- 1Faculty of Habitat, Autonomous University of San Luis Potosí, San Luis Potosí, Mexico
- 2Department of Work Coordination, HG Housing Developments, San Luis Potosí, Mexico
- 3Multidisciplinary Postgraduate Program for Environmental Sciences, Autonomous University of San Luis Potosí, San Luis Potosí, Mexico
- 4Postgraduate College San Luis Campus, Salinas de Hidalgo, Mexico
In the face of excessive urban growth, urban green spaces face the challenge of efficiently providing ecosystem and environmental services benefits. While public green spaces (PGS) stand out for their different environmental and social benefits, their efficiency depends on the vegetated cover, which can be evaluated in relation to the area, type of polygon, and degree of equipment. In this study, the effective green cover (EGC) assessed from a geographic information system, and the level of equipment–infrastructure were evaluated in different green spaces in the metropolitan area of San Luis Potosí, Mexico. The PGS categories included park with hydrological potential, urban park, linear park, neighborhood park, local garden, residual green space, and sports area. In our results, the urban park and the park with hydrological potential indicated 69.5 and 79.5% of EGC, respectively, and a value of 0.3 of Normalized Difference Vegetation Index (NDVI). Specifically, only in urban park, the land surface temperature (LST) decreased with the increase in the NDVI. The total green coverage of PGS was 6.7 m2 and the EGC was 5.8 m2, which is largely due to the large-sized parks. Furthermore, the provision of parks is insufficient compared to other metropolitan areas on national and international scales. In the spaces with the highest score of equipment, the outstanding elements were urban furniture, children’s playgrounds, and exercise areas, which varied according to the type of green space, indicating a differentiated social use. This study suggests that EGC can be a parameter to assess the green proportion of PGS in cities. Integrating this variable in PGS planning and design can enhance ecosystem services provision in metropolitan areas.
1 Introduction
In recent decades, green spaces have become an emerging environmental challenge in cities. The different services they offer are important, given the accelerated growth of urban areas, the current environmental conditions, and their social needs (Breuste et al., 2015; Paul and Nagendra, 2017; Barradas et al., 2022). This is the reason why aspects such as the distribution, typology, and quality of green areas reach special importance in studies of urban ecology and environment (McPhearson et al., 2016; Nor et al., 2017; Nur Hidayati et al., 2019). Nevertheless, the type of vegetation cover, the shape of the polygon, and the functions of the green spaces are immersed in a built domain matrix (Stewart and Oke, 2012; de la Barrera et al., 2016; Zhang et al., 2019) and, thence, contingent to such conditions. Some aspects that deserve attention are the spatial limitations that such surfaces present in the urban context as well as the functions and characteristics that they offer. The areas dedicated to hosting plants in the public space, generally considered as an aesthetic and utilitarian ornament, represent the green infrastructure whose environmental and social potential needs to be revaluated. Numerous and diverse publications agree that green infrastructure has high standards, regarding urban ecological, environmental, and quality of life, due to the intrinsic properties of the plant component (Norton et al., 2015; Calaza-Martínez, 2016; Jayasooriya et al., 2017; Geneletti et al., 2020).
Given the unequivocal increase of excessive urbanism, vegetation cover becomes an element to be attentively considered because of its properties. These features refer to ecosystem and environmental services such as atmospheric carbon dioxide capture, temperature mitigation, and air humidification, as well as absorption of water in subsoil, among others (Bolund and Hunhammar, 1999; Tratalos et al., 2007; Dobbs et al., 2011). Several studies agree on the need of increasing the green cover in quantity and quality so that such functions are significant in the environment. The ability to attenuate heating or provide oxygen to the environment is the property of great value as long as the vegetation cover is abundant and outstanding, especially at wooded (Georgi and Zafiriadis, 2006; Armson et al., 2012; Puliafito et al., 2013; Tan et al., 2018). For this reason, the environmental conditions are expected to improve in proportion to the quality of the green spaces. A technological tool that contributes to assessing the environmental quality of such surfaces in cities is remote sensing through the use of geographic information system (GIS) (Van Herzele and Wiedemann, 2003; de la Barrera and Henríquez, 2017). As a matter of principle, one of the parameters of great attention is the one that determines the square meters of green area in a specified urban space. According to the World Health Organization, the figures of 9 and 20 m2 of green area per inhabitant are the minimal recommended and the ideal, respectively (WHO, 2016). Such figures have become a generalized indicator to quantify green areas and a reference in different reports and publications (Bascuñán-Walker et al., 2007; PAOT, 2010; Morar et al., 2014; Russo and Cirella, 2018). Some studies even warn that the increase in the green proportion in residential communal spaces provides environmental functions (Szulczewska et al., 2014), ecosystem social services (Mao et al., 2020), and public health (Zhang et al., 2017). However, it is important to mention that there is no standardized method to determine this measure and its use is only complementary in the study of green areas.
The literature on public green areas is extensive in different latitudes and more numerous in regions of developed countries, as reported in a metadata study on the classification and categorization of green infrastructure (Bartesaghi-Koc et al., 2017). Similarly, only 1% of the studies of green areas and urban densification, which have been reported on a global scale, correspond to the Latin American region (Haaland and van den Bosch, 2015). The research in Latin American cities focuses on social topics, the plant cover composition, and the multi-scalar climatic conditions (Quintero, 2012; Vásquez et al., 2016). Excepting the capital of the country, Mexican cities are poorly researched and observe few published works about the proportion of green areas. According to the latest update of the green areas inventory, the distribution of green surface in Mexico City is unequal (PAOT, 2010) and with an average value of 7.5 m2 per inhabitant (SEDEMA, 2017), which is less than the minimum recommended by WHO. In this context, it is necessary to determine the conditions of the green cover and the type of green space in cities, whose accelerated growth and lack of planning presume important limitations. In this way, the detailed evaluation in the mapping of green spaces from satellite images becomes essential. Ju et al. (2022) warn that in an arid city like San Luis Potosí, urban green spaces may have spectral properties and background surface aggregates, which cause confusion when differentiating the covers. Therefore, the study of urban green spaces requires greater specificity, in terms of both their vegetated covers and their typology.
