- Department of Electrical and Computer Engineering, Temple University, Philadelphia, PA, United States
Integrating sensing and communication (ISAC) is a cutting-edge technology aimed at achieving high-resolution target sensing and high data-rate communications using a shared spectrum. This innovative approach optimizes the usage of the radio spectrum with no or minimal level of mutual interference. The capability of reconfigurable intelligent surface (RIS) to control the environment and provide additional degrees of freedom is driving the development of RIS-aided ISAC. In this mini-review, we provide an overview of the current state-of-the-art of RIS-aided ISAC technology, including various system configurations, approaches, and signal processing techniques.
1 Introduction
The needs for high-resolution sensing and high data-rate wireless communications demand broader frequency bands, resulting in increased spectrum congestion. An effective solution is to integrate sensing and communication infrastructures. The integrated sensing and communication (ISAC) technology improves spectral and energy efficiencies and reduce hardware costs (Hassanien et al., 2015; Hassanien et al., 2016; Mishra et al., 2019; Liu et al., 2020; Wu et al., 2022; Mishra et al., 2023). ISAC has a wide range of applications across various fields. In addition to supporting typical cellular wireless communications and radar sensing, it can also be used in geoscience, remote sensing, environmental monitoring, human-computer interaction, and autonomous vehicles (Cui et al., 2021; Elbir et al., 2021; Liu et al., 2022a; Cheng et al., 2022; Zhang et al., 2022; Xu et al., 2023).
ISAC systems can be classified into two main approaches, namely, radar-communications coexistence (RCC) and dual-functional radar-communications (DFRC) (Elbir et al., 2021; Chu et al., 2022). RCC systems employ efficient interference management techniques and optimized resource allocation in a manner that allows both communication and radar functions to operate effectively without unduly affecting each other. This involves designing the systems to avoid conflicts, minimize the impact of interference, and maximize system performance. On the other hand, DFRC systems perform both communication and radar tasks simultaneously through a shared transmitter.
Reconfigurable intelligent surface (RIS), also known as intelligent reflecting surface (IRS), is a metasurface typically constructed as a planar array of passive elements. An RIS is typically a two-dimensional array of small, passive, and reconfigurable elements. These arrays are engineered using advanced metamaterials that exhibit unique properties, such as negative refractive index, which enable fine-grained control over the direction and strength of reflected waves. Sophisticated signal processing techniques are employed to control the phase and amplitude of the reflected signals. By manipulating the propagation of electromagnetic waves in a wireless communication system, RISs can enhance signal quality, network capacity, and reduce interference (Basar et al., 2019; Boulogeorgos and Alexiou, 2020; Han et al., 2020).
For the convenience of hardware implementations, the RIS weights are often designed to be constant-modulus and use a limited number of discrete values in the phase shifters. RIS technology has received rapidly growing popularity because of its capability to control the propagation environment, thereby improving the performance of wireless sensing and communication systems. Offerings of RIS include achieving enhanced spectral efficiency, reduced base station (BS) transmit power requirement, better multi-user interference (MUI) mitigation, improved signal direction of arrival (DOA) estimation, and higher target localization and tracking accuracy (Asif Haider et al., 2022; Wei et al., 2022a; Shi et al., 2022; Asif Haider et al., 2023a). RISs have gained significant attention in recent years as a promising technology for 6G communications due to their aforementioned unique features. RISs can be deployed on various surfaces, including building facades, factory ceilings, and even human clothing. RIS-assisted wireless systems have been shown to outperform amplify-and-forward relaying counterparts in terms of signal-to-noise ratio (SNR), outage probability, symbol error rate, and ergodic capacity.
The implementation of ISAC systems faces several challenges. For example, ISAC systems encounter a unique problem of potential mutual interference (MI) between the sensing and communication functions. ISAC systems operating in the millimeter-wave band suffer from high propagation attenuation and their performance severely degrades when the line-of-sight (LOS) between the BS and the communication users (CUs) or targets is obstructed (Wei et al., 2022a; Shi et al., 2022). The RIS technology presents a solution to overcome these obstacles, thereby improving the sensing and communication capabilities and performance. By placing RISs between the BS, CUs, and targets and adaptive steering the beams, we can enhance the strength of both communication and sensing signals, provide LOS connections, extend the communication and sensing coverage, and reduce MI and MUI (Shtaiwi et al., 2021; He et al., 2022; Zhu et al., 2022). The RIS technology can also be used to provide additional physical layer security against eavesdroppers (Mishra et al., 2022). Figure 1 depicts differential scenarios where RIS-aided ISAC systems are used.
