- 1NASA Langley Research Center (LaRC), Hampton, VA, United States
- 2University of Rhode Island, Graduate School of Oceanography, Narragansett, RI, United States
- 3NASA Goddard Institute for Space Studies (GISS), New York, NY, United States
- 4Science Systems and Applications, Inc., Hampton, VA, United States
- 5Korea Astronomy and Space Science Institute (KASI), Daejeon, South Korea
- 6Department of Lunar and Planetary Science, University of Science and Technology, Daejeon, South Korea
- 7Department of Applied Physics and Applied Mathematics, Columbia University, Broadway, NY, United States
- 8Korea Advanced Institute of Science and Technology, Daejeon, South Korea
- 9Department of Astronomy and Space Science, Kyung Hee University, Yongin, South Korea
- 10Humanitas College, KHU, Yongin, South Korea
- 11Department of Atmospheric and Planetary Sciences, Hampton University, Hampton, VA, United States
- 12Center for Climate Systems Research, Columbia University, New York, NY, United States
- 13Now at SRON Netherlands Institute for Space Research, Leiden, Netherlands
- 14US Army Research Laboratory, Adelphi, MD, United States
- 15Space Science Institute, Boulder, CO, United States
- 16SciSpace LLC, Bethesda, MD, United States
We quantify the performance of aerosol and ocean remote sensing products from the PolCube instrument using a previously developed polarimeter retrieval algorithm based on optimal estimation. PolCube is a modified version of the PolCam lunar instrument on the Korea Pathfinder Lunar Orbiter that has been optimized for Earth-Science observations of aerosol, ocean, and thin cloud optical properties. The objective of the PolCube instrument is to retrieve detailed fine-mode (pollution and smoke) and coarse-mode (sea-salt and dust) aerosol properties over the ocean for a range of light to heavy aerosol loadings using its polarimetric-imaging capability at multiple angles and wavelengths from
1 Introduction
The 2017 Decadal Survey for Earth Science and Applications from Space identifies aerosol absorption as one of the key geophysical variables to address as part of the Aerosols Designated Observable (National Academies of Sciences and Medicine, 2018), which is now part of the future NASA Aerosols, Clouds, Convection and Precipitation (ACCP) mission (Braun et al., 2019). Aerosol absorption or SSA is one of the most difficult aerosol properties to retrieve from remote-sensing measurements. Retrieval of aerosol absorption requires simultaneous retrieval of aerosol height; currently, aerosol location represents one of the largest uncertainties of aerosol-absorption retrievals (Buchard et al., 2015). Improved quantification of aerosol absorption is critical for applications including aerosol direct radiative forcing, actinic flux for photochemistry applications, aerosol speciation, aerosol transport, aerosol processes such as aging, and the retrieval of ocean color properties (Stamnes et al., 2018). Aerosol absorption is critical for calculating aerosol direct radiative forcing, the uncertainty of which is still large even over clear-sky ocean (±1.25 W/m2) (Thorsen et al., 2020). Aerosol absorption is needed for atmospheric-chemistry applications to quantify accurately the actinic flux, which controls photolysis rates (Tian et al., 2019) and particle formation that create cloud-condensation nuclei (Zheng et al., 2021). Aerosol absorption is important for aerosol speciation since aerosols produced by biomass burning and combustion processes from industry and automobiles can be highly absorbing.
Aerosol absorption is critical for accurate retrieval of water-leaving radiances in coastal zones where the presence of multiple aerosol types above complex waters creates tremendous challenges for single-angle multi-wavelength instruments (Kahn et al., 2016). Yet the coastal zones are where a majority of people live and these zones support activities such as tourism, recreation, agriculture, transportation, and fisheries. Moreover, these estuarine environments are where the greatest concentrations of organic and inorganic nutrients are found and transformed. Complex aerosol situations can arise in coastal zones where the continental outflows of pollution, smoke and dust mix together in layers, become processed by clouds, and absorb water, change shape, bleach, and undergo photochemical transformations. Retrieval of aerosol properties in coastal zones is complicated by the complexity of ocean water because of terrestrial river outflows, sewer discharges, plankton blooms, and resuspension of unconsolidated bottom sediments (Loisel et al., 2013).
Aerosol speciation is significantly improved by knowledge of absorption, size, and real refractive index. Aerosol size and real refractive index are linked to relative humidity since hygroscopic aerosols grow in size and approach the real refractive index of water as they collect moisture (Schuster et al., 2009). Accurate quantification of aerosol size and real refractive index can improve our understanding of the Earth’s water cycle and aerosol-cloud interactions. In turn, improved aerosol speciation allows for improved aerosol air quality forecasts and monitoring (Lin et al., 2005; Dubovik et al., 2019), aerosol transport (Chin et al., 2007) and chemical transformations (Tian et al., 2019).
