- 1NASA Goddard Institute for Space Studies, New York City, NY, United States
- 2Terra Research Inc, Hoboken, NJ, United States
This conceptual study presents advanced radiative transfer computations of light polarization originating from a snowpack consisting of nonspherical grains and variable content of light-absorbing impurities, either embedded in the snowpack or (with the same optical properties) lofted above it in the form of atmospheric aerosols. The results highlight the importance of considering shapes other than spherical for the snow grains, which otherwise can lead to non-negligible errors in the retrieval of snow albedo from remote sensing observations. More importantly, it is found that polarimetric measurements provide a means to partition light-absorbing impurities embedded in the snowpack from absorbing aerosols aloft, a task traditionally prohibitive for sensors capable exclusively of measurements of total reflectance. Heritage techniques to obtain snow grain size from shortwave infrared observations of total reflectance are well established, as are those that leverage polarimetric, multiangular observations across the entire optical spectrum to characterize the optical and microphysical properties of atmospheric aerosols. The polarization signatures of near-infrared (e.g., 864 nm) observations carry critical information on snow grain shape. The prospected launch of space-borne polarimeters with proven accuracy, therefore, advocates for the development of data inversion schemes, to boost the accuracy of simultaneous retrievals of atmospheric and surface parameters in the polar and snow-covered regions, critical to climate studies.
1 Introduction
The scientific interest in quantifying the surface energy and mass balance (SEMB) of the ice sheets is obvious when considering the potential impacts on global climate of variations in the brightness of such regions (Hansen and Nazarenko, 2004; Fettweis et al., 2008; van den Broeke et al., 2011; Rae et al., 2012; Van Angelen et al., 2012; Tedesco et al., 2013; Alexander et al., 2014; Colgan et al., 2014). On the other hand, upper estimates of the projected sea level rise are still very uncertain because of poor knowledge of ice sheet dynamics and the SEMB in the polar areas (Van Angelen et al., 2012), which demand a better characterization of their albedo.
As the albedo of snow-covered regions depends on the microphysical and optical properties of the constituent ice crystals (Wiscombe and Warren, 1980; Aoki et al., 2000; Bartelt and Lehning, 2002; Flanner and Zender, 2006; Bougamont et al., 2007; Dang et al., 2016; He et al., 2018), and on the content of light-absorbing impurities (LAIs) (Warren and Wiscombe, 1980; Hansen and Nazarenko, 2004; Dumont et al., 2014), a better knowledge of such properties and their evolution is a high-priority objective for the remote sensing of the cryosphere (Tedesco et al., 2013; Dumont et al., 2014). In developing techniques for the retrieval of these climatologically relevant parameters (Krabill et al., 2004; Comiso, 2006; Zwally and Giovinetto, 2011; Zatko et al., 2013), particular attention has been given to the Greenland ice sheet (GrIS) (Hori et al., 2007; Tedesco et al., 2011, 2013; Box et al., 2012), for which quantitative explanations of the ascertained mass losses are still lacking (Velicogna, 2009). Aerosol (especially black carbon) transport (Dumont et al., 2014; Tedesco et al., 2016) and deposition (Doherty et al., 2014), consolidation of LAIs (including black and organic carbon, and dust) through melt and scavenging, exposure of bare ice, and biogenic contributions cause large variations in the albedo of the GrIS and deeply affect its SEMB, especially in the topographically complex coastal region known as the ablation zone (Alexander et al., 2014; Schmale et al., 2021). A reduction in surface albedo can increase the likelihood of wide swaths of melt, which can fundamentally change the structure of the ice sheets, thus leading to further changes in the albedo and meltwater retention. It is, therefore, essential to understand these factors and how well these changes are captured in the climate models used to simulate and project the GrIS SEMB. Advanced Radiative Transfer (RT) algorithms have been developed to extend the current knowledge to operational products of climatological relevance (Nolin and Dozier, 2000; Stamnes et al., 2007; Jin et al., 2008; Zege et al., 2008; Lyapustin et al., 2009; Painter et al., 2009; Kokhanovsky et al., 2011; Stamnes et al., 2011; Stroeve et al., 2013). For example, the retrieval of grain size in conjunction with standard thermal infrared techniques to infer surface temperature such as split-window algorithms (Key and Haefliger, 1992), gives information on the snow age and metamorphic state and, therefore, an indication of the onset of melting. Many of the retrieval schemes have been applied to the reflectance data from the MODerate resolution Imaging Spectroradiometer (MODIS) onboard the Terra and Aqua satellites, but the information content is often insufficient to unambiguously resolve the vertical distribution of grain size, nonsphericity, and LAI content (Warren, 2013) in the snow medium, critical to accurately estimate the albedo and its recent trends (Xie et al., 2006; Tedesco et al., 2013; Dumont et al., 2014; Dang et al., 2016; Tedesco et al., 2016).
Poorly constrained by the correct optical properties, many RT studies model snow as a collection of spherical grains. It is now established that such an assumption biases the derived albedo low by a few percent (Xie et al., 2006; Tedesco and Kokhanovsky, 2007; Kokhanovsky et al., 2011; Libois et al., 2013; Tedesco et al., 2013; Dumont et al., 2014; Dang et al., 2016; Räisänen et al., 2017). Such biases can be largely amplified by the feedback processes, measurably altering the modeled forcing in polar regions. Furthermore, since the crystal habit determines the asymmetry parameter, the assumption of spherical grains also impacts grain size retrievals, with additional inaccuracies in determining shortwave absorption. In fact, errors in the albedo due to wrong assumptions on grain shape can be offset by adjusting the grain size (Dang et al., 2016; Räisänen et al., 2017).
