported — see Equation (6.2).
Non-linearity in Upscaling Networks, the Case of Bitcoin Mining
A key feature of the previous cases is that while the performance of the product system is different at different scales due to improvements, the fundamental structure of the system does not change. More concretely, the structure of the inventory model remains the same and only the values of its exchanges change. However, there are situations where the upscaling of a technology might result in a structural change of the product system itself.
To account for this type of change, the third case considers the mining of Bitcoin as emerging technology and explores the non-linear effects of expanding its mining network. This builds on previous research (Köhler and Pizzol, 2019) that considers a model of the network of Bitcoin miners as a snapshot of the year 2018. In rather simplified mathematical terms, in this model the life cycle impact hm [kg CO2-eq/TH] associated with mining is given by the product of the horizontal vector sl [without unit] representing the share of mining performed in each location with the vertical vector hl [kg CO2-eq/kWh] representing the life cycle impact of electricity production in each location, and the energy consumption Em [kWh/TH] of the machines (special computers) used for mining.
In turn, the energy consumption is a function of the hash rate p [TH/s], and the power P [W] of the machine.
This product system does not scale linearly, which is mainly due to two factors: the new mining equipment employed is more energy efficient than the average equipment for 2018, and new mining capacity is not installed proportionally in current locations and is even installed in new locations.
To address this upscaling issue, previous research (Köhler and Pizzol, 2019) adopted a consequential approach and attempted to provide an outlook of the Bitcoin mining network upscaling for early 2019. Since then, however, the hashrate of the Bitcoin mining network has increased and both mining efficiency and miner locations have continued to change. This study considers new upscaling scenarios for early 2020 that allow a comparison with the upscaling scenarios of early 2019 taken from the previous study. The upscaling scenarios consider changes in location of miners and energy efficiency of the mining equipment.
A baseline business-as-usual (BAU) scenario was first obtained (Equation 7). This BAU scenario illustrated linear growth and was taken as reference against which all other scenarios were compared. The same prospective model for early 2019 as in the previous study (Köhler and Pizzol, 2019) was used in the calculation. The result is the Global Warming impact for increasing computing demand by one tera hash (TH).
Scenario 1 represented instead a location-sensitive scenario where new mining facilities are only installed in more competitive conditions (e.g., lower energy prices). In this scenario, the impact of the upscaled system was calculated as:
Scenario 2 represented then an equipment-sensitive scenario, where only more efficient mining equipment was used, intended as equipment that uses less energy in mining (E′ < E).
Finally, Scenario 3 assumed both more efficient mining equipment and more competitive mining locations.
For the BAU scenario and Scenario 2, which were not location-sensitive, the share of mining in each location sl was modeled using the distribution reported in Table 1. The data was taken from the Cambridge Bitcoin Electricity Consumption Index for September to December 2019 (Cambridge Centre for Alternative Finance, 2020). Only locations that contributed at least 2% (rounded) were included. The percentages were then scaled to 100%. For the location-sensitive Scenarios 1 and 3, the share of mining in each location reported in Table 1 was used. The data shows the difference from miner distribution between Sept-Dec 2019 and January–March 2020s (Cambridge Centre for Alternative Finance, 2020). Those locations where an increase in shares compared to September–December 2019 has occurred were included. Their shares were then scaled to 100% representing the marginal mining locations.
Details on the parameters used to model the material and energy requirements of the mining equipment in the different scenarios are provided in Table 2. For the BAU scenario and Scenario 1, data regarding the amount of equipment still in use was taken from “The Bitcoin Mining Network–December 2019 Update” (Gibbons and Bendiksen, 2019). The specifications for each machine were taken from the homepage ASIC Miner Value (ASIC-MinerValue, 2020) and a mining equipment mix for 2019 was modeled from these data sources. For the equipment-sensitive scenarios, the mining equipment that was still profitable and already produced by the beginning of 2020 was identified. A mining equipment mix for Scenario 2 and Scenario 3 was then determined based on the share of equipment in terms of profitability. Additional details on the methodology used to derive the mining equipment mixes are provided in Supplementary Tables 1, 2.
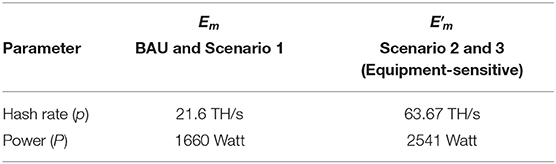
Table 2. Parameters used to model the energy consumption and of the mining equipment in the different scenarios.
Results
Non-linearities in Efficiency Improvements at Large Scale
Results reported in Figure 1 show clearly how a progressive increase in efficiency of electricity production in coal-based power plants results in a non-linear decrease in impact for several activities. The effect of such improvement, that one could theoretically ascribe to generic technological learning, is not equally pronounced for all activities under analysis, as this depends on the direct and indirect upstream inputs of electricity produced from coal to these activities. Supplementary Figure 1 shows the similar effect obtained by performing the analysis on a different database system model.
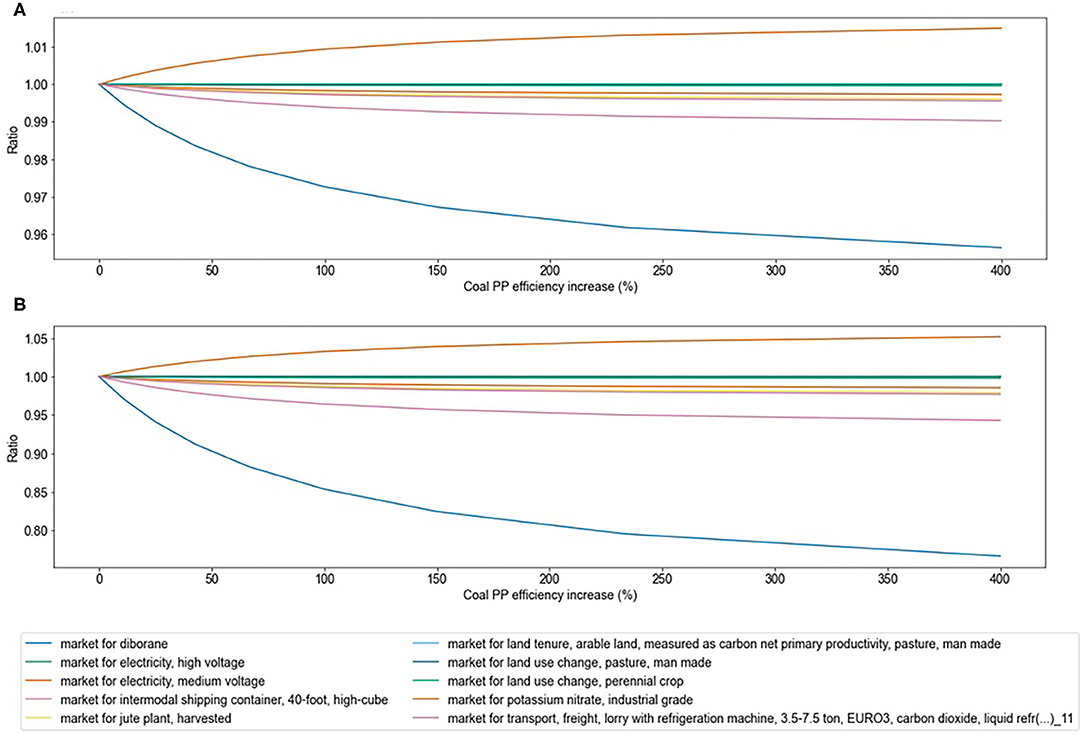
Figure 1. Effect of improving from 0–400% the efficiency of electricity production in all coal-based power plants datasets of the ecoinvent database (consequential) on the Global Warming impact calculated for 10 randomly selected activities from same database. The effect is expressed as the ratio between the impact of the improved dataset and the impact of the unmodified dataset: values closer to 1 indicate a weaker effect. (A) shows the effect of improving only technosphere exchanges, while (B) the effect of improving both technosphere and biosphere exchanges.