Thus, accurate and reliable remote sensing of aerosol microphysical properties, such as absorption and size, is necessary to address critical unanswered questions in Earth Science from aerosols’ impact on climate change to the health of the oceans. Single-angle multi-wavelength total radiance (intensity) measurements such as from the Moderate Resolution Imaging Spectroradiometer (MODIS) or the Visible Infrared Imaging Radiometer Suite (VIIRS) are unable to retrieve aerosol absorption, and aerosol absorption is difficult to characterize from multi-angle intensity measurements such as from the Multi-angle Imaging SpectroRadiometer (MISR). Multi-angle multi-channel total and polarized radiance measurements are necessary to determine aerosol absorption reliably. The only currently known and practical method to characterize column-averaged aerosol optical and microphysical properties from space is through multi-angle multi-wavelength polarimeter measurements (Cairns et al., 1999; Mishchenko et al., 2004; Mishchenko et al., 2007; Knobelspiesse et al., 2012; Stamnes et al., 2018; Chowdhary et al., 2019; Dubovik et al., 2019). Collocated multi-channel UV-VIS-NIR lidar measurements, particularly high-spectral-resolution lidar measurements are synergistic with polarimeter measurements and can enable the retrieval of vertically-resolved aerosol optical and microphysical properties (Liu et al., 2016). For that reason the NASA ACCP mission calls for a high-spectral resolution lidar together with a multi-angle multi-channel polarimeter to resolve vertical aerosol properties, such as absorption in the free troposphere and planetary boundary layer. Unfortunately, ACCP is not expected to launch until 2027 or later. Nonetheless, the outlook for polarimetry is promising with four set to launch in the 2023–2024 timeframe (3MI/MetOp-SG, SPEXone/PACE, HARP2/PACE, MAIA). Despite this promising outlook, currently there is a lack of satellite polarimetric measurements meaning aerosol absorption is poorly quantified. Polarimeters with finer spatial resolutions of ∼1 km and better allow for neighborhood-resolved air quality monitoring, enhanced water quality monitoring in heterogeneous coastal zones, improved cloud screening, and the retrieval of small, broken cumuliform cloud microphysical properties. Enhanced polarimetric accuracy enables improved measurements of aerosol properties such as absorption. Crucially, dramatically reduced instrument costs are needed to enable constellations of small satellite polarimeters that collect measurements at multiple times each day to resolve hourly variations in aerosol and cloud properties. An example of this dramatic reduction price without sacrifice in capability is the HARP polarimeter that deployed from ISS in 2020 (Martins et al., 2018).
To further the goal of high capability and low cost, we consider a modification to the Wide-Angle Polarimetric Camera (PolCam) (Jeong et al., 2018) that can reliably and accurately retrieve aerosol optical and microphysical properties across the VIS-NIR. PolCam was developed for the KPLO mission and will make lunar polarimetric observations beginning in 2023 (Ju, 2017; Jeong et al., 2018; Sim et al., 2019). The PolCam instrument was designed to measure the lunar bidirectional reflectance and polarized reflectance distribution functions (BRDF and BPDF) and to retrieve lunar grain size. In this paper we introduce a modified version of this polarimeter instrument for Earth-Science applications called PolCube. PolCube will provide improved characterization of the absorption and scattering by small particles (aerosols) in the Earth’s atmosphere, which requires total radiance and polarized radiance measurements from multiple angles and at multiple wavelengths. We quantify PolCube’s ability to characterize aerosols by analyzing the retrieval capability of detailed aerosol optical and microphysical properties including aerosol absorption, effective radius and refractive index using the Microphysical Aerosol Properties from Polarimetry (MAPP) retrieval algorithm (Stamnes et al., 2018). Another target of observation for PolCube is the detection of thin liquid water and cirrus clouds using polarized reflectance measurements near backscattering viewing geometries (Sun et al., 2014) and the characterization of ice cloud crystal shape and scattering properties (van Diedenhoven et al., 2012). The miniature and cost-effective design of new CubeSat polarimeters such as PolCube and HARP (Martins et al., 2018) are poised to provide greatly enhanced spatial (1 km) and temporal coverage of aerosol and cloud optical and microphysical properties. PolCube is a joint international and educational outreach mission between NASA Langley Research Center (LaRC), NASA Goddard Institute for Space Studies (GISS), the Korea Astronomy and Space Science Institute (KASI), Kyung Hee University, Hampton University, University of Rhode Island, Army Research Lab and the city of Busan, Republic of Korea. PolCube is expected to launch in 2023.
The PolCube design, modified for Earth observations, is based on a rich dataset of aircraft polarimeter measurements (Cairns et al., 1999). PolCube has two cameras whose wavelengths and viewing angles are optimized for remote sensing of aerosols in the Earth’s atmosphere. The PolCube instrument is outlined in Section 2. The aerosol and ocean remote-sensing retrieval capability of PolCube is analyzed starting from Section 2.1. PolCube’s measurement capabilities are described in Section 2.2, and the aerosol model is described in Section 2.3. PolCube’s products and their uncertainties are listed in Section 2.4. A desired orbit for PolCube and collocation of PolCube data with GEMS, TEMPO and Sentinel-4 is discussed in Section 2.5. A conclusion is provided in Section 3.