Decoupling the competing contributions of grain size and LAIs in determining absorption within the snowpack is an unresolved issue of undoubted urgency (Dumont et al., 2014; Tedesco et al., 2016), also affecting the altimetric measurements (Kwok, R., priv. comm.). Differentiating LAIs in the snow from atmospheric aerosols, of paramount climatological importance (Hansen and Nazarenko, 2004; Dumont et al., 2014), has proven to be very difficult (Warren, 2013).
2 Methods and Simulations
A most striking feature of ice is the eightfold-order-of-magnitude increase in absorption as the observational wavelength shifts from the visible part of the spectrum toward the Near-InfraRed (NIR) (Warren, 2019). For this reason, the total reflectance in the NIR is virtually unaffected by the presence of extraneous LAIs (Wiscombe and Warren, 1980), while in the visible even a minimal amount of LAIs is sufficient to cause a drastic reduction in the albedo. This phenomenon has received much attention since the darkening of the snowpack increases the absorption of radiation in the snow, potentially generating a runaway feedback mechanism (Nolin and Frei, 2003; Hansen and Nazarenko, 2004).
In terms of grain shape, the first natural approach to snow modeling is that of assuming a collection of spheres. Due to the lack of information about crystal shape, some authors (Stamnes et al., 2007) have employed the Henyey–Greenstein phase function (Henyey and Greenstein, 1941), after running Mie computations on spheres of the desired effective radii to determine the asymmetry parameter. The H–G phase function is an ad hoc way to mimic particle roughness by eliminating the resonant structures (rainbow and glory) associated with scattering from perfect spheres, not observed in snow. A growing body of research points out the limitations of using spherical shapes in the modeling of optical properties of snow grains (Xie et al., 2006; Tedesco and Kokhanovsky, 2007; Kokhanovsky et al., 2011; Libois et al., 2013; Tedesco et al., 2013; Dang et al., 2016; Räisänen et al., 2017; He et al., 2018). A database of ice crystal optical properties was therefore constructed at the NASA Goddard Institute for Space Studies (GISS), based on ray tracing and Geometric Optics (GO) (Macke et al., 1996; van Diedenhoven et al., 2012). Although snow and ice crystals can assume complex aggregated shapes, we used the fact that hexagonal columns and plates have been shown to be adequate radiative proxies for more elaborated structures (Fu, 2007; van Diedenhoven et al., 2012, 2014a). The results for hexagonal prisms were tabulated as a function of the ratio of their dimensions (the Aspect Ratio, AR, with AR>1 for columns and AR<1 for plates) and the microscale roughness of the crystal facets (Macke et al., 1996; Yang et al., 2008). Analogous to the Cox and Munk variance used for the water-wave slopes (Cox and Munk, 1956), the latter numerically represents the standard deviation of the distribution of angles used to randomly perturb the orientation of a crystal facet when the beam encounters it in the GO simulations. For a given combination of aspect ratio and roughness, the asymmetry parameter does not depend on the crystal size for the weakly absorbing wavelengths (van Diedenhoven et al., 2014a, 2012) such as 864 nm. This database yielded successful polarimetric retrievals for parameters descriptive of the crystals forming ice clouds (van Diedenhoven et al., 2014b).
The implementation of these aspects into a retrieval scheme addresses the long-standing challenges which limit the accurate determination of the relationship between the snow grain size and shape with the surface temperature, LAI content, location, and season. Retrievals exploiting the GO database of nonspherical ice crystals have been tested on airborne measurements over snow surfaces collected with the NASA GISS Research Scanning Polarimeter (RSP), and confirmed the superior capabilities of polarimetry to retrieve the snow grain size, shape, and microscale roughness (Ottaviani et al., 2015, 2012, hereafter referred to as O15 and O12). At the heart of such retrievals, and also used here, is a forward RT model based on the general doubling-adding formalism described by De Haan et al. (1987), which is capable to simulate the stokes vector of the light field at any desired set of illumination and viewing angles and altitude above the surface. Both the light field and the partial derivatives with respect to the individual parameters can be determined from a single run of the code without the additional computational cost, should the linearization of the model be desired in order to feed an inversion scheme (Rodgers, 2000). The code includes a rigorous treatment of gaseous absorption and multiple scattering processes by molecules, aerosols, and clouds. Bimodal, log-normal aerosol size distributions (Hansen and Travis, 1974) are typically employed for aerosols. The code has been validated against the benchmark results (see Appendix in De Haan et al. (1987)), and is routinely used at GISS to model the RSP measurements over land and ocean for the retrieval of aerosol, cloud, and surface properties (e.g., Waquet et al. (2009); Knobelspiesse et al. (2011, 2012); Ottaviani et al. (2019)).