Results reported in Figures 2, 3 show how a random change of efficiencies for several transformation activities is reflected on the impact of several randomly selected database activities. This effect is highly dependent on the activity under analysis, and no clear relationship can be identified between the change in efficiency and its effect. While the effect can be explained for single activities it is not generalizable in a straightforward way at database level, as each activity will behave differently and might be affected substantially even if the change occurs several steps upstream in its life cycle. Boxplots for each of the 50 functional units considered are provided in Supplementary information, Supplementary Figures 2–5.
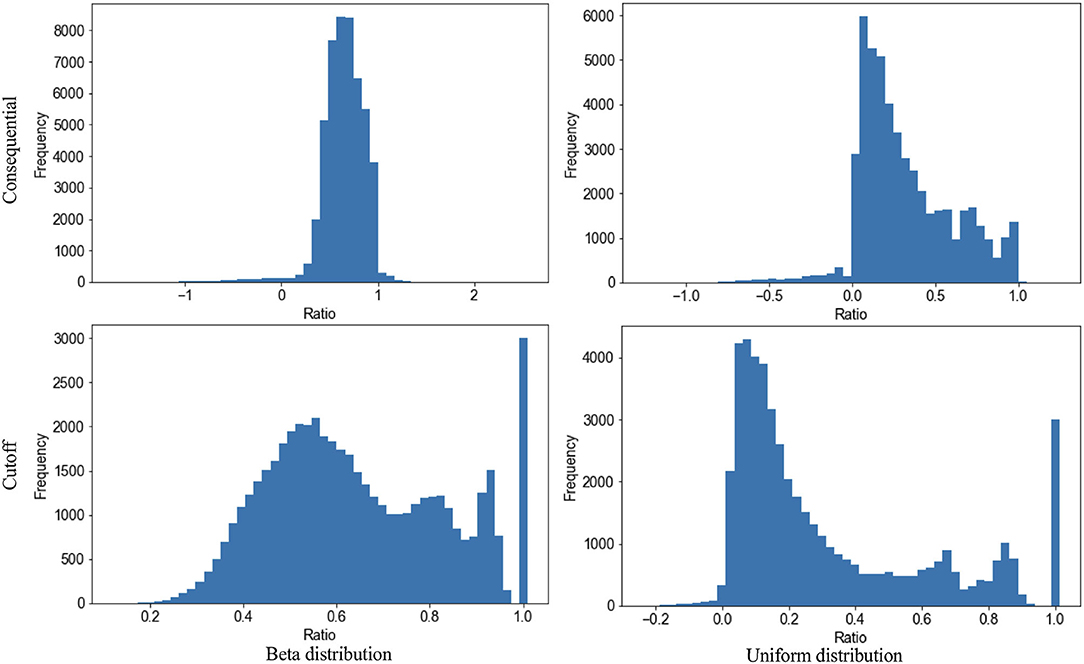
Figure 2. Effect of efficiency improvements at database scale. The effect is obtained by randomly modifying the efficiency of all transformation activity datasets in the ecoinvent database and calculating the global warming impact for 50 randomly selected market activities. The effect is expressed as the ratio between the impact calculated using the improved datasets and the impact calculated using the unmodified dataset: values closer to 1 indicate a weaker effect. The efficiency improvement is performed on the technosphere matrix only and the values of the efficiency improvement are randomly selected using a uniform and beta distribution, respectively, and in both the cut-off and consequential system models of the ecoinvent database.
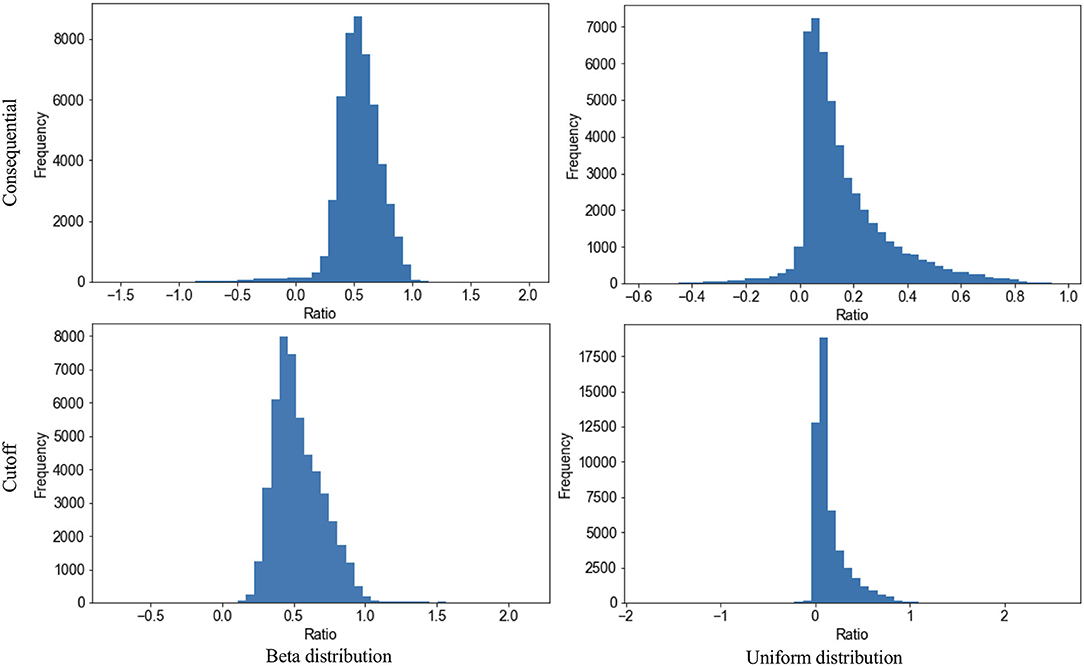
Figure 3. Effect of efficiency improvements at database scale. The effect is obtained by randomly modifying the efficiency of all transformation activity datasets in the ecoinvent database and calculating the global warming impact for 50 randomly selected market activities. The effect is expressed as the ratio between the impact calculated using the improved datasets and the impact calculated using the unmodified dataset: values closer to 1 indicate a weaker effect. The efficiency improvement is performed on both the technosphere and biosphere matrices and the values of the efficiency improvement are randomly selected using a uniform and beta distribution, respectively, and in both the cut-off and consequential system models of the ecoinvent database.
