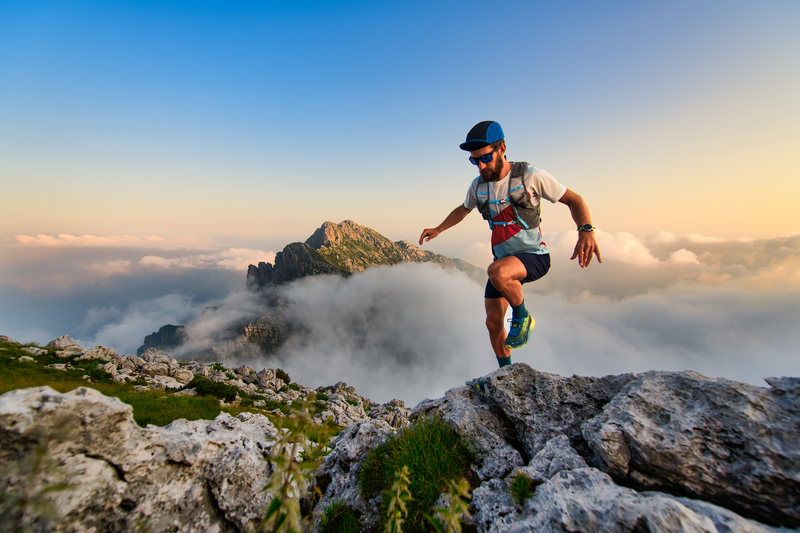
94% of researchers rate our articles as excellent or good
Learn more about the work of our research integrity team to safeguard the quality of each article we publish.
Find out more
CONCEPTUAL ANALYSIS article
Front. Sustain. Cities , 06 January 2025
Sec. Innovation and Governance
Volume 6 - 2024 | https://doi.org/10.3389/frsc.2024.1473123
This article is part of the Research Topic Data Analytics in Sustainable City Planning View all 3 articles
Integrating data-driven technologies is increasingly crucial for developing Smart Cities, particularly in enhancing urban operations and ensuring inclusivity. As cities worldwide adopt Smart City initiatives, understanding the role of data in optimising urban infrastructure and services becomes paramount. This research addresses the challenge of effectively utilising data to support inclusive Smart City operations in Thailand. It focuses on real-time monitoring, resource optimisation, and community engagement to improve urban management and sustainability. The study employs a comprehensive review of Thailand’s Smart City initiatives, including using applications like Traffy Fondue. It analyses data from environmental sensors, community engagement tools, and governance platforms to assess their impact on city operations. The research also explores future directions by synthesising current practices and potential data infrastructure and analytics advancements. The findings reveal that data-driven strategies significantly enhance city operations by facilitating real-time issue resolution, informed decision-making, and efficient resource allocation. Integrating environmental monitoring systems and community engagement platforms further promotes sustainability and inclusivity. The study concludes that continued investment in data infrastructure, advanced analytics, and comprehensive data governance is essential. Future research should focus on developing scalable solutions, fostering global collaboration, and implementing supportive policies to maximise the benefits of Smart City technologies. This research provides a model for cities worldwide striving to improve urban environments through data-driven innovations.
Since Thailand introduced the Industry 4.0 strategy into its national city planning and development, it has evolved into a framework describing the seven focus areas for Smart Cities in Thailand (Sagarik et al., 2018). These include Smart Environment, Smart Mobility, Smart Living, Smart People, Smart Energy, Smart Economy, and Smart Governance.
The development of Smart Cities in Thailand is evolving towards a citizens-centric approach, where design thinking is used to understand citizens’ challenges better, solve complex problems, and prioritise city projects. From DEPA’s perspective, cities can apply the development of one or more new projects that will become part of their journey towards a Smart City. The basic requirement is that the project be within one of the seven topic areas.
Thailand is working to transform its economy from an industry-driven one to a high-tech, value-based economy through initiatives such as Thailand 4.0 and the Digital Thailand plan launched in 2016. The aim is to pull Thailand out of the “middle-income trap” by transitioning production towards innovative products, driving industry with technology/creativity, and shifting focus from producing products to providing services (Sagarik et al., 2018). As part of the Thailand 4.0 strategy for a technology-driven economy, the government aims to build a “Digital Economy and Society” to become a digital leader in ASEAN. Key targets include placing Thailand in the top 40 globally for the ICT Development Index and the top 15 for the World Competitiveness Index. The Digital Development Plan for Economy and Digital Society outlines goals like establishing digital infrastructure, using technology to improve lives, promoting innovative digital businesses, building digital workforce skills, increasing GDP from digital industries, and enhancing ICT readiness based on global indices.
The plan involves a 20-year roadmap divided into four phases, to be achieved via six strategies: building high-capacity digital infrastructure nationwide, boosting the economy with digital tech, creating a knowledge-driven digital society, transforming into a digital government, developing a digital workforce, and building trust in digital technology (Sagarik et al., 2018).
Five key pillars underpin the digital economy drive: developing complex infrastructure like broadband and wireless networks; creating service infrastructure for e-government services; building soft infrastructure like cybersecurity, laws and verification systems; promoting the digital economy through entrepreneurship and innovation; and fostering a digital society with affordable access and enhanced quality of life (Heeks and Bukht, 2018).
This study aims to understand how inclusive Smart City operation leaders can use data in their planning and execution process; this will be evaluated with respect to Thailand’s Smart City operations strategies.
This will result in a data landscape which will illustrate how data can be leveraged to provide a supporting framework for decision-makers when operating city services.
This article primarily targets public sector agencies and academics who want to understand the current data landscape in Thailand 4.0. However, with the evolving development of Smart Cities in Thailand, this information will also be valuable for the private sector, enabling it to leverage existing data sources and contribute innovative solutions.
This article is structured into a methodology section after the introduction, a literature review, and a discussion section, ending with a conclusion.
This literature review examines citizen-centric Smart City frameworks and enumerates the various data types utilised in cities across Thailand. Following this analysis, the review discusses green initiatives implemented in urban areas and assesses the readiness and utilisation of data strategies in Thailand.
City 5.0 has been proposed as a design paradigm for creating liveable cities that eliminate citizens’ restrictions through digitalisation to provide public goods and services (Becker et al., 2023). The core of this concept lies in the idea of “restrictions”—a combination of constraints, specific citizen groups affected, and liveability aspects that matter to those groups (Becker et al., 2023). By understanding and managing these restrictions, City 5.0 aims to foster wider citizen involvement in the governance and design of future cities.
A key principle of City 5.0 is identifying and addressing restrictions citizens face in accessing public goods and services before implementing technology-enabled city solutions (Becker et al., 2023). This aligns the efforts of public administrations, private organisations, and researchers around eliminating such barriers to improve citizens’ quality of life and enable their active participation in shaping future cities (Becker et al., 2023). City 5.0 misses elements related to citizen-generated data in shaping city policies or services. Leveraging citizen-generated data can provide insights into behavior patterns, preferences, and specific community needs, which is essential for effectively tailoring services. With IoT data (Becker et al., 2023), mention technological solutions like chatbots and telemedical services but no concrete discussion of how big data and IoT devices are integrated to provide comprehensive city services.
Citizen-centricity has been a longstanding objective for governments, particularly in Smart City development (Berntzen et al., 2016). However, public services are often still designed from the government entity’s perspective, following the logic of internal operations and processes rather than the needs and experiences of citizens (Berntzen et al., 2016). This disconnect between government and citizen perspectives can significantly hinder achieving citizen-centric Smart Cities (Berntzen et al., 2016). Several case examples illustrate the consequences of this failure, such as the added burden placed on cancer patients to navigate complex public health systems and the disempowerment of citizens with disabilities due to inflexible service models (Berntzen et al., 2016).
While the potential of citizen-centric Smart City processes is clear, achieving this in practice is more complex (Berntzen et al., 2016). The paper (Berntzen et al., 2016) missed some evaluation of technology and data related to citizen-centric solutions. Still, then this paper did justify the importance of early, 2016, which may have been early when comparing technology solutions in cities today, 2024. Organisational cultures and identities within government can constrain the ability to prioritise the citizen perspective (Baxter et al., 2023).
To truly realise citizen-centric Smart Cities, a more holistic understanding of citizenship is required—one that encompasses rights, governance practices, and citizen responsibilities (Malek et al., 2021). Identifying clear social inclusion indicators can help governments and communities measure progress towards this ideal and address the shortcomings and contradictions that often arise in attempts to balance corporate interests, technological solutions, and meaningful citizen participation (Malek et al., 2021).
In the rapidly evolving landscape of urban development, the concept of Smart Cities has emerged as a transformative paradigm, placing data at the forefront of city planning and operations. As cities strive to address the challenges of population growth, resource scarcity, and environmental sustainability, the strategic utilisation of data has become essential in shaping the future of these metropolitan hubs (Kourtit et al., 2017).
The proliferation of big data and advanced analytics has profoundly impacted how cities are envisioned, planned, and managed. The vast array of data sources, including sensor networks, social media, and IoT (Internet of Things) devices, have enabled city planners and policymakers to gain unprecedented insights into the complex dynamics of urban environments (Hashem et al., 2016).