The green infrastructure includes a wide variety of differentiated spaces by its size and function. Brzoska and Spāģe (2020) indicate that ecosystem services that enhance human life quality are focused on large spatial scales. Such attributes can be associated with urban and peri-urban forests and parks, as well as some gardens, where such functions are more attainable if these places are public. An a priori statement is that the number and diversity of activities can increase in proportion to the green cover; thus, urban parks are places of great social appeal. This phenomenon can be observed in cities of different regions. Some recognized Mexican urban parks can be mentioned as examples: Bicentenario in Mexico City, Bosque Los Colmos in Guadalajara, Fundidora in Monterrey, and Tangamanga 1 in San Luis Potosí. Although it is recognized that urban parks encompass important functions for the city, other smaller public green spaces, such as linear parks, community gardens, and sports facilities, among others, are also important for social benefits provision (Byrne and Sipe, 2010). It could be said that each public space is a reflection of the visitors and activities that its design allows (Goličnik-Marušić, 2015).
Given the correspondence between the vegetation portion and the number of inhabitants, the easily quantifiable green areas are the public ones in contrast to the private ones, whose factors and conditions correspond to another type of research. An adequate use and approach to the concept of green space must consider the context in which the study is carried out (Taylor and Hochuli, 2017). In this sense, the public green space implies an open area, with free access, which hosts a set of planted vegetal species, and a certain degree of infrastructure and equipment attributes. All these properties assume a wide range of outdoor activities. In general, physical exercise, children’s play, walks, rest, and leisure are common activities in public spaces, but currently, such spaces can also be multifunctional and also have a high added environmental value (Byrne and Sipe, 2010; van Leeuwen et al., 2010; Shackleton et al., 2018). The different components, services, and amenities can have a great influence on the activities and social gatherings that each green space offers. In addition, the quantification of equipment such as urban furniture and infrastructure can be an indirect measure of knowing a green area in terms of its social functions. In urban parks, the location of the street furniture, in addition to the space quality, can influence citizens’ attraction to it as a leisure center (Kargar and Ghassemi, 2016). Moreover, multifunctional street furniture can be a reason for family gatherings and leisure, even in smaller parks (Thamrin et al., 2018). In this sense, the built components are as necessary as the ecological features in public green spaces for fulfilling social function.
In this direction, the present study consisted of assessing the quality of the effective green cover (EGC) and the current condition of equipment–infrastructure attributes in public green spaces (PGS) in the metropolitan area of San Luis Potosí, Mexico. These variables are relevant aspects of urban planning and design but are often left aside. In this manner, it is assumed that the environmental benefits will increase in proportion to the detectable green portion, resulting in a vegetated cover with higher quality. In addition, large green areas offer a socially added value if incorporating adequate and sufficient equipment–infrastructure attributes without suppressing the vegetated cover. These premises are based on determining the exclusive environmental capacity of the vegetation component and the benefits that public green infrastructure provides to society. Thus, given its disproportion to the growing urban development of the city, an aspect of the social functionality of green areas is the level of equipment–infrastructure they present. Based on this priority, and in accordance with the 11th sustainable development goal (UN, 2018), green spaces are an indicator of urban sustainability. In addition, an emerging challenge within the study area is increasing the cooling and shading through the vegetated cover, thereby contributing to mitigating the semi-arid condition of the city for a large part of the year. The high annual proportion of radiant energy and sunny days in the region indicate the need for cooler public environments. For this reason, the specific portion of the vegetated cover and the green area calculation per inhabitant are indicators of ecological interest. However, each indicator offers different information regarding the “status of green areas. In this context, the research question is which indicator is more realistic regarding the effective green cover of these spaces. Moreover, equipment–infrastructure attributes can be an indirect way of assessing the social attractiveness of a PGS. Thus, their quantification also contributes to measuring the green spaces” conditions. The research in the above-mentioned metropolitan area is mainly justified by its current urban growth, which does not correspond to the creation of new public green spaces with their respective equipment and infrastructure.
2 Methods
2.1 Description of the study site
The present study considered the metropolitan area of San Luis Potosí, Mexico, comprising the city of the same name and the municipality of Soledad de Graciano Sánchez. It is located at a latitude of 22° 01′ 34″–22° 13′ 06″ N and a longitude of 101° 02′ 39″–100° 53′ 21″ W (Figure 1). This area, whose average elevation is 1,863 m.a.s.l., stands out because it presents three climatic units: temperate arid with dry winters (BWkw), temperate semi-arid with dry winters (BS0kw), and temperate semi-dry (BS1kw). For this area, Noyola-Medrano et al. (2009) indicate a low saturation of water vapor and an average precipitation below 400 mm per year. The period with the highest frequency of rainfall in the year is from June to September. The average annual temperature is 17°C, with a maximum of 34°C and a minimum of 0°C. The warm period is from March to October, while the cold period is from November to February. In addition, it has been reported that San Luis Potosí is one of the Mexican regions with the highest hours of annual insolation, based on the frequency of cloudy days, average cloudiness, and clear days (Tejeda-Martínez and Gómez-Azpeitia, 2015). A recent study revealed an increase of 11°C in the surface temperature of the metropolitan area of San Luis Potosí in the last 15 years due to urban growth and land use change (Ovalle et al., 2021); therefore, this suggests important changes in the urban climate. Furthermore, considering all the most populated metropolitan areas in the country, the study area exhibits an urban population density of 105.6 inhabitants/ha (SEDATU, CONAPO and INEGI, 2018), with a total population of 1,243,980 inhabitants and an area of 1,787 km2, according to the 2020 population census (INEGI, 2022).
