- 1Science Directorate, NASA Langley Research Center, Hampton, VA, United States
- 2Physics Department, University of Maryland, Baltimore County, Baltimore, MD, United States
- 3NASA Goddard Institute for Space Studies, New York, NY, United States
- 4Space Science Institute, Boulder Suite, CO, United States
- 5US Army Research Laboratory, Adelphi, MD, United States
- 6Department of Atmospheric Sciences, University of Utah, Salt Lake City, UT, United States
- 7Department of Atmospheric Science, University of Wyoming, Laramie, WY, United States
- 8Department of Atmospheric Sciences, University of Washington, Seattle, WA, United States
- 9Rosenstiel School of Marine and Atmospheric Sciences, University of Miami, Miami, FL, United States
- 10University Corporation for Atmospheric Research, Boulder, CO, United States
A neural-network algorithm that uses CALIPSO lidar measurements to infer droplet effective radius, extinction coefficient, liquid-water content, and droplet number concentration for water clouds is described and assessed. These results are verified against values inferred from High-Spectral-Resolution Lidar (HSRL) and Research Scanning Polarimeter (RSP) measurements made on an aircraft that flew under CALIPSO. The global cloud microphysical properties are derived from 14+ years of CALIPSO lidar measurements, and the droplet sizes are compared to corresponding values inferred from MODIS passive imagery. This new product will provide constraints to improve modeling of Earth’s water cycle and cloud-climate interactions.
Introduction
CALIPSO Lidar measurements provide the first ever direct measurements of the global distribution of water-cloud extinction coefficients from space. Water-cloud backscattering β decays exponentially with propagation depth
It is also possible to estimate the water-cloud lidar ratio Sc, i.e. the ratio of the extinction to the backscattering cross section measured at 180° scattering angle obtained from CALIPSO measurements (Hu, 2007). Water-cloud lidar ratios are inversely proportional to cloud droplet sizes (Hu et al., 2006; Mace et al., 2020). Thus, it is theoretically possible to estimate the effective water-cloud droplet size Re from CALIPSO measurements. There are measurement issues to be resolved in order to derive Re accurately using CALIPSO observations, e.g., shot noise from sunlight creates difficulty in detecting aerosols and sub-visual clouds above water clouds, and thus causes biases in lidar-ratio estimates. Here we develop a Re retrieval technique that allows us to avoid such biases.
In this paper we describe the retrieval algorithm for estimating microphysical properties of water clouds (e.g., extinction coefficient, effective drop size, liquid water content, and droplet number concentration) from 14 + years of global lidar measurements acquired by NASA’s CALIPSO satellite.
Airborne measurements made by the co-manifested NASA Langley Research Center (LaRC) high spectral resolution lidar (HSRL) and the Goddard Institute of Space Studies (GISS) research scanning polarimeter (RSP) have been used to derive an identical set of water-cloud microphysical properties. The results obtained from these joint HSRL + RSP retrievals agree closely with the coincident in situ measurements acquired during multiple field campaigns conducted in various locations around the planet. These retrievals from airborne remote-sensing platforms are used in validating our experimental CALIPSO water-cloud microphysics products.
While space-based passive remote sensors only derive water-cloud microphysical parameters from daytime measurements, our experimental CALIPSO data product provides a full set of water-cloud microphysical information for both daytime and nighttime. In our initial studies of diurnal differences, we have found large day/night contrasts in water-cloud microphysical properties. It turns out that the difference is due to depolarization calibration inconsistency. After the inconsistency is removed, the day-night differences in cloud microphysics are reduced significantly.
The cloud microphysical properties derived from the CALIPSO lidar measurements will enable new and more accurate constraints to be developed and applied to weather and climate models, such as cloud parameterization schemes with their associated simulations of radiation and condensation (e.g., Wilson et al., 2008). This study provides the community with the first long-term, global, nighttime cloud microphysics data products. It also provides an independent, validated daytime water-cloud droplet number concentration data product complementary to those from passive remote sensing (Han et al., 1998; Wood, 2006; Grosvenor et al., 2018).
Technical Approach
In addition to cloud-top height (Mace et al., 2020) and cloud thermodynamic-phase identification (Hu et al., 2007), CALIPSO’s lidar measurements can be mined to retrieve many other important water-cloud microphysical properties. For example, lidar ratios derived from the CALIPSO water-cloud measurements have been shown to be well-correlated with effective cloud droplet size (Mace et al., 2020), and CALIPSO’s dual polarization backscattering profiles are sensitive to changes in extinction coefficients (Li et al., 2018). Estimation of cloud liquid-water content and droplet number density from CALIPSO observations hinges upon retrievals of cloud droplet effective radius and cloud extinction (Hu et al., 2007).
The retrieval of cloud-droplet effective radius from CALIPSO data is illustrated in Figure 1. Lidar ratios Sc of moderately thick water clouds (in this work defined as the effective optical depths at 532 nm larger than 2.5 and two-way transmittances less than 0.0067) can be derived from layer-integrated attenuated-backscattering and depolarization-ratio measurements of water clouds. The layer-integrated attenuated backscattering β of moderately thick water clouds is inversely proportional to Sc and the layer-integrated multiple scattering factor η; i.e., β = 1/[2η* Sc] (Platt, 1973). The multiple-scattering factor can be accurately computed from the layer-integrated volume depolarization ratio δ using η=(1−δ)2/(1+δ)2 (Hu et al., 2006; Hu, 2007; Hu et al., 2007). Lidar ratios of water clouds computed from the Lorenz-Mie theory are inversely correlated with the effective droplet radius (Figure 1, left panel) if the effective variance of the size distribution is greater than 0.1 (e.g., green and blue dotted lines). As the relationship between droplet size and lidar ratio varies with effective variance of size distribution, water-cloud droplet size cannot be estimated accurately from lidar ratio alone. To estimate droplet size accurately, we also need another independent measurement that is sensitive to the size distribution, e.g., color-ratio measurements. The right panel of Figure 1 shows that the color ratio also varies with particle size and effective variance.
