- 1STADIUS Center for Dynamical Systems, Signal Processing and Data Analytics, Department of Electrical Engineering (ESAT), KU Leuven, Leuven, Belgium
- 2Institute of Sound Recording, Department of Music and Media, University of Surrey, Guildford, United Kingdom
Room impulse responses (RIRs) between static loudspeaker and microphone locations can be estimated using a number of well-established measurement and inference procedures. While these procedures assume a time-invariant acoustic system, time variations need to be considered for the case of spatially dynamic scenarios where loudspeakers and microphones are subject to movement. If the RIR is modeled using image sources, then movement implies that the distance to each image source varies over time, making the estimation of the spatially dynamic RIR particularly challenging. In this paper, we propose a procedure to estimate the early part of the spatially dynamic RIR between a stationary source and a microphone moving on a linear trajectory at constant velocity. The procedure is built upon a state-space model, where the state to be estimated represents the early RIR, the observation corresponds to a microphone recording in a spatially dynamic scenario, and time-varying distances to the image sources are incorporated into the state transition matrix obtained from static RIRs at the start and end points of the trajectory. The performance of the proposed approach is evaluated against state-of-the-art RIR interpolation and state-space estimation methods using simulations, demonstrating the potential of the proposed state-space model.
1 Introduction
A room impulse response (RIR) is the time-domain representation of the linear time-invariant (LTI) system that uniquely characterizes the cumulative impact of a room on sound waves between a specific static source and a microphone’s position, effectively representing the room’s acoustic environment. This concept is fundamental to various acoustic signal processing applications, including source localization (Evers et al., 2020), dereverberation (Naylor and Gaubitch, 2010), echo cancellation (Elko et al., 2003), and spatial audio reproduction (Schissler et al., 2017). Considerable research has been dedicated to developing robust measurement (Stan et al., 2002; Szöke et al., 2018) and estimation (Lin and Lee, 2006; Crocco and Bue, 2015; Ratnarajah et al., 2022) techniques for capturing RIRs, particularly in scenarios where the source and microphone remain static within the acoustic environment (Stan et al., 2002; Szöke et al., 2018). However, real-world situations often involve spatially dynamic scenarios, where sources and microphones are subject to movement. This paper addresses the challenge of accurately estimating the early part of RIRs along a trajectory in a time-varying acoustic scenario with a stationary source and a moving microphone. This can be thought of as a time-varying system identification problem where the system to be identified may be referred to as a time-variant RIR.
In this context, it is important to consider the apparent contradiction between the previously defined RIR as an LTI system representation and the concept of a time-variant RIR. In this paper, the acoustic environment itself is assumed to be time-invariant, in which case an RIR between two static positions indeed corresponds to an LTI system. RIRs, however, are inherently location-variant—they depend on the locations of the source and the microphone. The time-variant RIR, as considered here, can be defined as the time-varying system representation (Cherniakov, 2003) relating the source signal to the microphone signal if the microphone moves—that is, has a time-varying location. In discrete time, this time-variant RIR can also be thought of as a collection of RIRs in the LTI sense over different time-instances: the location of the microphone changes at each time step, leading to a new discrete position in space associated with a time-invariant RIR. Time-variant RIR estimation, as defined above, has relevance across numerous acoustic signal processing applications, especially amid the growing interest in virtual acoustic environments. For instance, Ajdler et al. (2007) demonstrated the use of time-varying acoustic system models for enabling the rapid measurement of head-related impulse responses. Moreover, sub-optimal estimations of time-variant RIRs can impair the effectiveness of echo-cancellation systems in telepresence and communication technologies (Nophut et al., 2024).
In order to contextualize our contribution in estimating the early part of a time-variant RIR, it is necessary to provide a brief outline of the related literature. In this context, the concept of spatial RIR interpolation is highly relevant given that we can consider a time-varying RIR as a collection of RIRs over a discrete set of locations. RIR interpolation facilitates sound field rendering for dynamic source–microphone positions by filling spatial gaps in RIR data, as measurements or simulations are usually limited to sparse grids. Numerous approaches have been proposed for RIR interpolation, including compressed sensing methods (Mignot et al., 2013; Katzberg et al., 2018), spherical harmonics (Borra et al., 2019), physics-based models (Antonello et al., 2017; Hahmann and Fernandez-Grande, 2022), directional RIRs (Zhao et al., 2022), and neural networks (Pezzoli et al., 2022; Karakonstantis et al., 2024). Haneda et al. (1999) introduced a frequency-domain approach for interpolating room transfer functions (RTFs), which is particularly effective at lower frequencies; it was extended by Das et al. (2021). Additionally, several techniques for the interpolation of head-related transfer functions (HRTFs), which are critical for accurate binaural rendering and share some methodological approaches with RTF interpolation, have been explored (Carty, 2010). For the purpose of this paper, we focus on the interpolation of an RIR at a point between two microphone locations given their estimated RIRs for a common stationary source. Kearney et al. (2009) introduced Dynamic Time Warping (DTW)-based interpolation, dividing RIRs into early reflections and diffuse decay. This method temporally aligns and linearly interpolates early reflections while modeling the tail using the approach of Masterson et al. (2009) and laying the foundation for subsequent developments. Garcia-Gomez and Lopez (2018) expanded on Kearney’s work, enhancing algorithm robustness and computational efficiency while retaining the core interpolation technique. Building on this, Bruschi et al. (2020) refined peak finding and matching aspects of the interpolation technique. Geldert et al. (2023) proposed a novel approach using partial optimal transport for interpolation, enabling non-bijective mapping of sound events between the early part of RIRs. It is important to highlight that these interpolation approaches operate outside of a conventional system identification framework. Instead, they use a limited set of measured RIRs and predominantly rely on a room acoustic sound propagation model such as the image source method (ISM) (Allen and Berkley, 1979). As opposed to interpolation strategies, fully data-driven approaches for estimating time-variant RIRs have also been investigated. In this case, the estimation relies directly on the source and microphone signals and can be framed as a system identification or adaptive filtering problem. This approach has been largely motivated by the need to obtain rapid measurements of head-related impulse responses (Enzner, 2008; Hahn and Spors, 2015) and for echo cancellation (Antweiler and Symanzik, 1995; Enzner, 2010), where a typical scenario involves an excitation signal being continuously captured by a moving microphone. Using carefully designed excitation signals (Hahn and Spors, 2015; Kuhl et al., 2018), time-variant RIRs can be estimated using a normalized least mean squares (NLMS) algorithm (Enzner, 2008; Antweiler et al., 2012) or more generally using a Kalman filter (Enzner, 2010). In the context of this paper, a Kalman filter is of particular interest as it is derived from a state-space model of the dynamic system where the state to be estimated is the time-variant RIR (Enzner, 2010). In such a state-space model, the evolution of the time-variant RIR is explicitly modeled using a first-order difference equation, which allows more modeling flexibility than other adaptive algorithms such as NLMS. One popular choice for the first-order difference model is to relate the states at two time instants by a transition factor and an additional process noise term. The transition coefficient and process noise covariance are typically set according to the expected variability of the state, influencing the convergence behavior of the Kalman filter. For instance, in Nophut et al. (2024), the transition coefficient was modeled as a function of the microphone velocity.