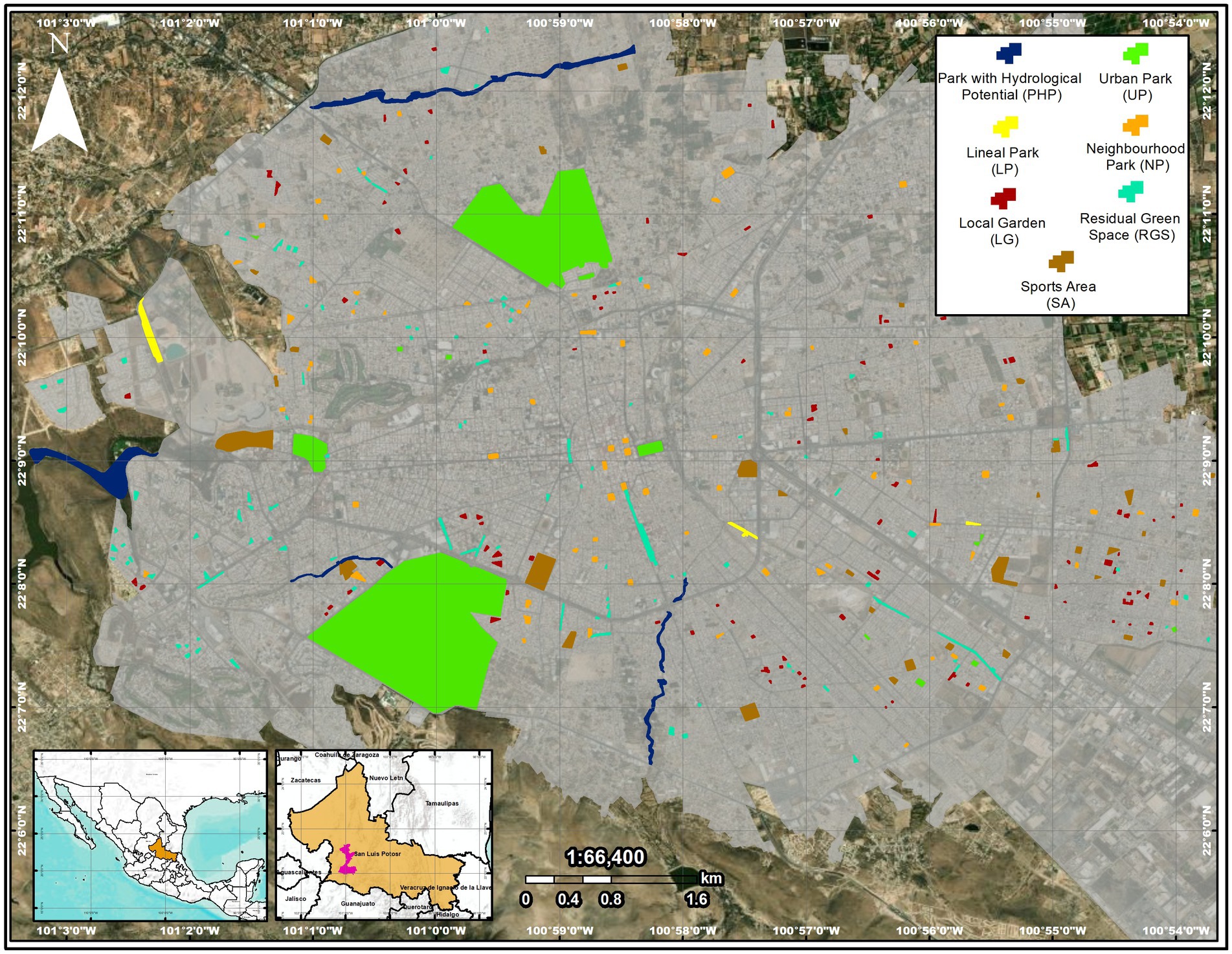
Figure 1. Location and distribution of the PGS categories in the metropolitan zone of San Luis Potosí, Mexico.
2.2 Classification and physical appraisal of green areas
The criteria for considering green areas as public green spaces (PGS) include a clearly defined outline, free access, the presence of vegetative cover, equipment, and infrastructure attributes, and public use. However, despite its low plant density or reduced equipment, space that exhibited recurrent social activity was also considered. Based on these parameters, all the green areas within the metropolitan area of San Luis Potosí were located and georeferenced using the Google Earth tool (U.S. Geological Survey, 2020). In this way, the different PGS were classified into the following categories: park with hydrological potential, urban park, linear park, neighborhood park, local garden, residual green spaces, and sports area.
In the specific case of parks with hydrological potential, the polygons delimitation depended on the vegetation patches continuity along the rivers. This category must be considered because of two important aspects: its hydrological and ecological potential, and its social function. The urban park was considered as a green area of important dimensions, equipment, and social presence for the citizens, while the linear park is differentiated by its typical longitudinal extension. Neighborhood park corresponded to the green space of residential areas or neighborhoods in the city, whereas local garden refers to green areas surrounded by a group of immediate houses. The central difference between neighborhood park and local garden is the location and the infrastructure–equipment provision, respectively. The residual green space was considered as the surplus site of the urban environment, which exhibits either some equipment or adaptations from the inhabitants. Within this category, roundabouts, ridges, and slopes were discarded because equipment and/or visitors were non-existent in comparison with the other classified green areas. Finally, the sports area with public sports facilities and available green areas was estimated (Table 1). The perspective views of the different PGS with their respective urban contexts can be observed in Figure 2. Once PGS were located and classified, they were visited to quantify and appraise their equipment components. The assessment visits were made over a year, from summer 2020 to summer 2021.
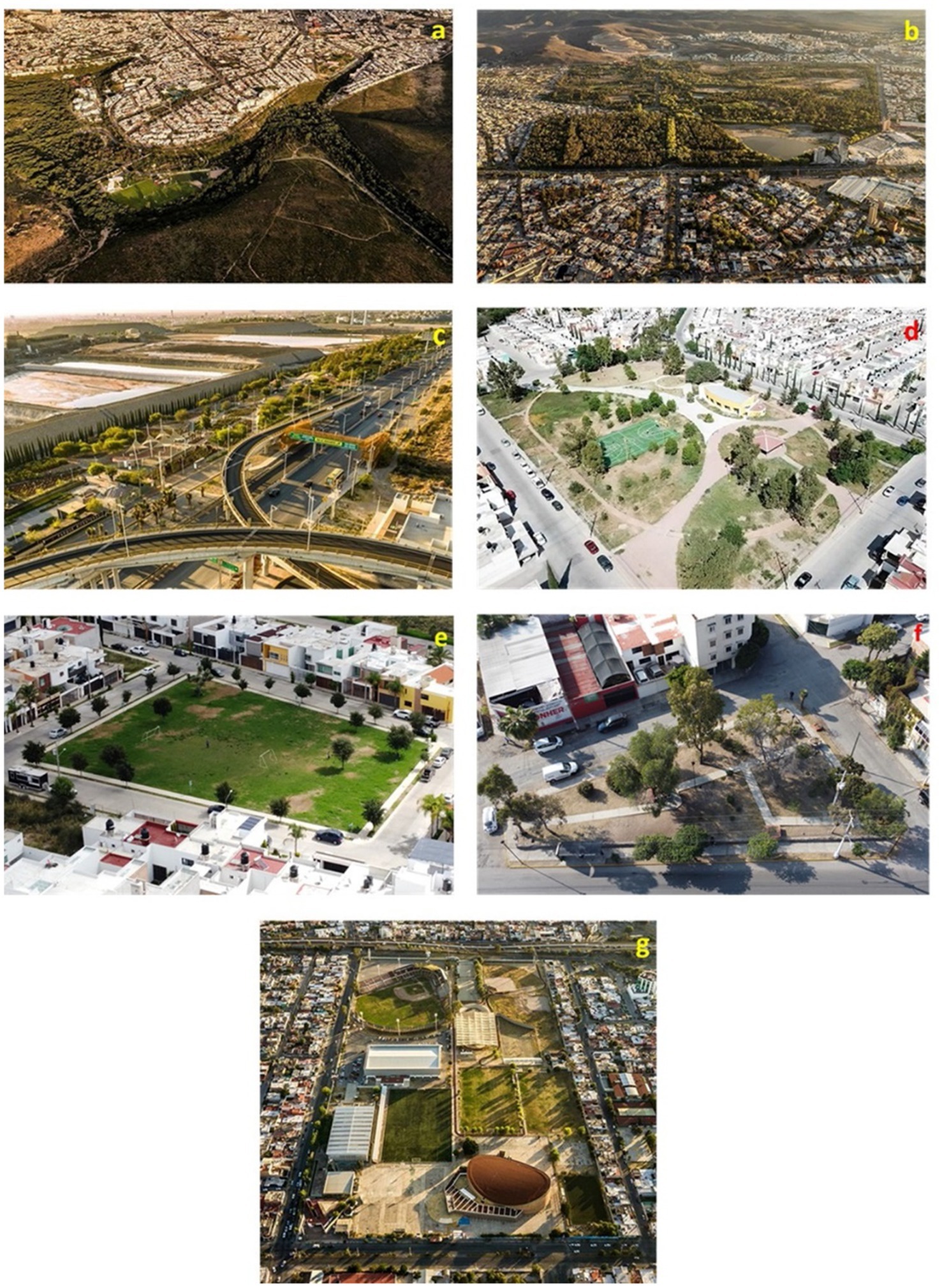
Figure 2. Photographs on the perspective of park with hydrological potential (A), urban park (B), linear park (C), neighborhood park (D), local garden (E), residual green spaces (F), and sports area (G). Photographs by Pedro Mena-Pirex (A–C,G), Andrea Candia Lomelí (D), and Jonathan Hammurabi González-Lugo (E,F).
2.3 Image processing and calculation of variables
First, a multispectral image was downloaded, and the file with the 11 bands and all their metadata was obtained (U.S. Geological Survey, 2020), in order to identify the base study area. The downloaded image was taken on 26 September 2020, from the Landsat 8 satellite with a resolution of 30 m. To ease the detection of the indicator on the surface scale, the image selection criteria were a clear day with humidity and 10% cloudiness during the rainy season. These criteria allow reflecting the period of the year in which vegetation cover is usually greener and more abundant and, hence, can be better visualized. In such a way, from the treatment to the above-mentioned image using the ENVI 5.3 and ArcMap 10.8 software, the following parameters were calculated: Normalized Difference Vegetation Index (NDVI), land surface temperature (LST), and effective green cover (EGC). This last variable was estimated by referring to the net portion of soil covered with vegetative cover and excluding the non-green portion within each polygon. Prior to this process, and according to the selected polygons within the city, the minimum green area detected and valued by the geographic information system was 616.2 m2. Under this criterion, the process of correction and calculation of each variable is described below:
In order to deal with distortions caused by the atmosphere or sensor operation, the radiometric correction was performed using the gain and bias method in the ENVI 5.3 software. Using data from the MTL metadata file of the satellite image, corresponding to the top-of-atmosphere (TOA), Equation 1 was used to convert the digital numbers obtained by the sensor to reflectance values:
The sun angle and azimuth data were obtained from the image attributes of the metadata file, which was converted to radians. For the conversion to radiance in the roof atmosphere, the data were taken from the metadata file where the bands were converted into TOA spectral radiance data, according to Ihlen AND Zanter (2019) and using Equation 4:
The obtained bands were converted from spectral radiance to sensor brightness temperature (Equation 5) (Ihlen and Zanter, 2019) in degrees Kelvin, using the thermal constant supplied in the metadata file:
The NDVI was evaluated using the radiometrically corrected multispectral image and ArcMap 10.8 software. As a result of this test, the vegetated cover and density were determined, with a value scale that goes from 0 to 1 and from blue to green, respectively, reflecting the gradient from lower to higher quality. This calculation was made through the relationship between vegetation and behavior of different wavelengths (Equation 6) (Sobrino et al., 2008):
In order to obtain the land surface temperature (LST) using ArcMap, the emissivity (ɛ) was first calculated, whose proportionality factor predicts the glow emitted and the efficiency of transmitting thermal energy through the surface toward the atmosphere (Sobrino et al., 2008), and according to Yu et al. (2014), Equation 7 is presented:
Therefore, the LST was calculated from the layer obtained from the emissivity and the sensor’s brightness temperature, and this is given using the equation of Ihlen and Zanter (2019) (Equation 9):
After the image correction process, a database was created for the registration and calculation of values. Once the NDVI, EGC, and LST layer was determined, the polygon of a typical PGS and its figure in pixels were chosen to indicate the estimation of such parameters in satellite image processing. An example of the polygons, after the image correction process, for every variable and PGS is presented in Table 2. Similarly, a location and distribution map of the different types of PGS studied in the metropolitan area was elaborated (Figure 1). On the other hand, databases were created with the values resulting from image processing and the evaluation of equipment. From the obtained data, the NDVI mean value, EGC percentage, and non-green cover (NGC) percentage were calculated as observed in Table 3. Moreover, in each PGS of the metropolitan area under study, the green area density per inhabitant was calculated. In this way, from the databases and using R programming software, a simple linear regression analysis was carried out between NDVI and EGC, between LST and EGC, and between LST and NDVI, in order to obtain the degree of environmental contribution of every PGS under study. In addition to evaluating the vegetated cover quality, the EGC proportion was determined, as well as the square meters of green area per inhabitant based on the total area and the EGC for each PGS.