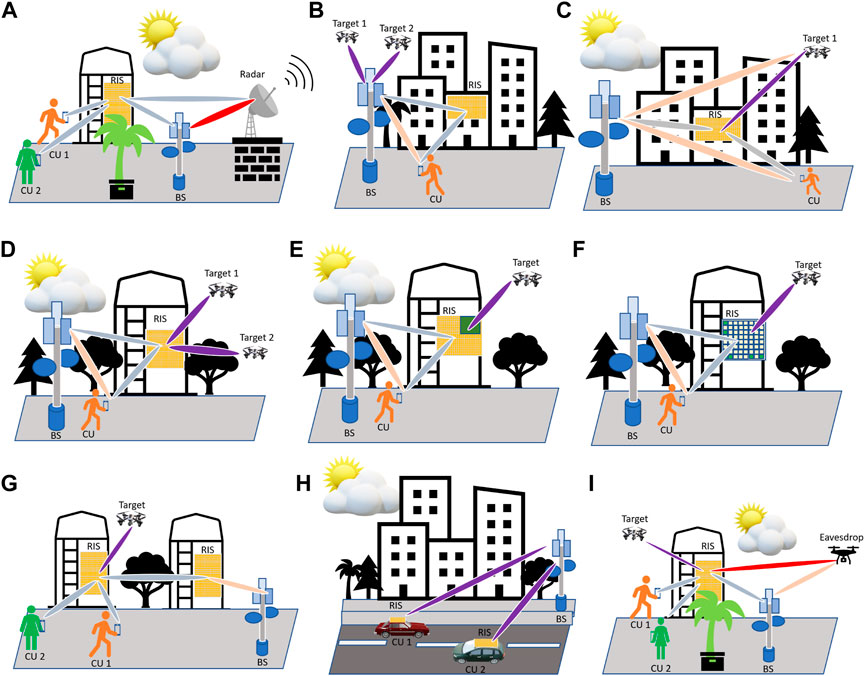
FIGURE 1. Examples of ISAC systems where RIS plays different roles: (A) RIS aids in interference management in RCC systems. (B) RIS assists communication only in DFRC systems where there is a strong LOS link between the BS and the targets. (C) A more general RIS-aided DFRC system involving both LOS and NLOS links. (D) In DFRC systems where the direct paths between the BS and the targets/users are blocked, RIS assists both sensing and communication. (E) Partitioning of the RIS elements for sensing and communication (F) Semi-passive elements are sparsely placed in the RIS for target detection and communication channel estimation. (G) Communication and sensing system aided by multiple RISs. (H) RIS-mounted users enhance localization and communication performance. (I) An RIS-aided DFRC system to enhance the secrecy rate in the presence of an eavesdropper.
In this mini-review, we provide an overview of the state-of-the-art research of RIS-aided ISAC systems. Such systems are classified based on their applications and configurations, and the optimization techniques exploited for maximizing system performance are also described. Table 1 summarizes the literature being studied in this mini-review.
2 Applications of RIS-aided ISAC systems
The applications of the RIS are vast in both RCC and DFRC systems. A critical issue in RCC systems is the interference management between the sensing and communication functions and among densely deployed wireless devices (Shtaiwi et al., 2021; Chu et al., 2022). By controlling the wireless environment, RIS can be used to boost desired signals and suppresses interference signals, thereby improving the performance of both communication and sensing tasks (He et al., 2022; Shi et al., 2022). As a result, exploiting an RIS in DFRC systems offers a range of benefits, including improved target detection, enhanced communication quality, more effective MI and MUI mitigation, and increased physical layer security (Jiang et al., 2021; Sankar et al., 2021; Wang et al., 2021; Liu et al., 2022b; Mishra et al., 2022; Song et al., 2022; Yan et al., 2022; Zhang, 2022; Zhu et al., 2022; Asif Haider et al., 2023b).