It is important to focus on the comparison between the random uniform sampling and the random beta sampling of efficiency improvements. The interesting aspect is that none of the distributions in fact resembles the distribution of the efficiency improvements. In other words, the distribution of the output does not reflect the distribution of the input, as it would be expected if the effect was linear. This confirms once again that the effect of technology upscaling on the impact of a system is non-linear.
A further note should be added on the comparison between databases and how the high variability in the type and nature of activities considered affects the results. In Figure 2, in all distributions and database versions, a small number of invariant activities can be observed (the ratio between base and simulation result close to one). These are activities that are not affected substantially by a change in efficiency for transformation activities; for example, “market for land tenure, arable land, measured as carbon net primary productivity, perennial crop” and “market for electricity, high voltage.” This might be due to several reasons, for example because they link to transformation activities that do not include any technosphere exchange or that only include biosphere exchanges that do not contribute to the Global Warming impact category. The comparison between Figures 2, 3 allows appreciation of this additional variability in the database.
Furthermore, when using the consequential system model of the ecoinvent database, ratios higher than one can be observed. This is explained by the fact that the substitution (system expansion) method is used to solve multifunctionality and therefore a number of activities return a negative impact. For these, an increase in efficiency as modeled in this simulation results in an increase in net impact.
Non-linearities in The Impact of Freight Transportation
As expected, total carbon emissions increase as the utilization rate of the available payload increases. As shown in the left panel of Figure 4, this corresponds to the minimum emission over the entire lifetime of the 40-ton diesel vehicle of 1 million kg of CO2-eq. with a load factor of 0%, to 1.6 million kg of CO2-eq. for a load factor of 100% (i.e., the transport of 25 t of cargo). On a per tkm basis, the first ton transported has a Global Warming impact of 0.6 kg CO2-eq., against 0.06 for the 25th ton, as shown in the center panel of the same figure. Hence, the assumed initial load factor is important in determining the environmental burden of a ton transported over 1 km.
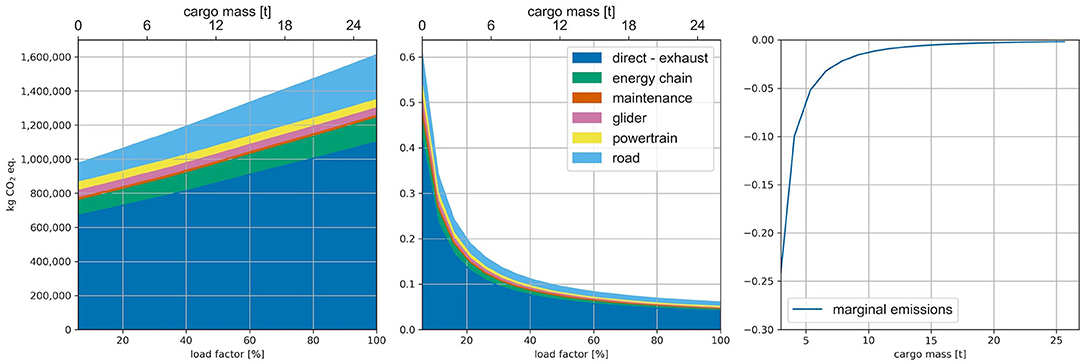
Figure 4. Upscaling scenarios for transportation with a 40-ton diesel truck. Left: Total Global Warming impact in kg CO2 -eq as a function of the capacity utilization. Center: Global Warming impact in kg CO2 -eq/tkm as a function of capacity utilization. Right: Change Global Warming impact in kg CO2 -eq/tkm as a function of cargo mass.
Similarly, it appears clear that transporting 1 ton of cargo (which corresponds to a load factor of about 40%) yields a different result than transporting 10 times 1 ton of cargo.
The right panel in Figure 4 shows the change in carbon emissions on a tkm basis associated with adding an extra ton of cargo, given an initial amount of cargo already loaded. For example, adding 1 ton of cargo with an initial load of 5 tons reduces the impact per tkm by about 0.05 kg CO2-eq. On the other end, past an initial load of 15 t, the benefits of adding an additional ton on the per tkm impacts become comparatively negligible.
In the second case, where the range autonomy of a 40-ton battery electric truck is incremented by steps of 100 km, another trend is observed. As the range autonomy increases, the available payload decreases because of the mass increase of the energy storage components. This effect is different for the cases of 2020 and 2050, due to improvements in the energy density of battery cells. On the left panel of Figure 5, a required range autonomy of 100 km with a truck in 2020 allows to transport 25 t of cargo for 0.95 million kg of CO2-eq., while a range autonomy of 1,000 km only allows transporting 10 t of cargo for a total emission of 2.2 million kg of CO2-eq. The dark blue area, which represents kg of CO2-eq. emissions associated to electricity supply, and indirectly, energy consumption, does not vary much as the range autonomy increases. This is because the driving mass of the vehicle does not increase despite the energy storage becoming voluminous, as the cargo mass diminishes. It also explains why the emissions associated to the road manufacture and maintenance remain constant, as they are scaled on the vehicle mass. This indicates a certain limitation of battery electric trucks for long distance trips.