2 PolCube Instrument Design
The PolCube concept and design, based on PolCam (Jeong et al., 2018; Sim et al., 2019), is described in Figure 1 and Table 1. PolCube consumes approximately 10 W, weighs 4 kg, occupies a volume of 8 U, and will launch as a 19 U CubeSat upon integration with the spacecraft bus. The two optical heads (cameras) of PolCube will be separated by
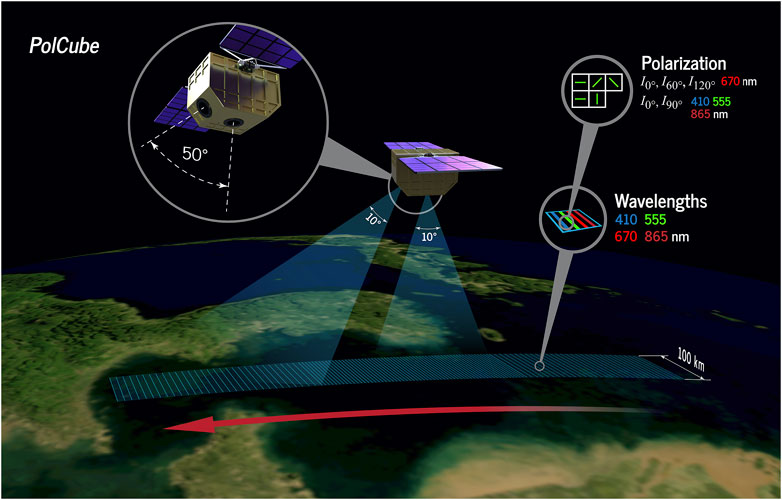
FIGURE 1. The PolCube CubeSat polarimeter mission will study aerosols and complement current GEMS, TEMPO and Sentinel-4 satellite observations, and future PACE, 3MI/MetOp-SG and ACCP missions. SSAI/Tim Marvel.
Each PolCube camera is a pushbroom imager as depicted in Figure 1. The cameras have a
The detector wavelengths and bandwidths for PolCube are based on the airborne NASA GISS Research Scanning Polarimeter (RSP) (Cairns et al., 1999) design that was optimized for aerosol observations. The center wavelengths and the full width half maximum (FWHM) bandwidths in parentheses are
There is a tradeoff between swath and the number of viewing angles. The goal is to achieve a swath of 100 km in the cross-track while ensuring enough viewing angles in order to retrieve aerosol optical and microphysical properties to within the desired uncertainties. A study was performed to trade the number of cameras (
2.1 PolCube Aerosol and Ocean Performance Analysis
The objective is to perform an aerosol and ocean property-retrieval performance study of aerosol optical and microphysical properties from the PolCube polarimeter satellite instrument. The performance of PolCube is assessed by performing retrievals on synthetic PolCube data for aerosol and ocean properties that were randomly generated by Monte Carlo as by Stamnes et al. (2018). These randomly varying input parameters are subsequently used by a vector radiative transfer (RT) code (Hansen and Travis, 1974; Cairns et al., 1999) to generate synthetic PolCube data for a wide range of scenarios with different aerosol properties, ocean windspeeds and sub-surface inherent optical properties (IOP)s and viewing geometries (Stamnes et al., 2018; Hasekamp et al., 2019).
The inversion of PolCube synthetic data is carried out using the MAPP algorithm. The state vector of PolCube MAPP retrieval parameters is defined as
where
where v is the windspeed in m/s and [Chla] is the chlorophyll-a concentration in mg/m3.
2.2 Synthetic PolCube Data and Measurement Error Model
PolCube measures the TOA total radiance and the degree of linear polarization
2.3 Aerosol Model
To simulate aerosol retrieval performance by PolCube, we use three types of distinct aerosols externally mixed together: a fine-mode aerosol component, a sea-salt aerosol component, and a dust aerosol component. The fine-mode aerosol component (used to denote aerosol particles that have effective radii
The following aerosol parameters and ranges are investigated for these three aerosol components (see the first three columns in Table 2). In terms of amount, the fine-mode AOD555 varies from 0 to 0.5, the sea-salt AOD555 varies from 0 to 0.2, and the dust AOD555 varies from 0 to 0.2. In terms of size, the fine-mode effective radius varies from 0.1 to 0.4 μm (in mode radius, 0.063–0.254 μm), the sea-salt effective radius from 1.0 to 3.5 μm (in mode radius, 0.308–1.079 μm), and the dust effective radius from 1.0 to 4.0 μm (in mode radius, 0.176–0.706 μm). In terms of composition, the fine-mode real part of the refractive index varies from 1.36 to 1.65 and the fine-mode imaginary part of the refractive index varies from 0 to 0.03 (resulting in a range of SSA from 0.8 to 1.0). In addition, the following two ocean surface parameters are varied: windspeed from 0.02 to 11.5 m/s and chlorophyll-a concentration from 0.025 to 9.9 mg/m3.