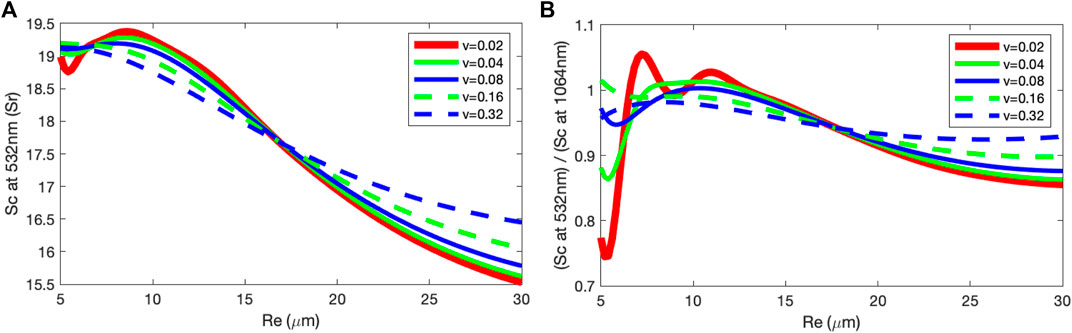
FIGURE 1. (A): relationship between water-cloud droplet effective radius Re and water-cloud lidar ratio Sc calculated using the Lorenz-Mie theory. (B): the single-scattering color ratio (1064 nm backscatter cross section/532 nm backscatter cross section) as a function of Re.
It is possible to estimate the effective radius of water clouds relatively accurately from lidar-ratio and color-ratio information derived from lidar measurements. Comparing with 532 nm, theoretical calculations (Hu et al., 2007) suggest that multiple scattering at 1064 nm is roughly 25% higher for CALIPSO, due to the fact that the size parameter at 1064 nm is half of 532 nm and multiple scattering is inversely proportional to the third power of the size parameter (Hu et al., 2007). Thus the effective color ratio from the CALIPSO measurements (right panels of Figure 2) is 25% higher compared with the single-scattering color ratio and is consistent with theoretical calculations. Other information, such as cloud temperature, estimated from CALIPSO cloud-height measurements, can also provide extra information about the effective variance of the droplet size distribution.
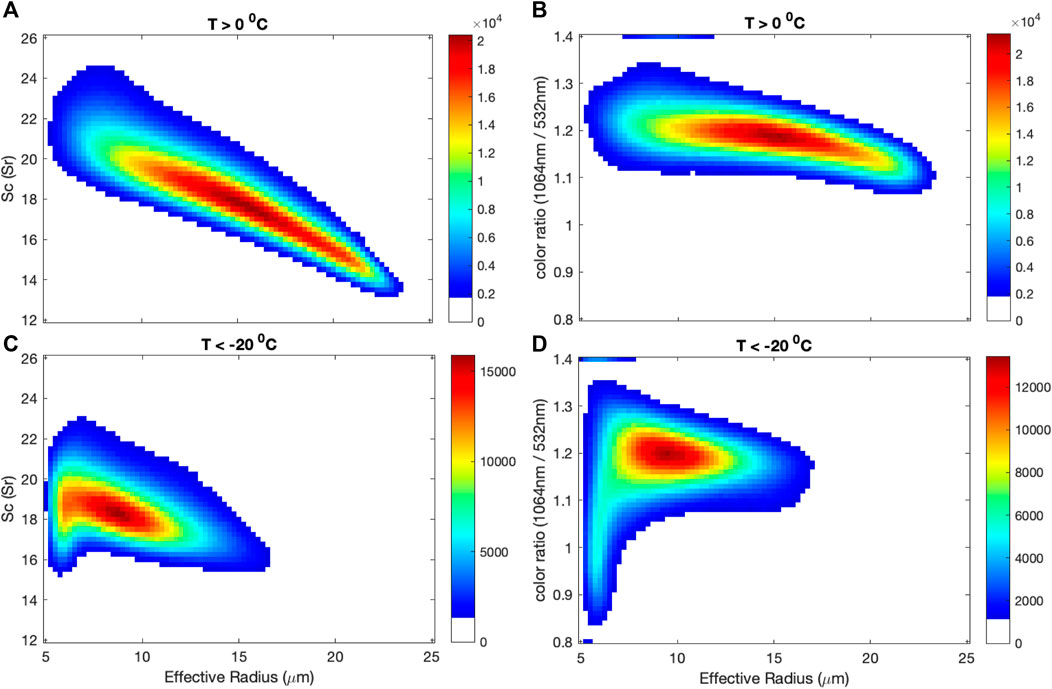
FIGURE 2. Left panels: relationship between the collocated MODIS water-cloud droplet effective radius Re and CALIPSO water-cloud lidar ratio Sc for warm water clouds (A) and supercooled liquid water clouds (B). Right panel: relationship between collocated MODIS Collection 6 water cloud droplet effective radius Re (Platnick et al., 2017) and CALIPSO water-cloud color ratio for warm water clouds (C) and supercooled liquid water clouds (D).