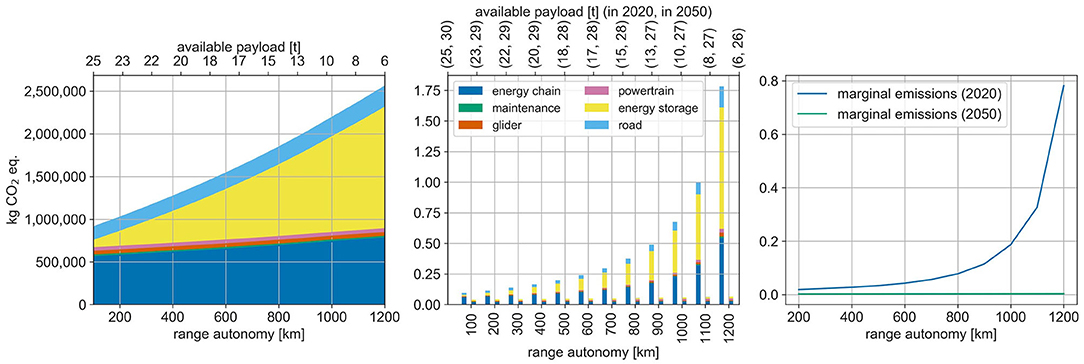
Figure 5. Upscaling scenarios for a 40-ton battery electric truck Left: Total Global Warming impact in kg CO2 -eq as a function of vehicle range autonomy in 2020. Center: Global Warming impact in kg CO2 -eq/tkm as a function of vehicle range autonomy in 2020 and 2050. Right: marginal Global Warming impact in kg CO2 -eq/tkm as a function of vehicle range autonomy in 2020 and 2050.
This pattern is equally illustrated in the central panel of Figure 5, where the performance per tkm of both trucks (2020 and 2050) is illustrated. Transporting 1 ton of cargo with a truck designed with a range autonomy of 1,200 km yields a different result per tkm than transporting the same ton of cargo with two trucks consecutively designed to have an autonomy of 600 km each. However, improving the energy density of battery cells by a factor of 2.5 between 2020 and 2050 yields to improvements far superior to a factor of 2.5 as the range autonomy increases. This is explained by the fact that the truck in 2050 would only reduce its payload capacity by 4 t to increase the autonomy range from 100 to 1,200 km, against 19 t for the truck in 2020.
The right panel in Figure 5 shows the change in carbon emissions per tkm from adding 100 km of range autonomy, function of an initial range autonomy, for both trucks. For example, for a 40-ton battery electric truck in 2020 with an initial range autonomy of 200 km, adding another 100 km of autonomy will only add 0.05 kg CO2-eq., to a tkm. This is to be contrasted with adding 100 km of range autonomy to the same truck with an initial autonomy of 1,000 km, where such change would add 0.2 kg CO2-eq. to the impacts per tkm. In parallel, a loss in utility is also observed as adding 100 km of range autonomy would lead to losing 2 t of payload capacity for the truck in 2020 (from 10 to 8 t), against <0.1 t for the truck in 2050. Thanks to expected improvements by 2050, increasing the range autonomy of the vehicle does not lead to marginally increasing emissions, as illustrated by the almost flat curve. This is because the battery has become by then a minor component in terms of mass, and a slight increase of its mass will not affect the driving mass or the electricity consumption of the truck in a significant manner.
Non-linearities in The Upscaling of The Bitcoin Mining Network
The results from the BAU scenario represent a linear growth of the Bitcoin mining network. However, in reality an expansion of such a network will result in changes in the geographical distribution of miners and in improvements in mining efficiency. In particular, Figure 6 shows the impact of the network under the various scenarios considered in this study. It is clear from the figure how the Global Warming impact of Bitcoin mining heavily depends on where the miners are located — thus on which electricity mix they rely on.
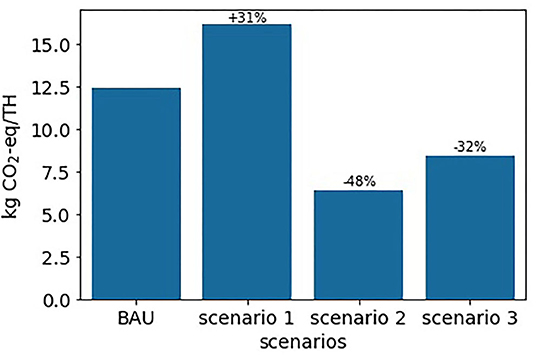
Figure 6. Global Warming impact in kg CO2 -eq/TH (Terahash) for different Bitcoin mining scenarios. BAU: Business as usual scenario (linear growth): Scenario 1: location-sensitive scenario. Scenario 2: equipment-sensitive scenario. Scenario 3: location- and equipment-sensitive scenario.
An enlargement in the Bitcoin mining network leading to miners choosing new locations results in a potential increase in Global Warming impact by 31%. The upscaling also substantially depends on the mining equipment efficiency and shows a potential decrease in impact by 48% using current projections for more efficient mining equipment.
The combined upscaling effect of both changing the geographical distribution and of using more efficient mining machines results in a net decrease in impact by 32%. Based on the historical record of increasing efficiency and varying geography of the Bitcoin mining network, it is very reasonable to assume that over time there will be an improvement in mining efficiency (TH/sec increase) and that new facilities will not be installed at every existing location. Consequently, a linear growth model that does not take into account these factors would likely return inaccurate results.
Discussion
Limitations of The Methods Used to Identify Non-linear Effects
It is important to address the validity of results both in light of the choice of methods and cases, and also how well they allow answering the research question of whether a non-linear effect can be observed for scalable and emerging technologies.
The first case is defined as theoretical because substantial simplifications were made in the simulation due to lack of information on the values of the efficiency improvement c. For example, in reality not all coal-based power plants will be improved in the same way (fixed c). Similarly, some improvements like fuel use and emission generation would be correlated and therefore using a different coefficient for each exchange of a transformation activity (randomly sampled instances of Ck) was also a simplification. These simplifications were however necessary to performing the simulation at the scale of the entire database, which was the primary purpose of the analysis.
When looking at the improvement of a specific activity such as coal-based electricity production the non-linear relation between changes in efficiency and changes in impacts is clear for single activities. The magnitude of this non-linear effect is activity-specific and therefore hardly generalizable at database level. Technological maturity is relative to time, thus the database is bound to have dated information, including when it comes to efficiency. It is, however, largely unknown to what extent the database fails to represent technologies at their highest technological maturity, and which specific flows can be improved in terms of efficiency.
When conducting the analysis at database scale, it is unfeasible to hand-pick activities or flows to selectively improve, and the stochastic approach remains the most pragmatic solution. An alternative approach could have been to selectively extract specific types of activities, for example all activities related to energy production or raw material extraction, or to specific sectors known to be highly impacting (e.g., energy, transportation, agriculture), and evaluate the effect of upscaling-related efficiency improvements in these groups. This could potentially result in more easily explainable relationships between the change in efficiency and the change in impact, but the validity of this conclusion would remain constrained by subjective selection of the groups of activities and would still remain difficult to generalize.