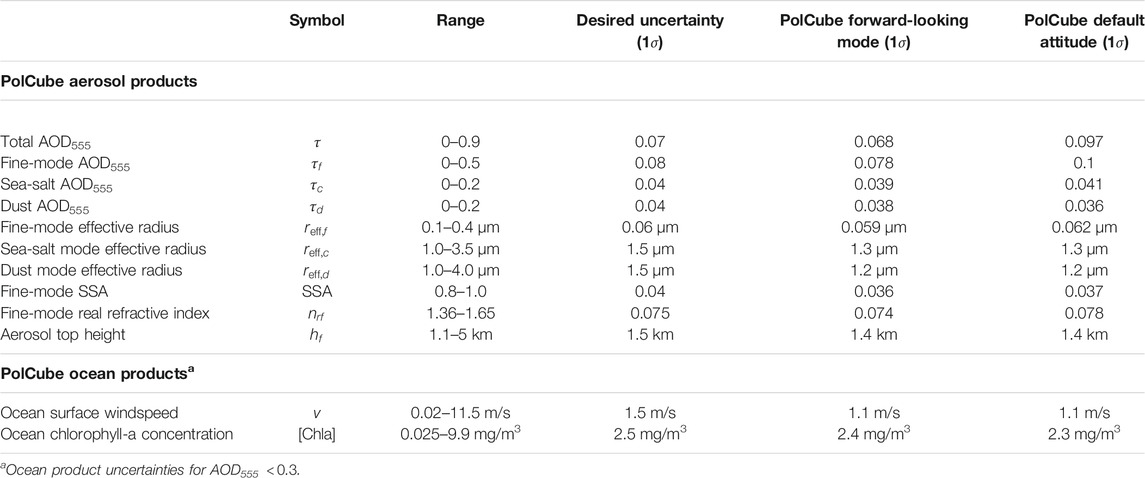
TABLE 2. PolCube aerosol and ocean products. The ranges used in the Monte Carlo simulations are listed in the third column. The fourth column lists desired uncertainties, and the corresponding PolCube simulated retrieval performance is in the fifth column (forward-looking attitude) and sixth column (default attitude). All uncertainties are given as
The following assumptions for the aerosol and ocean surface parameters are made, or assumed, to be known a priori. It is assumed that the aerosol particles can be approximated by spherical particles with lognormal size distributions. The fine-mode effective variance is assumed to be 0.2 (in mode width, 0.427). The fine-mode real and imaginary parts of the complex refractive index can vary, but are assumed to be constant with wavelength, which is generally considered to be a reasonable assumption in the VIS-NIR. The sea-salt complex refractive index is fixed to 1.01 times that of water (
The aerosol state vector is therefore defined as
where
2.4 PolCube Aerosol and Ocean Products
The PolCube retrieval performance of the forward-looking mode for several aerosol and ocean properties are given in Figure 2. The total AOD555 is depicted in Figure 2A. Using RMSD as a measure of retrieval performance, such that we expect to be within
where
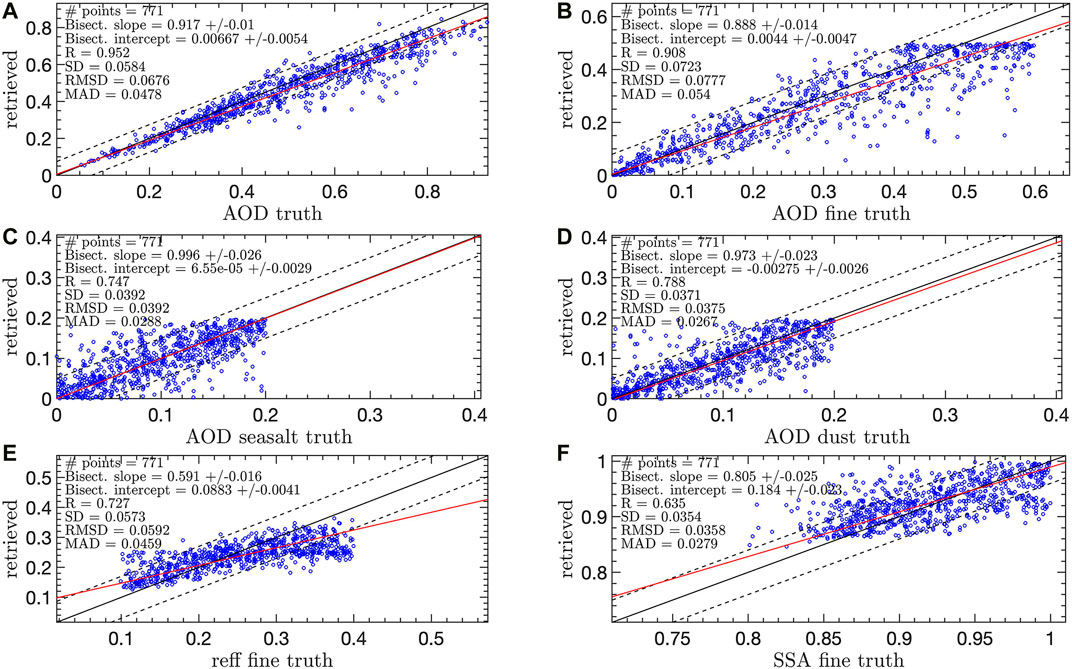
FIGURE 2. PolCube retrieval performance of aerosol optical and microphysical properties of the forward-looking mode. The root-mean-square deviation (RMSD) is used to estimate
The PolCube retrieval performance of the AOD555 for each of the three aerosol species separately is depicted in Figures 2B–D. The
A summary of PolCube retrieval performance for all aerosol and ocean products is given in Table 2. The desired uncertainty targets are listed in the fourth column. The performance of PolCube’s forward-looking mode, which can be used to target four regions per day, is given in the fifth column. By comparison, the default observation attitude leads to increased uncertainties by single digit percentages for all properties except total and fine-mode AOD, which have increased uncertainties of 43 and 33%, respectively. All of the aerosol and ocean products meet the desired uncertainties under the forward-looking mode. And all products except AOD meet or nearly meet the desired uncertainties under the default operating attitude. On this last point, we note that for global observations using Polcube’s default observing attitude, the presence of three aerosol modes is probably overly complex and may underestimate performance: we would expect improved total and fine-mode AOD performance when the aerosol is only a mixture of two species, e.g. fine mode and sea-salt only.