In a preliminary study, we applied a root-mean-square-error search through the GO database to the surface polarized reflectance signal isolated after an accurate atmospheric correction from the measurements acquired during a survey of alpine snowfields in the Sierra Nevada range (O12). The collection of these measurements, the first of its kind, was personally designed during the Carbonaceous Aerosol and Radiative Effects Study (CARES) mission. The results pointed to snow composed of, or behaving as, a collection of ice crystals with roughness values of ∼0.3 and above, a range of quite extreme aspect ratios, and correspondingly high asymmetry parameters (g ∼ 0.85). Subsequently, a dedicated science flight over a dozen targets in Colorado and California was designed during the POlarimeter Definition EXperiment (PODEX) campaign, which served as a training activity for the inter-agency Studies of Emissions and Atmospheric Composition, Clouds, and Climate Coupling by Regional Surveys (SEAC4RS) mission, with the RSP deployed on the ER-2 aircraft. With 95% of the atmospheric mass residing below the nominal cruising altitude, this stratospheric platform provides a perspective close to that of a satellite. The analysis (O15) replicated the results from the previous limited RSP dataset, confirming the solidity of the method. Building on the improved estimates of the asymmetry parameter, the results were also augmented with grain size retrievals from the RSP shortwave infrared measurements in a MODIS-like fashion (Lyapustin et al., 2009).
The O12 and O15 studies did not include the basic plots showing the sensitivity of the polarization signal to the crystal habits spanned by the GO dataset. For this reason, Figure 1 presents the simulations of the radiances emerging from a 1 m-thick, single-layer snowpack (density = 0.1 g/cm3, optically semi-infinite), with an exponential distribution of hexagonal prism ice grains of different ARs, and with effective radii of 50 μm typical of fresh snow conditions. The roughness parameter was set to 0.3 as retrieved from the real data (O15, O12). The upper and lower rows correspond to the total reflectance and the polarized reflectance, respectively, defined as Rp =
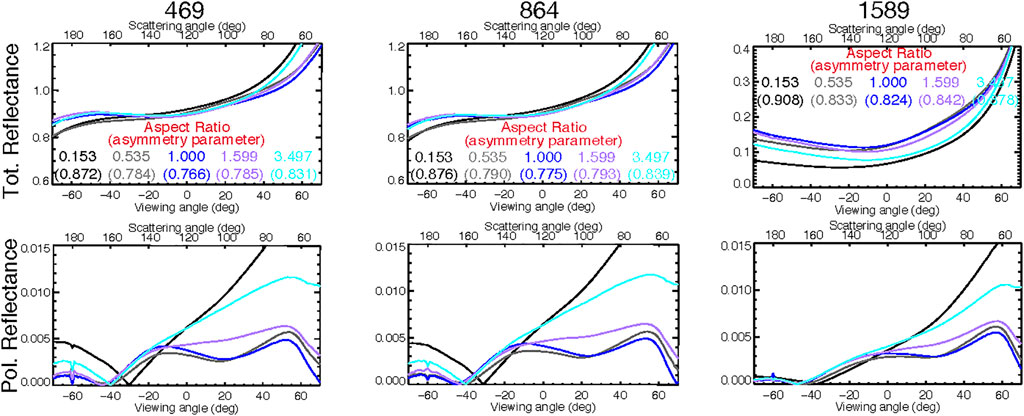
FIGURE 1. Top row: total reflectance (469, 864 and 1,589 nm) emerging from a snow layer observed along the principal plane, for SZA = 60°. Different colors correspond to different grain shapes (reff = 50 μm) and microscale roughness equal to 0.30. Bottom row: the corresponding polarized reflectance, also showing sensitivity.
Since the intention of Figure 1 is to highlight the effects of crystal shape on the surface contribution (i.e., on a signal that has hypothetically been atmospherically corrected), no atmosphere is included. The sensitivity to crystal shape emerging from these simulations is an important result, given that the total reflectance at absorbing wavelengths is used to estimate the grain size (in the top layer of a centimeter or so depending on the specific penetration depth), and demonstrates that the retrievals can be improved by including polarization to achieve a better determination of the grain shape. Albeit low, the magnitude of the polarized reflectance does not cause detection problems given the polarimetric accuracy and the signal-to-noise ratio of heritage (POLDER (Fougnie et al., 2007; van Diedenhoven et al., 2012)) and upcoming polarimeters, such as those slated for launch onboard the NASA Plankton, Aerosol, Ocean, and Ecosystem (PACE) and the European MetOP-SG (Lang et al., 2019) satellite missions. More importantly, the polarized signal carries specific angular signatures. A substantial variation in the behavior occurs for crystal plates across the AR value of 0.5, mirrored by a jump in the asymmetry parameter as large as 0.05. This situation is replicated to a lesser extent by columns as the AR varies across the reciprocal value (i.e., AR = 2).
To simulate the sensitivity of satellite observations to impurities in or above the snow, a crystal habit with AR = 1 (blue curves in Figure 1) was used to model the bottom layer of a model atmosphere. Contamination was added in the form of plausible, increasing concentrations of 1) impurities embedded in the snowpack (0, 0.01, 0.1, and 10 ppmw of soot as in Fig. 7 of Warren and Wiscombe (1980)), with a refractive index n = 1.8–0.6i and density of 2 g/cm3) and 2) tropospheric aerosol similar to the “Boreal Forest” smoke class by Dubovik et al. (2002), with a refractive index n = 1.5–0.01i and the optical depths at 555 nm equal to 0, 0.1, 0.4, 0.8, and 1.6. The impurities in the snowpack are considered as externally mixed to the snow grains, and their optical properties are calculated by Mie calculations internal to the RT code, as done for the aerosols. The results of these simulations are shown in Figures 2, 3, where the columns pertain to the same wavelengths as in Figure 1. The TOA polarized reflectance at 469 nm is dominated by Rayleigh scattering. At all wavelengths, comparing the two figures, it is evident that the model predicts very different TOA reflectances depending on whether the contamination is embedded in the snowpack or suspended above it (tropospheric aerosols), because the overwhelming amount of multiple scattering in the snowpack suppresses any polarization effect due to the small fraction of LAIs. A complete sensitivity study on an extended set of atmospheric aerosols is outside the scope of this article, and does not invalidate the basic finding that the polarization signatures of aerosols overwhelm those of the impurities, which are de facto null.