The choice of using a specific beta distribution for efficiency improvements is also subjective and was here presented in comparison with the choice of a uniform distribution. While assuming that all flows could be improved in any amount was considered an excessively unrealistic assumption, assuming that all flows could be potentially improved marginally seemed a more conservative and realistic one. It should be stressed that while to the best of the Authors' knowledge no information is available in literature on the observed distribution of efficiency improvements, previous studies in specific domains show that, in fact, efficiency improvements are usually of relatively contained size, e.g., 14–16% for CO2-eq/kWh from wind power (Caduff et al., 2012).
Therefore, selecting random activities within the database and changing their efficiency is beneficial to provide an indication of the potential upscaling-related uncertainty. Even if a single relationship cannot be clearly generalized for all activities, the simulation provides evidence suggesting that globally, at database scale, technological upscaling on the impact of a system is in fact non-linear.
Regarding the transportation case, several assumptions were made. In the first example, a truck with a load factor ranging from 0 to 100% was considered. In reality, the load factor for trucks in Europe is rather constant and comprised between 20 and 40% for the size considered in this study (Eurostat, 2020). Trucks with a load factor below 20% or above 50% are not highly representative of the transport market. If the average load factor is in fact constant and always within the same range of values, one may not encounter the non-linear variations that have been shown here. In the second example, the most critical assumption is probably relating to the future development of battery technology and whether the energy density of battery cells will reach the value used for the year 2050. However, even if this assumption is inaccurate, it would not invalidate the non-linear relations observed.
The model of the Bitcoin mining network includes only parameters that directly influence the environmental impacts of Bitcoin mining. Such a model is not able to reflect the emerging technology's vulnerability to outside shocks such as changes of miner revenues like Bitcoin halving (Meynkhard, 2019), or legislative changes like restricting miner locations (Alvarez, 2018) that likely would also lead to non-linear changes in environmental impacts. Changes to miner revenues directly impact which locations are profitable. Both an increase and a decrease in miner revenues caused by large Bitcoin market price fluctuations or the Bitcoin halving can influence where new mining locations are opened. Legislative changes can also be important for modeling upscaling of Bitcoin mining. China's crackdown on China-based miners in 2018 is one example of legislative changes that influenced the mining locations of Bitcoin miners (Alvarez, 2018). These factors are not included in this model but can be relevant when modeling the impacts of the Bitcoin mining network and lead to further non-linear effects.
It is important to discuss the general validity of these findings beyond the cases presented here. While it is beyond the ambition of this work to provide a comprehensive overview of all possible cases of non-linearity in the LCA of scalable and emerging technologies, these cases are exemplary as they allow appreciation of several different facets of the non-linearity problem and thus address it in its complexity. In particular, the selection covered the non-linearity due to both foreground and background modeling assumption, both theoretical and concrete examples of non-linearity (considering several activities at once with low detail vs. one activity with high detail), and the non-linearity introduced by changes in the values of a model vs. the change in the structure of a model.
On the Relationship With Uncertainty and Sensitivity Analysis
Essentially, the present work can be interpreted as a study of uncertainty and sensitivity in the LCA models referring to a specific domain: technology upscaling and emerging technologies. It is thus relevant to draw a parallel between existing research on uncertainty in LCA and the current study.
The LCA literature on uncertainty is already mature, as testified by recent remarkable contributions where advanced probabilistic techniques are used to study the uncertainty of LCA models for current technologies (AzariJafari et al., 2018) or emerging ones (Mendoza Beltran et al., 2018a; Blanco et al., 2020), as well as more theoretical contributions (Bisinella et al., 2016; Cucurachi et al., 2016; Mendoza Beltran et al., 2018b). There are different ways of defining uncertainty, and for the LCA domain Igos et al. (2019) suggest classifying uncertainty either according to its intrinsic nature - epistemic or aleatory — or according to its location in a LCA model. In the latter case, one can distinguish between uncertainty regarding the structure of the model, the quantities used in the model, or the context in which the model is used. While some techniques like stochastic simulation allow quantification of the uncertainty associated with the output of a LCA model, techniques like global sensitivity analysis allow linking it to the uncertainty of the model inputs.
The uncertainties related to location are considered more closely here as they fit well to the analysis of the cases presented in this study and are also easily linked with other literature addressing uncertainty in models more generally (Saltelli, 2008). Briefly, while quantity-uncertainties reflect the unknowns associated with the specific value associated to a model parameter or input, model-uncertainties refer to the unknowns associated with how the model operates on these quantities, intended as how the different model parameter and inputs are combined together in a structure that provides a simplified representation of reality. Context-uncertainties refer to how the context of the decision affects the LCA modeling, and while they can be significant, they are not particularly relevant in the analysis of the specific cases presented in this study.
The simulation performed at database level by increasing efficiency for specific activities could be defined as a semi-Monte Carlo approach (Heijungs and Lenzen, 2014). This analysis targeted quantity-uncertainties specifically and disregarded model-uncertainties. The approach indeed has limitations as it is reasonable to assume that when upscaling a specific activity, some inputs would be replaced by others, for example when changing the material composition of specific components of the technology or shifting from one source of energy to another. This has been observed in previous studies, for example by Blanco et al. (2020) and van der Hulst et al. (2020). The simulation performed here was not able to take this sort of model-uncertainty into account and the conclusions provided here should be interpreted considering such limitation. Changes in the number and type of inputs of an activity might lead to possibly even more non-linear effects.
The case of the transportation model focuses again on quantity-uncertainty, investigating the effect of changes in the value of two specific model parameters: load factor and range autonomy. This is not a stochastic approach but could be intended as a simple local (one at the time) sensitivity analysis (Bisinella et al., 2016) showing how variations in their value leads to non-linear effects on the model output. In this case the interesting part is that a parametrized model is used to build a foreground inventory and therefore it is possible to study the non-linear effects of changing the quantity of one parameter at the time. Many LCA studies in fact operate similarly, as especially nowadays the domain of LCA has been enriched by the use of models taken from other disciplines (De Rosa et al., 2017; Pizzol, 2019). Thus, the case of transportation here presented is representative of those situations where a complex phenomenon - characterized by a dynamic and possibly non-linear element that nevertheless can be described in good detail with a parametrized model — is then simplified to generate a static life cycle inventory that is thus a snapshot of such complexity.
The LCA model of Bitcoin mining is dependent on data for mining locations and mining equipment use. This data is scarce and, in some cases, diverging. It is therefore important to highlight that this analysis and its results have an intrinsic uncertainty. These have been addressed in a previous study (Köhler and Pizzol, 2019) by conducting both a stochastic simulation and a sensitivity analysis for all parameters and providing an insight of the range of results and of which parameters most strongly influence the results. However, for the purpose of this study — addressing non-linearity of upscaling — this quantity-uncertainty is subordinate as it only influences the magnitude of results, but not the conclusion that upscaling the Bitcoin mining network does not lead to linear increases of impact.