2.5 PolCube Orbit and GEMS, TEMPO and Sentinel-4 Collocated Data
GEMS (Kim et al., 2020) is a geostationary mission designed to monitor trans-boundary pollution events for the Korean peninsula and the Asia-Pacific region using a scanning ultraviolet-visible spectrometer (300–500 nm) with hyperspectral resolution (0.6 nm). GEMS will contribute to the understanding of pollution events, source/sink identification, and long-range transport of pollutants and SLCFs (Short-Lived Climate Forcers) as a part of the activities of the Atmospheric Composition Constellation under CEOS (Committee on Earth Observation Satellites). GEMS, which obtains observations of the Asia Pacific region, is part of a constellation that includes other geostationary pollution monitoring satellites: TEMPO (Tropospheric Emissions: Monitoring Pollution, North America) and Sentinel-4 (Europe and North Africa). GEMS has been designed to provide hourly measurements of aerosols and ozone at high spatial resolution over Asia and to monitor regional transport events of transboundary pollution and Asian dust. GEMS is also designed to improve our understanding of the interactions between atmospheric chemistry and meteorology, and interhemispheric transport of Asian pollution across the Pacific.
A proposed orbit that would maximize synergies between PolCube and GEMS (and TEMPO and Sentinel-4) is depicted in Figure 3. This particular sun-synchronous orbit has a ground-track repeat of one day, which means the ground tracks would not shift. The spacecraft is moving approximately northward when it passes over the ground tracks directed from southeast to northwest; each point at which the spacecraft passes northbound through the equatorial plane is known as an ascending node, and the time of each such crossing is local solar noon. The subsatellite point is in daylight in the sections of ground track shown with a black curve. After the spacecraft reaches its northernmost latitude, it moves approximately southward over tracks (not shown) directed from northeast to southwest and each southbound pass through the equatorial plane occurs at local solar midnight. A non-shifting ground track together with PolCube’s pointing capabilities can maximize collocation of PolCube VIS-NIR data with GEMS for a target region. Collocated PolCube data will improve GEMS aerosol retrievals by simultaneously measuring multi-angular total and polarized radiances across a wavelength range of 400–865 nm. In particular, PolCube’s longer wavelength observations will help GEMS to improve retrieval of the coarse-mode AOD555 (sea-salt and dust aerosol) and aerosol speciation via separation of the total AOD555 into fine- and coarse-mode AOD555. Critically, it is expected that PolCube will be able to improve GEMS estimates of aerosol absorption, aerosol height, and surface reflectance, which represent the largest uncertainties in retrievals of ozone and trace gases. PolCube’s polarized radiance measurements at 410 nm are particularly useful for constraining aerosol height compared to radiance-only measurements (Wu et al., 2015), and can also assist GEMS to correct for intensity polarization sensitivity effects.
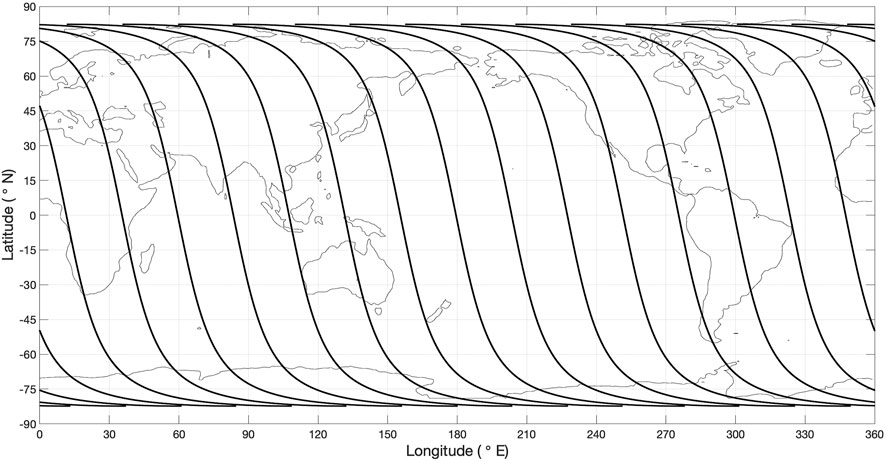
FIGURE 3. A proposed orbit for the PolCube instrument chosen for synergy with the GEMS, TEMPO and Sentinel-4. This unique sun-synchronous orbit has an altitude of 566.90 km with an inclination of
PolCube will help address GEMS objectives related to aerosol transport and air quality, including outflows from the Asian continent, and can contribute to atmospheric-chemistry studies by improving retrievals of the actinic flux that is required for photochemistry applications and is a function of AOD555, height and SSA (absorption). PolCube is also expected to improve estimates of particulate composition through the retrieval of aerosol effective radius (size) and refractive index (chemical composition). PolCube will make measurements in the Yellow Sea of trans-Pacific fine-mode pollution transport, separate from sea-salt and dust aerosol, providing critically needed data for regional air-quality studies (Shin et al., 2015; Noh et al., 2016). PolCube can help GEMS provide enhanced retrievals of spatial and temporal variations of aerosol properties, facilitate studies of aerosol-cloud interactions and meteorological impacts, and improve air quality forecasts.
3 Conclusion and Future Work
Accurate and reliable retrieval of aerosol microphysical and optical properties such as SSA (absorption) is a difficult problem. However, solving this aerosol problem becomes possible using a multi-channel, multi-angle polarimeter together with retrieval algorithms that correctly treat the atmosphere and ocean as a coupled system and that can optimize total and polarization measurements from multiple channels simultaneously. We have used an accurate, coupled vector radiative-transfer model with optimal estimation to investigate the performance of the future PolCube CubeSat polarimeter which takes its heritage from the PolCam lunar polarimeter on KPLO. PolCube significantly improves upon current aerosol satellite measurements with its capability to retrieve aerosol microphysical properties such as speciated AOD, effective radius and SSA.