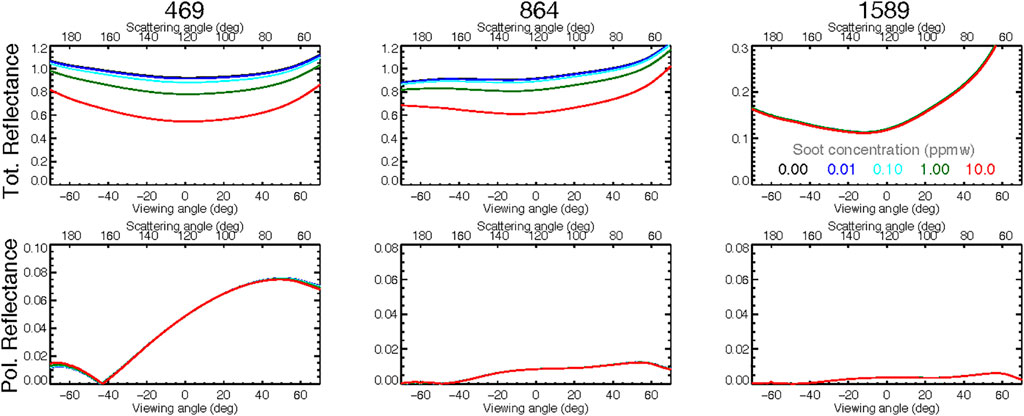
FIGURE 2. The effect of growing concentrations of LAIs in a snowpack consisting of nonspherical grains (AR = 1, roughness = 0.3) on the radiances emerging at the top of a standard atmosphere. The total reflectance (top row) experiences the well-known depression in the visible. The polarized reflectance (bottom row) is essentially unaffected.
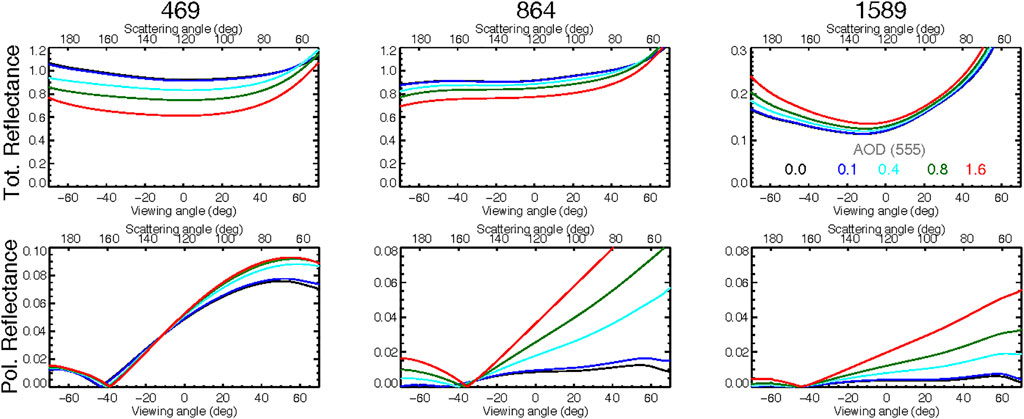
FIGURE 3. As in Figure 2, but for increasing the loads of absorbing aerosols. The response of the TOA total reflectance is similar in the two figures, but now the known polarized reflectance sensitivity to aerosols enables to distinguish them from the LAIs.
We also modeled (not shown) the impurities embedded in the snowpack with the optical properties typical of dust (n = 1.5–0.01i, r eff = 1 μm, v eff = 0.3, density = 2.6 g/cm3 and concentrations of 1, 10, 100, 1,000 ppmw), obtaining the same insensitivity of the polarized reflectance as obtained for soot. These findings confirm that it is impossible to differentiate between the two kinds of contaminants as long as their refractive indices, although different, are taken as spectrally invariant. To this regard, note that the recent efforts have aimed at estimating the absorption properties of these contaminants further into the UV region where dust absorption increases dramatically (Müller et al., 2008; Moosmüller et al., 2009; Wagner et al., 2012), since some of the current space-borne sensors such as the Thermal And Near-infrared Sensor for carbon Observation (TANSO), the Cloud and Aerosol Imager (CAI) on GOSAT, and the JAXA Second-generation GLobal Imager (SGLI) on GCOM-C1 have moderate (1 km) resolution observations at 380 nm.
3 Conclusion and Outlook
The simulations presented in this study confirm the importance of accounting for the grain shape in remote sensing of snow properties, and illustrate the benefits of polarimetry in correctly partitioning LAIs between the snowpack and the atmosphere. For instruments equipped with polarization and multiangular capabilities, they suggest that a comprehensive database of ice crystals’ shapes can be exploited within a simultaneous inversion scheme to largely improve the description of both the surface and atmospheric parameters, and avoid unphysical correlations stemming from a lack of information content. After a tailored atmospheric correction procedure applied to dedicated airborne measurements collected by the RSP sensor and previously published (O15 and O12), we are in the process of constructing a general inversion scheme, and we prospect in the near future to validate the model simulations presented here starting from the augmentation of MODIS-like observations with the data from the POLDER sensor, insofar the only space-borne polarimeter with the sufficient capabilities ever to furnish a reliable data record.