We here consider the problem of estimating the early part of the time-variant RIR between a stationary source and a microphone moving on a linear trajectory at constant velocity. We propose integrating RIR interpolation, derived from a room acoustic model, into a state-space-based framework for RIR estimation, thereby merging data-driven approaches with physical modeling. More specifically, rather than relying solely on a state transition factor within the state equation, we propose incorporating the ISM into a state transition matrix between the early segments of consecutive RIRs. We derive an analytical model for this transition matrix and subsequently propose estimating it from static RIRs at the trajectory’s start and end points using a DTW-based algorithm. The proposed approach's performance is evaluated through simulations by comparing it to two alternatives: one that relies solely on the state equation for RIR estimation, resembling a pure interpolation method with the ISM-based transition matrix, and another that uses a conventional state-space estimation with a simple state transition factor. Our findings suggest that the proposed state-space model outperforms both alternatives in terms of normalized misalignment between the simulated “ground-truth” RIRs and estimated RIRs.
The subsequent sections of this paper are organized as follows. Section 2 introduces the signal model and provides an overview of the most pertinent state-of-the-art methods. Section 3 elaborates on the proposed RIR state-space model and outlines the update equations of the Kalman filter used to recursively estimate the defined state. Section 4 offers detailed derivations of the proposed room acoustic model-based state transition matrix. Finally, Section 5 presents experimental validation through simulations, followed by a discussion of the results.
2 Signal model, problem statement, and related state of the art
2.1 Signal model and problem statement
We first introduce the signal model to formally define the concept of an RIR as employed in this paper. When we henceforth use the term “RIR”, it will specifically pertain to the early part of the RIRs, as we do not address the estimation of the late reverberant tail. We also assume that the source location remains static. Let
where
the convolution in Equation 1 can alternatively be written as
Within the scope of this paper, we assume a linear microphone trajectory of length
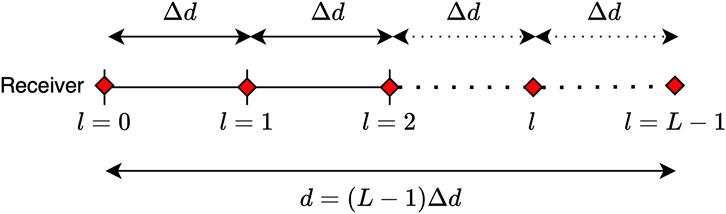
Figure 1. Linear microphone trajectory of length
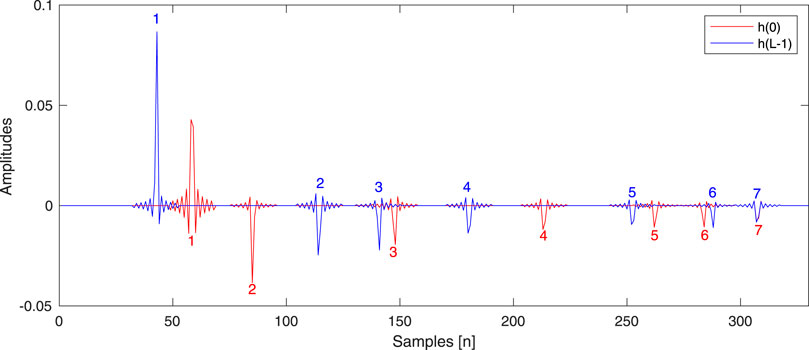
Figure 2. An example of simulated RIRs,
2.2 Related state of the art
Before introducing the proposed approach, it is instructive to briefly introduce the most relevant concepts used in the state of the art. On the one hand, we consider RIR interpolation approaches that estimate
As previously mentioned, some RIR interpolation approaches (Kearney et al., 2009; Geldert et al., 2023) are motivated by the ISM for room acoustic sound propagation, which expresses an RIR as a sum of contributions from the original source and so-called image sources representing reflections from the boundaries of the room. Based on this model, RIR interpolation approaches infer the location-variant time of arrival (TOA) as well as the amplitude of the direct component and individual reflections (or equivalently, source and image source components) at a particular location
where
Data-driven approaches (Enzner, 2010) to the estimation of location- or time-variant RIRs do not rely on explicit modeling of room acoustic sound propagation, but instead perform adaptive system identification given a data set containing
This can be expressed similarly to Equation 4 using the definitions in Equations 2 and 3. A popular approach to adaptively estimate
where the state equation in Equation 7 models the evolution of the state as a first-order difference equation, and the observation equation (Equation 8) relates the state to the observation
3 Proposed RIR state-space model
In the proposed approach, we aim to include prior knowledge on room acoustic sound propagation into a state-space model for time-variant RIR estimation. To this end, rather than resorting to a scalar factor as in Equation 7, we assume that the relation between two RIRs
for
While it is possible to interpolate between
where
With Equation 9 and defining
where
3.1 Update equations of the Kalman filter
The Kalman filter (Simon, 2006) can be used to recursively estimate the state defined by a state-space model. For the proposed state-space model in Equations 10 and 11, let
where Equations 12 and 13 are commonly referred to as the prediction step producing prior estimates, and Equations 14–16 are referred to as the update step producing posterior estimates. In these equations,
To implement the Kalman filter,
At this point, it is instructive to interpret Equations 12–16 in relation to the state of the art as discussed in Section 2. In the interpolation approaches in Kearney et al. (2009) and Geldert et al. (2023) on the one hand, recorded signals are not available, which corresponds to the assumption that
4 Proposed room acoustic model-based transition matrix
This section provides a detailed explanation of the methodology followed to obtain a suitable room acoustic model-based transition matrix for use in Equation 10 and is organized as follows. In Section 4.1, we derive an analytical expression for a location-variant transition matrix model
4.1 Analytical location-variant transition matrix model
The objective of this section is to derive a transition matrix model
An illustration of this relation along a given trajectory is shown in Figure 3a.