2.1 RIS-aided RCC
In (Shtaiwi et al., 2021), a large RIS is used in a multiple-input multiple-output (MIMO) RCC system to achieve a high beamforming gain and mitigate MI between communication and RADAR system, thereby improving the sum rate of the communication system subjected to the radar performance constraints. The transmit power of both the radar transmitter and the BS is kept within their respective budgets. The optimization problem of the BS transmit beamformer and the RIS phase shifters is solved using a local search approach. Incorporating RIS in NLOS systems establishes a communication link between users and the BS, and it is confirmed that using more RIS elements improves beamforming gain, enhances interference mitigation, and leads to a monotonically increasing sum-rate in the communication system. An RIS-aided multi-user MIMO RCC system is illustrated in Figure 1A.
A double-RIS RCC system is considered in (He et al., 2022) to improve the communication performance and reduce MI while guaranteeing the radar detection performance for each detection direction. To achieve this objective, a double-loop penalty dual decomposition-based algorithm is employed. Two scenarios are considered in which the radar transmit power is respectively high and low. In the former, a closed-form optimal solution for joint beamforming is derived, while in the latter, the MI is minimized using the block coordinate descent method. It is shown that introducing a single 100-element RIS to an RCC system improves the output communication signal-to-interference-plus-noise ratio (SINR) by 6 dB. On the other hand, a double-RIS-assisted system further suppresses interference and enhances the output communication SINR by 11 dB, highlighting the effectiveness of deploying two RISs.
2.2 RIS-aided DFRC
Studies conducted on DFRC systems that utilize RIS are concentrated on either optimizing communication performance while upholding a certain level of sensing performance, or optimizing the sensing performance while preserving a certain communication rate. Figures 1B, C respectively depict the scenarios where RIS assists either the communication or the sensing function. In Figure 1D, the targets are detected through virtual LOS connections from the BS to the targets via the RIS.
2.2.1 RIS enhancing communication performance
In (Zhu et al., 2022), the transmission rate of an ISAC system is enhanced while meeting the radar’s desired beampattern requirement by jointly designing the radar signal covariance matrix, the communication system’s beamforming vector, and the RIS phase shifters. The non-convex optimization problem is solved by splitting it into two sub-problems. First, a closed-form solution is obtained for the radar signal covariance matrix that achieves the desired beampattern. Joint optimization of the communication beamforming vector and RIS phase shifters is then carried out using the quadratic transformation and alternating optimization (AO) technique. The computational complexity is reduced by applying the majorization-minimization algorithm to optimize the RIS phase shifters using AO. By incorporating RIS, this algorithm attains a greater beam pattern gain and achieves a higher CU sum rate compared to the scenarios without using an RIS.
2.2.2 RIS deployed for enhanced sensing
In (Jiang et al., 2021), a solution is proposed to address the issue of a weak echo signal received by radar-aided BS in crowded areas with significant channel attenuation. Placing an RIS in the vicinity of the DFRC system provides additional transmission links to reduce channel attenuation and fading effects, thereby enhancing the radar detection performance. A joint optimization problem is formulated to maximize the output SNR at the radar receiver while satisfying both sensing and communication constraints. The optimization problem involves optimizing the precoding matrix of the radar-aided BS and the RIS phase shifters. The non-convex problem is solved using an AO approach and the majorization-minimization algorithm, followed by the semi-definite relaxation approach.
The problem addressed in (Yan et al., 2022) is similar to that in (Jiang et al., 2021), but it considers all the reflecting paths among the BS, RIS, and targets. This problem is divided into two sub-problems, which respectively optimize the transmit beamformer and the RIS phase shifters. The former sub-problem has a closed-form solution, while the latter is solved using the successive lower bound maximization (SLBM) method. By taking all the reflecting paths into account, this approach provides higher target output SNR compared to (Jiang et al., 2021).
In (Song et al., 2022), the transmit beamformer at the BS and the reflective beamformer at the RIS are jointly optimized in a situation where the BS cannot establish direct LOS links with the targets but can leverage both direct and reflected LOS/NLOS links with the CU for efficient communications. Utilizing the AO approach, the proposed design attains higher beampattern gains towards all targets while meeting the minimum output SNR requirement at the CU.