Collocated MODIS and CALIPSO measurements show that for warm water clouds with cloud-top temperatures higher than 0°C (upper-left panel of Figure 2), the lidar ratio increases as the effective radius decreases. The effective variance of the droplet size distribution from both the lidar ratios and color ratios is likely greater than 0.16 when effective radius is less than 10 μm. For warm clouds with effective radius larger than 15 μm, lidar ratios are considerably lower than theoretical Lorenz-Mie calculations. For cold water clouds with cloud-top temperatures colder than −20°C (lower panels of Figure 2), both the lidar-ratio and color-ratio measurements suggest that the effective variance of the size distribution is likely smaller than 0.04.
Although there is sufficient information about cloud droplet effective radius in lidar measurements, there are measurement issues that may affect the accuracy of effective-radius estimates from the lidar-ratio and color-ratio measurements. Subvisual cirrus and background aerosols above the clouds may cause over-estimations of the lidar ratio. Fine-mode aerosols above water clouds cause over-estimation of the color ratio. For water clouds having droplets with an effective radius larger than 20 μm, lidar ratios estimated from the CALIPSO measurements are significantly smaller than the ones from Lorenz-Mie calculations.
A neural-network-based nonlinear functional approximation (Beal et al., 1992; Beal et al., 2021) that links CALIPSO water-cloud measurements to effective radius derived from collocated MODIS observations is developed in order to overcome these issues effectively. The neural-network algorithm takes in the CALIPSO measurements, such as the layer-integrated attenuated water-cloud backscattering and the vertically integrated, attenuated backscattering of the air above water clouds, as input and the effective radius of collocated MODIS as output for training the neural network. The collocated MODIS effective radius and CALIPSO lidar measurements during January 2008 are used for training the neural-network algorithm and applied to all CALIPSO daytime measurements between 2008 and 2020. The effective radius from CALIPSO (right panel of Figure 3) agrees with MODIS (left panel of Figure 3) within ± 2 microns.
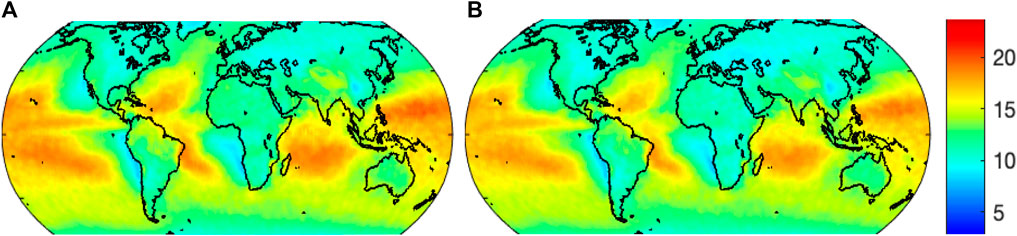
FIGURE 3. Comparisons of water-cloud-droplet effective radius Re from 2008 MODIS (A) and CALIPSO (B) measurements.
We also applied the algorithm to the CALIPSO nighttime measurements and found that the droplet sizes are unrealistically large compared with daytime measurements. This is most likely due to differences in the calibration. While daytime and nighttime statistics of all three channels of the lidar measurements suggest good consistency of daytime and nighttime 532 nm parallel and 1064 nm total backscattering measurements, there are significant differences between daytime and nighttime 532 nm perpendicular lidar backscattering statistics. For example, while nighttime 532 nm parallel-polarized backscattering from land surfaces agrees with daytime measurements, nighttime backscattering depolarization ratios of land surfaces (upper right panel of Figure 4) are very different from daytime measurements (upper left panel of Figure 4), and the nighttime depolarization ratios are roughly 7% lower compared with daytime measurements (lower panels of Figure 4). We raised nighttime depolarization ratios by 7% to make it consistent with daytime measurements and thus possible to apply the neural-network algorithm to nighttime CALIPSO measurements for effective radius estimation. With the adjustment of nighttime depolarization ratios, the daytime and nighttime difference in cloud effective radius estimated from the neural-network algorithm is significantly reduced (Figure 5).
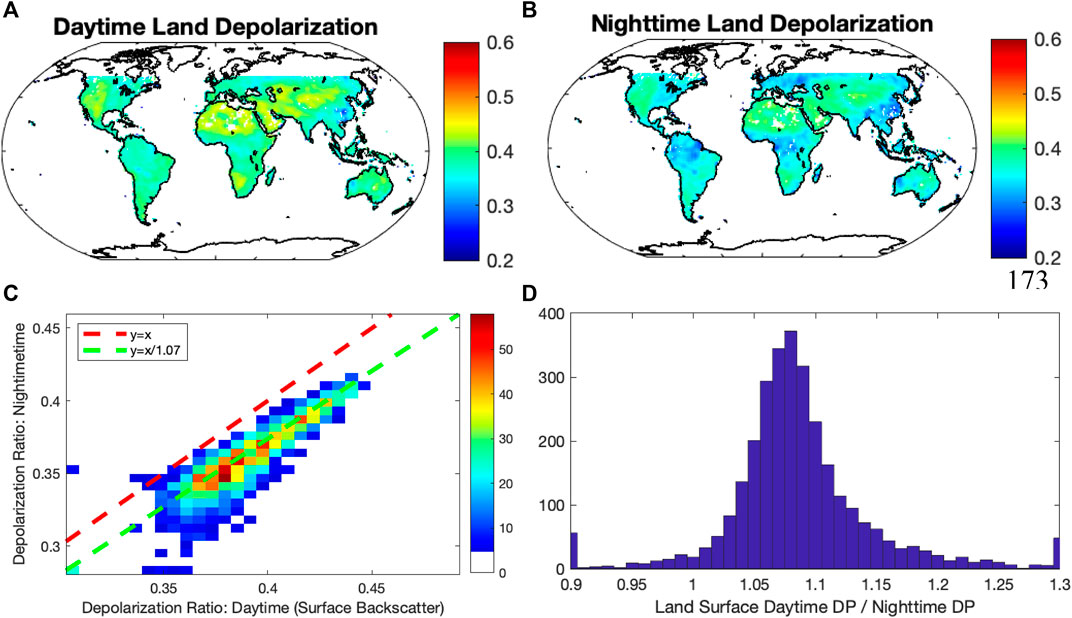
FIGURE 4. Comparisons of daytime (A) and nighttime (B) land surface depolarization ratio from CALIPSO measurements, as well as the 3D histogram (C) and 2D histogram (D) of the day-night land surface difference.