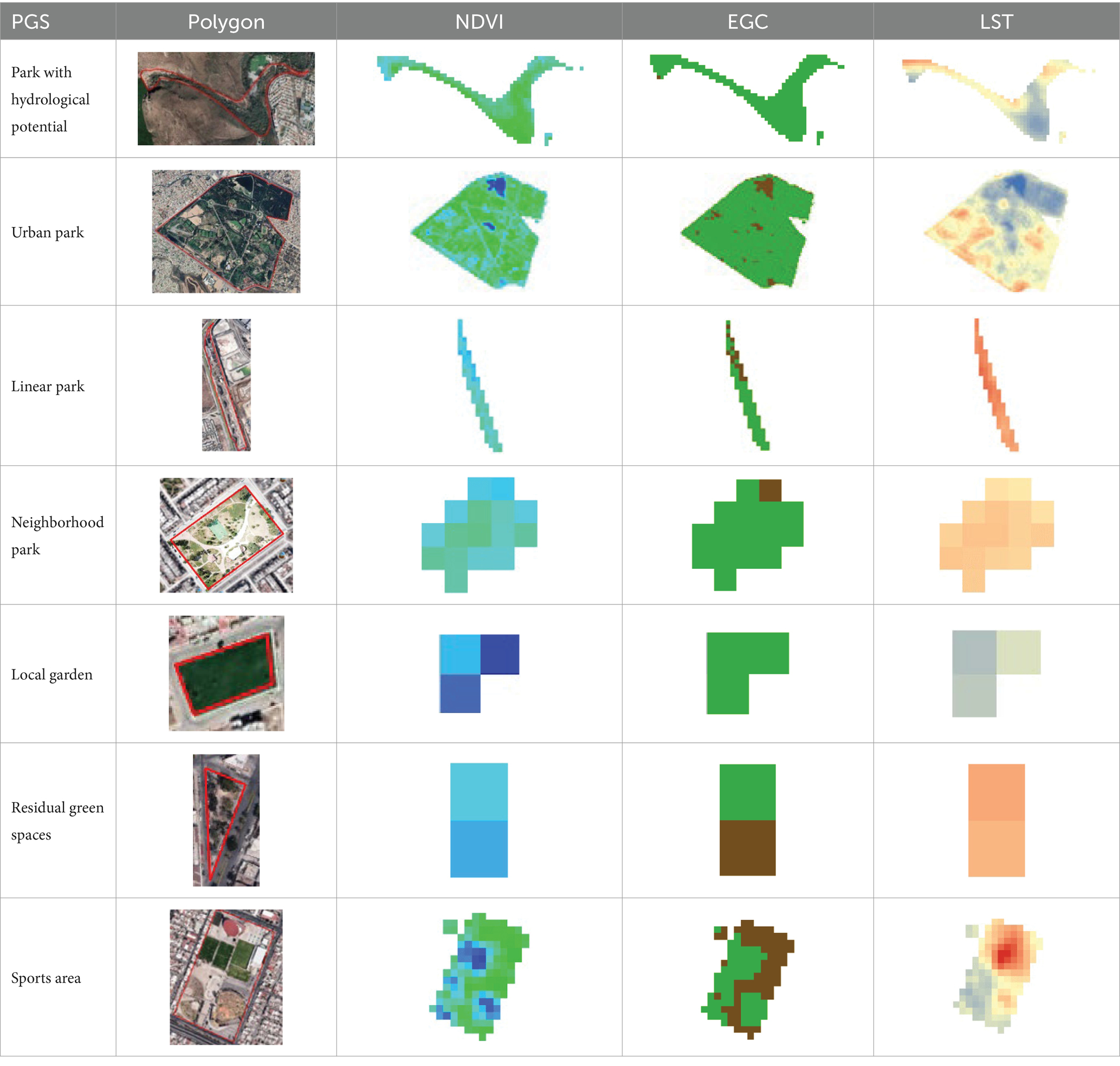
Table 2. Delimitation of a public green space (PGS) by category, and satellite image processing obtained variables as polygon examples: Normalized Difference Vegetation Index (NDVI), effective green cover (EGC), and land surface temperature (LST).
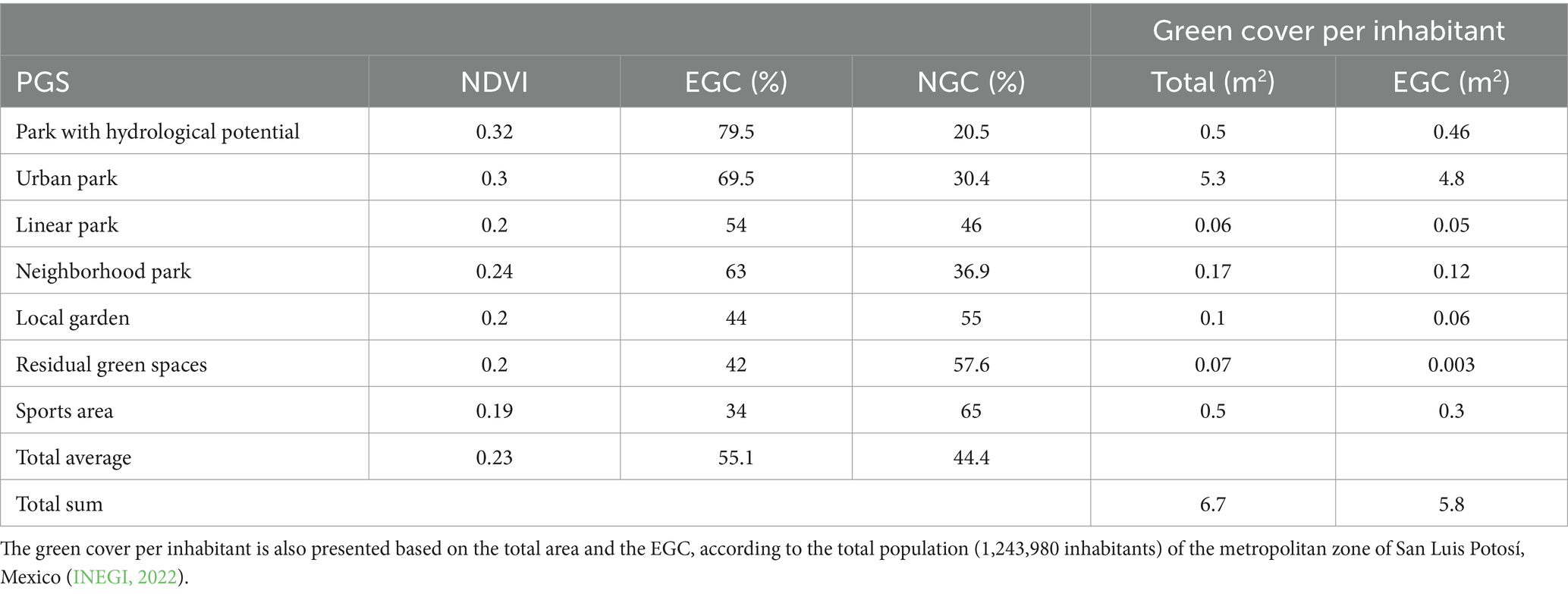
Table 3. Average value of the NDVI, and percentage of the effective green cover (EGC), and non-green cover (NGC) per public green space (PGS) from the GIS analysis data.