2.2.3 Performance trade-off
Performance trade-offs can be made between the sensing and communication functions. Consider a scenario that a BS has LOS only to the CUs but not to the targets, as illustrated in Figure 1E. A multi-stage hierarchical codebook method is developed to locate a single NLOS target and establish a reliable communication link with a single CU (Sankar et al., 2021). During the surveillance operations in the absence of detected targets, a small subset of RIS elements are used to perform surveillance with wide beams, while most of the RIS elements are utilized for the communication task. When targets are detected, to achieve high-precision target localization, more RIS elements can be designated for target localization. The proposed algorithm requires fewer snapshots compared to an exhaustive search scheme. Numerical results show that, by exploiting the RIS, the spectral efficiency of the MIMO system is more than doubled. More specifically, in the surveillance mode, as most of the RIS elements are used for communications, the spectral efficiency is increased by 30 bps/Hz. On the other hand, in the target localization mode, the spectral efficiency is improved by 28 bps/Hz, only slightly lower than that achieved in the surveillance mode.
Unlike multi-target detection approaches that are based on multiple rounds of channel estimation, the beampattern towards all targets is optimized using combined mutual information from all target channels (Zhang, 2022). This approach maximizes the overall utility, which combines information for sensing and the total data rate for communication. The optimization problem is divided into two sub-problems, with the first one optimizing the BS beamformer using a successive convex approximation (SCA), whereas the second one optimizing the RIS phase shifters using a manifold optimization-based algorithm. The RIS-aided system achieves a higher overall utility which increases with the number of elements. Such increase ranges between 20% and 45% when the number of RIS elements varies between 20 and 120.
3 Channel estimation with RIS-aided ISAC systems
Achieving the offerings of RIS-aided ISAC systems requires the channel state information (CSI) of the links between the BS, RIS, CUs, and targets. In this section, we outline various channel estimation approaches for RIS-aided ISAC systems.
3.1 Active or semi-passive RIS
In (Liu et al., 2022b), a distributed RIS system consisting of both passive and semi-passive RISs conducts location sensing and data transmission simultaneously for a single user scenario. A semi-passive RIS contains some active sensors that can be used to estimate the CSI associated with the RIS. Unlike other ISAC systems which aim to perform separate sensing and communication functions, the purpose of sensing here is to assist the communication function in the sense that the estimated CU location facilitates the optimization of the RIS beamformer. Each transmission period is divided into two-time blocks. During each time block, passive RIS elements reflect the uplink transmission signals from the CU to the BS while the semi-passive RIS elements estimate the CU’s location in a sensing mode. This is achieved by using the total least squares ESPRIT method and pairing the effective two-dimensional DOAs using the MUSIC algorithm. The estimated location from the first time block is then used for beamforming design at the BS in the second time block. By implementing this location sensing scheme, it is possible to achieve millimeter-level positioning accuracy. Additionally, the proposed beamforming design enables near-optimal bit rates at a single CU, even when only a small number of RIS elements are used.
In (Asif Haider et al., 2023b), a semi-passive RIS-aided ISAC system is considered where a small number of active RIS elements are sparsely placed in an L-shape structure, as shown in Figure 1F. With the large array aperture, this structure improves channel estimation performance and reduces the required length of the pilot overheads. The CU and target channels are first estimated using a two-dimensional interpolated covariance matrix of the array data signal and the MUSIC algorithm. Joint optimization of the BS beamformer and RIS phase shifters maximizes the beamforming gain towards the direction of desired sensing and ensures minimum output SNR at the CU. The study provides insights into the design for different arrangements of the active sparse elements for performance improvement without increasing hardware costs.
3.2 Deep learning approaches
A deep learning-based framework is considered for the estimation of the sensing and communication channels in an RIS-aided ISAC system (Liu et al., 2022c). Two deep neural networks (DNN) architectures are proposed where the first one is located at the ISAC BS to estimate the sensing channel, whereas the second one is assigned to each downlink CU equipment to estimate its communication CSI. The BS is operated in a full-duplex mode. The self-interference due to full-duplex operation is pre-estimated and compensated before estimating the BS-target-BS channel. The deep learning framework contains three hidden layers with two convolutional layers and a flattened layer which are used for the estimation of the communication CSI, whereas two feed-forward layers with the tanh activation function are used for sensing channel estimation. By training the DNN using multiple SNR conditions, this method can be applied to a broad range of SNR regions. It achieves better sensing and communication channel estimation performance compared to the least squares estimator benchmark scheme.