By harnessing the power of data-driven decision-making, cities can optimise infrastructure, improve resource allocation, enhance public services, and ultimately foster more livable, sustainable, and resilient communities. The cornerstone of this data-driven approach to Smart City planning and operations is the ability to collect, integrate, and analyse large volumes of diverse data. This data can be leveraged to identify patterns, predict trends, and develop targeted interventions, empowering city leaders to make more informed, evidence-based decisions (Batty, 2013).
For instance, transportation planning can be improved by integrating real-time data from traffic sensors, public transit systems, and ride-sharing platforms. By analysing this data, cities can optimise traffic flows, reduce congestion, and enhance multi-modal connectivity, improving residents’ commuting experience and reducing transportation’s environmental impact (Silva et al., 2018).
Similarly, the application of data analytics in urban planning can facilitate the creation of more sustainable and livable cities. Using Geographic Information Systems (GIS) and predictive modelling, city planners can assess the potential impact of new developments, evaluate the trade-offs between economic growth and environmental preservation, and make informed decisions that prioritise the community’s well-being (Kaluarachchi, 2022).
Integrating sensor technology and big data analytics has revolutionised how cities manage resources, such as water and energy. By monitoring real-time consumption patterns and identifying areas of inefficiency, cities can optimise the distribution and utilisation of these critical resources, leading to significant cost savings and environmental benefits. Furthermore, applying data-driven techniques in public safety has yielded remarkable results. By analysing crime data, incident reports, and surveillance footage, cities can identify high-risk areas, predict the likelihood of criminal activity, and deploy resources more effectively to enhance the security and well-being of their communities (Barns, 2018).
The role of data in Smart City planning and operations extends beyond optimising infrastructure and service delivery. It has also become a driving force in fostering citizen engagement and improving urban residents’ overall quality of life. By integrating data-driven platforms and interactive dashboards, cities can empower citizens to participate actively in decision-making, provide real-time feedback, and develop tailored solutions that address their unique needs (Walentek, 2021).
This participatory approach to Smart City planning enhances transparency and accountability and cultivates a sense of community ownership, where citizens feel invested in their city’s success. Moreover, the availability of granular data and real-time information has enabled cities to be more responsive to their residents’ changing needs and preferences, allowing for the rapid implementation of initiatives that cater to the evolving landscape of urban communities (Rathore et al., 2018).
However, successfully integrating data in Smart City planning and operations is challenging. The sheer volume and complexity of data generated by modern cities can be overwhelming, and the effective management, storage, and analysis of this information requires significant technological infrastructure and expertise. Data privacy, security, and ethical use issues must be carefully navigated to protect citizen information and maintain public trust (Sta, 2017).
Despite these challenges, the benefits of data-driven Smart City planning and operations are clear, and cities worldwide increasingly embrace this transformative approach. By harnessing the power of data, city leaders can make more informed, evidence-based decisions, optimise resource allocation, enhance public services, and ultimately create vibrant, sustainable, and livable communities that meet the evolving needs of their residents (Fang et al., 2021).
As the field of data-driven, smart, sustainable urbanism continues to evolve, it is essential to recognise its underlying interdisciplinary and transdisciplinary nature. The successful implementation of data-driven Smart City initiatives requires integrating knowledge and expertise from various academic and scientific disciplines, including urban planning, computer science, environmental science, social sciences, and public policy. This multifaceted approach ensures that the complex challenges facing modern cities are addressed holistically, focusing on the three dimensions of sustainability: economic, environmental, and social (Bokolo, 2023).
This data-driven approach has enabled cities to optimise critical services and infrastructure delivery. For instance, the analysis of real-time traffic data can help cities improve the efficiency of their transportation systems, reducing congestion and enhancing the commuting experience for residents. Similarly, using Geographic Information Systems and predictive modelling can support urban planners in evaluating the potential impact of new developments, balancing economic growth and environmental preservation, and creating more sustainable and livable communities (Chaturvedi et al., 2018).
Integrating sensor technology and big data analytics has also revolutionised how cities manage their resources, such as water and energy. By monitoring real-time consumption patterns and identifying areas of inefficiency, cities can optimise the distribution and utilisation of these critical resources, leading to significant cost savings and environmental benefits. Moreover, the application of data-driven techniques in public safety has yielded remarkable results, enabling cities to predict the likelihood of criminal activity and deploy resources more effectively to enhance the security and well-being of their communities (Radziszewska, 2023).
Smart Cities generate vast amounts of data from various sources (Nimmanphatcharin et al., 2020), which can be leveraged to improve urban planning, service delivery, and decision-making. Some of the data produced includes:
Smart Environment Data, such as air quality, water quality, noise levels, temperature, humidity, and waste management in real-time (Djordjevic and Dankovic, 2019). For example, a weather station collects and analyses temperature, humidity, and wind speed data. Real-time weather data can issue alerts for extreme weather events like storms or heat waves, allowing people to take precautions and minimise risks (Jantakat et al., 2018). The Sphere platform (sphere.gistda.or.th) (Geo-Informatics and Space Technology Development Agency, 2024) is managed by Thailand’s Geo-Informatics and Space Technology Development Agency (GISTDA), offers access to satellite data that provides significant advantages for Smart City planning and management.
A review of data sources listed in Tables 1–7 suggests that while these datasets are marked as “used in city operation,” their primary application may be for reporting purposes. This is evident in examples like weather data utilised for flood forecasting research (Chitwatkulsiri et al., 2022), water volume data employed in irrigation system performance assessments (Mainuddin et al., 2000), and renewable energy data informing low-carbon city development policy (Phdungsilp, 2010). Similarly, pollution data finds application in waste management sustainability evaluations (Chanhthamixay et al., 2017) and health studies (Pongpiachan and Paowa, 2015), while water resource data serves broader water management needs (Nikomborirak and Ruenthip, 2013). While the Open Government Data portal offers valuable resources for city operations (Kittima, 2016), further investigation reveals the use of these datasets for research on road accidents (Tippayanate et al., 2024), tourist transportation emissions (Jamnongchob et al., 2017), and pollution management (Pinyochatchinda, 2014). Notably, CCTV data in Bangkok prioritises security monitoring (Trimek, 2016). This analysis highlights the need to explore how these datasets can be more effectively utilised for direct city operations while recognising their continued importance for research and informing future initiatives like technology-driven human development projects (Kamnuansilpa et al., 2020).
Smart Governance Data focuses on the digital transformation of governmental processes and services. This includes digitising administrative tasks, automating workflows, and adopting e-governance platforms for citizen interactions. Traditional administrative data from city departments, such as property records, permit applications, and service requests, provides important context on the city’s built environment, resource usage, and service delivery. Integrating this data with other sources can lead to more holistic and evidence-based decision-making (Jinda and Manmart, 2013; Srimuang et al., 2017). For example, data.go.th is akin to a central library of city information.1 Platforms like api.data.go.th in Thailand offer user-friendly access to a comprehensive data catalogue and open data portal. By making this information readily available, cities empower citizens and businesses to develop data-driven solutions that address urban challenges and improve quality of life. Today, only a small scale of the data available is used through a smart and innovative process. An example of innovative data usage comes from using Traffy Fondue and My City platforms.
Smart Mobility Data integrates advanced technologies and data-driven solutions to optimise transportation systems within a Smart City context. It focuses on improving accessibility, safety, efficiency, and sustainability of urban transportation networks. For example, sensors that collect real-time data on traffic flows can provide granular insights into the city’s pulse and enable data-driven management of urban systems. Table 3 shows smart mobility data used in Thailand.
Smart Energy Data refers to applying advanced technologies and innovative solutions to optimise energy generation, distribution, consumption, and management within a Smart City framework. It aims to enhance energy efficiency, promote renewable energy sources, reduce carbon emissions, and improve overall energy sustainability.
Smart Economy Data focuses on digitalising industries, businesses, and public services. It leverages AI, IoT, blockchain, big data analytics, and cloud computing technologies to drive innovation and efficiency across economic sectors. Smart Economy initiatives aim to leverage digital technologies and innovation to transform traditional economic models, drive sustainable growth, and improve the quality of life in Smart Cities. For example, delivery platforms can serve as digital marketplaces for local businesses, giving them access to a larger customer base without needing physical storefronts. Moreover, factory integration with smart inventory management systems helps companies to keep track of stock levels in real-time, reducing overstock and stockouts and ensuring timely replenishment.
Smart Living Data focuses on leveraging technology and innovative solutions to enhance personal living management, improve access to healthcare services, and promote overall well-being within a Smart City context. Data from health monitoring devices and apps are analysed using AI and data analytics to generate personalised health insights and recommendations. This empowers individuals to make informed decisions about diet, exercise, medication adherence, and lifestyle modifications. For example, Wristbands for health checking, also known as fitness trackers or health bands, are wearable devices that monitor various health metrics. These bands typically use built-in sensors to track basic information like heart rate, steps taken, and calories burned. More advanced models can measure additional metrics like sleep quality, blood oxygen levels, and skin temperature. The data collected by the wristband is usually synced with a smartphone app, allowing users to view trends over time, set goals, and gain insights into their overall health and fitness (Wu, 2020).