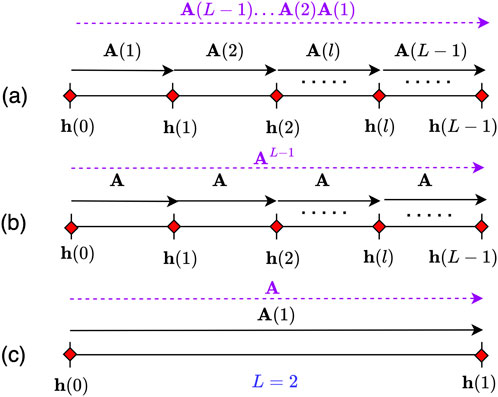
Figure 3. (a) Location-variant transition matrix model
To achieve this objective, we make use of the ISM. In this model, the RIR is expressed as the sum of contributions from the original sound source and additional image sources, which represent reflections within the room. Let the RIR at location
where
The inclusion of
Therefore, Equation 19 can alternatively be expressed as
To obtain a discrete time-shift representation in terms of the time-shift index
as the TDOA of reflection
At this point, it is advantageous to introduce a time-shift-dependent approximation of Equation 22. At any
which contains the TOAs of the non-negligible components of reflection
With Equation 24, we can therefore approximate Equation 22 as
where we have swapped the order of summation over
and rewrite Equation 25 as
with the error term
Given the relation between
which essentially says that the reflections in
if
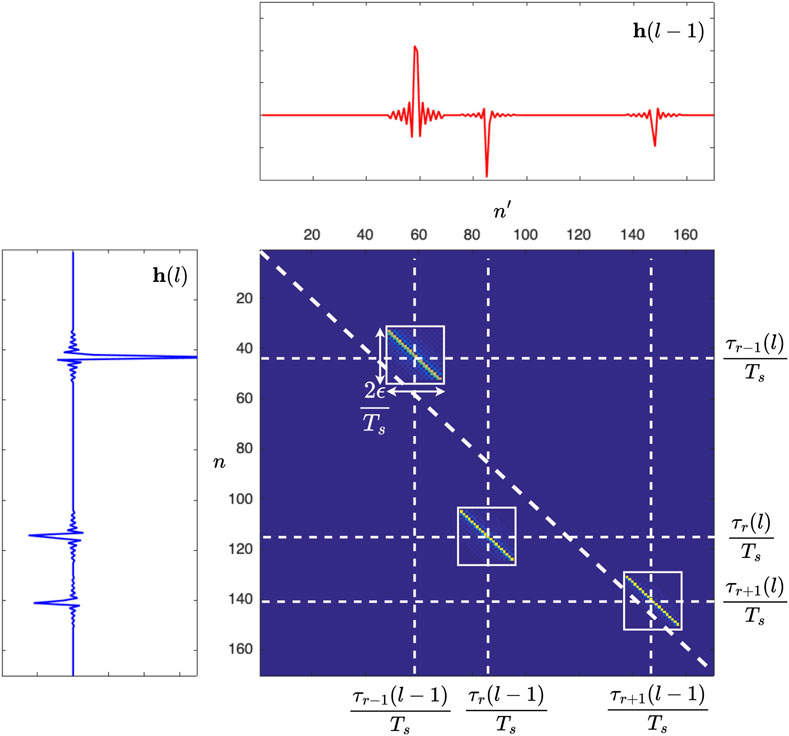
Figure 4. An example of an analytical location-variant transition matrix
4.2 Analytical location-invariant transition matrix model
For the application at hand, we anticipate employing a location-invariant transition matrix model as described in Equation 9 and illustrated in Figure 3b. We show that by using a location-invariant matrix, in which TOA intervals are defined to span the entire trajectory, we can reduce the necessity for accurate TOA estimates. This efficiency is achieved because these TOA intervals, along with TDOA estimates, can be obtained directly from
In Equation 29, we observe two dependencies on
The dependence of
which can be understood as an extension of Equation 23.3
Based on Equation 31, we define the set similar to Equation 24 without the dependency on
The approximation in Equation 26 can then be replaced by
with the error term