4 Miscellaneous considerations in RIS-aided ISAC
4.1 Discrete phase shifters
RIS beamformers often exploit discrete phase shifters to facilitate low-cost hardware implementations compared to continuous phase shifter counterparts. Discrete phase shifters use a limited number of phase levels, such as those based on PIN diodes or varactor diodes (Wu and Zhang, 2019). It is shown, however, that there is a trade-off between the discrete quantization levels and the achievable sum rate as well as sensing performance (Wang et al., 2021; Wei et al., 2022b; He et al., 2022; Zhu et al., 2022).
4.2 Wideband scenarios
Wideband signals support the transmission of a high data rate and provide higher sensing resolutions. An RIS-aided wideband DFRC system with multi-carrier signaling is considered in (Wei et al., 2022b). Because the direction of the beam can change significantly over the operating frequency range, the beam squint problem becomes more severe as the signal bandwidth increases. When this problem is not properly addressed, the beam may no longer be aligned with the intended target, thereby leading to degraded performance. The quantized phase-shifter model utilizes frequency-dependent transmit beamforming to overcome the beam-squint effect in a wideband DFRC system. By jointly designing the radar receive filter, transmit precoding matrix, and discrete phase shifters, the radar output SINR is maximized while the required level of communication SINR is maintained for single-antenna CUs. The resulting optimization problem is solved using the alternating maximization method.
4.3 Multiuser interference cancellation
In ISAC systems, the inclusion of sensing functionality presents a significant challenge as it limits the available degree of accuracy for waveform design, leading to increased MUI and reduced communication performance. In (Wang et al., 2021), RIS is explored to mitigate MUI, with joint optimization of beamforming at the BS and discrete RIS phase shifters to meet the Cramer-Rao bound (CRB) requirement for multi-target DOA estimation. To achieve this objective, the exact penalty method and manifold optimization approach are used for constant-modulus waveform optimization at the ISAC BS, and manifold optimization and successive optimization methods are used to optimize the discrete RIS phase shifters. The proposed algorithm yielded a significantly increased multi-user sum rate compared to pure ISAC systems without RIS.
4.4 Multiple RIS-aided ISAC
As shown in Figure 1G, multiple RISs can be utilized in ISAC systems to further enhance object tracking performance, maximize communication bit rate, mitigate MI, and increase user SINR (Wei et al., 2022a; He et al., 2022). In (He et al., 2022), the use of multiple RISs in an RCC system improves the MI suppression performance compared to a single-RIS scenario. The study in (Wei et al., 2022a) focuses on the joint design of frequency-dependent beamforming and phase shifters for a wideband orthogonal frequency division multiplexing (OFDM) transmit signaling scheme, aiming to maximize the average radar output SINR and the minimal communication output SINR among all users using an alternating maximization method. The non-convex problem comprises a maximin objective function with a difference of convex constraint, which is solved through the alternating direction method of multipliers (ADMM) and Dinkelbach’s methods. It is shown that, by utilizing two RISs, the proposed method achieves a 3.3 dB improvement of the radar output SINR improvement and a 0.9 dB enhancement of the minimal communication output SINR compared to the single-RIS counterpart.
4.5 RIS-mounted communication users and targets
In (Shao et al., 2022), the BS communicates with multiple devices in a full-duplex mode while simultaneously sensing their positions. As shown in Figure 1H, each device is equipped with an RIS to enhance the reflected echoes and passively transfer information to the BS through the reflection modulation. To address the challenges of joint localization and information retrieval at the BS, a grid-based parametric model is constructed and the problem is formulated as a compressive sensing problem. The study focuses on designing the BS receiver to jointly locate the positions of the devices and retrieve the information, which is transmitted from the CUs and transferred by the RISs, through the exploitation of the sparsity in the considered parameter grid. The results confirm that the proposed algorithms outperform commonly used compressive sensing algorithms, such as orthogonal matching pursuit (OMP) and sparse Bayesian learning (SBL), in terms of the achieved minimal error in the recovered transmit information, particularly in multi-user off-grid scenarios.