Additionally, the scenarios are a projection of the future, and should therefore be considered an exploration of potential future impacts. In particular the location-sensitive scenarios provide insights on how changing the model structure influence the results and thus address directly the model-uncertainty. Here, not only the quantities (percentage of total mining performed in each location) but also the model structure (number and types of locations) are different from the BAU scenario. The equipment-sensitive scenarios focus on one parameter only, the efficiency of mining equipment, and thus address quantity-uncertainty directly. Due to its simplicity, considering the combined effect in changes of both location and efficiency via scenarios cannot be formally considered as a global sensitivity analysis (Saltelli et al., 2008) but is indeed a step toward this direction.
How Much Is Non-linearity a Problem?
What the present work suggests is that the uncertainties introduced by non-linear effects can be substantial and should be explicitly considered in the life cycle assessment of technologies that are emerging and not yet operating at scale. These findings confirm previous research on the uncertainties in the LCA of emerging technologies (Lacirignola et al., 2017; van der Hulst et al., 2020).
The non-linear effect of improving the efficiency of a technology can have unexpected consequences at database level, when considering all the upstream and downstream processes that are interconnected with the activities employing such technology. The non-linearity becomes critical when investigating the impact of new technologies that are energy and material intensive in their early stages, but also when forecasting the impact of existing mature technologies under different future technology scenario mixes, for example for energy production. The effect of upscaling specific activities, under the assumption that this upscaling returns higher efficiency — which is justified by examples in literature — might have an unpredictable and non-linear effect on the impact of a product system and of related product systems.
Using datasets that are built using data from pilot scale activities and are not representative of the potential of such activities at large industrial scale might thus skew results in unexpected ways. Broad and updated data coverage of the database used for the foreground modeling is thus of critical importance in LCA studies of emerging technologies. While database providers should naturally strive for data collection on unit processes that are as close as possible to a high maturity stage, the practitioner should model background systems that are relevant to the time when the system is modeled, to avoid temporal mismatch — as Arvidsson et al. (2017) point out. This study confirms this finding showing the non-linear effect that using an efficiency-improved version of the database might introduce and highlights the importance of considering background system changes in the assessment of emerging technologies. The feasibility and relevance of this approach has already been demonstrated in practice (Hertwich et al., 2015; Mendoza Beltran et al., 2018a).
In the case of the transport model, non-linear relationships between inputs and outputs of the truck model are less of an issue, as those linearities are in fact considered in both real life and current models. Hence, improving the engine efficiency of a vehicle by a factor of two will certainly not affect the fuel consumption to a similar extent in real life, and nor will it in the LCA model used, as fuel consumption is the result of complex interactions between components placed between the tank and the wheels of a vehicle (e.g., the engine, but also the gearbox, transmission shaft, wheels, etc.). In this case, the issue of non-linearity and the uncertainty associated to it lies outside the truck model and becomes more relevant when addressing future technological scenarios such as how the fuel cell suppliers and the energy efficiency of fuel cell stacks will develop as demand increases, and whether the lithium-ion based batteries will be replaced by a disruptive technology.
This study shows how using a linear assumption in the modeling of the Bitcoin mining network is a strong and excessive simplification of reality. In the location-sensitive scenario (Scenario 1), the impact per additional TH computed increases by over 30% compared to the BAU scenario, while in the equipment-sensitive scenario (Scenario 2), the impact decreases by almost half. In contrast, Köhler and Pizzol (2019) model upscaling scenarios for the Bitcoin mining network for early 2019 and show a decrease in the impacts for both their technology and equipment-sensitive scenarios. The location and equipment-sensitive scenario (Scenario 3) has 32% lower impacts than the linear BAU scenario. In Köhler and Pizzol (2019), the results from the location and equipment-sensitive scenario are 76% lower. It seems therefore to be especially important in the Bitcoin mining case to retrieve accurate data on where new mining facilities are installed and what kind of electricity they consume as the impacts can both decrease or increase per additional TH computed, depending on the assumptions on the geographical distribution of miners. This highlights the importance of building relevant scenarios when upscaling an LCA model for a product system where the structure is expected to change at different scales and levels of maturity.
Conclusion
By challenging the idea that product systems scale linearly, this work shows that non-linear effects should be explicitly considered in the life cycle assessment of technologies that are scalable or emerging. In these cases, a production activity will perform differently and with different efficiencies at different scales and levels of maturity, and its impact per unit of production output is therefore not fixed. Thus, the product system model should reflect the scale and technological maturity of the activities under analysis.
One innovation that this paper introduced is highlighting that — especially for the case for scalable and emerging technologies – the production output (y) of an activity is a separately varying entity than the functional unit of a product system f. In mainstream LCA,
is a linear function (Heijungs, 2020). What is proposed here is instead that:
where the dependence on f is linear but the dependence on y is not, as h1(y) is a non-linear function.
While the LCA is only linear in terms of functional unit dependence, the coefficients that define each activity (values used in A and B) are based on the technologies as the practitioner defines them. In this sense, the use of parametrized LCA is one useful way of modeling systems that exhibit non-linear properties. In this respect, Heijungs (2020) already observes that “the whole idea of parametrized LCA obviously deserves a more rigorous treatment” and the results of this study strengthen this hypothesis and make steps forward in this direction. However, cases such as the Bitcoin network one here presented show that not all non-linearity problems can be addressed by only changing parameters in one single model structure, as it is expectable that system upscaling will influence the type of inputs needed to generate the production output, and this will require changing the structure of the LCA model (number and type of activities and exchanges in A and B).
The study has also shown that addressing non-linearity is essentially a matter of addressing uncertainty in LCA models, and therefore classic uncertainty and sensitivity analysis techniques can be used effectively to investigate and highlight non-linearity. These include, for example, stochastic simulation, developing and assessing scenarios, and studying the effect of a change in output due to a change in specific inputs.
There is currently great attention and expectations to the role of new innovative and emerging technologies for the sustainability transition. The study of the environmental benefits of these technologies is challenged by the availability of pilot-scale data only, and inevitably requires the use of assumptions and the generation of scenarios, and therefore is characterized by intrinsic uncertainties. This study has shown that non-linearity is definitely an uncertainty issue in the study of new technological developments and their impact. Thus, future studies operating in this line of research are strongly encouraged to manifest an explicit awareness of where technological upscaling could occur and to address potential non-linearity issues as part of the uncertainties, and the examples provided in the present work can ideally provide a good inspiration for both identifying and addressing non-linearity.