Again, the product
where
and the limits in Equations 39, 40 are obtained from Equations 32, 33, and
if
Given the assumption of access to exact reflection TOAs
Note that from a conceptual point of view, the terms
Keeping in mind Figures 3a and b, for an intuitive understanding of the relationship between
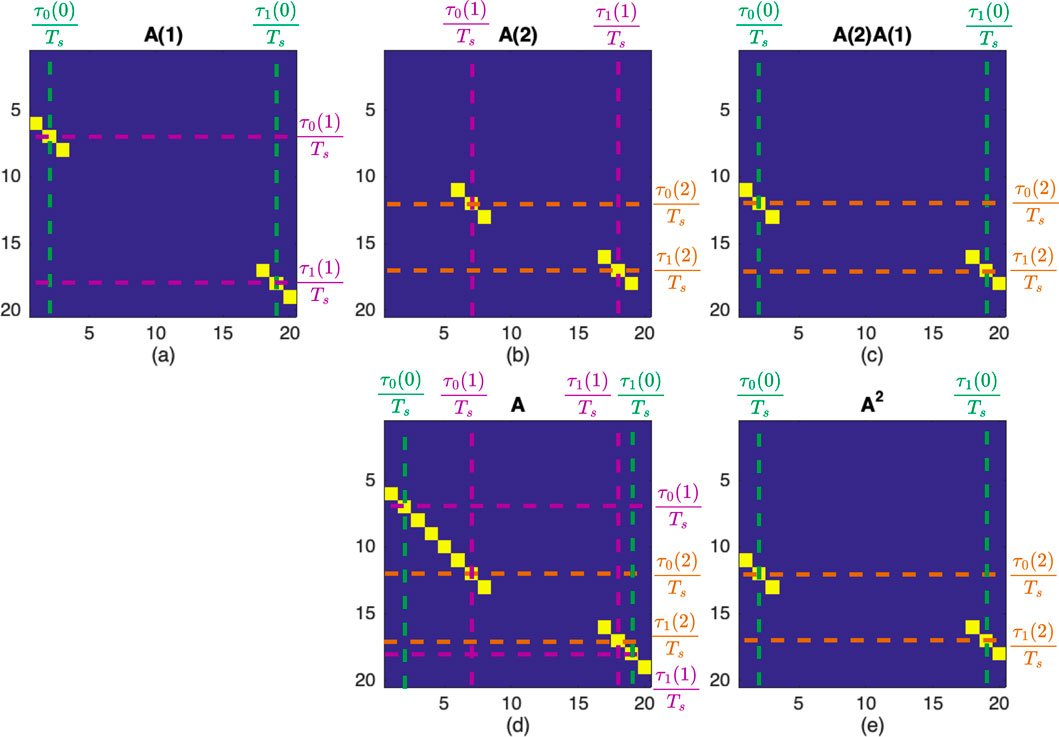
Figure 6. Illustration of the analytical transition matrices for a toy problem along a trajectory with
4.3 Dynamic Time Warping transition matrices
As it stands, the analytical solution proposed in Section 4.2 cannot be applied directly to RIRs and requires inherent knowledge of the TOAs in
The DTW algorithm (Müller, 2007) involves computing an accumulated cost matrix
which is initialized such that
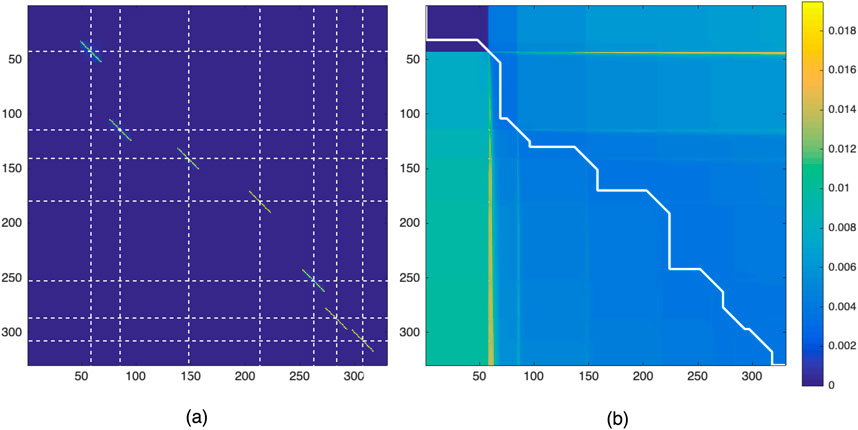
Figure 7. (a) Location-invariant matrix
A warp path, denoted by pairs of indices
The elements along the horizontal and vertical “gridlines” in
In order to use the shape of the warp path through
Such a matrix
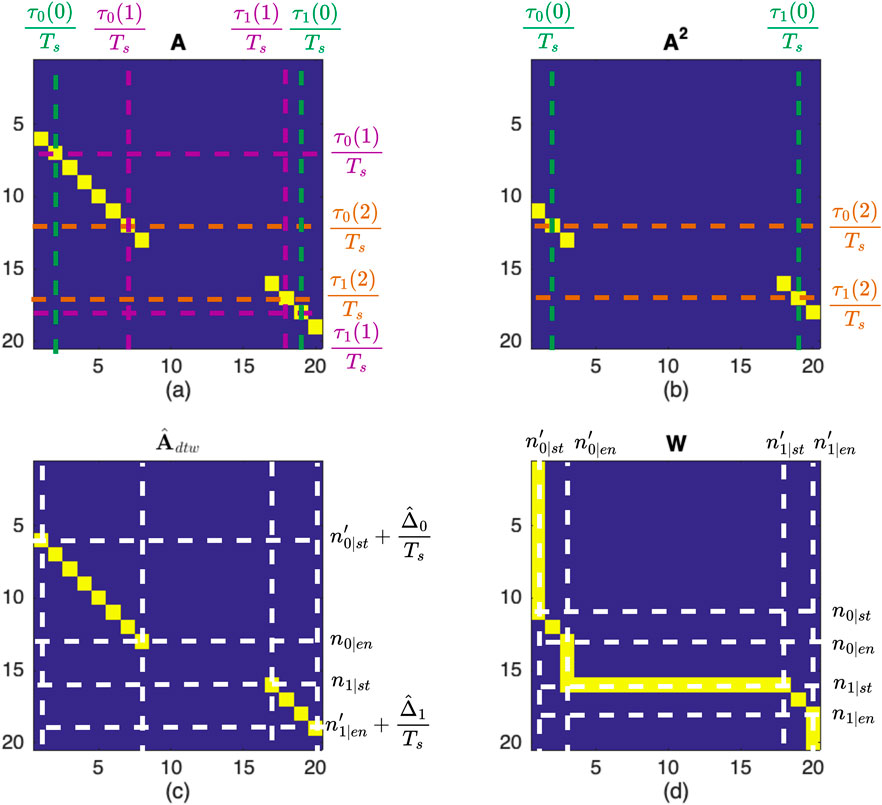
Figure 8. Illustration of the analytical transition matrices for a toy problem along a trajectory with
Firstly, integer estimates of
Secondly the start and end indices of the diagonal segments, denoted, respectively, as
It should be ensured that the estimated ranges still result in the condition in Equation 37 being met—that is, that corresponding column ranges do not overlap. Finally, we construct the matrix
5 Simulations
In this section, we systematically evaluate the performance of transition matrices
5.1 Acoustic environment
For simplicity, we consider a box-shaped room with dimensions bounded by
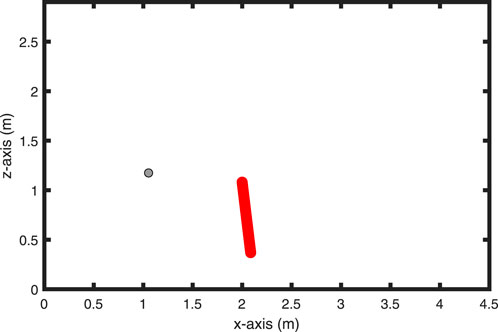
Figure 9. 2-D illustration depicting the source position and trajectory of the microphone within a box-shaped room, as utilized for simulations.