4.6 Clutter consideration
The presence of clutter can have a significant impact on the performance of target detection in ISAC systems. In (Liu et al., 2022d), the problem of target detection is considered in the presence of strong clutter while ensuring the quality of multi-user communications. The space-time adaptive processing technique is used in the BS to achieve effective target detection in the presence of strong clutter that spreads out over large ranges and angular regions. By optimizing the nonlinear spatio-temporal transmit waveform and receive filter, this method makes use of all available degrees of freedom in both the spatial and temporal domains to improve the target detection performance.
4.7 Physical layer security
The issue of eavesdropping in a DFRC system, as illustrated in Figure 1I, is considered in (Mishra et al., 2022). In this system, the radar target acts as an eavesdropper that receives a high-energy signal, leading to additional security challenges. A multi-antenna DFRC system is considered in the presence of multiple eavesdroppers, and a physical-layer approach is designed to maximize the secrecy rate while meeting the total transmit power constraint and maintaining a required output SINR for each radar target. The multi-eavesdropper multi-cast multi-antenna DFRC system is optimized to maximize the secrecy rate. The RIS is used to enhance the quality of signal transmission to users, which indirectly allows additional degrees of freedom for the injection of artificial noise to distort signals arriving at eavesdroppers.
5 Conclusion
This mini-review offers an overview of the latest research outcomes in the field of RIS-aided ISAC systems. RIS-aided ISAC systems have been proven to be effective in achieving efficient spectrum sharing between sensing and communication tasks in both DFRC and RCC systems. By incorporating RIS, ISAC systems can overcome a range of problems encountered in sensing and communication, including MUI, user channel estimation and NLOS target detection, data rate and target detection performance improvement, physical layer security, clutter suppression, and more. With RIS technology still evolving, there are numerous possibilities yet to be explored that could greatly enhance the performance of ISAC systems.
Author contributions
MA and YZ contributed to article review and the preparation and organization of this mini-review article. MA wrote the first draft of the manuscript. YZ revised the manuscript. All authors contributed to the article and approved the submitted version.
Conflict of interest
The authors declare that the research was conducted in the absence of any commercial or financial relationships that could be construed as a potential conflict of interest.
Publisher’s note
All claims expressed in this article are solely those of the authors and do not necessarily represent those of their affiliated organizations, or those of the publisher, the editors and the reviewers. Any product that may be evaluated in this article, or claim that may be made by its manufacturer, is not guaranteed or endorsed by the publisher.
References
Asif Haider, M., Chowdhury, M. W. T., and Zhang, Y. D. (2022). “Sparse channel estimation for IRS-aided systems exploiting 2-D sparse arrays,” in Proc. IEEE sensor array and multich. Signal process. Workshop (SAM) (IEEE, 31–35.
Asif Haider, M., Pavel, S. R., Zhang, Y. D., and Aboutanios, E. (2023). “Active IRS-assisted MIMO channel estimation and prediction,” in Ieee int. Conf. Acoust., speech signal process. (ICASSP).
Asif Haider, M., Zhang, Y. D., and Aboutanios, E. (2023). ISAC system assisted by RIS with sparse active elements. EURASIP J. Adv. Signal Process. 2023 (1), 20–22. doi:10.1186/s13634-023-00977-5
Boulogeorgos, A.-A. A., and Alexiou, A. (2020). Performance analysis of reconfigurable intelligent surface-assisted wireless systems and comparison with relaying. IEEE Access 8, 94 463–494 483. doi:10.1109/access.2020.2995435
Cheng, X., Duan, D., Gao, S., and Yang, L. (2022). Integrated sensing and communications (ISAC) for vehicular communication networks (VCN). IEEE Internet Things J. 9 (23), 23 441–523 451. doi:10.1109/jiot.2022.3191386
Chu, J., Liu, R., Li, M., Liu, Y., and Liu, Q. (2022). Joint secure transmit beamforming designs for integrated sensing and communication systems. IEEE Trans. Veh. Technol.