The snow grain shape retrievals hinge on the exploitation of multi-angle, polarimetric measurements at NIR wavelengths. Together with traditional, MODIS-like techniques for the retrieval of grain size and impurity content based on VIS and SWIR channels, the results are therefore of special interest for the development of novel algorithms to be applied to the next generation of polarimeters planned for upcoming space missions, such as PACE and those identified in the Decadal Survey Report, or the European 3MI (Lang et al., 2019) sensor onboard MetOP-SG. The results from polarimeters with limited angular capabilities, such as JAXA’s SGLI sensor, are to be evaluated with rigorous information content studies.
Data Availability Statement
The results of the simulations can be made available by the author upon request.
Author Contributions
MO conceived this study, carried out the simulations, and wrote the manuscript.
Funding
This work was partially supported by the NASA Remote Sensing Theory program (grant #80NSSC21K0569).
Conflict of Interest
MO was employed by Terra Research Inc.
Publisher’s Note
All claims expressed in this article are solely those of the authors and do not necessarily represent those of their affiliated organizations, or those of the publisher, the editors, and the reviewers. Any product that may be evaluated in this article, or claim that may be made by its manufacturer, is not guaranteed or endorsed by the publisher.
Acknowledgments
The author wishes to thank Bastiaan van Diedenhoven, Nan Chen, and Knut Stamnes for continuing discussions related to the project.
References
Alexander, P. M., Tedesco, M., Fettweis, X., Van De Wal, R. S. W., Smeets, C. J. P. P., and Van Den Broeke, M. R. (2014). Assessing Spatio-Temporal Variability and Trends in Modelled and Measured Greenland Ice Sheet Albedo (2000-2013). Cryosphere 8, 2293–2312. doi:10.5194/tc-8-2293-2014
Aoki, T., Aoki, T., Fukabori, M., Hachikubo, A., Tachibana, Y., and Nishio, F. (2000). Effects of Snow Physical Parameters on Spectral Albedo and Bidirectional Reflectance of Snow Surface. J. Geophys. Res. 105, 10219–10236. doi:10.1029/1999jd901122
Bartelt, P., and Lehning, M. (2002). A Physical SNOWPACK Model for the Swiss Avalanche Warning: Part I: Numerical Model. Cold Regions Sci. Technol. 35, 123–145. doi:10.1016/S0165-232X(02)00074-5
Bougamont, M., Bamber, J. L., Ridley, J. K., Gladstone, R. M., Greuell, W., Hanna, E., et al. (2007). Impact of Model Physics on Estimating the Surface Mass Balance of the Greenland Ice Sheet. Geophys. Res. Lett. 34, L17501. doi:10.1029/2007gl030700
Box, J. E., Fettweis, X., Stroeve, J. C., Tedesco, M., Hall, D. K., and Steffen, K. (2012). Greenland Ice Sheet Albedo Feedback: Thermodynamics and Atmospheric Drivers. Cryosphere 6, 821–839. doi:10.5194/tc-6-821-2012
Colgan, W., Box, J. E., Fausto, R. S., van As, D., Barletta, V. R., and Forsberg, R. (2014). Surface Albedo as a Proxy for the Mass Balance of Greenland’s Terrestrial Ice. Geol. Surv. Denmark Greenland Bull. 31, 93–96. doi:10.34194/geusb.v31.4671
Comiso, J. C. (2006). Arctic Warming Signals from Satellite Observations. Wea 61, 70–76. doi:10.1256/wea.222.05
Cox, C., and Munk, W. (1956). Slopes of the Sea Surface Deduced from Photographs of Sun Glitter. Bull. Scripps Inst. Oceanogr. 6, 401–488.
Dang, C., Fu, Q., and Warren, S. G. (2016). Effect of Snow Grain Shape on Snow Albedo. J. Atmos. Sci. 73, 3573–3583. doi:10.1175/JAS-D-15-0276.1
De Haan, J., Bosma, P., and Hovenier, J. (1987). The Adding Method for Multiple Scattering Calculations of Polarized Light. Astron. Astrophys. 183, 371–391.