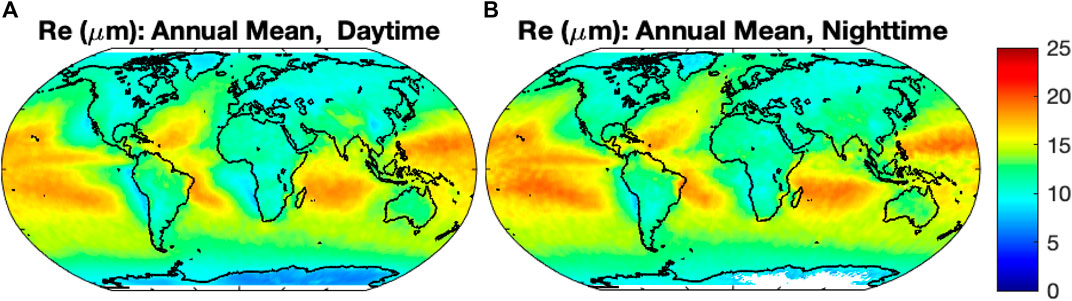
FIGURE 5. Comparisons of daytime (A) and nighttime (B) water-cloud droplet effective radius Re estimated from CALIPSO measurements (2008).
We also developed a second neural-network algorithm (CALIPSO 2) to reduce potential uncertainties in cloud-droplet radius estimates due to undetected aerosols above water clouds and calibration errors. This method uses depolarization ratios and color ratios as input parameters of the neural-network algorithm to replace the three CALIPSO 1 attenuated-backscattering channels. The effective radii derived from these two algorithms agree with each other in general (lower right panel of Figure 8 and middle panel of Figure 11).
We also developed two different algorithms to determine the water-cloud extinction coefficient from CALIPSO measurements. Method #1 is a profile-shape algorithm in which the water-cloud extinction coefficient σ is determined from the five range bins that measure the largest attenuated-backscattering coefficients (i.e., including one prior and three subsequent to the peak backscattering, β-1, β0, … , β3). This technique is based on simulations of the CALIPSO water-cloud measurements for various extinction coefficients that fully account for measurement complexity, such as the non-ideal CALIPSO receiver transient responses (Hu et al., 2007), cloud top bumpiness, and issues associated with averaging over 30 m range bins (Figures 6 and 7). Using these simulated lidar measurements, a neural network nonlinear functional approximation, f(βI) = σ, is trained with attenuated-backscattering coefficients computed for various extinction coefficients as the input and the extinction coefficients as the output (Figure 7).
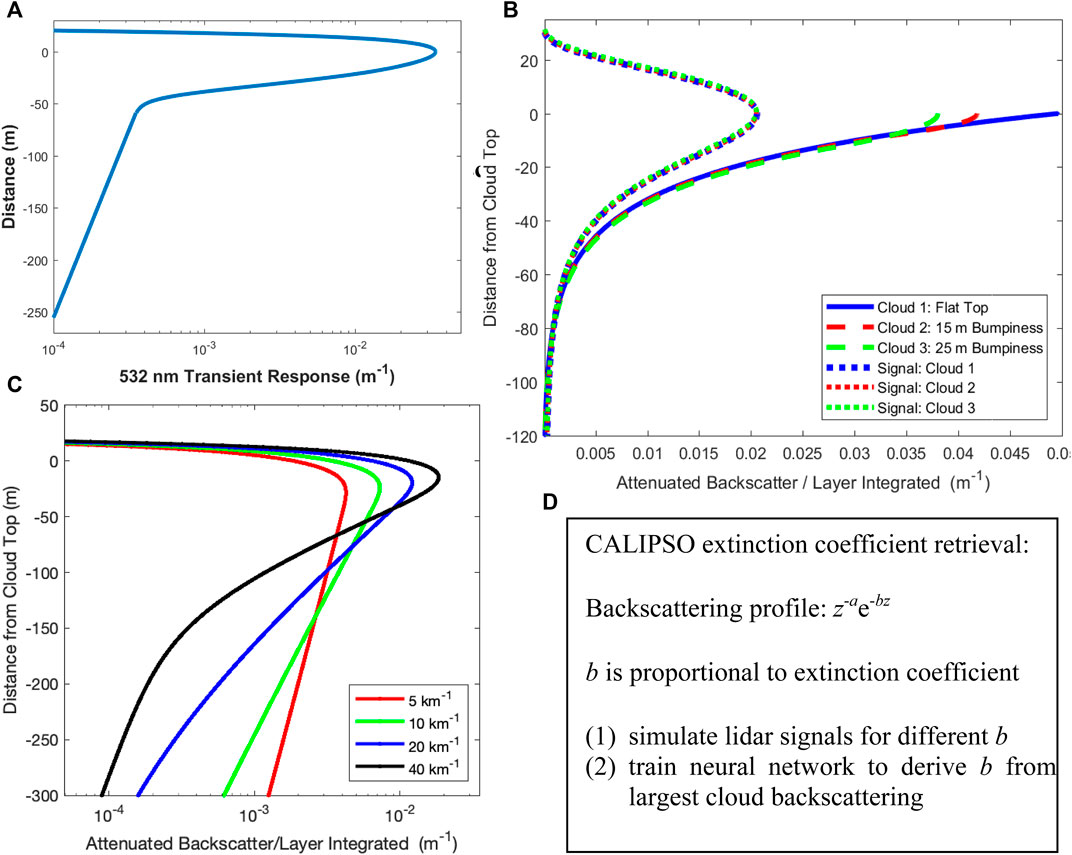
FIGURE 7. Physics behind water-cloud extinction-coefficient retrievals using five largest cloud backscattering range bins. (A): transient response function CALIPSO’s 532 nm channel. (B): one silver lining of CALIPSO’s transient response and coarse-resolution sampling is that the backscattering profiles are insensitive to cloud-top bumpiness. (C): backscattering profiles before discretized sampling for different effective extinction coefficients. (D): a neural-network algorithm for determining extinction coefficients.