Smart People Data focuses on improving their quality of life, engaging in collaborative problem-solving, and contributing to the sustainable development of their city. Smart People cities prioritise education and training to equip residents with the necessary skills and knowledge to thrive in the digital age. This includes promoting STEM education, digital literacy programs, and lifelong learning opportunities. All citizens have access to the tools and technologies they need to participate fully in the economy. For example, a percentage of the population has internet and digital devices. Many startups and small businesses, particularly those leveraging technology (Gupta et al., 2017).
Thailand mostly uses data collection to generate reports, which can be greatly improved with innovative data usage.
For example, IoT-enabled air pollution monitoring systems, such as those discussed in Kanabkaew et al. (2019), gather real-time air quality data, including PM2.5 concentrations. Kanabkaew et al. (2019) also highlight that the data is primarily used to provide insights rather than drive immediate action. This is also indicated in Table 1, as the PM2.5 is presented as a website, but air quality is available as JSON data. Access to JSON data through an API could offer opportunities to collect real-time data and develop predictive models (Kanabkaew et al., 2019).
Data collected from the water management systems from smart technologies and IoT devices are currently not used to proactively address issues such as leakage or abnormal usage. The case study by Subha and Kowsigan (2022) illustrates that although smart water distribution systems have the potential to optimise water usage through real-time sensor data, actual usage has often been limited to monitoring purposes instead of creating a closed-loop system for rapid response to changes in the environment (Subha and Kowsigan, 2022). Similarly, in Thailand, the data from the Water resource website, see Table 1 does not show signs of providing API or real-time data that can be used to automate.
In other sectors, drone-based air quality monitoring in hard-to-reach areas shows similar tendencies. The data collected by drones in the study by Duangsuwan and Jamjareekulgarn (2020) helps to visualise pollutant levels in real-time. Still, again, this data is mainly used for generating visual reports rather than for automating the decision-making process to mitigate pollution sources (Duangsuwan and Jamjareekulgarn, 2020).
When it comes to disaster response systems, for example, for flooding, the deployment of sensor networks has been highlighted by Chien et al. (2013), where satellites and ground sensors are used to monitor flooding in real-time (Chien et al., 2013). However, the usage of citizens-generated data, for example, from the Traffy Fondue platform, Table 2 shows progress on proactive warning systems, where data is used to address and fix flood incidents.
Jitkajornwanich et al. (2018) describe the usage of social media and crowdsourcing for early flood warnings. The project demonstrated using Twitter data to provide early warning for floods, employing natural language processing and geolocation to pinpoint areas at risk. Despite the success in using crowdsourced data, the implementation stopped at the reporting phase rather than integrating the findings into emergency response protocols to improve response times (Jitkajornwanich et al., 2018).
The need for more understanding and designing efficient data integration across sectors also creates barriers to achieving proactive data usage. This was described by Nanthaamornphong et al. (2020) in their study on the Phuket City Data Platform. The Phuket City Data Platform had numerous data sources from various sectors, such as tourism, safety, and environmental monitoring. Unfortunately, the data was not used in further innovative actions or projects to develop proactive or automated response systems (Nanthaamornphong et al., 2020).
Table 8 discusses the findings; the rapid technological advancements and exponential growth in Southeast Asia have created an increasing demand for a highly skilled labour force (ASEAN Secretariat, 2021; World Economic Forum, 2020; Jobs on the Rise in 2021: Southeast Asia Report, 2021). As more organisations and systems move online, the need for specialised engineers and data-driven strategies has become crucial. This demand is driven by the widespread adoption of new technologies such as 5G, IoT, and other Industry 4.0 innovations, which require a robust pool of technical talent to support implementation and management (Commonwealth Secretariat, 2022).
However, the region faces unique challenges in talent management, with a mismatch between the skills taught in educational institutions and the requirements of industries (Almeida, 2009). This skills gap has resulted in a shortage of qualified workers, threatening to impede the region’s economic transition (Coxhead, 2014). For instance, countries like Malaysia and Thailand have struggled with technological upgrading in their industries, undermining their capacity to sustain rapid growth in sectors such as electronics manufacturing (Rasiah, 2009).
To address this issue, it is essential to understand Southeast Asian cities’ readiness to adopt and implement effective data strategies. This fosters collaboration between businesses and public bodies to advance tech skill sets within their markets (Commonwealth Secretariat, 2022). Additionally, there is a need for targeted educational reforms that align curricula with the evolving demands of the job market. Vocational education programs at the local level can play a significant role in helping national economies adjust to changes in the labour market and technological advances (Barr and Attrey, 2017).
Moreover, the region must address migration policies that impact the availability of skilled labour. Countries like Singapore, Malaysia, and Thailand rely heavily on foreign workers and have implemented migration policies to manage labour market gaps (Kaur, 2010). Effective migration management can help mitigate the challenges of skills mismatches and labour shortages.
Data strategy readiness refers to a city or organisation’s capability and maturity to effectively manage and use data to drive decision-making, support citizen and private sector innovation, and improve overall governance efficiency (Open Data Maturity Model, 2019). This concept is crucial for developing Smart Cities, which require the latest technology infrastructure, a robust data governance framework, expertise in data management, and an organisational culture that supports data-driven initiatives.
Several components must be in place for a city to achieve data strategy readiness. Firstly, an advanced technology infrastructure must include high-speed internet, widespread use of IoT devices, and cloud computing capabilities. These technologies enable the collection and processing of vast amounts of data, which can be used to optimise city operations and services (Shafiullah et al., 2023).
Secondly, a comprehensive data governance framework is essential. This framework should include policies and regulations that ensure data privacy and security and standards for data interoperability and quality. Effective data governance ensures that data can be trusted and used efficiently across different sectors and applications (Goreczky, 2022).
In addition, the role of data experts cannot be overstated. Cities need skilled professionals who can analyse data and derive actionable insights. These experts must be supported by continuous training and development programs to keep up with the latest technological advancements and data analytics techniques (Mutiarin and Lawelai, 2023). Moreover, the organisational culture must prioritise data-driven decision-making. This involves encouraging the use of data at all levels of government and ensuring that decision-makers have access to relevant data and the tools to interpret it effectively. Such a culture fosters innovation and continuous improvement in public services (Kong and Woods, 2021).
Thailand, for example, has made significant strides in developing its Smart City initiatives. The Thai government has invested in building digital infrastructure and enhancing data governance to support Smart City projects. These efforts include establishing data centres and promoting public-private partnerships to leverage data for urban development (Stephenson and Dobson, 2020). However, challenges remain in fully integrating these systems and ensuring comprehensive coverage across all regions. The current difficulties remain with limited data literacy and understanding of cross-organisational and sectoral data usage. Moreover, the local governments will need to develop a proper Smart City strategy, which includes embracing some Smart City principles, such as (i) Openness and Transparency to empower citizens to understand public operations and build trust. (ii) Open data and Open source enable the local government to share data with the citizens while enabling innovative opportunities for entrepreneurs in the city. (iii) Sustainable environment, building processes to manage waste, developing renewable energy strategies, and green environments and spaces. (iv) Satisfaction and happy citizens by creating a system that attracts businesses to invest will also develop jobs and improve the economy, providing a better life for the citizens. (v) Healthy environment, including an inclusive healthcare system, ensures that citizens strive for a healthy lifestyle.
The evolution of Smart Cities in Thailand has been significantly driven by the strategic use of data across various sectors, facilitating more inclusive and sustainable urban development. This section highlights key findings from academic research on data utilisation in Thai Smart Cities, focusing on mobile network optimisation, green energy integration, community acceptance of new technologies, and the overarching vision for sustainable and resilient urban communities.
Thailand is at the forefront of a revolution. The country actively embraces the Smart City concept, a vision for urban centres that leverage technology and data to improve residents’ lives. Several exciting initiatives are underway, all aimed at harnessing the power of information to make Thai cities more efficient, sustainable, and livable.
Behind the scenes, a network of dedicated agencies is collaborating to make this vision a reality. Digital Government Development Agency (DGA) plays a central role in spearheading the development of digital infrastructure for Thailand’s cities. This includes creating a central data catalogue and an open portal accessible through the user-friendly data.go.th platform. Think of it as a central library of city-related information readily available to fuel innovation (Thongprakob, 2018).
Another key player is the National Statistical Office (NSO). They act as the government’s data custodian, collecting and disseminating official statistics on various socio-economic aspects of Thailand. Through their gdcatalog.go.th platform, they provide a rich resource of information that’s invaluable for Smart City development (see Figure 1).
Figure 1. The seven aspects of Smart Cities development. Source: DEPA (2021).
Digital Economy Promotion Agency (DEPA) brings a different expertise to the table. Their focus is on promoting the adoption of digital technologies across Thailand. This includes developing innovative Smart City platforms and solutions to improve urban living. They share this data through the CityData.in.th platform, making it readily accessible to those working on Smart City initiatives (Nimmanphatcharin et al., 2020).