Doherty, S. J., Bitz, C. M., and Flanner, M. G. (2014). Biases in Modeled Surface Snow BC Mixing Ratios in Prescribed-Aerosol Climate Model Runs. Atmos. Chem. Phys. 14, 11697–11709. doi:10.5194/acp-14-11697-2014
Dubovik, O., Holben, B., Eck, T. F., Smirnov, A., Kaufman, Y. J., King, M. D., et al. (2002). Variability of Absorption and Optical Properties of Key Aerosol Types Observed in Worldwide Locations. J. Atmos. Sci. 59, 590–608. doi:10.1175/1520-0469(2002)059<0590:voaaop>2.0.co;2
Dumont, M., Brun, E., Picard, G., Michou, M., Libois, Q., Petit, J.-R., et al. (2014). Contribution of Light-Absorbing Impurities in Snow to Greenland’s Darkening Since 2009. Nat. Geosci. 7, 509–512. doi:10.1038/ngeo2180
Fettweis, X., Hanna, E., Gallée, H., Huybrechts, P., and Erpicum, M. (2008). Estimation of the Greenland Ice Sheet Surface Mass Balance for the 20th and 21st Centuries. Cryosphere 2, 117–129. doi:10.5194/tc-2-117-2008
Flanner, M. G., and Zender, C. S. (2006). Linking Snowpack Microphysics and Albedo Evolution. J. Geophys. Res. Atmosph. 111, 208. doi:10.1029/2005JD006834
Fougnie, B., Bracco, G., Lafrance, B., Ruffel, C., Hagolle, O., Tinel, C., et al. (2007). PARASOL In-Flight Calibration and Performance. Appl. Opt. 46, 5435–5451. doi:10.1364/ao.46.005435
Fu, Q. (2007). A New Parameterization of an Asymmetry Factor of Cirrus Clouds for Climate Models. J. Atmos. Sci. 64, 4140–4150. doi:10.1175/2007jas2289.1
Hansen, J., and Nazarenko, L. (2004). Soot Climate Forcing via Snow and Ice Albedos. Proc. Natl. Acad. Sci. U. S. A. 101, 423–428. doi:10.1073/pnas.2237157100
Hansen, J., and Travis, L. (1974). Light Scattering in Planetary Atmospheres. Space Sci. Rev. 16, 527–610. doi:10.1007/bf00168069
He, C., Liou, K.-N., Takano, Y., Yang, P., Qi, L., and Chen, F. (2018). Impact of Grain Shape and Multiple Black Carbon Internal Mixing on Snow Albedo: Parameterization and Radiative Effect Analysis. J. Geophys. Res. Atmosph. 123, 1253–1268. doi:10.1002/2017jd027752
Henyey, L. G., and Greenstein, J. L. (1941). Diffuse Radiation in the Galaxy. Astrophys. J. 93, 70–83. doi:10.1086/144246
Hori, M., Aoki, T., Stamnes, K., and Li, W. (2007). ADEOS-II/GLI Snow/Ice Products—Part III: Retrieved Results. Remote Sensing Environ. 111, 291–336. doi:10.1016/j.rse.2007.01.025
Jin, Z., Charlock, T., Yang, P., Xie, Y., and Miller, W. (2008). Snow Optical Properties for Different Particle Shapes with Application to Snow Grain Size Retrieval and MODIS/CERES Radiance Comparison Over Antarctica. Remote Sens. Environ. 112, 3563–3581. doi:10.1016/j.rse.2008.04.011
Key, J., and Haefliger, M. (1992). Arctic Ice Surface Temperature Retrieval from AVHRR Thermal Channels. J. Geophys. Res. 97, 5885–5893. doi:10.1029/92jd00348
Knobelspiesse, K., Cairns, B., Redemann, J., Bergstrom, R., and Stohl, A. (2011). Simultaneous Retrieval of Aerosol and Cloud Properties During the MILAGRO Field Campaign. Atmos. Chem. Phys. 11, 6245–6263. doi:10.5194/acp-11-6245-2011
Knobelspiesse, K., Cairns, B., Mishchenko, M., Chowdhary, J., Tsigaridis, K., van Diedenhoven, B., et al. (2012). Analysis of Fine-Mode Aerosol Retrieval Capabilities by Different Passive Remote Sensing Instrument Designs. Opt. Express 20, 21457–21484. doi:10.1364/oe.20.021457
Kokhanovsky, A., Rozanov, V., Aoki, T., Odermatt, D., Brockmann, C., Krüger, O., et al. (2011). Sizing Snow Grains Using Backscattered Solar Light. Int. J. Remote Sens. 32, 6975–7008. doi:10.1080/01431161.2011.560621
Krabill, W., Hanna, E., Huybrechts, P., Abdalati, W., Cappelen, J., Csatho, B., et al. (2004). Greenland Ice Sheet: Increased Coastal Thinning. Geophys. Res. Lett. 31, L24402. doi:10.1029/2004gl021533
Lang, R., Poli, G., Fougnie, B., Lacan, A., Marbach, T., Riedi, J., et al. (2019). The 3MI Level-1C Geoprojected Product – Definition and Processing Description. J. Quant. Spectrosc. Radiat. Transfer 225, 91–109. doi:10.1016/j.jqsrt.2018.12.022
Libois, Q., Picard, G., France, J., Arnaud, L., Dumont, M., Carmagnola, C., et al. (2013). Influence of Grain Shape on Light Penetration in Snow. Cryosphere 7, 1803–1818. doi:10.5194/tc-7-1803-2013
Lyapustin, A., Tedesco, M., Wang, Y., Aoki, T., Hori, M., and Kokhanovsky, A. (2009). Retrieval of Snow Grain Size Over Greenland from MODIS. Remote Sens. Environ. 113, 1976–1987. doi:10.1016/j.rse.2009.05.008
Macke, A., Mueller, J., and Raschke, E. (1996). Single Scattering Properties of Atmospheric Ice Crystals. J. Atmos. Sci. 53, 2813–2825. doi:10.1175/1520-0469(1996)053<2813:sspoai>2.0.co;2
Moosmüller, H., Chakrabarty, R., and Arnott, W. (2009). Aerosol Light Absorption and its Measurement: A Review. J. Quant. Spectrosc. Radiat. Transfer 110, 844–878. doi:10.1016/j.jqsrt.2009.02.035
Müller, T., Schladitz, A., Maßling, A., Kaaden, N., Kandler, K., and Wiedensohler, A. (2008). Spectral Absorption Coefficients and Imaginary Parts of Refractive Indices of Saharan Dust During SAMUM-1. Tellus B 61, 79–95.