Data Availability Statement
The datasets presented in this study can be found at the online repository: https://github.com/massimopizzol/non-linearity-LCA. The repository is archived on Zenodo (Pizzol et al., 2020).
Author Contributions
MP contributed with the initial original idea and coordinated, supervised and quality-checked the entire research effort, and contributed to perform the ecoinvent simulation. RS contributed to the ecoinvent simulation and performed the entire transport simulation. SK performed the entire bitcoin simulation. AA contributed to the ecoinvent simulation and wrote the initial paper draft. All authors contributed to the writing and revising of the manuscript.
Funding
SK and MP contribution has been funded by the Grant No. 7015-00006A of the Independent Research Fund Denmark–Social Sciences. The research contribution of RS was primarily funded by the Swiss Competence Center for Energy Research (SCCER) Efficient Technologies and Systems for Mobility, financed by the Swiss Innovation Agency (Innosuisse).
Conflict of Interest
The authors declare that the research was conducted in the absence of any commercial or financial relationships that could be construed as a potential conflict of interest.
Acknowledgments
The Authors thank the two reviewers for their constructive comments that have contributed to substantially improving the quality of the manuscript.
Supplementary Material
The Supplementary Material for this article can be found online at: https://www.frontiersin.org/articles/10.3389/frsus.2020.611593/full#supplementary-material
Footnotes
References
Alvarez, M. (2018). A comparative analysis of cryptocurrency regulation in the United States, Nigeria, and China: the potential influence of illicit activities on regulatory evolution. ILSA J. Int. Comp. Law 25, 33–56. Available online at: https://heinonline.org/HOL/LandingPage?handle=hein.journals/ilsaic25&div=7&id=&page=
Arvidsson, R., Tillman, A.-M., Sandén, B. A., Janssen, M., Nordelöf, A., Kushnir, D., et al. (2017). Environmental assessment of emerging technologies: recommendations for prospective LCA. J. Ind. Ecol. 22, 1286–1294. doi: 10.1111/jiec.12690
ASIC-MinerValue (2020). ASIC Miner Value. Available online at: https://www.asicminervalue.com/ (accessed September 15, 2020).
AzariJafari, H., Yahia, A., and Amor, B. (2018). Assessing the individual and combined effects of uncertainty and variability sources in comparative LCA of pavements. Int. J. Life Cycle Assess. 23, 1888–1902. doi: 10.1007/s11367-017-1400-1
Bergerson, J. A., Brandt, A., Cresko, J., Carbajales-Dale, M., MacLean, H. L., Matthews, H. S., et al. (2019). Life cycle assessment of emerging technologies: evaluation techniques at different stages of market and technical maturity. J. Indust. Ecol. 24, 11–25. doi: 10.1111/jiec.12954
Bisinella, V., Conradsen, K., Christensen, T. H., and Astrup, T. F. (2016). A global approach for sparse representation of uncertainty in life cycle assessments of waste management systems. Int. J. Life Cycle Assess. 21, 378–394. doi: 10.1007/s11367-015-1014-4
Blanco, C. F., Cucurachi, S., Guinée, J. B., Vijver, M. G., Peijnenburg, W. J. G. M., Trattnig, R., et al. (2020). Assessing the sustainability of emerging technologies: a probabilistic LCA method applied to advanced photovoltaics. J. Clean. Prod. 259:120968. doi: 10.1016/j.jclepro.2020.120968
Caduff, M., Huijbregts, M. A. J., Althaus, H. J., and Hendriks, A. J. (2011). Power-law relationships for estimating mass, fuel consumption and costs of energy conversion equipments. Environ. Sci. Technol. 45, 751–754. doi: 10.1021/es103095k
Caduff, M., Huijbregts, M. A. J., Althaus, H. J., Koehler, A., and Hellweg, S. (2012). Wind power electricity: the bigger the turbine, the greener the electricity? Environ. Sci. Technol. 46, 4725–4733. doi: 10.1021/es204108n
Caduff, M., Huijbregts, M. A. J., Koehler, A., Althaus, H. J., and Hellweg, S. (2014). Scaling relationships in life cycle assessment: the case of heat production from biomass and heat pumps. J. Ind. Ecol. 18, 393–406. doi: 10.1111/jiec.12122
Cambridge Centre for Alternative Finance (2020). Bitcoin Mining Map. Cambridge Centre for Alternative Finance. Available online at: https://cbeci.org/mining_map
Cucurachi, S., Borgonovo, E., and Heijungs, R. (2016). A protocol for the global sensitivity analysis of impact assessment models in life cycle assessment. Risk Anal. 36, 357–377. doi: 10.1111/risa.12443
De Rosa, M., Schmidt, J., Brandão, M., and Pizzol, M. (2017). A flexible parametric model for a balanced account of forest carbon fluxes in LCA. Int. J. Life Cycle Assess. 22, 172–184. doi: 10.1007/s11367-016-1148-z
Ding, Y., Cano, Z. P., Yu, A., Lu, J., and Chen, Z. (2019). Automotive Li-ion batteries: current status and future perspectives. Electrochem. Energy Rev. 2, 1–28. doi: 10.1007/s41918-018-0022-z
Eurostat (2020). Annual Road Freight Transport, by Load Capacity of Vehicle. Eurostat. Availabe online at: http://appsso.eurostat.ec.europa.eu/nui/show.do?dataset=road_go_ta_lcandlang=en (accessed September 28, 2020).
Gibbons, S., and Bendiksen, C. (2019). The Bitcoin Mining Network - December 2019 Update. New York, NY: CoinShares Research. Available online at: https://coinshares.com/assets/resources/Research/bitcoin-mining-network-december-2019.pdf
Grubb, M. (2004). Technology innovation and climate change policy: an overview of issues and options. Keio Econ. Stud. 41, 103–132. Available online at: https://discovery.ucl.ac.uk/id/eprint/1471206/
Heijungs, R. (2020). Is mainstream LCA linear? Int. J. Life Cycle Assess. 25, 1872–1882. doi: 10.1007/s11367-020-01810-z
Heijungs, R., and Lenzen, M. (2014). Error propagation methods for LCA - a comparison. Int. J. Life Cycle Assess. 19, 1445–1461. doi: 10.1007/s11367-014-0751-0
Heijungs, R., and Suh, S. (2002). “The basic model for inventory analysis,” in The Computational Structure of Life Cycle Assessment, ed Tukker, A (London: Kluver Academic Publisher), 11–28.