At a standard speech sampling rate of 16 kHz (Nophut et al., 2024) and with a microphone velocity of 0.25 m/s, the ISM (Allen and Berkley, 1979) is used to simulate the ground-truth early RIRs
5.2 Kalman filter parameters
As outlined in Section 3.1, the Kalman filter relies on specific input parameters for its operation. These parameters include the process noise covariance matrix
Given the assumption of knowledge of the RIR at the start of the trajectory
5.3 Performance measure
In assessing the accuracy of our estimated RIRs
Here,
5.4 Experiments
We categorize experiments into the following four sets to evaluate algorithm performance.
6 Results
We present the results corresponding to the experiments outlined above. The reader is urged to take careful note of the different
6.1 Result 1: ideal case
The results of Experiment 1 are presented at the top of Figure 10, where the normalized misalignment
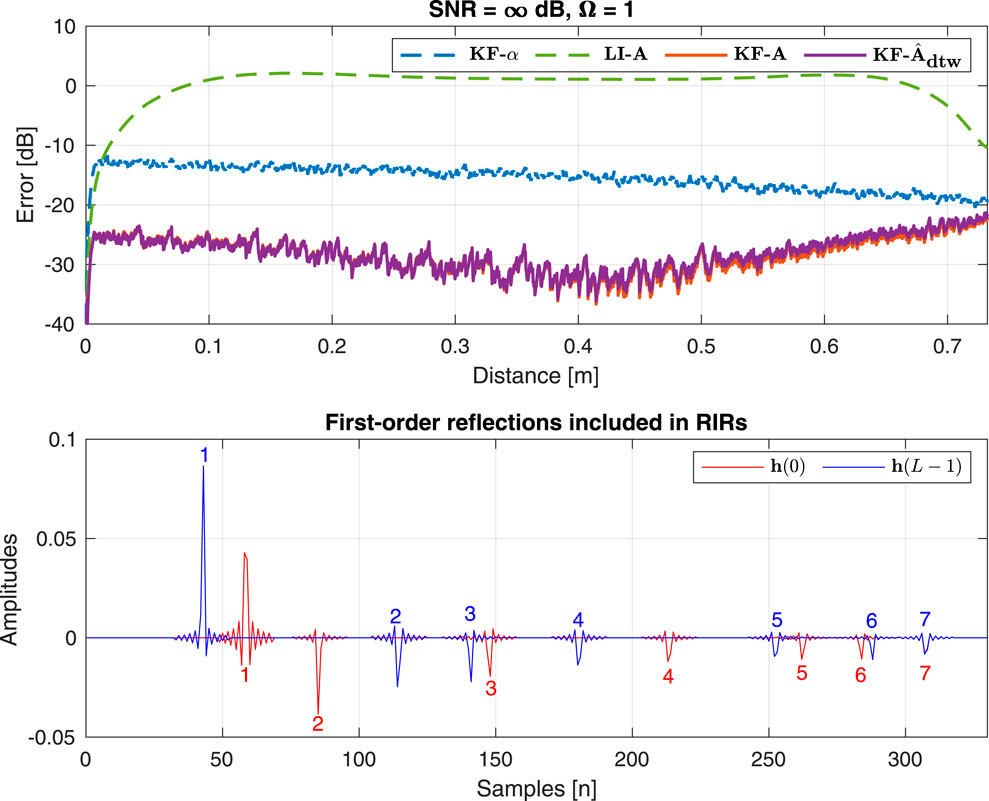
Figure 10. (Top) Result 1: Normalized misalignment along the trajectory between the “ground-truth” RIR
At the beginning of the trajectory, all algorithms exhibit zero error owing to their initialization with a known RIR
In general, changes in the error curve of the Kalman filter algorithms using a transition matrix (
If we now consider the relative performance of the algorithms, we note that the Kalman filter employing “ideal” analytical transition matrix Algorithm
6.2 Result 2: noise sensitivity
The results of Experiment 2 are presented in Figure 11 and once again exhibit error curve shapes consistent with those observed in Experiment 1. However, as anticipated, overall performance is worse, except for Algorithm
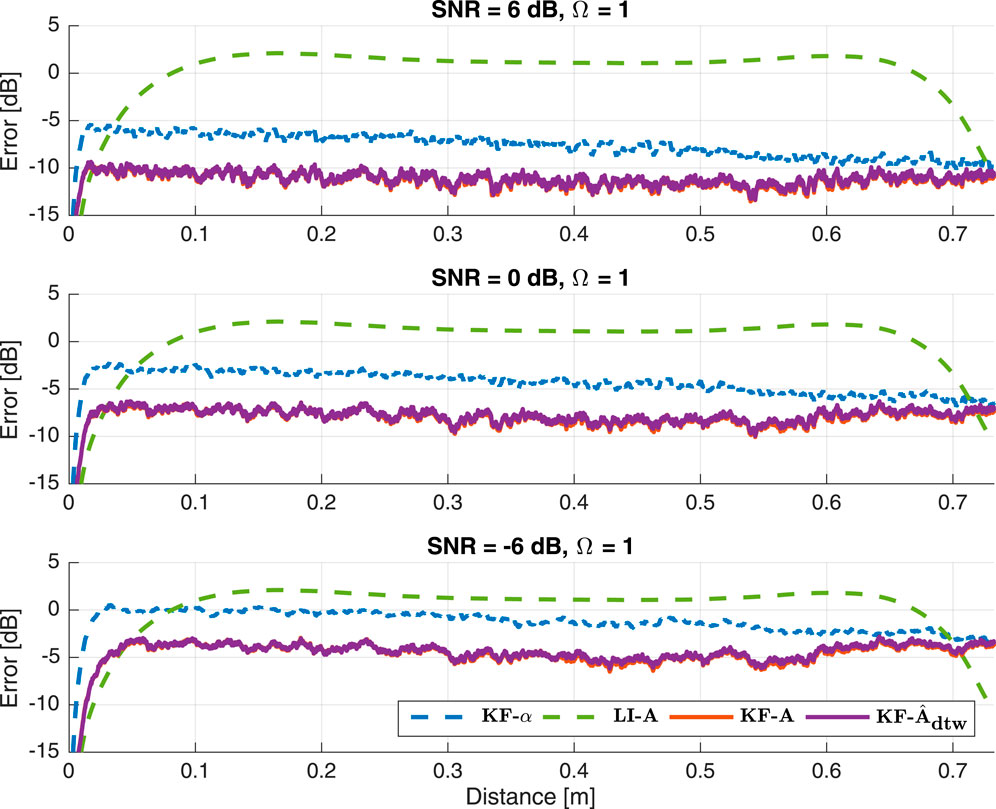
Figure 11. Result 2: Normalized misalignment along the trajectory between the “ground-truth” RIR
It is evident that even under poor SNR conditions, our methods consistently outperform both reference algorithms. Notably, at an SNR of