Moreover, DEPA sets the foundation for managing a city’s data by defining rules and standards as Figure 2. A data catalogue is an inventory of this data, making it easily searchable and understandable. Data exchange enables the secure sharing of this data between different city departments and organisations, facilitating collaboration and data-driven decision-making. Together, these components form a crucial framework for effective data management in Smart Cities (DEPA, 2021).
Figure 2. Key elements of DEPA’s city data platform. Source: DEPA (2021).
Finally, the Geo-Informatics and Space Technology Development Agency (GISTDA) contributes geospatial expertise (Kanchanarat et al., 2023). They provide crucial data and services, such as satellite imagery and detailed maps, accessible through their Sphere platform (sphere.gistda.or.th) (Geo-Informatics and Space Technology Development Agency, 2024). This information is essential for Smart City planning and management, allowing for a deep understanding of the city’s physical layout and infrastructure.
The agencies mentioned above exhibit a collaborative approach, fostering a comprehensive data ecosystem for Thailand’s Smart City initiatives. They are collaborating to create a comprehensive data ecosystem for Smart Cities in Thailand. Data is collected from various sources, including sensors, cameras, and CCTV footage. This information is then stored and processed in centralised platforms, ensuring its security and accessibility. The real power comes when this data is shared with relevant stakeholders. Government agencies, businesses, and citizens can access and utilise this information to make data-driven decisions and develop innovative solutions for their communities (Smitananda and Akahat, 2024).
Data is the fuel that drives Smart City development. This valuable resource is used in various ways to improve urban life in Thailand. Traffic congestion is a major concern in many cities. By analysing data from CCTV cameras, urban planners can identify areas with high traffic volumes and implement solutions to improve flow and reduce travel times. This is just one example – data is also being used to optimise energy consumption through smart meters and renewable energy integration, promote public acceptance of new technologies, and build more sustainable and resilient communities (Khianmeesuk, 2017).
Thailand’s commitment to Smart City development is a testament to the country’s forward-thinking approach. By strategically utilising data and fostering collaboration between various agencies, Thai cities are well on their way to becoming vibrant, sustainable, and livable centres for the future.
Several gaps that affect urban environment transformation have been identified from the above. These include (i) Limited integration of technology for responsive city management. (ii) Data infrastructure for monitoring and reporting needs additional implementation of automated, real-time responses to address issues such as traffic congestion, water distribution, environmental hazards and more. (iii) Inconsistent interconnectivity of data systems. Even with the existing platforms like the City Data Platform (CDP), the data sources operate in silos, which prevents comprehensive analysis and integrated solutions for city operations. The lack of seamless data interconnectivity undermines the potential of Smart Cities to improve efficiency across sectors. (iv) Public engagement also presents a gap in Smart City development. The People-Public-Private-Partnership (PPPP) model depends on cross-sector collaboration, but the involvement of citizens is still limited. Active resident participation is important to improve Smart City operations and projects. (v) Inadequate synchronisation between physical and digital infrastructures also poses challenges. Some efforts have been made to develop basic utilities and digital systems. The coordination between them seems insufficient, and without close integration between physical infrastructure and digital solutions, the full benefits of Smart City initiatives are not realised.
This study adopts a mixed-methods approach to investigate data utilisation in Smart City operations within Thailand. The research aims to explore the role of sensor-generated data and open government data in supporting inclusive urban planning and efficient city management. The methodology includes qualitative and quantitative elements to address the research questions comprehensively.
Some advantages of Smart City initiatives are derived from innovation and data usage. This study analyses open government data sources provided by local governments in Thailand. The sources include data from traffic, environmental, and utility services. A systematic review of open data platforms and repositories will help understand how these datasets support Smart City innovation and, in some cases, leverage citizen engagement. Additionally, publicly available documentation and reports from Smart City projects will provide context and qualitative insights into data-driven decision-making. Refer to Tables 1–7 for a list of data sources.
To address the research questions, we will utilise a comprehensive data collection method. This involves desk research through a systematic review of literature, data sources, open government data, and associated documentation and reports to identify the types of data generated, their sources, and their current and potential uses in city planning and operations. Additionally, reports and documents published by government bodies, city authorities, and Smart City project teams will be examined to understand the role of data in driving smart urban initiatives and decision-making processes.
The research aims to answer the following questions:
Main research question: How can inclusive Smart City leaders leverage data in their planning and operational processes to enhance city services and citizen well-being?
Sub-question 1: What types of data are currently being produced by cities?
Sub-question 2: How are cities planning and developing data strategies, and in what ways do these strategies benefit both the city and its citizens?
Sub-question 3: How does the overall data landscape align with city operations and planning activities?
The conceptual framework presented in Figure 3 was developed to align with the data landscape in Thailand, ensuring that it reflects the local context and challenges. This framework defines the scope of the study by outlining the specific dimensions of the data landscape pertinent to Thailand’s urban management and Smart City initiatives. By identifying these key areas, the framework enables the research to focus on the factors influencing the successful implementation of data-driven strategies (see Figures 4, 5).
Figure 4. Data management framework recreated by authors. Source: DAMA International (2017).
Additionally, the conceptual framework serves as the foundation for the entire research process, guiding the formulation of the study’s objectives, research questions, and hypotheses. It establishes a structure for exploring how data and strategy can contribute to Smart City operations, ensuring that all research activities align with the study’s goals. This framework provides a comprehensive understanding of the interplay between data and strategy and the data contribution to urban innovation. Framing the research within this structure ensures that the study addresses the complexities of Smart City initiatives, including data generation, analysis, and practical applications in urban governance. The conceptual framework also systematically examines how data-driven strategies can be leveraged to enhance citizen engagement, optimise resource allocation, and improve overall quality of life.
The literature review begins by exploring what is already known about the constructs, variables, and relationships in the data landscape. This includes data generation, collection, and usage, described from previous research findings on data landscapes, open data, and data-driven decision-making. The aim is to understand the current state and identify potential areas for improvement and innovation. Some services involve citizens, which allows assessing how citizens contribute to the data landscape, such as through social media, mobile app usage, and community-driven data collection. This will also determine the impact of citizen-generated data on policy-making and social services.
To complement the literature review, other data sources in Thailand, including official statistics, open data portals, and non-traditional data sources, will be analysed to understand the country’s data landscape.
The study will investigate the methods and protocols for data generation and explore current uses of data in Thailand, highlighting innovative practices and successful case studies. It will also identify gaps and challenges in effectively utilising data sources for various purposes.
The outcome will suggest frameworks for innovative solutions towards efficient Smart City strategies and projects.
Implementing Smart City initiatives in Thailand demonstrates significant strides in leveraging technology to address urban challenges. These initiatives, which span various domains such as living, environment, people, mobility, economy, energy, and governance, illustrate the transformative potential of sensor data and smart technologies. However, the journey towards a fully functional Smart City is challenging in terms of data collection, analysis, and utilisation.
This section gathers data and insights from the literature, reports, and open data sources. The section is structured to show the results from each of the research questions in this paper, starting with “Type of data currently being produced by cities,” and “How is the data landscape for Smart City projects,” then “How data strategies are planned for the benefit of the city and the citizens”.
To bring insights from the findings for the sub-research question 1: “What types of data are currently being produced by cities?” we evaluated their capability to provide data via a programmable interface (API). The following Table contains the list of valuable data sources that can be used to implement innovative and responsive city solutions.
Compared with many data sources, the listed sources in Table 9 are the only sources currently fit to integrate with Smart City innovative solutions. They may need minor updates to their current data format, security and server for performance. Still, at their current state, it is possible to develop innovative solutions that can retrieve and use data from these sources. The rest of the identified data sources in Tables 1–7 are not designed to be used with Smart City solutions. These sources must develop an API interface to provide the data from their databases for better usage with innovative solutions.
As in many global Smart Cities, project initiatives have been executed in collaboration with the private sector and academia. For example, The Healthcare Wristband for Elderly Project monitors vital health parameters such as heart rate, blood pressure, and oxygen levels, providing real-time insights to healthcare providers. This data facilitates early detection of health risks and timely interventions, promoting independent living among senior citizens. The wristband’s fall detection feature triggers emergency alerts, ensuring prompt assistance, and personalised healthcare plans tailored to individual needs improve the quality of life for elderly citizens. Such projects are often smaller and defined as Proof of Concepts (PoC). In the context of Smart City initiatives, PoC delivers potential results to scale up and deploy solutions in the city. Other projects tackle environmental challenges affecting public health, ecosystems, and economic well-being, including air and water pollution, climate change, and waste management. Addressing these requires technology adoption, policy interventions, and behavioral change initiatives. Developing real-time monitoring systems for air quality, noise, and water pollution is crucial. The Weather Station Project, which installs air quality sensors to collect data, analyse relationships with various factors, and present results in an understandable format, exemplifies efforts to tackle these challenges. With the educational gap across Thailand, the larger cities face the challenge of bridging the digital divide, developing essential life skills, fostering citizen engagement, and enhancing financial literacy. Expanding access to digital infrastructure and providing digital skills training are crucial. Educational programs and community-based initiatives can foster these skills and empower individuals. Notably, the “Digital Skills Development for Unemployed and At-Risk Workers” and the “Future Digital Skills Enhancement for a Better Quality of Life for All Thais” projects aim to develop an online platform that provides free access to educational resources.