Nolin, A., and Dozier, J. (2000). A Hyperspectral Method for Remotely Sensing the Grain Size of Snow. Remote Sensing Environ. 74, 207–216. doi:10.1016/s0034-4257(00)00111-5
Nolin, A. W., and Frei, A. (2003). “Remote Sensing of Snow and Characterization of Snow Albedo for Climate Simulations,” in Remote Sensing and Climate Modeling: Synergies and Limitations. Advances in Global Change Research. Editors M. Beniston, and M. M. Verstraete (Springer Netherlands), 7, 159–180.
Ottaviani, M., Cairns, B., Ferrare, R., and Rogers, R. (2012). Iterative Atmospheric Correction Scheme and the Polarization Color of Alpine Snow. J. Quant. Spectrosc. Radiat. Transfer 113, 789–804. doi:10.1016/j.jqsrt.2012.03.014
Ottaviani, M., van Diedenhoven, B., and Cairns, B. (2015). Photopolarimetric Retrievals of Snow Properties. Cryosphere 9, 1933–1942. doi:10.5194/tc-9-1933-2015
Ottaviani, M., Chowdhary, J., and Cairns, B. (2019). Remote Sensing of the Ocean Surface Refractive Index via Short-Wave Infrared Polarimetry. Remote Sens. Environ. 221, 14–23. doi:10.1016/j.rse.2018.10.016
Painter, T., Rittger, K., McKenzie, C., Slaughter, P., Davis, R., and Dozier, J. (2009). Retrieval of Subpixel Snow Covered Area, Grain Size, and Albedo from MODIS. Remote Sens. Environ. 113, 868–879. doi:10.1016/j.rse.2009.01.001
Räisänen, P., Makkonen, R., Kirkevåg, A., and Debernard, J. B. (2017). Effects of Snow Grain Shape on Climate Simulations: Sensitivity Tests with the Norwegian Earth System Model. Cryosphere 11, 2919–2942. doi:10.5194/tc-11-2919-2017
Rae, J., Aðalgeirsdóttir, G., Edwards, T., Fettweis, X., Gregory, J., Hewitt, H., et al. (2012). Greenland Ice Sheet Surface Mass Balance: Evaluating Simulations and Making Projections with Regional Climate Models. Cryosphere 6, 1275–1294. doi:10.5194/tc-6-1275-2012
Rodgers, C. (2000). “Inverse Methods for Atmospheric Sounding Theory and Practice,” in Series on Atmospheric, Oceanic and Planetary Physics (World Scientific Publishing Company), 2.
Schmale, J., Zieger, P., and Ekman, A. M. (2021). Aerosols in Current and Future Arctic Climate. Nat. Clim. Change 11, 95–105. doi:10.1038/s41558-020-00969-5
Stamnes, K., Li, W., Eide, H., Aoki, T., Hori, M., and Storvold, R. (2007). ADEOS-II/GLI Snow/Ice Products–Part I: Scientific Basis. Remote Sens. Environ. 111, 258–273. doi:10.1016/j.rse.2007.03.023
Stamnes, K., Hamre, B., Stamnes, J., Ryzhikov, G., Biryulina, M., Mahoney, R., et al. (2011). Modeling of Radiation Transport in Coupled Atmosphere-Snow-Ice-Ocean Systems. J. Quant. Spectrosc. Radiat. Transfer 112, 714–726. doi:10.1016/j.jqsrt.2010.06.006
Stroeve, J., Box, J. E., Wang, Z., Schaaf, C., and Barrett, A. (2013). Re-Evaluation of MODIS MCD43 Greenland Albedo Accuracy and Trends. Remote Sens. Environ. 138, 199–214. doi:10.1016/j.rse.2013.07.023
Tedesco, M., and Kokhanovsky, A. (2007). The Semi-Analytical Snow Retrieval Algorithm and its Application to Modis Data. Remote Sens. Environ. 111, 228–241. doi:10.1016/j.rse.2007.02.036
Tedesco, M., Fettweis, X., Van den Broeke, M., Van de Wal, R., Smeets, C., van de Berg, W. J., et al. (2011). The Role of Albedo and Accumulation in the 2010 Melting Record in Greenland. Environ. Res. Lett. 6, 014005. doi:10.1088/1748-9326/6/1/014005
Tedesco, M., Fettweis, X., Mote, T., Wahr, J., Alexander, P., Box, J. E., et al. (2013). Evidence and Analysis of 2012 Greenland Records from Spaceborne Observations, a Regional Climate Model and Reanalysis Data. Cryosphere 7, 615–630. doi:10.5194/tc-7-615-2013
Tedesco, M., Doherty, S., Fettweis, X., Alexander, P., Jeyaratnam, J., and Stroeve, J. (2016). The Darkening of the Greenland Ice Sheet: Trends, Drivers, and Projections (1981-2100). Cryosphere 10, 477–496. doi:10.5194/tc-10-477-2016
Van Angelen, J., Lenaerts, J., Lhermitte, S., Fettweis, X., Kuipers Munneke, P., van den Broeke, M., et al. (2012). Sensitivity of Greenland Ice Sheet Surface Mass Balance to Surface Albedo Parameterization: A Study with a Regional Climate Model. Cryosphere 6, 1175–1186. doi:10.5194/tc-6-1175-2012
van den Broeke, M., Smeets, C., and Van de Wal, R. (2011). The Seasonal Cycle and Interannual Variability of Surface Energy Balance and Melt in the Ablation Zone of the West Greenland Ice Sheet. Cryosphere 5, 377–390. doi:10.5194/tc-5-377-2011