6.3 Result 3: spatial sampling effects
The results of Experiment 3 are presented in Figure 12. As fewer measurements are used, the disparity between the results obtained using the linear interpolation method and those obtained using a Kalman filter naturally diminishes. Additionally, the performance of the reference Kalman filter
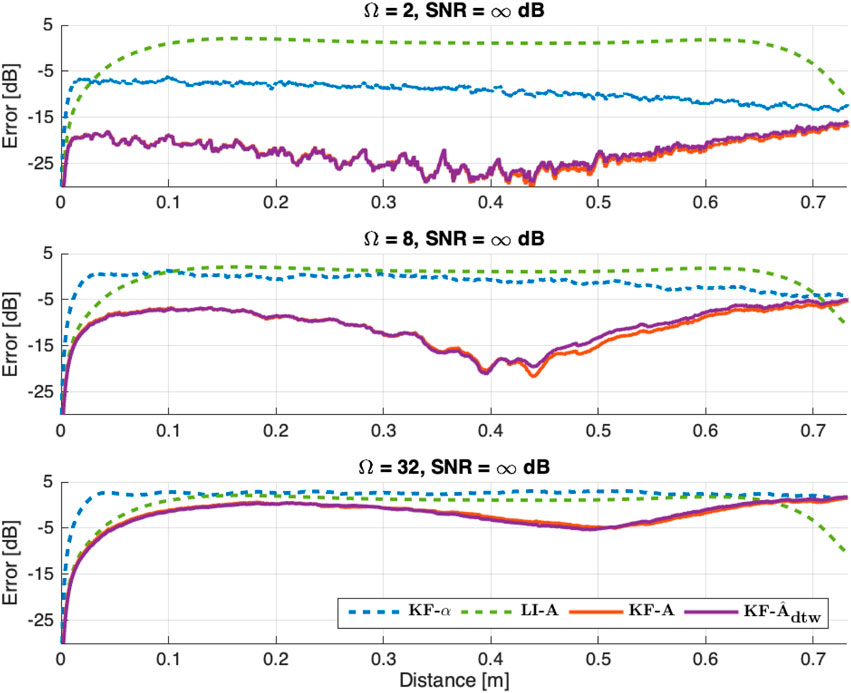
Figure 12. Result 3: Normalized misalignment along the trajectory between the “ground-truth” RIR
For
6.4 Result 4: second-order reflections included
The results of Experiment 4 are shown at the top of Figure 13. It is important to recall that in Experiment 4, the analytical transition matrix
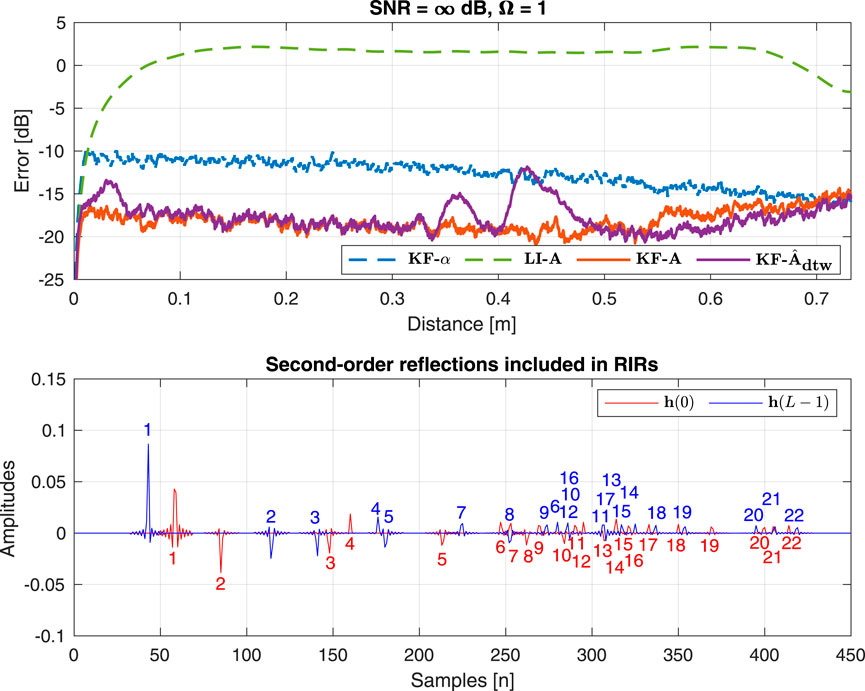
Figure 13. (Top) Result 4: Normalized misalignment along the trajectory between the “ground-truth” RIR
It is important to reiterate that in estimating the matrix
7 Conclusion
This paper investigates the estimation of early segments of time-varying RIRs through a state-space model incorporating the ISM within the state transition matrix. Simulation results indicate that this approach outperforms both RIR interpolation and a purely data-driven state-space model using a transition factor. Moreover, a practical method for estimating such a matrix has been proposed and has a similar performance to the “ideal” analytical transition matrix derived. It is important to acknowledge that certain assumptions inherent to our method limit its application within specific areas of a room. This necessitates further research in order to improve the robustness of the approach, potentially through adaptive estimation of the transition matrix. Furthermore, experimental validation using real measurement data is required to assess the effectiveness of the proposed approach in real-world scenarios.
Data availability statement
The raw data supporting the conclusions of this article will be made available by the authors, without undue reservation.