Extinction-coefficient retrieval method #2 derives extinction coefficients σ from depolarization ratios δ and effective radii Re by applying the theoretical relationship f (σ, Re, δ) = 0 established by extensive Monte Carlo simulations of laser-light propagation in water clouds (Hu et al., 2007; Li et al., 2011; Zeng et al., 2014) while the formula is modified based on the measurements:
Where λ is the lidar wavelength (0.532 μm) and the unit of Re is μm.
As seen in the lower left panel of Figure 8, the extinction coefficients derived using the two different methods agree reasonably well. Similarly, estimates of effective radius also can be derived from depolarization ratios and extinction coefficients. The water-cloud effective radii derived from this method also agree with the effective radii obtained from lidar-ratio measurements (lower right panel of Figure 8).
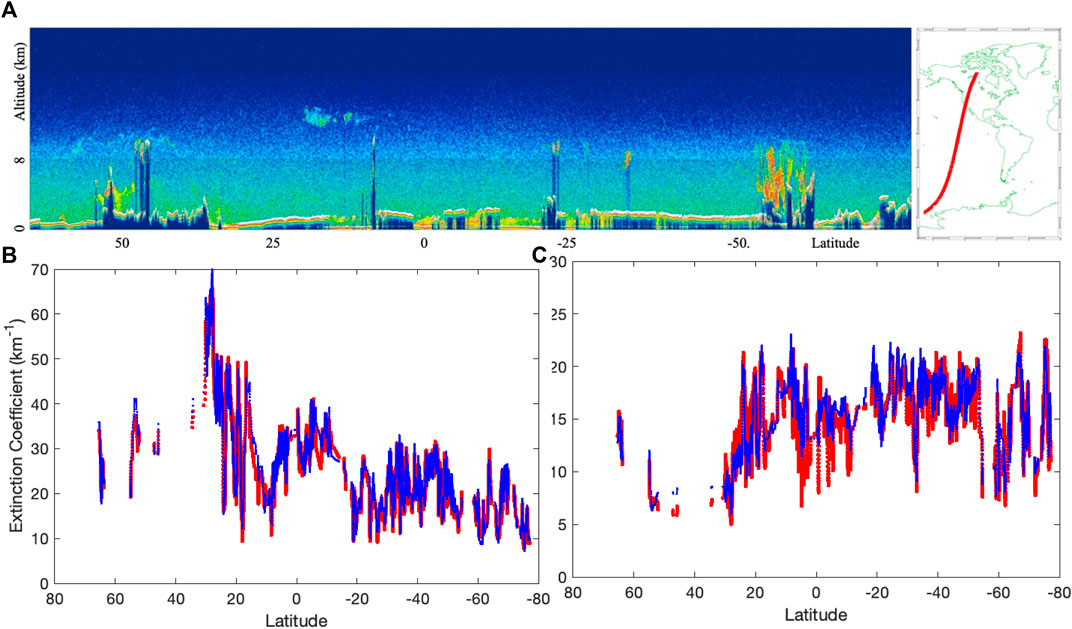
FIGURE 8. Examples of the extinction coefficient (A) and effective radius (B) estimates for water-cloud measurements of a nighttime CALIPSO orbit (C). Method 1 (blue lines) and Method 2 (red lines) are two different retrieval algorithms described in the text. The unit of latitudes in all the figures are in degrees.
Other physical properties of water clouds, such as liquid-water content w and droplet number concentration Nd, can be derived from the extinction coefficient σ and effective radius Re (Hu et al., 2007):
Here ρ is the density of water (1 g/cm3), Qc is the extinction efficiency of water-cloud droplets, which for droplets large compared with the wavelength
Figure 9 shows the liquid-water content (left panel) and droplet number concentration (right panel) derived from Re and extinction coefficient for the same section of the orbit as in Figure 8. The blue and red lines represent w and Nd derived from Re and extinction coefficient using Method 1 and Method 2 respectively, which agree with each other reasonably well.