The urban park cover per inhabitant for metropolitan areas of Mexico and other countries was analyzed. For this evaluation, all metropolitan areas within a range of 1,300,000 to 1,500,000 inhabitants—both in Mexico and worldwide—were searched. The 24 identified and assessed metropolitan areas with this population density included Aguascalientes, Mexico; Antwerp, Belgium; Bordeaux, France; Bremen, Germany; Buffalo-Cheektowaga, USA; Campinas, Brazil; Da Nang, Vietnam; Dallas, USA; Dublin, Ireland; Florianopolis, Brazil; Gdansk, Poland; Hartford, USA; Louisville-Jefferson, USA; Maceio, Brazil; Mannheim-Ludwigshafen, Germany; Marseille, France; Mendoza, Argentina; Mérida, Mexico; Mexicali, Mexico; New Orleans-Metairie, USA; San Luis Potosí, Mexico; South Yorkshire, UK; Zagreb, Croatia y Zapopan, Mexico (Brinkhoff, 2023). In spite of the fact that other cities in Russia, China, and India were also identified in the aforementioned population range, they were discarded due to the lack of accessibility to information on official websites. After that, the area of a maximum of 30 different urban parks was counted using the official web pages of each city. For those cases, in which information was unavailable, urban parks were identified in Google Maps and their areas were measured using the Google Earth tool. The criteria for identifying public urban parks within each metropolitan area included (a) a well-defined polygon and (b) equipment such as children’s playgrounds, exercise equipment, or sports courts. As a result of the observations, three sizes of urban parks were defined: small, from 1,000 to 100,000 m2; medium, from 100,001 to 500,000 m2; and large, with an area greater than 500,001 m2. Once the values were obtained, they were sorted in a database and analyzed to find some relationship between the variables of number, density, and size of the parks.
2.4 Equipment–infrastructure assessing
Appraisal of equipment was determined from the presence of urban furniture, children’s playgrounds, exercise areas, infrastructure, and services in each assessed green area. Urban furniture elements were counted as equipment attributes; such count included not only the official ones but also equipment improvised by residents, as it also reflects the use and harnessing of the site. Thus, in the street furniture category, a value of 1 was assigned to the green area, if it exhibited benches and garbage cans; and another value of 1 was assigned when it also included picnic tables or bicycle racks. If the green area presented children’s playgrounds—even if it had only one component, but in operation—it was valued with a score of 2. This same criterion was used for the exercise area when presenting a sports field or exercise equipment. The value of 2 assigned to the children’s playgrounds and the exercise areas is based on the fact that these are the types of equipment that promote health and exercise among the population. In order to assess the infrastructure, 1 was assigned to lamps or signage, and another 1 if the site included suitable walkways or ramps for people with disabilities. Regarding the category of services, a value of 1 was given if bathrooms and a guardhouse were present or a value of 2 if the green area included commerce or space for cultural activities. In such a way, the maximum score for equipment that a PGS could achieve was 10 (Table 4). After counting and registering equipment and infrastructure attributes per site, a database was developed for further analysis. Based on the averages of the attributes set for each green space, a one-factor analysis of variance was performed. This allowed distinguishing differences in the general equipment–infrastructure per PGS. In addition, equipment–infrastructure was evaluated disaggregated, through the calculation of averages by attribute and the comparison between PGS and all other PGS. To do this, the Wilcoxon rank-sum test was used to analyze the variability of non-parametric data with different n samples.
3 Results
The PGS presented differences in their quantity and area, influencing the calculated parameters and the respective statistical analysis. The total number of green areas was 401 PGS, of which the most numerous categories and in descending order were residual green spaces, local garden, and neighborhood park with 35.4, 26.4, and 20.4%, respectively, totaling 82.2%. The category of sports area indicated 8.2%, while in park with hydrological potential, urban park, and linear park, the value was 5, 3.5, and 1%, which together encompass 9.5% of the green infrastructure (Figure 3). This same value indicates the total sum of the area that each category presented in the urban space. In this sense, urban park was the category with 605.6 ha, park with hydrological potential with 58.6 ha, and sports area with 38.4 ha. Meanwhile, in neighborhood park, local garden, and residual green spaces, the values were 15.3, 13.8, and 10.8 ha, respectively. In that way, urban park and park with hydrological potential correspond to the largest, but they were the least numerous of all PGS. Instead, neighborhood park, local garden, and residual green spaces categories were the most abundant of the public green infrastructure, but they exhibited the lowest areas of the metropolitan area. In addition to the previous quantification, the following determinations in the PGS focused on their vegetal component and thermal response.