The real-world is rich in diversity and cannot be easily replicated via simulations, but we outline a simple yet robust performance analysis that uses Monte Carlo-style simulations to randomly vary aerosol and ocean conditions. By combining this Monte Carlo-style aerosol and ocean model with accurate vector radiative-transfer calculations of the coupled atmosphere and ocean system, together with reasonable estimates for the PolCube instrument measurement uncertainties, we can provide a reasonable estimate of real-world aerosol-retrieval performance. The validity assumes that the ranges and assumptions that we made reasonably cover the actual range of observations. The assumption that all the aerosol and ocean parameters are essentially uncorrelated, when in fact they may be correlated, may cause performance to be underestimated. This underestimation is to some extent balanced by a potential overestimate of performance because variability in aerosol and ocean properties that are not captured completely by our simplified Monte Carlo simulations will also need to be retrieved or constrained by adding a priori information. Furthermore, proper cloud screening is needed to perform aerosol retrievals. The multi-angle polarization measurements provide sensitivity to detect clouds, including thin cirrus (Sun et al., 2014; Stap et al., 2015).
Overall, the aerosol retrieval of PolCube with four channels 410, 555, 670, and 865 nm and four viewing zenith angles from two cameras centered at nadir and approximately
Future work involves analyzing and applying PolCube for additional applications. These include air quality, atmospheric chemistry, water quality, and terrestrial biogeophysical properties, including cryosphere properties, such as snow grain impurity, size and shape, in addition to exploring the use of combined PolCube measurements and retrieval products with other satellite measurements such as from GEMS, TEMPO and Sentinel-4.
Data Availability Statement
The raw data supporting the conclusion of this article will be made available by the authors, without undue reservation.
Author Contributions
PolCube design: SS, RB, PB, BC, Y-JC, YH, MJ, K-IK, SK, DM, BM, AO, CS, WS, and GV. PolCube hardware: Y-JC, MJ, K-IK, and BM. Orbit and viewing geometry considerations: SS, BC, Y-JC, JC, YH, BD, JC, YH, MJ, SK, AO, CR, CS, WS, BD, GV, and AW. Algorithm development and quality control: SS, BC, EC, JC, YH, SK, XL, RL, PM, AO, CS, and GV. Text: All authors.
Funding
The work by authors SS, RB, EC, YH, DM, AO, CR and WS was funded by NASA Langley Research Center. The work by SK was supported by the National Research and ICT (2019R1A2C1009004).
Conflict of Interest
Author EC was employed by the company Science Systems and Applications, Inc. Author AW was employed by the company SciSpace LLC.
The remaining authors declare that the research was conducted in the absence of any commercial or financial relationships that could be construed as a potential conflict of interest.
Publisher’s Note
All claims expressed in this article are solely those of the authors and do not necessarily represent those of their affiliated organizations, or those of the publisher, the editors and the reviewers. Any product that may be evaluated in this article, or claim that may be made by its manufacturer, is not guaranteed or endorsed by the publisher.
Acknowledgments
We would like to acknowledge Tim Valle for his contributions to the text and instrument descriptions. We would like to acknowledge Dave Young for his support and encouragement.
References
Braun, S. A., da Silva, A., Ferrare, R., Kacenelenbogen, M., Petersen, W., Stephens, G., et al. (2019). “The NASA Decadal Survey Observing-System Study for Aerosols and Clouds, Convection, and Precipitation (ACCP),” in AGU Fall Meeting 2019 (AGU).
Buchard, V., da Silva, A. M., Colarco, P. R., Darmenov, A., Randles, C. A., Govindaraju, R., et al. (2015). Using the OMI Aerosol index and Absorption Aerosol Optical Depth to Evaluate the NASA MERRA Aerosol Reanalysis. Atmos. Chem. Phys. 15, 5743–5760. doi:10.5194/acp-15-5743-2015
Cairns, B., Russell, E. E., and Travis, L. D. (1999). “Research Scanning Polarimeter: Calibration and Ground-Based Measurements,” in SPIE’s International Symposium on Optical Science, Engineering, and Instrumentation(International Society for Optics and Photonics), 186–196.