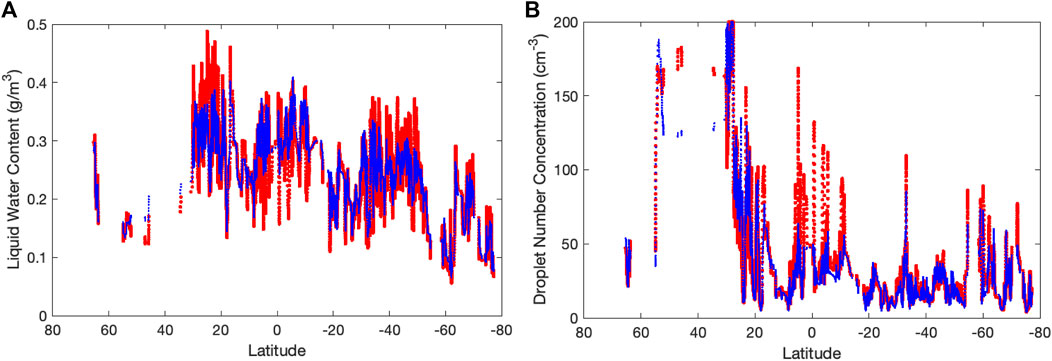
FIGURE 9. CALIPSO measurements of cloud liquid-water content (A) and droplet number concentration (B) from the same section of orbit as in as Figure 8.
Figure 10 shows the annual mean microphysical properties of water clouds, including Re (upper left panel), extinction coefficient (upper right panel), liquid-water content (lower left panel) and droplet number concentration (lower right panel).
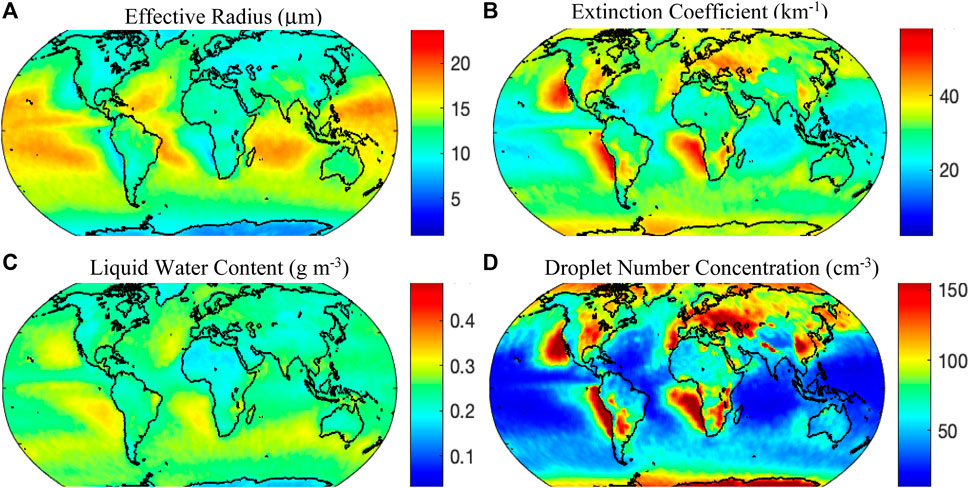
FIGURE 10. Annual mean effective radius (A), extinction coefficient (B), cloud liquid-water content (C) and droplet number concentration (D) of CALIPSO water-cloud measurements.
Comparisons of CALIPSO Water-Cloud Properties With Cloud Microphysical Properties From HSRL and RSP Measurements
Over the last several years, the NASA HSRL and RSP teams have invested heavily in both instrument and algorithm development in order to improve the accuracy of their measurements and optimize their retrievals of cloud microphysical properties. The HSRL/RSP water-cloud-microphysical-property product is validated against in situ measurements acquired during the North Atlantic Aerosols and Marine Ecosystems Study (NAAMES). The extinction coefficients from the HSRL measurements agree reasonably well with in situ measurements (Alexandrov, et al., 2018; Hair et al., 2018). Similarly, the mean droplet extinction cross section areas (2π * Re2) from the RSP measurements (Cairns et al., 2020) also show reasonable agreement with the corresponding in situ measurements.
Water-cloud measurements have been made by the HSRL and RSP instruments in many field campaigns that have taken place in different parts of the world over the last few years. Thus, we can use the global data set of HSRL and RSP measurements to assess uncertainties in the CALIPSO water-cloud-microphysical-property data products as discussed below.
Figure 11 shows an example of the HSRL/RSP water-cloud measurements when the aircraft underflew the CALIPSO orbit track (blue line in the upper panel) on May 27, 2016 during the NAAMES mission. The water-cloud effective radii determined from the RSP measurements (blue line in the middle panel of Figure 11) agree better with CALIPSO’s Re estimates derived with the lidar ratio method (green and red line) within uniform clouds. For relatively broken clouds, Re derived from the CALIPSO extinction coefficient and depolarization-ratio method (red line) agrees better with the RSP data (blue). CALIPSO extinction coefficients (green and red lines of lower panel of Figure 11) also agree with the ones from HSRL measurements (blue line). Cloud microphysical properties derived from the under-flying aircraft measurements agree similarly well with collocated water-cloud microphysical properties derived from CALIPSO.