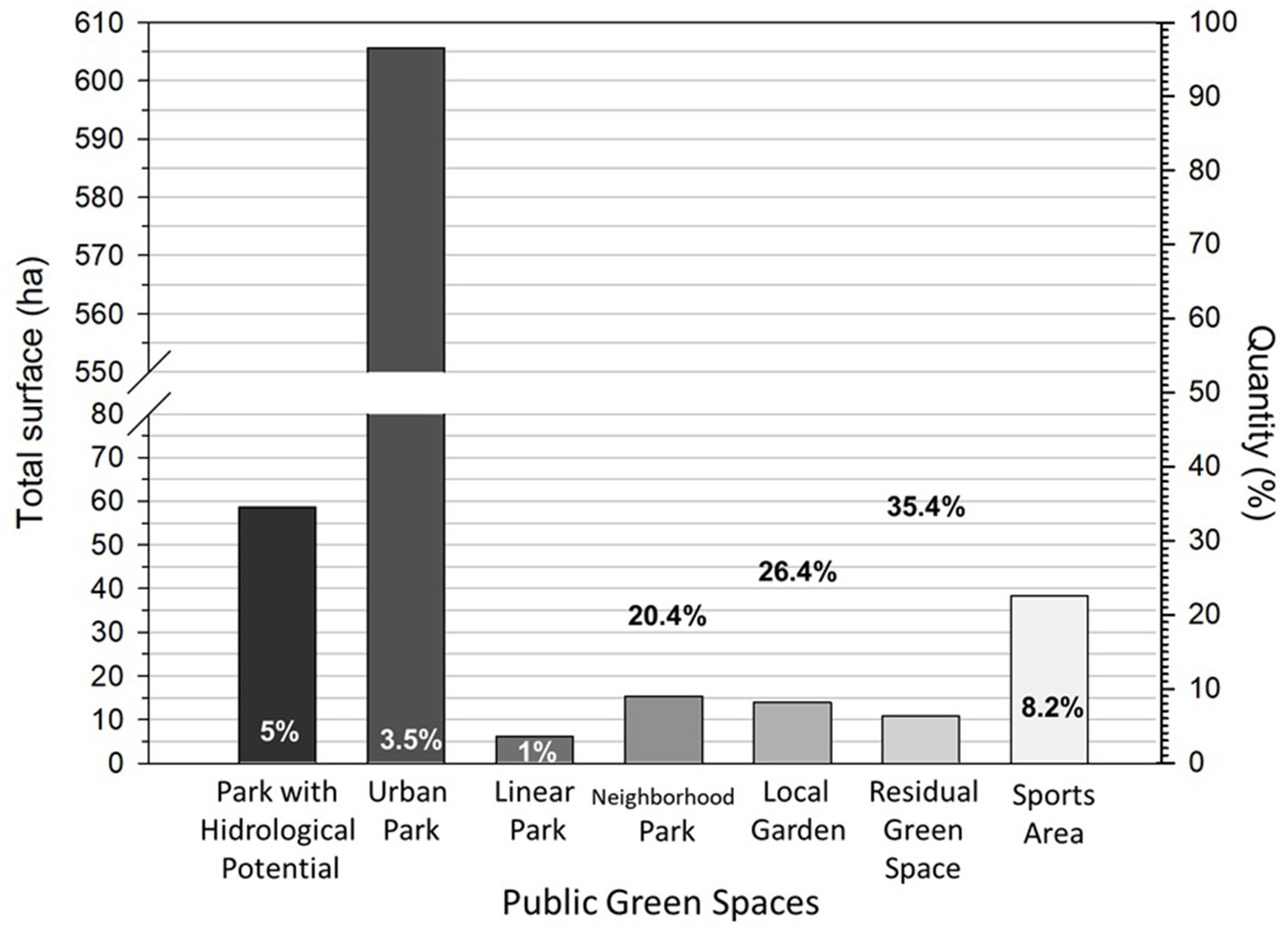
Figure 3. Total surface of the PGS in hectares on the left axis, and the PGS quantity in percentage on the right axis of the bar graph.
3.1 Environmental and ecological parameters in public green spaces
Regarding the green cover evaluated through NDVI in the linear regression, the R2 coefficient resulted in values of 0.5, 0.47, and 0.46 in the sports area, linear park, and local garden, respectively, and less than 0.3 in the other PGS. However, the linear regression model with the best fit to the data and symmetry in residuals was that of the park with hydrological potential (F = 7.6, p = 0.01), urban park (F = 4.83, p = 0.04), local garden (F = 8.15, p = 0.005), and sports area (F = 32.9, p = 0.000) (Figure 4). It can then be said that the NDVI exhibits a slight increase as the EGC is higher for parks with hydrological potential, urban parks, local gardens, and sports areas, whereas the relation was not evident for linear parks, neighborhood parks, and residual green spaces. Independent of the linear regression analysis, the NDVI value stands out, as the highest average value was 0.3 in park with hydrological potential and urban park and 0.2 in the other categories of green spaces as a whole. In addition, the average value of all green infrastructure analyzed was 0.23 NDVI, as can be seen in Table 3.
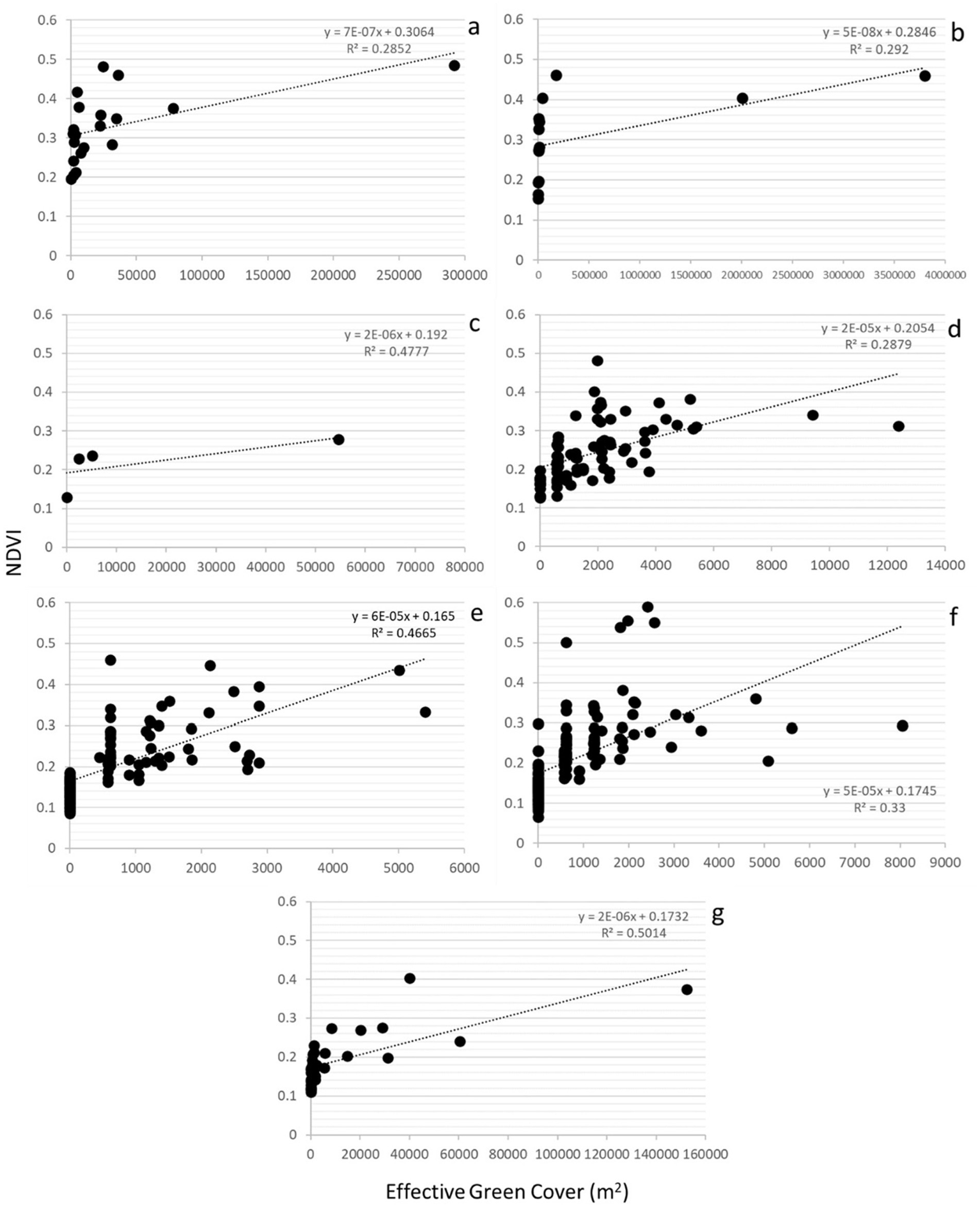
Figure 4. Linear regression between the Normalized Difference Vegetation Index (NDVI) and the effective green cover (EGC) in park with hydrological potential (A), urban park (B), linear park (C), Neighborhood park (D), local garden (E), residual green spaces (F), and sports area (G).