Cui, Y., Liu, F., Jing, X., and Mu, J. (2021). Integrating sensing and communications for ubiquitous IoT: Applications, trends, and challenges. IEEE Netw. 35 (5), 158–167. doi:10.1109/mnet.010.2100152
Basar, E., Di Renzo, M., De Rosny, J., Debbah, M., Alouini, M.-S., and Zhang, R. (2019). Wireless communications through reconfigurable intelligent surfaces. IEEE Access 7. 116753–116773. doi:10.1109/access.2019.2935192
Elbir, A. M., Mishra, K. V., and Chatzinotas, S. (2021). Terahertz-band joint ultra-massive MIMO radar-communications: Model-based and model-free hybrid beamforming. IEEE J. Sel. Top. Signal Process. 15 (6), 1468–1483. doi:10.1109/jstsp.2021.3117410
Han, Y., Zhang, S., Duan, L., and Zhang, R. (2020). Cooperative double-IRS aided communication: Beamforming design and power scaling. IEEE Wirel. Commun. Lett. 9 (8), 1206–1210. doi:10.1109/lwc.2020.2986290
Hassanien, A., Amin, M. G., Zhang, Y. D., and Ahmad, F. (2015). Dual-function radar-communications: Information embedding using sidelobe control and waveform diversity. IEEE Trans. Signal Process. 64 (8), 2168–2181. doi:10.1109/tsp.2015.2505667
Hassanien, A., Amin, M. G., Zhang, Y. D., and Ahmad, F. (2016). Signaling strategies for dual-function radar communications: An overview. IEEE Aerosp. Electron. Syst. Mag. 31 (10), 36–45. doi:10.1109/maes.2016.150225
He, Y., Cai, Y., Mao, H., and Yu, G. (2022). RIS-assisted communication radar coexistence: Joint beamforming design and analysis. IEEE J. Sel. Areas Commun.
Jiang, Z.-M., Rihan, M., Zhang, P., Huang, L., Deng, Q., Zhang, J., et al. (2021). Intelligent reflecting surface aided dual-function radar and communication system. IEEE Syst. J. 16 (1), 475–486. doi:10.1109/jsyst.2021.3057400
Liu, C., Hu, X., Peng, M., and Zhong, C. (2022). “Sensing for beamforming: An IRS-enabled integrated sensing and communication framework,” in Proc. IEEE int. Conf. Commun. (ICC), 5567–5572.
Liu, F., Cui, Y., Masouros, C., Xu, J., Han, T. X., Eldar, Y. C., et al. (2022). Integrated sensing and communications: Toward dual-functional wireless networks for 6G and beyond. IEEE J. Sel. Areas Commun. 40 (6), 1728–1767. doi:10.1109/jsac.2022.3156632
Liu, F., Masouros, C., Petropulu, A. P., Griffiths, H., and Hanzo, L. (2020). Joint radar and communication design: Applications, state-of-the-art, and the road ahead. IEEE Trans. Commun. 68 (6), 3834–3862. doi:10.1109/tcomm.2020.2973976
Liu, R., Li, M., Liu, Y., Wu, Q., and Liu, Q. (2022). Joint transmit waveform and passive beamforming design for RIS-aided DFRC systems. IEEE J. Sel. Top. Signal Process. 16 (5), 995–1010. doi:10.1109/jstsp.2022.3172788
Liu, Y., Al-Nahhal, I., Dobre, O. A., and Wang, F. (2022). “Deep-learning-based channel estimation for IRS-assisted isac system,” in Proc. IEEE global commun. Conf. (GLOBECOM) (IEEE), 4220–4225.
Mishra, K. V., Chattopadhyay, A., Acharjee, S. S., and Petropulu, A. P. (2022). “OptM3Sec: Optimizing multicast IRS-aided multiantenna DFRC secrecy channel with multiple eavesdroppers,” in Proc. IEEE int. Conf. Acoust., speech signal process. (ICASSP), 9037–9041.
Mishra, K. V., Shankar, M. B., Koivunen, V., Ottersten, B., and Vorobyov, S. A. (2019). Toward millimeter-wave joint radar communications: A signal processing perspective. IEEE Signal Process. Mag. 36 (5), 100–114. doi:10.1109/msp.2019.2913173
Mishra, K. V., Shankar, M. B., Ottersten, B., and Swindlehurst, A. L. (2023). Signal processing for joint radar communications. John Wiley.
Sankar, R. P., Deepak, B., and Chepuri, S. P. (2021). “Joint communication and radar sensing with reconfigurable intelligent surfaces,” in Proc. IEEE int. Workshop signal process. Adv. Wireless commun. (SPAWC), 471–475.