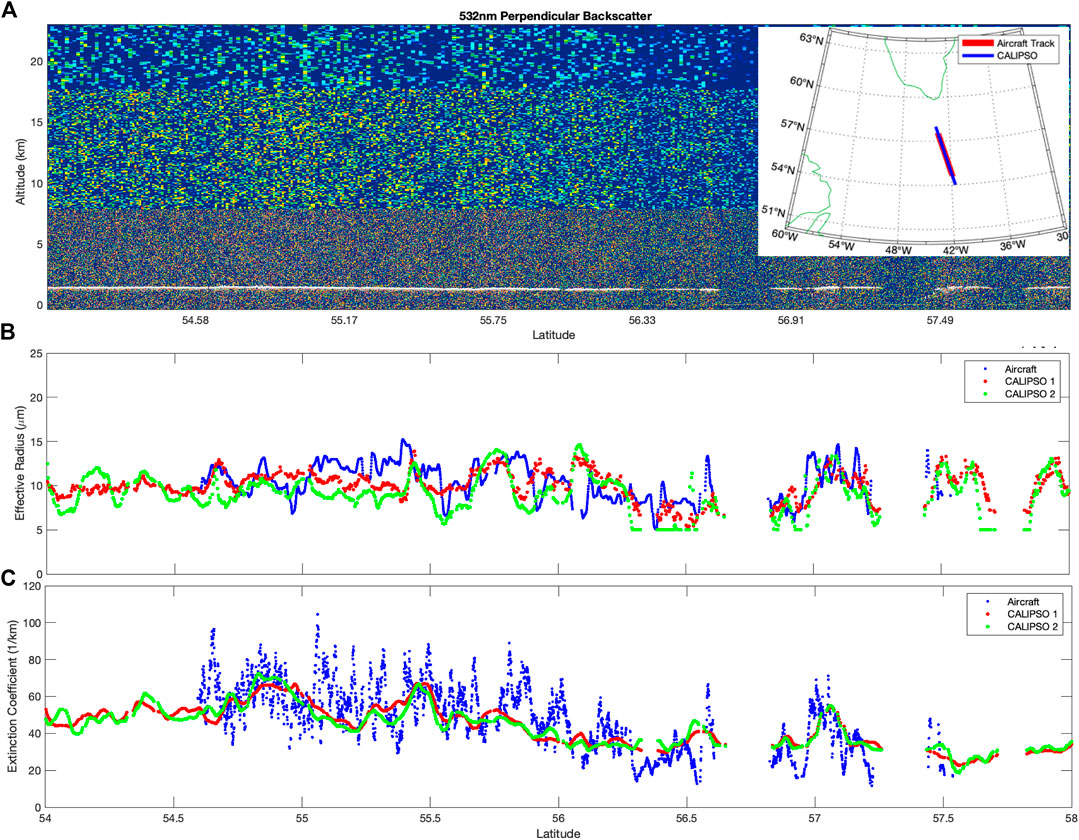
FIGURE 11. (A): NAAMES May 27, 2016 flight track (red) and CALIPSO overflight (blue). (B): comparisons of CALIPSO measurements of water-cloud effective radius (green and red) with effective-radius measurements from RSP (blue). (C): comparisons of CALIPSO measurements of water-cloud extinction coefficient (green and red) with extinction-coefficient measurements from HSRL (blue).
Summary
Water-cloud lidar ratios can be derived from water-cloud layer-integrated attenuated-backscattering and depolarization ratios of CALIPSO lidar measurements. Lidar ratios and color ratios of water clouds are both sensitive to changes in effective radius and variance of the water-cloud size distribution. Using the CALIPSO lidar measurements and collocated MODIS effective-size measurements, a neural-network algorithm is developed to retrieve water-cloud effective radius from CALIPSO’s water-cloud backscattering measurements.
Vertical profiles of lidar backscattering from water clouds are sensitive to changes in cloud extinction coefficients. It is challenging to deriving extinction coefficients from the CALIPSO lidar backscattering profile because of the cloud top heterogeneity and CALIPSO’s detector transient response. Based on simulations of the cloud measurements, a neural-network algorithm is developed to determine extinction coefficients accurately from the water-cloud backscattering profile.
Based on daytime and nighttime land surface depolarization statistics, adjustments are made to the nighttime water-cloud depolarization ratios in order to apply an algorithm to determine effective radii from nighttime CALIPSO measurements. This algorithm is trained from daytime MODIS and CALIPSO measurements.
Based on the theoretical analysis, we developed an experimental data product that retrieves microphysical properties of water clouds (e.g., extinction coefficient, effective drop size, liquid-water content, and droplet number concentration) from 14 + years of global lidar measurements acquired by NASA’s CALIPSO satellite. This new product will provide constraints to improve modeling of the water cycle and cloud-climate interactions. To realize this potential, the product must be properly validated.
Airborne measurements made by the co-manifested LaRC HSRL and the GISS RSP can be used to derive an identical set of water-cloud microphysical properties. These retrievals from airborne remote-sensing platforms agrees with our experimental CALIPSO water-cloud microphysical product.
For future studies, we plan to improve the algorithm with more sophisticated treatments of droplet variances, vertical changes of cloud droplet sizes, and aerosols/subvisual clouds located above the water cloud. Additional information from aircraft measurements and collocated MODIS, AMSR-E and other A-Train satellite measurements will be analyzed for future algorithm improvements.
Data Availability Statement
The original contributions presented in the study are included in the article/supplementary material, further inquiries can be directed to the corresponding author.
Author Contributions
YH developed the algorithm, with considerable guidance and corrections from RW, JM, DM, IM, WS, SS, AO, RB, GV, XL, and PZ provided algorithm support and data analysis. CH, JH, BC provided aircraft measurements and data analysis for validation.
Funding
This study is supported by NASA Radiation Science Program and Atmospheric Composition Campaign Data Analysis and Modeling Program, and by NASA CALIPSO project.
Conflict of Interest
The authors declare that the research was conducted in the absence of any commercial or financial relationships that could be construed as a potential conflict of interest.
Publisher’s Note
All claims expressed in this article are solely those of the authors and do not necessarily represent those of their affiliated organizations, or those of the publisher, the editors and the reviewers. Any product that may be evaluated in this article, or claim that may be made by its manufacturer, is not guaranteed or endorsed by the publisher.
Acknowledgments
We would like to thank Mark Vaughan and CALIPSO team for discussions and the CALIPSO data.