The analysis of Thailand’s Smart City initiatives underscores the pivotal role of data in enhancing urban operations across various domains. Sensor data from projects like the Rain Gauge Application and Healthcare Wristband (Leesa-nguansuk, 2016) for the Elderly have improved weather prediction, agricultural planning, healthcare monitoring, and emergency response (Idris et al., 2022). These applications demonstrate how real-time data can drive timely interventions and personalised services, enhancing citizens’ quality of life (Chokphukhiao et al., 2020). Efforts to bridge the digital divide and improve digital literacy through projects like Digital Skills Development have empowered citizens to participate actively in the digital economy.
Another example is the CCTV Traffic Counting Project, an intelligent system that uses data to manage traffic flow, reduce congestion, and enhance road safety (Choosakun et al., 2021). These initiatives highlight the efficiency gains from data-driven traffic management and promote sustainable mobility options. The collection and analysis of business data, such as restaurant transaction data, support SMEs by providing insights into consumer behavior and improving business performance. Moreover, data-driven strategies are essential for economic growth and attracting foreign investment.
Adopting Enterprise Resource Planning (ERP) systems and City Data Platforms (CDPs) has streamlined municipal management, improved decision-making processes, and enhanced transparency. However, challenges regarding policy mandates, data sharing, and adopting these technologies still need to be addressed.
To illustrate the data landscape, sub-question 3, “How does the overall data landscape align with city operations and planning activities?” provides an example of contributing to Smart City projects. The Traffy Fondue, is a platform that allows citizens to report urban issues such as infrastructure damage, waste management problems, and other public service concerns. As soon as a report is registered in Traffy Fondue, it is automatically sent to the relevant municipal agency for action. This creates a direct line of communication between the citizens and the city officials, enabling quicker response times. What sets Traffy Fondue apart is its real-time tracking feature, allowing citizens to follow their complaints’ status from submission to resolution. This system encourages public trust and participation in city governance by enabling transparency and accountability.
From Table 2, the Traffy Fondue platform is currently the only platform offering open data access and having a well-integrated solution for city operations (Traffy Fondue, 2022). The platform has been implemented in 77 provinces and used by over 15,000. Since 2022, more than 940,000 complaints have been recorded, and over 733,000 cases have been resolved with a 77% success rate (Rojanasuvan, 2024).
The Bangkok Metropolitan Administration has been pushing the usage of Traffy Fondue, which the citizens have adopted. They have taken to report complaints, mostly about road issues, traffic, flooding, waste, footpaths, street lights, and safety. The platform continues to evolve so that citizens can reach out to agencies via the platform and submit reports (see Tables 10, 11).
Table 10. Traffy Fondue data snapshot on October 14, 2024 from Bangkok (Traffy Fondue, 2024).
Table 11. Traffy Fondue statistics across Thailand (Traffy Fondue, 2024).
With open data access, the Traffy Fondue platform empowers citizens to engage and use their skills to innovate with new ideas and solutions. Traffy Fondue improves the speed of issue resolution and provides valuable data for city planners. By analysing complaint data over time, city officials can identify recurring issues, such as frequent road damage in a particular area or persistent waste collection failures, and make strategic decisions to address these root causes. This real-time, data-driven approach ensures that urban management is responsive and adaptive to the community’s needs, critical for fostering an inclusive and sustainable Smart City environment.
The second sub-research question, “How are cities planning and developing data strategies, and in what ways do these strategies benefit both the city and its citizens?” dives into city management and strategies. These often depend on policies and larger projects, as well as public or semi-public services, which support the backbone of the city infrastructure.
In Thailand, transitioning to renewable energy sources like solar, wind, and responsibly utilised hydropower is crucial. Enhancing energy efficiency through demand-side management, energy-efficient appliances, and stricter building codes is essential. Developing smart grid infrastructure with real-time monitoring, energy storage, and demand response programs will optimise energy use. Strengthening policies with renewable energy incentives, carbon pricing mechanisms, and streamlined permitting processes will accelerate the adoption of these solutions.
Smart Governance advocates adopting Enterprise Resource Planning (ERP) systems to streamline municipal management. Odoo, a prominent ERP system, enables efficient data collection and administration related to organisational structures, documents, financial records, inventory, and resources within the public sector. This integration enhances operational efficiency, data accuracy, accessibility, and decision-making processes, empowering public sector agencies to operate more effectively. City Data Platforms (CDPs) have emerged as critical tools for local governments to enhance decision-making, improve service delivery, and promote transparency. The lack of understanding of CDP guidelines and inadequate tools and software suggests a need for comprehensive training and capacity-building programs. Promoting data-sharing practices and addressing budget constraints are also essential for CDP implementation.
Senior management’s absence of a policy mandate is a significant barrier to CDP adoption. This underscores the importance of advocating for CDP at the highest levels of local government, emphasising its potential to enhance governance and service delivery.
City-data platforms (CDPs) hold immense potential to transform local governance in Thailand. However, this study reveals a significant gap between CDP potential and current adoption. Addressing the identified challenges through capacity building, policy support, and data sharing initiatives is crucial for realising CDPs’ transformative power in Thai local governments.
Public awareness campaigns can highlight the benefits of Smart City technologies, encouraging broader participation and acceptance. Community-based initiatives, such as the Digital Skills Development projects, empower individuals by enhancing their digital literacy and life skills, enabling them to fully participate in the digital economy.
Because the landscape of city operations increasingly relies on real-time, citizen-generated data to inform decision-making and resource allocation, cities that map various data sources into data operation platforms like Traffy Fondue provide granular insights into urban challenges. Data from diverse sources should be consolidated into a central hub to facilitate management and provide a comprehensive overview. This centralised approach enables urban leaders to gain a holistic perspective of challenges, thus enhancing their ability to conduct comprehensive analyses. These data-driven approaches enable cities to prioritise issues based on community needs, optimise service delivery, and measure performance effectively. For instance, studies have shown that cities using similar platforms experienced reduced response times to citizen complaints and improved overall satisfaction. While specific survey data might be required for a comprehensive analysis, the literature consistently highlights the positive correlation between citizens. Consolidating data from various sources into a central hub is essential for effective city management. Using data integration techniques, cities can create a comprehensive overview and utilise data visualisation to identify trends and patterns. This empowers urban leaders to make informed decisions, optimise resource allocation, and enhance overall city performance.
The results from data literacy and management, together with the consolidated findings from the sub-research questions, bring insights to the main research question: “How can inclusive Smart City leaders leverage data in their planning and operational processes to enhance city services and citizen well-being?”
To ensure practical data strategy readiness, the DAMA framework (DAMA International, 2017) is a best practice framework for managing data across an organisation, including governmental bodies and the public sector. This framework is one solution and approach to mitigate gaps listed in the above chapter on data strategy readiness; it highlights key data management knowledge areas critical for bridging the gap and supporting better use of data in Smart City initiatives.
Data Governance definition: Policies, procedures and standards to manage data effectively and consistently across the organisation.
DAMA framework areas:
1. Data architecture: design and maintain the data infrastructure to support data integration, quality, and accessibility.
2. Data modeling and design: definition of data structures and relationships to support business processes and objectives.
3. Data storage and operations: efficient and secure data storage that supports data availability and performance.
4. Data security: protection from unauthorised access, disclosure or misuse while ensuring compliance with applicable regulations.
5. Data integration and interoperability: combine data from disparate sources to enable the exchange and use of data across different systems.
6. Document and content management: control unstructured data such as documents and media content to ensure accessibility, accuracy and compliance.
7. Data warehousing and business intelligence: storage, analysis and presentation of data to support informed decision-making.
8. Metadata management: capture, storage and control of information about data such as data lineage, definitions and classifications.
9. Data quality management: assurance of data accuracy, completeness, timeliness and consistency with business requirements.
10. Reference and master data: manage the “single source of truth” (e.g., key concepts within the government like citizens, addresses, employees) shared across the organization to ensure consistent, accurate, and authoritative data across all systems.
Advanced data analytics, artificial intelligence (AI), and machine learning (ML) are critical in processing and deriving insights from large data sets. For instance, Intelligent Transportation Systems (ITS) can dynamically manage traffic signals and provide real-time traffic information, significantly improving urban mobility. Investing in Internet of Things (IoT) networks for real-time data collection and monitoring will also enhance the efficacy of Smart City projects. Data governance is defined as the exercise of authority and control over the management of data assets (Gils, 2020; DAMA International, 2017). It involves creating and enforcing policies, procedures, and standards to ensure data quality, security, and compliance with regulatory requirements. Emphasis is also placed on the importance of technical expertise, data literacy, domain-specific knowledge, data stewardship, and soft skills in data professionals for effective governance.