Chin, M., Diehl, T., Ginoux, P., and Malm, W. (2007). Intercontinental Transport of Pollution and Dust Aerosols: Implications for Regional Air Quality. Atmos. Chem. Phys. 7, 5501–5517. doi:10.5194/acp-7-5501-2007
Chowdhary, J., Cairns, B., Waquet, F., Knobelspiesse, K., Ottaviani, M., Redemann, J., et al. (2012). Sensitivity of Multiangle, Multispectral Polarimetric Remote Sensing over Open Oceans to Water-Leaving Radiance: Analyses of RSP Data Acquired during the MILAGRO Campaign. Remote Sensing Environ. 118, 284–308. doi:10.1016/j.rse.2011.11.003
Chowdhary, J., Zhai, P.-W., Boss, E., Dierssen, H., Frouin, R., Ibrahim, A., et al. (2019). Modeling Atmosphere-Ocean Radiative Transfer: A PACE mission Perspective. Front. Earth Sci. 7, 100. doi:10.3389/feart.2019.00100
Dubovik, O., Holben, B., Eck, T. F., Smirnov, A., Kaufman, Y. J., King, M. D., et al. (2002). Variability of Absorption and Optical Properties of Key Aerosol Types Observed in Worldwide Locations. J. Atmos. Sci. 59, 590–608. doi:10.1175/1520-0469
Dubovik, O., Li, Z., Mishchenko, M. I., Tanré, D., Karol, Y., Bojkov, B., et al. (2019). Polarimetric Remote Sensing of Atmospheric Aerosols: Instruments, Methodologies, Results, and Perspectives. J. Quantitative Spectrosc. Radiative Transfer 224, 474–511. doi:10.1016/j.jqsrt.2018.11.024
Hansen, J. E., and Travis, L. D. (1974). Light Scattering in Planetary Atmospheres. Space Sci. Rev. 16, 527–610. doi:10.1007/bf00168069
Hasekamp, O. P., Fu, G., Rusli, S. P., Wu, L., Di Noia, A., Brugh, J. a. d., et al. (2019). Aerosol Measurements by SPEXone on the NASA PACE mission: Expected Retrieval Capabilities. J. Quantitative Spectrosc. Radiative Transfer 227, 170–184. doi:10.1016/j.jqsrt.2019.02.006
Jeong, M., Choi, Y.-J., Kim, S. S., Kim, I.-H., Shkuratov, Y. G., and Yang, H. (2018). Multi-band Polarimetry of the Lunar Surface. Ii. Grain Size Evolutionary Pathway. Astrophysi. J. 869, 67. doi:10.3847/1538-4357/aae9ed
Ju, G. (2017). “Korean Pathfinder Lunar Orbiter (Kplo) Status Update,” in 2017 Annual Meeting of the Lunar Exploration Analysis Group, October 10–12, 2107, Columbia, MarylandUniversities Space Research Association Headquarters at 7178 Columbia Gateway Dr., 1–19.
Kahn, R. A., Sayer, A. M., Ahmad, Z., and Franz, B. A. (2016). The Sensitivity of Seawifs Ocean Color Retrievals to Aerosol Amount and Type. J. Atmos. Oceanic Tech. 33, 1185–1209. doi:10.1175/jtech-d-15-0121.1
Kim, J., Jeong, U., Ahn, M.-H., Kim, J. H., Park, R. J., Lee, H., et al. (2020). New era of Air Quality Monitoring from Space: Geostationary Environment Monitoring Spectrometer (GEMS). Bull. Am. Meteorol. Soc. 101, E1–E22. doi:10.1175/bams-d-18-0013.1
Knobelspiesse, K., Cairns, B., Mishchenko, M., Chowdhary, J., Tsigaridis, K., van Diedenhoven, B., et al. (2012). Analysis of fine-mode Aerosol Retrieval Capabilities by Different Passive Remote Sensing Instrument Designs. Opt. Express 20, 21457–21484. doi:10.1364/oe.20.021457
Lin, C., Liu, S., Chou, C., Huang, S., Liu, C., Kuo, C., et al. (2005). Long-range Transport of Aerosols and Their Impact on the Air Quality of Taiwan. Atmos. Environ. 39, 6066–6076. doi:10.1016/j.atmosenv.2005.06.046
Liu, X., Stamnes, S., Ferrare, R. A., Hostetler, C. A., Burton, S. P., Chemyakin, E., et al. (2016). “Development of a Combined Lidar-Polarimeter Inversion Algorithm for Retrieving Aerosol.” in AGU Fall Meeting Abstracts, December 12–16, 2016, San Francisco, California 2016, A54E–A06.
Loisel, H., Vantrepotte, V., Jamet, C., and Dat, D. N. (2013). Challenges and New Advances in Ocean Color Remote Sensing of Coastal Waters. Top. Oceanography, 1–38. doi:10.5772/56414
Martins, J. V., Fernandez-Borda, R., McBride, B., Remer, L., and Barbosa, H. M. (2018). “The HARP Hyperangular Imaging Polarimeter and the Need for Small Satellite Payloads with High Science Payoff for Earth Science Remote Sensing,” in IGARSS 2018-2018 IEEE International Geoscience and Remote Sensing Symposium(IEEE), 6304–6307. doi:10.1109/igarss.2018.8518823
Mishchenko, M. I., Cairns, B., Hansen, J. E., Travis, L. D., Burg, R., Kaufman, Y. J., et al. (2004). Monitoring of Aerosol Forcing of Climate from Space: Analysis of Measurement Requirements. J. Quantitative Spectrosc. Radiative Transfer 88, 149–161. doi:10.1016/j.jqsrt.2004.03.030
Mishchenko, M. I., Cairns, B., Kopp, G., Schueler, C. F., Fafaul, B. A., Hansen, J. E., et al. (2007). Accurate Monitoring of Terrestrial Aerosols and Total Solar Irradiance: Introducing the Glory mission. Bull. Am. Meteorol. Soc. 88, 677–692. doi:10.1175/bams-88-5-677
National Academies of Sciences, E. and Medicine (2018). Thriving on Our Changing Planet: A Decadal Strategy for Earth Observation from Space. Washington, DC: The National Academies Press. doi:10.17226/24938
Noh, Y. M., Lee, K., Kim, K., Shin, S.-K., Müller, D., and Shin, D. H. (2016). Influence of the Vertical Absorption Profile of Mixed Asian Dust Plumes on Aerosol Direct Radiative Forcing over East Asia. Atmos. Environ. 138, 191–204. doi:10.1016/j.atmosenv.2016.04.044