Author contributions
KM: conceptualization, formal analysis, investigation, methodology, validation, writing–original draft, and writing–review and editing. TD: conceptualization, methodology, supervision, writing–original draft, and writing–review and editing. RA: conceptualization, supervision, writing–original draft, and writing–review and editing. TV: conceptualization, funding acquisition, supervision, and writing–review and editing.
Funding
The authors declare that financial support was received for the research, authorship, and/or publication of this article. This work was supported by
Conflict of interest
The authors declare that the research was conducted in the absence of any commercial or financial relationships that could be construed as a potential conflict of interest.
Publisher’s note
All claims expressed in this article are solely those of the authors and do not necessarily represent those of their affiliated organizations, or those of the publisher, the editors, and the reviewers. Any product that may be evaluated in this article, or claim that may be made by its manufacturer, is not guaranteed or endorsed by the publisher.
Supplementary material
The Supplementary Material for this article can be found online at: https://www.frontiersin.org/articles/10.3389/frsip.2024.1426082/full#supplementary-material
Footnotes
1For notational convenience when working with an interval
2In the special case that
3Note that if
4In this paper, matrices are indexed from (1,1), following the convention of many programming languages. However, our RIR sequences are indexed from 0. To align with the zero-based indexing of our RIRs and accommodate the additional row and column used solely for initialization, we index the entries of the accumulated cost matrix as
References
Ajdler, T., Sbaiz, L., and Vetterli, M. (2007). Dynamic measurement of room impulse responses using a moving microphone. J. Acoust. Soc. Amer. (JASA) 122, 1636–1645. doi:10.1121/1.2766776
Allen, J. B., and Berkley, D. A. (1979). Image method for efficiently simulating small-room acoustics. J. Acoust. Soc. Amer. (JASA) 65, 943–950. doi:10.1121/1.382599
Antonello, N., De Sena, E., Moonen, M., Naylor, P. A., and Van Waterschoot, T. (2017). Room impulse response interpolation using a sparse spatio-temporal representation of the sound field. IEEE/ACM Trans. Audio Speech Lang. Process. 25, 1929–1941. doi:10.1109/taslp.2017.2730284
Antweiler, C., and Symanzik, H. G. (1995). “Simulation of time variant room impulse responses,” in Proc. 1995 IEEE Int. Conf. Acoust., Speech, Signal Process. (ICASSP ’95), Detroit, MI, May 08-12, 1995, Vol. 5, 3031–3034. doi:10.1109/icassp.1995.479484
Antweiler, C., Telle, A., Vary, P., and Enzner, G. (2012). “Perfect-sweep NLMS for time-variant acoustic system identification,” in Proc. 2012 IEEE Int. Conf. Acoust., Speech, Signal Process. (ICASSP ’12), Kyoto, Japan, 25-30 March 2012, 517–520. doi:10.1109/ICASSP.2012.6287930
Borra, F., Gebru, I. D., and Markovic, D. (2019). “Soundfield reconstruction in reverberant environments using higher-order microphones and impulse response measurements,” in Proc. 2021 IEEE Int. Conf. Acoust., Speech, Signal Process. (ICASSP ’19), Brighton, Great Britain, 12-17 May 2019, 281–285. doi:10.1109/ICASSP.2019.8682961
Bruschi, V., Nobili, S., Cecchi, S., and Piazza, F. (2020). “An innovative method for binaural room impulse responses interpolation,” in Audio Engineering Society Convention 148 (AES148Conv).
Carty, B. (2010). “Movements in binaural space: issues in HRTF interpolation and reverberation, with applications to computer music,”. Ph.D. thesis (Maynooth, Ireland: National University of Ireland).
Cherniakov, M. (2003). An introduction to parametric digital filters and oscillators. John Wiley & Sons.
Crocco, M., and Bue, A. D. (2015). “Room impulse response estimation by iterative weighted l1-norm,” in Proc. 23rd European Signal Process. Conf. (EUSIPCO ’15), Nice, France, August 31-September 4, 2015, 1895–1899.
Das, O., Calamia, P., and Gari, S. V. A. (2021). “Room impulse response interpolation from a sparse set of measurements using a modal architecture,” in Proc. 2021 IEEE Int. Conf. Acoust., Speech, Signal Process. (ICASSP ’21), Toronto, Canada, 6-11 June 2021, 960–964. doi:10.1109/ICASSP39728.2021.9414399
Elko, G. W., Diethorn, E., and Gänsler, T. (2003). “Room impulse response variation due to temperature fluctuations and its impact on acoustic echo cancellation,” in Proc. 2003 Int. Workshop Acoustic Echo Noise Control (IWAENC ’03), Kyoto, Japan, September 8-11, 2003, 67–70.
Enzner, G. (2008). “Analysis and optimal control of lms-type adaptive filtering for continuous-azimuth acquisition of head related impulse responses,” in Proc. 2008 IEEE Int. Conf. Acoust., Speech, Signal Process. (ICASSP ’08), Las Vegas, Nevada, 31 March-4 April 2008, 393–396. doi:10.1109/ICASSP.2008.4517629
Enzner, G. (2010). “Bayesian inference model for applications of time-varying acoustic system identification,” in Proc. 18th European Signal Process. Conf. (EUSIPCO ’10), Aalborg, Denmark, August 23-27, 2010, 2126–2130.
Evers, C., Löllmann, H. W., Mellmann, H., Schmidt, A., Barfuss, H., Naylor, P. A., et al. (2020). The locata challenge: Acoustic source localization and tracking. IEEE/ACM Trans. Audio Speech Lang. Process. 28, 1620–1643. doi:10.1109/taslp.2020.2990485
Garcia-Gomez, V., and Lopez, J. J. (2018). “Binaural room impulse responses interpolation for multimedia real-time applications,” in AES 144th Convention (AES148Conv), Milan, Italy, May 23-26, 2018.