Some of the best practices in governmental data strategies include the United Nations’ Data Strategy, a comprehensive data governance approach (UN Secretary-General’s Data Strategy, 2020). Data management frameworks provide a structured approach to managing data assets for value and associated risks, such as regulation and security. A good data framework for a Smart City encompasses data architecture, modelling, infrastructure, security, integration, content management, and master and metadata management (Gils, 2020).
This study shows that cities worldwide continue to embrace smart technologies, and the concept of inclusive Smart City operations has gained significant traction. In Thailand, integrating data-driven strategies in Smart City planning and execution is critical to addressing urban challenges and improving the quality of life for all citizens. Inclusive Smart City leaders can leverage data to enhance decision-making, optimise resource allocation, and foster community engagement. This article explores how Thailand’s inclusive Smart City operation leaders can effectively use data in their planning and execution processes.
Thailand’s Smart City initiatives focus on seven key areas: Smart Environment, Smart Mobility, Smart Living, Smart People, Smart Energy, Smart Economy, and Smart Governance. This holistic framework aims to transform the economy through high-tech, value-based development and ensure that all citizen groups are included. The development of these areas relies heavily on the collection and analysis of diverse data types.
Data plays a pivotal role in Smart City planning and operations. For instance, transportation planning can be improved by integrating real-time data from traffic sensors, public transit systems, and ride-sharing platforms. This enables cities to optimise traffic flows, reduce congestion, and enhance multi-modal connectivity.
Implementing real-time monitoring systems for air quality, water quality, and other environmental parameters is crucial to the success of Smart City initiatives. These systems enable city officials to make timely, informed decisions that improve urban sustainability and resilience. For example, the Sphere platform, managed by Thailand’s Geo-Informatics and Space Technology Development Agency (GISTDA), provides access to satellite data that significantly enhances Smart City planning and management. By monitoring environmental conditions, such as air quality and disaster responses, the platform enables authorities to respond quickly to emerging issues, reducing public health and property risks.
When looking into Thailand’s Smart City initiatives, it becomes clear that data plays a pivotal role in enhancing city operations. Data-driven strategies enable real-time monitoring and management of urban infrastructure, optimising resource allocation and improving service delivery. Solutions like Traffy Fondue, which facilitate the real-time reporting of urban issues, lead to quicker identification and resolution, thereby enhancing operational efficiency. This understanding of the role of data in city operations is crucial for informed decision-making in Smart City development.
These research findings highlight the need to upskill staff within local governments and implement robust data management strategies and processes to support Smart City initiatives effectively. Upskilling is crucial to ensure that municipal staff can handle digital tools and make informed decisions based on the data insights available. Implementing proper data management strategies, such as adopting standardised frameworks like the DAMA framework, will help streamline data governance, integration, and utilisation, driving better decision-making and operational efficiency.
Moreover, empowering citizens with learning resources is essential to help them understand the significance of data, contribute to city operations, and actively engage in improving their communities. Providing citizens with accessible training and learning opportunities can enhance their digital literacy, enabling them to play a role in the city’s data-driven initiatives, thereby ensuring a better quality of life.
Implementing these changes requires a carefully planned and phased approach to data usage in city operations. Where the first stage of data collection and basic integration includes training and data literacy, with a second stage defining visions and strategies, which include citizens and communities. A third stage offer PoC and community centered test-beds, where entrepreneurs can also bid for trials. A fouth stage brings on a PPP approach to implement PoC into production in the city, and a stage five have the fully integrated data flow and management strategies deployed acorss the city services and with private sector involved. This phased approach provides reassurance and a clear roadmap for transitioning to a data-driven Smart City.
While Thailand’s experience with Smart City solutions like Traffy Fondue is promising, further research is needed on how advanced data analytics can be more effectively integrated into city operations and strategic planning. Future studies should focus on applying data analytics in urban management, exploring how AI and machine learning can contribute to predictive maintenance, optimised resource management, and enhanced public service delivery. Additionally, piloting new technologies in diverse urban contexts can offer insights into how scalable solutions can be adapted to different cities globally. This will not only maximise the impact of Smart City initiatives but also provide valuable knowledge for broader implementation across various regions.
Supportive policies and funding mechanisms will continue to drive innovation and the successful implementation of Smart City technologies. Incentives for renewable energy, sustainable mobility, and digital literacy programs can accelerate progress. Sharing best practices and lessons learned from Thailand’s Smart City projects will contribute to global efforts, and collaboration with international partners will help Thai cities stay at the forefront of smart urban development.
One key limitation of this study is that the data was sourced from theoretical models and simulations rather than real-world implementations. While these models provide valuable insights into how data-driven strategies could enhance Smart City operations, they need to account for practical challenges that might arise in actual deployments. Specifically, data availability, quality, and integration issues were not addressed in the theoretical framework. These challenges significantly impact the success of data-driven strategies in real-world scenarios.
Furthermore, the findings could serve as a model for cities worldwide. However, urban environments and Smart City initiatives can vary significantly across geographical, economic, and cultural contexts. The specific conditions in Thailand may not directly translate to other cities, especially those in different regions or with varying technological infrastructure and governance levels. Generalising these findings beyond Thailand requires careful consideration of local contexts, and future research should explore how these strategies can be adapted to different urban environments. Addressing these limitations is crucial for ensuring that the proposed methods are theoretically sound and practically viable across diverse settings.
Ethical review and approval was not required for the study on human participants in accordance with the local legislation and institutional requirements. Written informed consent from the patients/participants OR patients/participants legal guardian/next of kin was not required to participate in this study in accordance with the national legislation and the institutional requirements.
MH: Conceptualization, Formal analysis, Funding acquisition, Investigation, Methodology, Project administration, Resources, Validation, Writing – original draft, Writing – review & editing. KK: Conceptualization, Data curation, Formal analysis, Funding acquisition, Investigation, Methodology, Project administration, Resources, Supervision, Validation, Writing – original draft, Writing – review & editing. VK: Writing – review & editing.
The author(s) declare that no financial support was received for the research, authorship, and/or publication of this article.
The authors thank the Digital Economy Promotion Agency for their support and collaboration. Special thanks to the study participants for their valuable insights and contributions. Additionally, we appreciate the assistance our colleagues at the Digital Economy Promotion Agency in Thailand provided for their technical support and feedback.
VK was employed by the company datacompany.ai.
The remaining authors declare that the research was conducted in the absence of any commercial or financial relationships that could be construed as a potential conflict of interest.
All claims expressed in this article are solely those of the authors and do not necessarily represent those of their affiliated organizations, or those of the publisher, the editors and the reviewers. Any product that may be evaluated in this article, or claim that may be made by its manufacturer, is not guaranteed or endorsed by the publisher.
Almeida, R. (2009). Openness and technological innovation in East Asia: Have they increased the demand for skills? Rochester, NY: SSRN Scholarly Paper.
ASEAN Secretariat. ASEAN Digital Masterplan 2025. ASEAN, (2021). Available at: https://asean.org/book/asean-digital-masterplan-2025/
Barns, S. (2018). Smart Cities and urban data platforms: designing interfaces for smart governance. City Cult. Soc. Innov. Ident. Next Gene. Smart Cities 12, 5–12. doi: 10.1016/j.ccs.2017.09.006
Barr, J., and Attrey, A. (2017). Building local responsiveness in employment and skills Systems in Southeast Asia: Lessons from Malaysia, the Philippines, Thailand and Vietnam. Paris: OECD.
Batty, M. (2013). Big data, Smart Cities and City planning. Dial. Hum. Geograph. 3, 274–279. doi: 10.1177/2043820613513390
Baxter, S., Barnes, A., Lee, C., Mead, R., and Clowes, M. (2023). Increasing public participation and influence in local decision-making to address social determinants of health: a systematic review examining initiatives and theories. Local Government Studies 49:861–887. doi: 10.1080/03003930.2022.2081551
Becker, J., Chasin, F., Rosemann, M., Beverungen, D., Priefer, J., vom Brocke, J., et al. (2023). City 5.0: citizen involvement in the Design of Future Cities. Electron. Mark. 33:10. doi: 10.1007/s12525-023-00621-y
Bentotahewa, V., Hewage, C., and Williams, J. (2022). The normative power of the GDPR: a case study of data protection Laws of south Asian countries. SN Comput. Sci. 3:183. doi: 10.1007/s42979-022-01079-z
Berntzen, Lasse, Johannessen, Marius Rohde, and Ødegård, Ansgar. (2016). A citizen-centric public sector: why citizen centricity matters and how to obtain it. 14-20. Available at: https://openarchive.usn.no/usn-xmlui/handle/11250/2645409.