Hertwich, E. G., Gibon, T., Bouman, E. A., Arvesen, A., Suh, S., Heath, G. A., et al. (2015). Integrated life-cycle assessment of electricity-supply scenarios confirms global environmental benefit of low-carbon technologies. Proc. Natl. Acad. Sci. U.S.A. 112, 6277–6282. doi: 10.1073/pnas.1312753111
Igos, E., Benetto, E., Meyer, R., Baustert, P., and Othoniel, B. (2019). How to treat uncertainties in life cycle assessment studies? Int. J. Life Cycle Assess. 24, 794–807. doi: 10.1007/s11367-018-1477-1
IPCC (2013). Climate Change 2013: The Physical Science Basis. Contribution of Working Group I to the Fifth Assessment Report of the Intergovernmental Panel on Climate Change (IPCC). Bern: Intergovernmental Panel on Climate Change (IPCC).
Köhler, S., and Pizzol, M. (2019). Life cycle assessment of bitcoin mining. Environ. Sci. Technol. 53, 13598–13606. doi: 10.1021/acs.est.9b05687
Lacirignola, M., Blanc, P., Girard, R., Pérez-López, P., and Blanc, I. (2017). LCA of emerging technologies: addressing high uncertainty on inputs' variability when performing global sensitivity analysis. Sci. Total Environ. 578, 268–280. doi: 10.1016/j.scitotenv.2016.10.066
Mendoza Beltran, A., Cox, B., Mutel, C., van Vuuren, D. P., Font Vivanco, D., Deetman, S., et al. (2018a). When the background matters: using scenarios from integrated assessment models in prospective life cycle assessment. J. Ind. Ecol. 24, 436–439. doi: 10.1111/jiec.12825
Mendoza Beltran, A., Prado, V., Font Vivanco, D., Henriksson, P. J. G., Guinée, J. B., and Heijungs, R. (2018b). Quantified uncertainties in comparative life cycle assessment: what can be concluded? Environ. Sci. Technol. 52, 2152–2161. doi: 10.1021/acs.est.7b06365
Meynkhard, A. (2019). Fair market value of bitcoin: Halving effect. Invest. Manag. Financ. Innov. 16, 72–85. doi: 10.21511/imfi.16(4).2019.07
Miotti, M., Hofer, J., and Bauer, C. (2017). Integrated environmental and economic assessment of current and future fuel cell vehicles. Int. J. Life Cycle Assess. 22, 94–110. doi: 10.1007/s11367-015-0986-4
Mutel, C. (2017). Brightway: an open source framework for life cycle assessment. J. Open Source Softw. 2, 1–2. doi: 10.21105/joss.00236
Nemet, G. F., Zipperer, V., and Kraus, M. (2018). The valley of death, the technology pork barrel, and public support for large demonstration projects. Energy Policy 119, 154–167. doi: 10.1016/j.enpol.2018.04.008
Padey, P., Girard, R., Le Boulch, D., and Blanc, I. (2013). From LCAs to simplified models: a generic methodology applied to wind power electricity. Environ. Sci. Technol. 47, 1231–1238. doi: 10.1021/es303435e
Piccinno, F., Hischier, R., Seeger, S., and Som, C. (2016). From laboratory to industrial scale: a scale-up framework for chemical processes in life cycle assessment studies. J. Clean. Prod. 135, 1085–1097. doi: 10.1016/j.jclepro.2016.06.164
Pizzol, M. (2019). Deterministic and stochastic carbon footprint of intermodal ferry and truck freight transport across Scandinavian routes. J. Clean. Prod. 224, 626–636. doi: 10.1016/j.jclepro.2019.03.270
Pizzol, M., romainsacchi, Erjavec, A., and susannekoehler. (2020). massimopizzol/Non-linearity-LCA: First Release (Version v1.0.0). Zenodo. doi: 10.5281/zenodo.4278067
Rizet, C., Cruz, C., and Mbacké, M. (2012). Reducing freight transport CO2 emissions by increasing the load factor. Procedia Soc. Behav. Sci. 48, 184–195. doi: 10.1016/j.sbspro.2012.06.999
Sacchi, R., Besseau, R., Pérez-López, P., and Blanc, I. (2019). Exploring technologically, temporally and geographically-sensitive life cycle inventories for wind turbines: a parameterized model for Denmark. Renew. Energy 132, 1238–1250. doi: 10.1016/j.renene.2018.09.020
Saltelli, A. (2008). “Global sensitivity analysis: an introduction,” in Sensitivity Analysis of Model Output, eds K. M. Hanson and F. M. Hemez (Washington, DC), 27–43.
Saltelli, A., Ratto, M., Andres, T., Campolongo, F., Cariboni, J., Gatelli, D., et al. (2008). global sensitivity analysis. The Primer, Global Sensitivity Analysis. The Primer. doi: 10.1002/9780470725184
Tsoy, N., Steubing, B., van der Giesen, C., and Guinée, J. (2020). Upscaling methods used in ex ante life cycle assessment of emerging technologies: a review. Int. J. Life Cycle Assess. 25, 1680–1692. doi: 10.1007/s11367-020-01796-8
Valsasina, L., Pizzol, M., Smetana, S., Georget, E., Mathys, A., and Heinz, V. (2017). Life cycle assessment of emerging technologies: the case of milk ultra-high pressure homogenisation. J. Clean. Prod. 142, 2209–2217. doi: 10.1016/j.jclepro.2016.11.059
van der Hulst, M. K., Huijbregts, M. A. J., van Loon, N., Theelen, M., Kootstra, L., Bergesen, J. D., et al. (2020). A systematic approach to assess the environmental impact of emerging technologies: a case study for the GHG footprint of CIGS solar photovoltaic laminate. J. Ind. Ecol. 24, 1234–1249. doi: 10.1111/jiec.13027
Keywords: uncertainty analysis, technological learning, efficiency, bitcoin, transportation
Citation: Pizzol M, Sacchi R, Köhler S and Anderson Erjavec A (2021) Non-linearity in the Life Cycle Assessment of Scalable and Emerging Technologies. Front. Sustain. 1:611593. doi: 10.3389/frsus.2020.611593
Received: 29 September 2020; Accepted: 08 December 2020;
Published: 06 January 2021.
Edited by:
Dingsheng Li, University of Nevada, United StatesReviewed by:
Reinout Heijungs, Vrije Universiteit Amsterdam, NetherlandsStefano Cucurachi, Leiden University, Netherlands
Copyright © 2021 Pizzol, Sacchi, Köhler and Anderson Erjavec. This is an open-access article distributed under the terms of the Creative Commons Attribution License (CC BY). The use, distribution or reproduction in other forums is permitted, provided the original author(s) and the copyright owner(s) are credited and that the original publication in this journal is cited, in accordance with accepted academic practice. No use, distribution or reproduction is permitted which does not comply with these terms.
*Correspondence: Massimo Pizzol, bWFzc2ltb0BwbGFuLmFhdS5kaw==