Shao, Z., Yuan, X., Zhang, W., and Di Renzo, M. (2022). “Joint localization and information transfer for RIS aided full-duplex systems,” in Proc. IEEE global commun. Conf. (GLOBECOM), 3253–3258.
Shi, W., Xu, W., You, X., Zhao, C., and Wei, K. (2022). Intelligent reflection enabling technologies for integrated and green internet-of-everything beyond 5G: Communication, sensing, and security. IEEE Wireless Commun.
Shtaiwi, E., Zhang, H., Abdelhadi, A., and Han, Z. (2021). “Sum-rate maximization for RIS-assisted radar and communication coexistence system,” in Proc. IEEE global commun. Conf. (GLOBECOM), 1–6.
Song, X., Zhao, D., Hua, H., Han, T. X., Yang, X., and Xu, J. (2022). “Joint transmit and reflective beamforming for IRS-assisted integrated sensing and communication,” in Proc. IEEE wireless commun. Netw. Conf. (WCNC), 189–194.
Wang, X., Fei, Z., Huang, J., and Yu, H. (2021). Joint waveform and discrete phase shift design for RIS-assisted integrated sensing and communication system under Cramér-Rao bound constraint. IEEE Trans. Veh. Technol. 71 (1), 1004–1009. doi:10.1109/tvt.2021.3122889
Wei, T., Wu, L., Mishra, K. V., and Shankar, M. B. (2022). “IRS-aided wideband dual-function radar-communications with quantized phase-shifts,” in Proc. IEEE sensor array and multich. Signal process. Workshop (SAM), 465–469.
Wei, T., Wu, L., Mishra, K. V., and Shankar, M. B. (2022). “Multiple IRS-assisted wideband dual-function radar-communication,” in Proc. IEEE int. Symp. Joint commun. Sensing (JC&S), 1–5.
Wu, K., Zhang, J. A., and Guo, Y. J. (2022). Joint communications and sensing: From fundamentals to advanced techniques. John Wiley.
Wu, Q., and Zhang, R. (2019). Beamforming optimization for wireless network aided by intelligent reflecting surface with discrete phase shifts. IEEE Trans. Commun. 68 (3), 1838–1851. doi:10.1109/tcomm.2019.2958916
Xu, L., Sun, S., Zhang, Y. D., and Petropulu, A. (2023). “Joint antenna selection and beamforming in integrated automotive radar sensing-communications with quantized double phase shifters,” in Ieee int. Conf. Acoust., speech signal process. (ICASSP).
Yan, S., Cai, S., Xia, W., Zhang, J., and Xia, S. (2022). “A reconfigurable intelligent surface aided dual-function radar and communication system,” in Proc. IEEE int. Symp. Joint commun. Sensing (JC&S), 1–6.
Zhang, H. (2022). Joint waveform and phase shift design for RIS-assisted integrated sensing and communication based on mutual information. IEEE Commun. Lett. 26 (10), 2317–2321. doi:10.1109/lcomm.2022.3195062
Zhang, R., Jiang, C., Wu, S., Zhou, Q., Jing, X., and Mu, J. (2022). Wi-Fi sensing for joint gesture recognition and human identification from few samples in human-computer interaction. IEEE J. Sel. Areas Commun. 40 (7), 2193–2205. doi:10.1109/jsac.2022.3155526
Keywords: reconfigurable intelligent surface (RIS), integrated sensing and communication (ISAC), RIS-aided ISAC, dual-functional radar-communication (DFRC), radar-communication coexistence (RCC)
Citation: Asif Haider M and Zhang YD (2023) RIS-aided integrated sensing and communication: a mini-review. Front. Sig. Proc. 3:1197240. doi: 10.3389/frsip.2023.1197240
Received: 30 March 2023; Accepted: 17 April 2023;
Published: 05 May 2023.
Edited by:
Fulvio Gini, University of Pisa, ItalyReviewed by:
Brian Ng, University of Adelaide, AustraliaCopyright © 2023 Asif Haider and Zhang. This is an open-access article distributed under the terms of the Creative Commons Attribution License (CC BY). The use, distribution or reproduction in other forums is permitted, provided the original author(s) and the copyright owner(s) are credited and that the original publication in this journal is cited, in accordance with accepted academic practice. No use, distribution or reproduction is permitted which does not comply with these terms.
*Correspondence: Yimin D. Zhang, ydzhang@temple.edu