Bokolo, A. J. (2023). Data driven approaches for Smart City planning and design: a case scenario on urban data management. Digital Policy Regul. Govern. 25, 351–367. doi: 10.1108/DPRG-03-2022-0023
Chanhthamixay, B., Vassanadumrongdee, S., and Kittipongvises, S. (2017). Assessing the sustainability level of municipal solid waste Management in Bangkok, Thailand by Wasteaware benchmarking indicators. Appl. Environ. Res. 39, 49–61. doi: 10.35762/AER.2017.39.3.6
Chaturvedi, Kanishk, Matheus, Andreas, Nguyen, Son H., and Kolbe, Thomas H. (2018). ‘Securing spatial data infrastructures in the context of Smart Cities’. In 2018 international conference on Cyberworlds (CW), 403–408.
Chien, S., Doubleday, J., Mclaren, D., Tran, D., Tanpipat, V., Chitradon, R., et al. (2013). Monitoring flooding in Thailand using earth observing one in a Sensorweb. IEEE J. Select. Top. Appl. Earth Observ. Remote Sens. 6, 291–297. doi: 10.1109/JSTARS.2013.2247974
Chitwatkulsiri, D., Miyamoto, H., Irvine, K. N., Pilailar, S., and Loc, H. H. (2022). Development and application of a real-time flood forecasting system (RTFlood system) in a tropical urban area: a case study of Ramkhamhaeng polder, Bangkok, Thailand. Water 14:1641. doi: 10.3390/w14101641
Chokphukhiao, Chaturapron, Patramanon, Rina, Maitree, Kitkamon, and Kasemvilas, Sumonta. (2020). ‘Health monitoring platform for emergency medicine: user perspective and implementation’. In 2020 joint international conference on digital arts, media and technology with ECTI northern section conference on electrical, electronics, computer and telecommunications engineering (ECTI DAMT & NCON), 133–136.
Choosakun, A., Chaiittipornwong, Y., and Yeom, C. (2021). Development of the cooperative intelligent transport system in Thailand: a prospective approach. Inf. Dent. 6:36. doi: 10.3390/infrastructures6030036
Commonwealth Secretariat (2022). Regional youth digital skill strategies: South Asia, phase 1 : Commonwealth iLibrary.
DAMA International (2017). DAMA-DMBOK: Data management body of knowledge. 2nd Edn. Vancouver, WA: Technics Publications.
DEPA. (2021). ‘Depa Thailand—Smart City plan’. 18 August 2021. Available at: https://www.depa.or.th/en/smart-city-plan/smart-city-office
Djordjevic, M., and Dankovic, D. (2019). A smart weather station based on sensor technology. Facta Univ. Series 32, 195–210. doi: 10.2298/fuee1902195d
Duangsuwan, S., and Jamjareekulgarn, P. (2020). Development of drone real-time air pollution monitoring for Mobile smart sensing in areas with poor accessibility. Sensors Materials 32:511. doi: 10.18494/SAM.2020.2450
Fang, Y., Shan, Z., and Wang, W. (2021). Modeling and key Technologies of a Data-Driven Smart City System. IEEE Access 9, 91244–91258. doi: 10.1109/ACCESS.2021.3091716
Geo-Informatics and Space Technology Development Agency. (2024). ‘GISTDA sphere platform’. GISTDA Sphere Platform (blog). 2024. Available at: https://sphere.gistda.or.th/
Gils, B. (2020). Data management: a gentle introduction: Balancing theory and practice, Van Haren Publishing.
Goreczky, Péter. (2022). ‘Southeast Asia in the US-China tech rivalry II. Digital Infrastructure Development and Data Governance | Magyar Külügyi Intézet’. 28 April 2022. Available at: https://hiia.hu/en/southeast-asia-in-the-us-china-tech-rivalry-2-digital-infrastructure-development-and-data-governance/
GSMA. Mobile connectivity index. GSMA, (2021). Available at: https://www.gsma.com/r/somic-2021/
Gupta, Shristi, Mustafa, Syed Ziaul, and Kumar, Harish. (2017). ‘Smart people for Smart Cities: a behavioral framework for personality and roles’. In Advances in Smart Cities, 23–30. Chapman and Hall/CRC. Available at: https://www.taylorfrancis.com/chapters/edit/10.1201/9781315156040-3/smart-people-smart-cities-behavioral-framework-personality-roles-shristi-gupta-syed-ziaul-mustafa-harish-kumar
Hashem, I. A., Targio, V. C., Anuar, N. B., Adewole, K., Yaqoob, I., Gani, A., et al. (2016). The role of big data in Smart City. Int. J. Inf. Manag. 36, 748–758. doi: 10.1016/j.ijinfomgt.2016.05.002
Heeks, R., and Bukht, R. (2018). Digital economy policy: the case example of Thailand. SSRN Scholarly Paper: Rochester, NY.
Idris, Syahril Anuar, Lazam, Nor Azlinah Md, Izhar, Lila Iznita, Azman, Dharwisyah Bt, Way, Lim Jin, and Aida, Intan. (2022). ‘Smart Health Monitoring Wristband with Auto-Alert Function’. In 2022 IEEE 5th international symposium in robotics and manufacturing automation (ROMA), 1–7.
International Telecommunication Union. Measuring digital development. ITU, (2021). Available at: https://www.itu.int/en/ITU-D/Statistics/Documents/facts/FactsFigures2021.pdf
Jamnongchob, A., Duangphakdee, O., and Hanpattanakit, P. (2017). CO 2 emission of tourist transportation in Suan Phueng Mountain, Thailand. Energy Procedia 136, 438–443. doi: 10.1016/j.egypro.2017.10.300
Jantakat, Yaowaret, Juntakut, Pongpun, and Kranka, Sanun. (2018). ‘Applied geo-informatics technology to urban green space management on role of Stormwater runoff reducing and increasing of subsurface water’. Available Source: http://nriis.nrct.go.th (April 25:2560).
Jinda, Phadet, and Manmart, Lampang. (2013). ‘Information Management for Community Management of local administrative Organizations in Thailand’. Available at: https://citeseerx.ist.psu.edu/document?repid=rep1&type=pdf&doi=0cb1ac508642c0ce9edefcccdc092c5e55aad228
Jitkajornwanich, Kulsawasd, Kongthong, Chanwit, Khongsoontornjaroen, Nattaya, Kaiyasuan, Jeedapa, Lawawirojwong, Siam, Srestasathiern, Panu, et al. (2018). ‘Utilizing twitter data for early flood warning in Thailand’. In 2018 IEEE international conference on big data (big data), 5165–5169.
Jobs on the Rise in 2021: Southeast Asia Report. (2021). Available at: https://business.linkedin.com/talent-solutions/resources/talent-acquisition/jobs-on-the-rise-sea.
Kaluarachchi, Y. (2022). Implementing data-driven Smart City applications for future cities. Smart Cities 5, 455–474. doi: 10.3390/smartcities5020025
Kamnuansilpa, P., Laochankham, S., Crumpton, C. D., and Draper, J. (2020). Citizen awareness of the Smart City: a study of Khon Kaen, Thailand. J. Asian Finan. Econ. Business 7, 497–508. doi: 10.13106/jafeb.2020.vol7.no7.497
Kanabkaew, T., Mekbungwan, P., Raksakietisak, S., and Kanchanasut, K. (2019). Detection of PM2.5 plume movement from IoT ground level monitoring data. Environ. Pollut. 252, 543–552. doi: 10.1016/j.envpol.2019.05.082
Kanchanarat, B., Akkathai, U., Pimno, A., and Malisuwan, S. (2023). Monitoring wildfires in Thailand: a case study of the ECSTAR-TeroSpace’s earth observation project. J. Geosci. Environ. Protect. 11, 23–36. doi: 10.4236/gep.2023.116002
Kaur, A. (2010). Labour migration in Southeast Asia: migration policies, labour exploitation and regulation. J. Asia Pac. Econ. 15, 6–19. doi: 10.1080/13547860903488195
Khianmeesuk, Verathian. (2017). ‘A conceptual framework to develop Smart Cities in Thailand’. In international conference on literature, history, humanities and interdisciplinary studies (LHHISS-17), Bangkok (Thailand), 79–84.
Kong, L., and Woods, O. (2021). Scaling smartness, (de)Provincialising the City? The ASEAN Smart Cities network and the translational politics of technocratic regionalism. Cities 117:103326. doi: 10.1016/j.cities.2021.103326
Kourtit, K., Nijkamp, P., and Steenbruggen, J. (2017). The significance of digital data Systems for Smart City Policy. Socio Econ. Plan. Sci. 58, 13–21. doi: 10.1016/j.seps.2016.10.001
Leesa-nguansuk, Suchit. "Saensuk kicks off Smart City for the elderly." Bangkok Post, July 27, (2016). Available at: https://www.bangkokpost.com/life/tech/1045417/saensuk-kicks-off-smart-city-for-the-elderly
Mainuddin, M., Loof, R., and Abernethy, C. L. (2000). Operational plans and performance in the Phitsanulok irrigation system, Thailand. Int. J. Water Res. Dev. 16, 321–342. doi: 10.1080/07900620050059205
Malek, J. A., Lim, S. B., and Yigitcanlar, T. (2021). Social inclusion indicators for building citizen-centric Smart Cities: a systematic literature review. Sustain. For. 13:376. doi: 10.3390/su13010376
McKinsey and Company. Unlocking success in digital transformations. McKinsey & Company, (2018). Available at: https://www.mckinsey.com/~/media/McKinsey/Business%20Functions/Organization/Our%20Insights/Unlocking%20success%20in%20digital%20transformations/Unlocking-success-in-digital-transformations.pdf
Kittima, M. (2016). ‘Towards modeling linked open data for decision support: an example application of Thailand tourism linked data visualization’. In 2016 Management and innovation technology international conference (MITicon), MIT-88. IEEE. Available at: https://ieeexplore.ieee.org/abstract/document/8025240/
Mutiarin, Dyah, and Lawelai, Herman. (2023). ‘Optimizing the role of ICT and citizen participation: analysis of Smart City governance implementation in Jakarta, Indonesia and Kuala Lumpur, Malaysia’. Edited by D. Mutiarin, M. Alam, D. Cahill, J. Sharifuddin, M. Senge, A. Robani, P. Saiyut, and A. Nurmandi. E3S web of conferences 440:03027.