Schuster, G. L., Lin, B., and Dubovik, O. (2009). Remote Sensing of Aerosol Water Uptake. Geophys. Res. Lett. 36, a–n. doi:10.1029/2008GL036576
Shin, S.-K., Müller, D., Lee, C., Lee, K. H., Shin, D., Kim, Y. J., et al. (2015). Vertical Variation of Optical Properties of Mixed Asian Dust/pollution Plumes According to Pathway of Air Mass Transport over East Asia. Atmos. Chem. Phys. 15, 6707–6720. doi:10.5194/acp-15-6707-2015
Sim, C. K., Kim, S. S., Jeong, M., Choi, Y.-J., and Shkuratov, Y. G. (2019). Observational Strategy for KPLO/PolCam Measurements of the Lunar Surface from Orbit. Pasp 132, 015004. doi:10.1088/1538-3873/ab523d
Stamnes, S., Hostetler, C., Ferrare, R., Burton, S., Liu, X., Hair, J., et al. (2018). Simultaneous Polarimeter Retrievals of Microphysical Aerosol and Ocean Color Parameters from the "MAPP" Algorithm with Comparison to High-Spectral-Resolution Lidar Aerosol and Ocean Products. Appl. Opt. 57, 2394–2413. doi:10.1364/ao.57.002394
Stap, F. A., Hasekamp, O. P., and Röckmann, T. (2015). Sensitivity of PARASOL Multi-Angle Photopolarimetric Aerosol Retrievals to Cloud Contamination. Atmos. Meas. Tech. 8, 1287–1301. doi:10.5194/amt-8-1287-2015
Sun, W., Videen, G., and Mishchenko, M. I. (2014). Detecting Super-thin Clouds with Polarized Sunlight. Geophys. Res. Lett. 41, 688–693. doi:10.1002/2013gl058840
Thorsen, T. J., Ferrare, R. A., Kato, S., and Winker, D. M. (2020). Aerosol Direct Radiative Effect Sensitivity Analysis. J. Clim. 33, 6119–6139. doi:10.1175/jcli-d-19-0669.1
Tian, R., Ma, X., Jia, H., Yu, F., Sha, T., and Zan, Y. (2019). Aerosol Radiative Effects on Tropospheric Photochemistry with GEOS-Chem Simulations. Atmos. Environ. 208, 82–94. doi:10.1016/j.atmosenv.2019.03.032
van Diedenhoven, B., Cairns, B., Geogdzhayev, I. V., Fridlind, A. M., Ackerman, A. S., Yang, P., et al. (2012). Remote Sensing of Ice crystal Asymmetry Parameter Using Multi-Directional Polarization Measurements - Part 1: Methodology and Evaluation with Simulated Measurements. Atmos. Meas. Tech. 5, 2361–2374. doi:10.5194/amt-5-2361-2012
Wu, L., Hasekamp, O., Van Diedenhoven, B., and Cairns, B. (2015). Aerosol Retrieval from Multiangle, Multispectral Photopolarimetric Measurements: Importance of Spectral Range and Angular Resolution. Atmos. Meas. Tech. 8, 2625–2638. doi:10.5194/amt-8-2625-2015
Keywords: polarimeter, aerosol, ocean, cubesat, remote sensing
Citation: Stamnes S, Baize R, Bontempi P, Cairns B, Chemyakin E, Choi Y-J, Chowdhary J, Hu Y, Jeong M, Kang K-I, Kim SS, Liu X, Loughman R, MacDonnell D, McCormick MP, Moon B, Omar A, Roithmayr CM, Sim CK, Sun W, van Diedenhoven B, Videen G and Wasilewski A (2021) Simultaneous Aerosol and Ocean Properties From the PolCube CubeSat Polarimeter. Front. Remote Sens. 2:709040. doi: 10.3389/frsen.2021.709040
Received: 13 May 2021; Accepted: 05 July 2021;
Published: 10 August 2021.
Edited by:
Zhengqiang Li, Aerospace Information Research Institute (CAS), ChinaReviewed by:
Weizhen Hou, Aerospace Information Research Institute (CAS), ChinaYuanjian Yang, Nanjing University of Information Science and Technology, China
Copyright © 2021 United States Government as represented by the Administrator of the National Aeronautics and Space Administration and Bontempi, Choi, Jeong, Kang, Kim, Loughman, McCormick, Moon, Sim. At least a portion of this work is authored by Snorre Stamnes, Rosemary Baize, Brian Cairns, Yongxiang Hu, Xu Liu, Dave MacDonnell, Ali Omar, Carlos Roithmayr and Gorden Videen on behalf of the U.S. Government and, as regards Dr. Stamnes, Ms. Baize, Dr. Cairns, Dr. Hu, Dr. Liu, Dr. MacDonnell, Dr. Omar, Dr. Roithmayr and Dr. Videen. U.S. Copyright protection does not attach to separable portions of a Work authored solely by U.S. Government employees as part of their official duties. The U.S. Government is the owner of foreign copyrights in such separable portions of the Work and is a joint owner (with any non-U.S. Government author) of U.S. and foreign copyrights that may be asserted in inseparable portions the Work. The U.S. Government retains the right to use, reproduce, distribute, create derivative works, perform and display portions of the Work authored solely or co- authored by a U.S. Government employee. Non-U.S copyrights also apply. This is an open access article distributed under the terms of the Creative Commons Attribution License (CC BY). The use, distribution or reproduction in other forums is permitted, provided the original author(s) and the copyright owner(s) are credited and that the original publication in this journal is cited, in accordance with accepted academic practice. No use, distribution or reproduction is permitted which does not comply with these terms.
*Correspondence: Snorre Stamnes, c25vcnJlLmEuc3RhbW5lc0BuYXNhLmdvdg==