References
Alexandrov, M. D., Cairns, B., Sinclair, K., Wasilewski, A. P., Ziemba, L., Crosbie, E., et al. (2018). Retrievals of Cloud Droplet Size from the Research Scanning Polarimeter Data: Validation Using In Situ Measurements. Remote Sensing Environ. 210, 76–95. doi:10.1016/j.rse.2018.03.005
Beal, M. H., Hagan, M. T., and Demuth, H. B. (2021). Deep Learning Toolbox Reference. Available at: https://www.mathworks.com/help/pdf_doc/deeplearning/nnet_ref.pdf.
Beal, M. H., Hagan, M. T., and Demuth, H. B. (1992). Neural Network Toolbox: User’s Guide. Available at: https://citeseerx.ist.psu.edu/viewdoc/download?doi=10.1.1.699.4831.
Grosvenor, D. P., Sourdeval, O., Zuidema, P., Ackerman, A., Alexandrov, M. D., Bennartz, R., et al. (2018). Remote Sensing of Droplet Number Concentration in Warm Clouds: A Review of the Current State of Knowledge and Perspectives. Rev. Geophys. 56, 409–453. doi:10.1029/2017rg000593
Hair, J. W., Cairns, B., Alexandrov, M. D., Hostetler, C. A., Hu, Y., Scarino, A. J., et al. (2018). Lidar and Polarimeter Measurements of Water Clouds during the North Atlantic Marine Aerosols and Ecosystems Study (NAAMES) Campaigns. American Geophysical Union Fall Meeting 2018, Washington DC.
Han, Q., Rossow, W. B., Chou, J., and Welch, R. M. (1998). Global Variation of Column Droplet Concentration in Low-Level Clouds. Geophys. Res. Lett. 25, 1419–1422. doi:10.1029/98gl01095
Hu, Y. (2007). Depolarization Ratio-Effective Lidar Ratio Relation: Theoretical Basis for Space Lidar Cloud Phase Discrimination. Geophys. Res. Lett. 34, L11812. doi:10.1029/2007GL029584
Hu, Y., Liu, Z., Winker, D., Vaughan, M., Noel, V., Bissonnette, L., et al. (2006). Simple Relation between Lidar Multiple Scattering and Depolarization for Water Clouds. Opt. Lett. 31, 1809–1811. doi:10.1364/ol.31.001809
Hu, Y., Vaughan, M., McClain, C., Behrenfeld, M., Maring, H., Anderson, D., et al. (2007). Global Statistics of Liquid Water Content and Effective Number Concentration of Water Clouds over Ocean Derived from Combined CALIPSO and MODIS Measurements. Atmos. Chem. Phys. 7, 3353–3359. doi:10.5194/acp-7-3353-2007
Li, J., Hu, Y., Huang, J., Stamnes, K., Yi, Y., and Stamnes, S. (2011). A New Method for Retrieval of the Extinction Coefficient of Water Clouds by Using the Tail of the CALIOP Signal. Atmos. Chem. Phys. 11, 2903–2916. doi:10.5194/acp-11-2903-2011
Li, J., Jian, B., Huang, J., Hu, Y., Zhao, C., Kawamoto, K., et al. (2018). Long-term Variation of Cloud Droplet Number Concentrations from Space-Based Lidar. Remote Sensing Environ. 213, 144–161. doi:10.1016/j.rse.2018.05.011
Mace, G. G., Benson, S., and Hu, Y. (2020). On the Frequency of Occurrence of the Ice Phase in Supercooled Southern Ocean Low Clouds Derived from CALIPSO and CloudSat. Geophys. Res. Lett. 47, e2020GL087554. doi:10.1029/2020GL087554
Platnick, S., Meyer, K. G., King, M. D., Wind, G., Amarasinghe, N., Marchant, B., et al. (2017). The MODIS Cloud Optical and Microphysical Products: Collection 6 Updates and Examples from Terra and Aqua. IEEE Trans. Geosci. Remote Sensing 55 (1), 502–525. doi:10.1109/TGRS.2016.2610522
Wood, R. (2006). Rate of Loss of Cloud Droplets by Coalescence in Warm Clouds. J. Geophys. Res. 111, D21205. doi:10.1029/2006JD007553
Keywords: CALIPSO, water cloud, microphysics, number concentration, water content
Citation: Hu Y, Lu X, Zhai P-W, Hostetler CA, Hair JW, Cairns B, Sun W, Stamnes S, Omar A, Baize R, Videen G, Mace J, McCoy DT, McCoy IL and Wood R (2021) Liquid Phase Cloud Microphysical Property Estimates From CALIPSO Measurements. Front. Remote Sens. 2:724615. doi: 10.3389/frsen.2021.724615
Received: 13 June 2021; Accepted: 18 August 2021;
Published: 08 September 2021.
Edited by:
Howard Barker, Environment and Climate Change, CanadaReviewed by:
Husi Letu, Institute of Remote Sensing and Digital Earth (CAS), ChinaWeizhen Hou, Aerospace Information Research Institute, (CAS), China
Copyright © 2021 Hu, Lu, Zhai, Hostetler, Hair, Cairns, Sun, Stamnes, Omar, Baize, Videen, Mace, McCoy, McCoy and Wood. This is an open-access article distributed under the terms of the Creative Commons Attribution License (CC BY). The use, distribution or reproduction in other forums is permitted, provided the original author(s) and the copyright owner(s) are credited and that the original publication in this journal is cited, in accordance with accepted academic practice. No use, distribution or reproduction is permitted which does not comply with these terms.
*Correspondence: Yongxiang Hu, yongxiang.hu-1@nasa.gov