Nanthaamornphong, Aziz, Holmes, Jeffrey, and Asawateera, Pracha. (2020). ‘A case study: Phuket City data platform’. In 2020 17th international conference on electrical engineering/electronics, computer, telecommunications and information technology (ECTI-CON), 717–722.
Nikomborirak, Deunden, and Ruenthip, Kittipong. (2013). ‘Policy brief: history of water resource and flood Management in Thailand’. TDRI. Available at: https://tdri.or.th/wp-content/uploads/2013/11/Policy-Brief-01-History-of-Flood-Management-in-Thailand.pdf
Nimmanphatcharin, N., Prathombutr, P., Siddhichai, S., Non, A., and Treethidtaphat, W. (2020). Smart City initiatives in Thailand: key concepts and methods. Hitachi Rev. 70, 106–110. Available at: https://www.depa.or.th/storage/app/media/file/Smart-City-Thailand-Hitachi-Review-20210624.pdf
OECD. OECD digital economy outlook. OECD, (2021). Available at: https://www.oecd-ilibrary.org/economics/oecd-economic-outlook/volume-2021/issue-1_edfbca02-en
Open Data Maturity Model’. (2019). The ODI. 2019. Available at: https://theodi.org/
Phdungsilp, A. (2010). Integrated energy and carbon modeling with a decision support system: policy scenarios for low-Carbon City development in Bangkok. Energy Policy 38, 4808–4817. doi: 10.1016/j.enpol.2009.10.026
Pinyochatchinda, S. (2014). Pollution management and Industrial Estates: perceptions of residents in the vicinity of Map Ta Phut industrial estate, Thailand. Inform. Manag. Bus. Rev. 6, 42–48. doi: 10.22610/imbr.v6i1.1099
Pongpiachan, S., and Paowa, T. (2015). Hospital out-and-in-patients as functions of trace gaseous species and other meteorological parameters in Chiang-Mai, Thailand. Aerosol Air Qual. Res. 15, 479–493. doi: 10.4209/aaqr.2013.09.0293
Radziszewska, A. (2023). Data-driven approach in knowledge-based Smart City management. European Conf. Knowl. Manag. 24, 1090–1098. doi: 10.34190/eckm.24.2.1600
Rasiah, R. (2009). Expansion and slowdown in southeast Asian electronics manufacturing. J. Asia Pac. Econ. 14, 123–137. doi: 10.1080/13547860902785963
Rathore, M. M., Paul, A., Hong, W.-H., Seo, H. C., Awan, I., and Saeed, S. (2018). Exploiting IoT and big data analytics: defining smart Digital City using real-time urban data. Sustain. Cities Soc. 40, 600–610. doi: 10.1016/j.scs.2017.12.022
Rojanasuvan, Naark. (2024). ‘NBT CONNEXT’. 18 July 2024. Available at: https://thainews.prd.go.thnull
Sagarik, D., Chansukree, P., Cho, W., and Berman, E. (2018). E-government 4.0 in Thailand: the role of central agencies. Inform. Polity 23, 343–353. doi: 10.3233/IP-180006
Shafiullah, M., Rahman, S., Imteyaz, B., Aroua, M. K., Hossain, M. I., and Rahman, S. M. (2023). Review of Smart City energy modeling in Southeast Asia. Smart Cities 6, 72–99. doi: 10.3390/smartcities6010005
Silva, B., Khan, M., Jung, C., Seo, J., Muhammad, D., Han, J., et al. (2018). Urban planning and Smart City decision management empowered by real-time data processing using big data analytics. Sensors 18:2994. doi: 10.3390/s18092994
Smitananda, Phanompatt, and Akahat, Noppon. (2024). ‘Digital technology policy initiation of local administrative ORGANIZATIONS: the lessons of SMART city development in Thailand’. Asian Administration & Management Review 7 (1). Available at: https://papers.ssrn.com/sol3/papers.cfm?abstract_id=4764591
Srimuang, Chatipot, Cooharojananone, Nagul, Tanlamai, Uthai, and Chandrachai, Achara. (2017(. ‘Open Government Data Assessment Model: An Indicator Development in Thailand’. In 2017 19th international conference on advanced communication technology (ICACT), 341–47. IEEE. Available at: https://ieeexplore.ieee.org/abstract/document/7890110/.
Sta, H. B. (2017). Quality and the efficiency of data in “smart-cities”. Futur. Gener. Comput. Syst. 74, 409–416. doi: 10.1016/j.future.2016.12.021
Stephenson, M. L., and Dobson, G. (2020). Deciphering the development of smart and sustainable tourism cities in Southeast Asia: a call for research. Adv. Southeast Asian Stud. 13, 143–153. doi: 10.14764/10.ASEAS-0033
Subha, J, and Kowsigan, M. (2022). ‘IoT enabled water distribution Systems for Energy Efficiency in WSN’. In 2022 international conference on innovative computing, intelligent communication and smart electrical systems (ICSES), 1–8.
Thongprakob, Thanadol. (2018). ‘The role of central Agency in Promoting Open Government in Thailand: the case study of digital government development agency (DGA)’. Available at: https://digital.car.chula.ac.th/chulaetd/2401/
Tippayanate, N., Impool, T., Sujayanont, P., Muttitanon, W., Chemin, Y. H., and Som-ard, J. (2024). Spatial distribution and cluster analysis of road traffic accidents in Khon Kaen municipality, Thailand. Int. J. Geoinform. 20, 43–55. doi: 10.52939/ijg.v20i4.3149
Traffy Fondue. (2022). Traffy fondue. 18 February 2022. Available at: https://www.nectec.or.th/news/news-article/traffy-article-2022.html
Traffy Fondue. (2024). ‘Traffy’. Fondue. October 2024. Available at: https://www.traffy.in.th/
Trimek, J. (2016). Public confidence in CCTV and fear of crime in Bangkok, Thailand. Int. J. Crim. Justice Sci. 11, 17–19. Available at: https://ijcjs.com/menu-script/index.php/ijcjs/article/view/171
UN Secretary-General’s Data Strategy. (2020). UN Data Strategy. 2020. Available at: https://www.un.org/en/content/datastrategy/
Walentek, D. (2021). Datafication process in the concept of Smart Cities. Energies 14:4861. doi: 10.3390/en14164861
World Bank (2021). World development report 2021: Data for better lives : World Bank https://www.worldbank.org/en/publication/wdr2021.
World Economic Forum (2020). The future of jobs report 2020 : World Economic Forum https://www.weforum.org/publications/the-future-of-jobs-report-2020/.
Keywords: Smart Cities, data strategy, citizen participation, urban innovation, sustainable urban development, sensors, data platforms
Citation: Hansen MM, Koonsanit K and Kulmala V (2025) How can data contribute to Smart City innovation: a study from Thailand’s Smart City initiatives. Front. Sustain. Cities. 6:1473123. doi: 10.3389/frsc.2024.1473123
Received: 30 July 2024; Accepted: 29 November 2024;
Published: 06 January 2025.
Edited by:
Miao Yu, University of Lincoln, United KingdomReviewed by:
Syed Waqar Ahmed, National University of Computer and Emerging Sciences, PakistanCopyright © 2025 Hansen, Koonsanit and Kulmala. This is an open-access article distributed under the terms of the Creative Commons Attribution License (CC BY). The use, distribution or reproduction in other forums is permitted, provided the original author(s) and the copyright owner(s) are credited and that the original publication in this journal is cited, in accordance with accepted academic practice. No use, distribution or reproduction is permitted which does not comply with these terms.
*Correspondence: Michael Motet Hansen, bWljaGFlbC5oYW5AZG9tZS50dS5hYy50aA==
Disclaimer: All claims expressed in this article are solely those of the authors and do not necessarily represent those of their affiliated organizations, or those of the publisher, the editors and the reviewers. Any product that may be evaluated in this article or claim that may be made by its manufacturer is not guaranteed or endorsed by the publisher.
Research integrity at Frontiers
Learn more about the work of our research integrity team to safeguard the quality of each article we publish.