van Diedenhoven, B., Cairns, B., Geogdzhayev, I., Fridlind, A., Ackerman, A., Yang, P., et al. (2012). Remote Sensing of Ice Crystal Asymmetry Parameter Using Multi-Directional Polarization Measurements - Part 1: Methodology and Evaluation with Simulated Measurements. Atmos. Meas. Tech. 5, 2361–2374. doi:10.5194/amt-5-2361-2012
van Diedenhoven, B., Ackerman, A. S., Cairns, B., and Fridlind, A. M. (2014a). A Flexible Parameterization for Shortwave Optical Properties of Ice Crystals. J. Atmos. Sci. 71, 1763–1782. doi:10.1175/jas-d-13-0205.1
van Diedenhoven, B., Fridlind, A. M., Cairns, B., and Ackerman, A. S. (2014b). Variation of Ice Crystal Size, Shape, and Asymmetry Parameter in Tops of Tropical Deep Convective Clouds. J. Geophys. Res. Atmosph. 119, 11809–11825. doi:10.1002/2014jd022385
Velicogna, I. (2009). Increasing Rates of Ice Mass Loss from the Greenland and Antarctic Ice Sheets Revealed by GRACE. Geophys. Res. Lett. 36, L19503. doi:10.1029/2009gl040222
Wagner, R., Ajtai, T., Kandler, K., Lieke, K., Linke, C., Müller, T., et al. (2012). Complex Refractive Indices of Saharan Dust Samples at Visible and Near UV Wavelengths: A Laboratory Study. Atmos. Chem. Phys. 12, 2491–2512. doi:10.5194/acp-12-2491-2012
Waquet, F., Cairns, B., Knobelspiesse, K., Chowdhary, J., Travis, L., Schmid, B., et al. (2009). Polarimetric Remote Sensing of Aerosols Over Land. J. Geophys. Res.-Atmosph. 114, D01206. doi:10.1029/2008jd010619
Warren, S., and Wiscombe, W. (1980). A Model for the Spectral Albedo of Snow. II: Snow Containing Atmospheric Aerosols. J. Atmos. Sci. 37, 2734–2745. doi:10.1175/1520-0469(1980)037<2734:amftsa>2.0.co;2
Warren, S. G. (2013). Can Black Carbon in Snow Be Detected by Remote Sensing? J. Geophys. Res. (Atmospheres) 118, D018476. doi:10.1029/2012jd018476
Warren, S. G. (2019). Optical Properties of Ice and Snow. Philos. Trans. R. Soc. A 377, 20180161. doi:10.1098/rsta.2018.0161
Wiscombe, W., and Warren, S. (1980). A Model for the Spectral Albedo of Snow. I: Pure Snow. J. Atmos. Sci. 37, 2712–2733. doi:10.1175/1520-0469(1980)037<2712:amftsa>2.0.co;2
Xie, Y., Yang, P., Gao, B.-C., Kattawar, G. W., and Mishchenko, M. I. (2006). Effect of Ice Crystal Shape and Effective Size on Snow Bidirectional Reflectance. J. Quant. Spectrosc. Radiat. Transfer 100, 457–469. doi:10.1016/j.jqsrt.2005.11.056
Yang, Y., Marshak, A., Chiu, J. C., Wiscombe, W. J., Palm, S. P., Davis, A. B., et al. (2008). Retrievals of Thick Cloud Optical Depth from the Geoscience Laser Altimeter System (GLAS) by Calibration of Solar Background Signal. J. Atmos. Sci. 65, 3513–3526. doi:10.1175/2008jas2744.1
Zatko, M. C., Grenfell, T. C., Alexander, B., Doherty, S. J., Thomas, J. L., and Yang, X. (2013). The Influence of Snow Grain Size and Impurities on the Vertical Profiles of Actinic Flux and Associated Nox Emissions on the Antarctic and Greenland Ice Sheets. Atmos. Chem. Phys. 13, 3547–3567. doi:10.5194/acp-13-3547-2013
Zege, E., Katsev, I., Malinka, A., Prikhach, A., and Polonsky, I. (2008). New Algorithm to Retrieve the Effective Snow Grain Size and Pollution Amount from Satellite Data. Ann. Glaciol. 49, 139–144. doi:10.3189/172756408787815004
Keywords: light polarization, snow, remote sensing, cryosphere, light-absorbing impurities
Citation: Ottaviani M (2022) Polarization as a Discriminator of Light-Absorbing Impurities in or Above Snow. Front. Remote Sens. 3:863239. doi: 10.3389/frsen.2022.863239
Received: 27 January 2022; Accepted: 07 April 2022;
Published: 06 June 2022.
Edited by:
Jeremy Werdell, Goddard Space Flight Center, United StatesReviewed by:
Peng-Wang Zhai, University of Maryland, Baltimore County, United StatesLei Li, Chinese Academy of Meteorological Sciences, China
Copyright © 2022 Ottaviani. This is an open-access article distributed under the terms of the Creative Commons Attribution License (CC BY). The use, distribution or reproduction in other forums is permitted, provided the original author(s) and the copyright owner(s) are credited and that the original publication in this journal is cited, in accordance with accepted academic practice. No use, distribution or reproduction is permitted which does not comply with these terms.
*Correspondence: Matteo Ottaviani, bWF0dGVvLm90dGF2aWFuaUBuYXNhLmdvdg==