Geldert, A., Meyer-Kahlen, N., and Schlecht, S. J. (2023). “Interpolation of spatial room impulse responses using partial optimal transport,” in Proc. 2023 IEEE Int. Conf. Acoust., Speech, Signal Process. (ICASSP ’23), Rhodes Island, Greece, June 4-June 10, 2023, 1–5. doi:10.1109/ICASSP49357.2023.10095452
Hahmann, M., and Fernandez-Grande, E. (2022). A convolutional plane wave model for sound field reconstruction. J. Acoust. Soc. Amer. (JASA) 152 (5), 3059–3068. doi:10.1121/10.0015227
Hahn, N., and Spors, S. (2015). “Continuous measurement of impulse responses on a circle using a uniformly moving microphone,” in Proc. 23rd European Signal Process. Conf. (EUSIPCO ’15), Nice, France, August 31-September 4, 2015, 2536–2540. doi:10.1109/EUSIPCO.2015.7362842
Haneda, Y., Kaneda, Y., and Kitawaki, N. (1999). Common-acoustical-pole and residue model and its application to spatial interpolation and extrapolation of a room transfer function. IEEE Trans. Speech, Audio Process 7, 709–717. doi:10.1109/89.799696
Karakonstantis, X., Caviedes-Nozal, D., Richard, A., and Fernandez-Grande, E. (2024). Room impulse response reconstruction with physics-informed deep learning. J. Acoust. Soc. Amer. (JASA) 155 (2), 1048–1059. doi:10.1121/10.0024750
Katzberg, F., Mazur, R., Maass, M., Böhme, M., and Mertins, A. (2018). “Spatial interpolation of room impulse responses using compressed sensing,” in Proc. 2018 Int. Workshop Acoustic Signal Enhancement (IWAENC ’18), Tokyo, Japan, September 17–20, 2018, 426–430. doi:10.1109/IWAENC.2018.8521390
Kearney, G., Masterson, C., Adams, S., and Boland, F. (2009). “Dynamic time warping for acoustic response interpolation: Possibilities and limitations,” in Proc. 17th European Signal Process. Conf. (EUSIPCO ’09), Glasgow, Scotland, 24-28 August 2009, 705–709.
Kuhl, S., Nagel, S., Kabzinski, T., Antweiler, C., and Jax, P. (2018). “Tracking of time-variant linear systems: Influence of group delay for different excitation signals,” in Proc. 2018 Int. Workshop Acoustic Signal Enhancement (IWAENC ’18), Tokyo, Japan, September 17–20, 2018, 131–135. doi:10.1109/IWAENC.2018.8521372
Lin, Y., and Lee, D. (2006). Bayesian regularization and nonnegative deconvolution for room impulse response estimation. IEEE Trans. Signal Process 54, 839–847. doi:10.1109/tsp.2005.863030
Masterson, C., Kearney, G., and Boland, F. (2009). “Acoustic impulse response interpolation for multichannel systems using dynamic time warping,” in Proc. AES 35th Int. Conf. Audio for Games, London, United Kingdome, February 11-13, 2009.
Mignot, R., Daudet, L., and Ollivier, F. (2013). Room reverberation reconstruction: Interpolation of the early part using compressed sensing. IEEE Trans. Audio Speech Lang. Process. 21, 2301–2312. doi:10.1109/tasl.2013.2273662
Müller, M. (2007). “Dynamic time warping,” in Information retrieval for music and motion. Berlin, German: Springer, 69–84.
Naylor, P. A., and Gaubitch, N. D. (2010). Speech dereverberation. Vol. 2. Berlin, Germany: Springer.
Nophut, M., Preihs, S., and Peissig, J. (2024). Velocity-controlled Kalman filter for an improved echo cancellation with continuously moving microphones. J. Audio Eng. Soc. (JAES) 72, 33–43. doi:10.17743/jaes.2022.0116
Pezzoli, M., Perini, D., Bernardini, A., Borra, F., Antonacci, F., and Sarti, A. (2022). Deep prior approach for room impulse response reconstruction. Sensors 22, 2710. doi:10.3390/s22072710
Ratnarajah, A., Ananthabhotla, I., Ithapu, V. K., Hoffmann, P. F., Manocha, D., and Calamia, P. T. (2022). “Towards improved room impulse response estimation for speech recognition,” in Proc. 2023 IEEE Int. Conf. Acoust., Speech, Signal Process. (ICASSP ’23), Rhodes Island, Greece, 4-10 June 2023, 1–5. doi:10.1109/ICASSP49357.2023.10094770
Schissler, C., Stirling, P., and Mehra, R. (2017). “Efficient construction of the spatial room impulse response,” in 2017 IEEE Virtual Reality (VR), Los Angeles, CA, 18-22 March 2017, 122–130. doi:10.1109/VR.2017.7892239
Simon, D. (2006). Optimal state estimation: Kalman, H infinity, and nonlinear approaches. Hoboken, NJ: John Wiley & Sons.
Stan, G.-B., Embrechts, J.-J., and Archambeau, D. (2002). Comparison of different impulse response measurement techniques. J. Audio Eng. Soc. (JAES) 50, 249–262.
Szöke, I., Skácel, M., Mošner, L., Paliesek, J., and Černocký, J. H. (2018). Building and evaluation of a real room impulse response dataset. IEEE J. Sel. Topics Signal Process. (JSTSP) 13, 863–876. doi:10.1109/JSTSP.2019.2917582
Keywords: state-space model, transition matrix, acoustic room impulse response interpolation, time-varying system, dynamic time warping
Citation: MacWilliam K, Dietzen T, Ali R and van Waterschoot T (2024) State-space estimation of spatially dynamic room impulse responses using a room acoustic model-based prior. Front. Sig. Proc. 4:1426082. doi: 10.3389/frsip.2024.1426082
Received: 30 April 2024; Accepted: 01 August 2024;
Published: 02 September 2024.
Edited by:
David V. Anderson, Georgia Institute of Technology, United StatesReviewed by:
Jelena Ćertić, University of Belgrade, SerbiaVictor Lazzarini, Maynooth University, Ireland
Copyright © 2024 MacWilliam, Dietzen, Ali and van Waterschoot. This is an open-access article distributed under the terms of the Creative Commons Attribution License (CC BY). The use, distribution or reproduction in other forums is permitted, provided the original author(s) and the copyright owner(s) are credited and that the original publication in this journal is cited, in accordance with accepted academic practice. No use, distribution or reproduction is permitted which does not comply with these terms.
*Correspondence: Kathleen MacWilliam, a2F0aGxlZW4ubWFjd2lsbGlhbUBlc2F0Lmt1bGV1dmVuLmJl