The regression model allowed observing an indirect relationship between LST and EGC, where the R2 coefficient was 0.3 in urban park and below 0.01 in the other categories, except in linear park whose value was 0.5, but with direct proportionality. The green areas in which the regression model was better fit to the data, with symmetric residuals were urban park (F = 5.9, p = 0.03), neighborhood park (F = 5.45, p = 0.02), and local garden (F = 8.87, p = 0.003) (Figure 5). Moreover, in the regression test, the lowest LST average values were 31.6 and 31.8°C in park with hydrological potential and urban park, respectively. In contrast, the highest average value was 33°C in the sports area. Meanwhile, in the other green spaces, the average value oscillated approximately 32°C. In this way, the LST difference between park with hydrological potential and urban park, regarding sports area, was 1.4 and 1.2°C; and 1.3 and 1.1°C in relation to linear park, respectively.
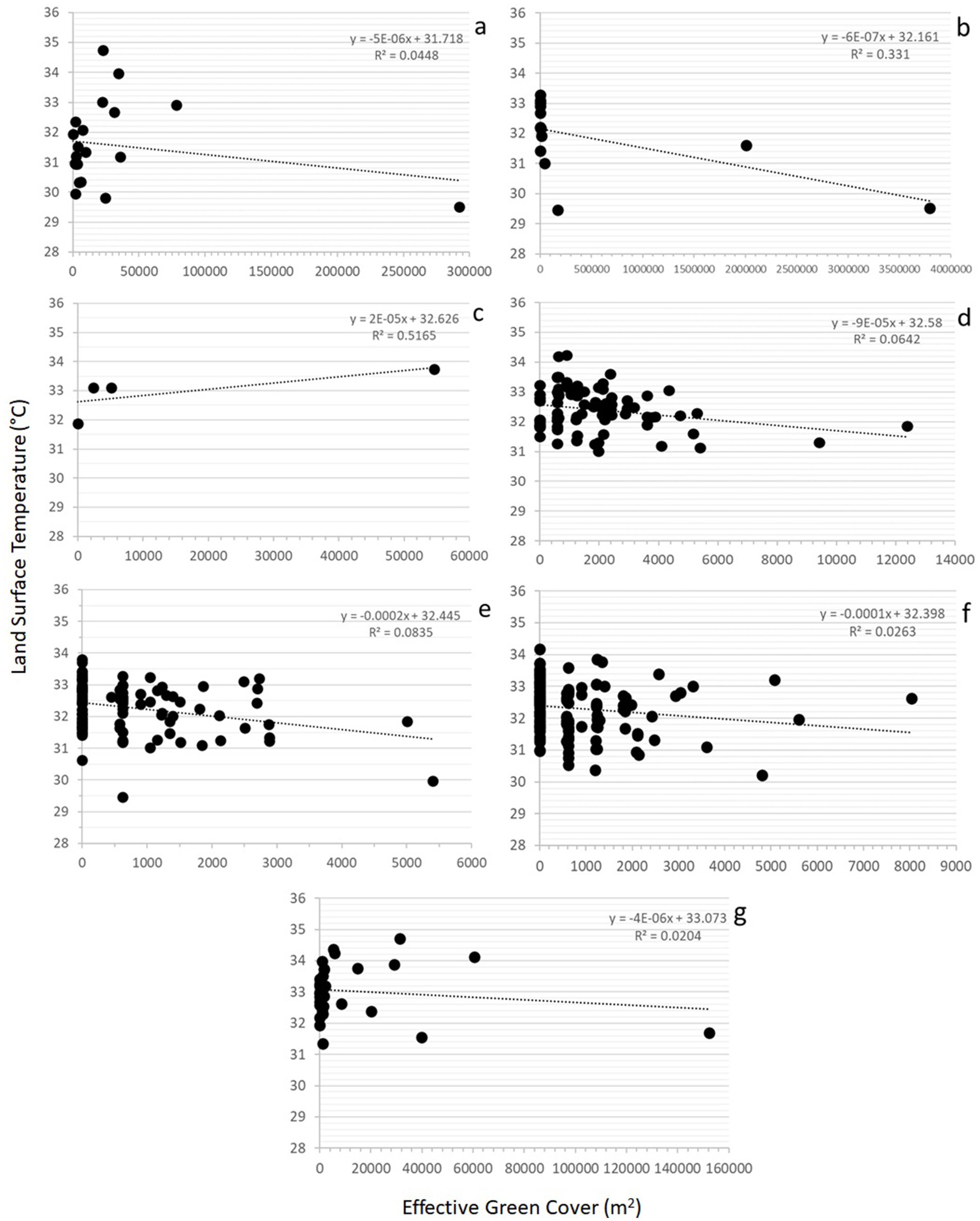
Figure 5. Linear regression between the land surface temperature (LST) and the effective green cover (EGC) in park with hydrological potential (A), urban park (B), linear park (C), Neighborhood park (D), local garden (E), residual green spaces (F), and sports area (G).
Another assessment was the relationship between LST and NDVI, where the value of R2 in the linear regression was 0.58 in urban park, indicating an indirect proportional relationship between such variables. In the other sites, the R2 coefficient was less than 0.01 and in local garden less than 0.1, so the model did not explain any portion of the response data variability. On the other hand, in linear park, the value of R2 was 0.9, representing a direct proportional relationship between the variables, which indicates an opposite effect of NDVI on LST (Figure 6). The green spaces with statistical significance were urban parks (F = 16.1, p = 0.001), local garden (F = 19.9, p = 0.000), and residual green spaces (F = 8.5, p = 0.004); although only in the first one, the residuals were symmetrical and its data are explained with the linear model.
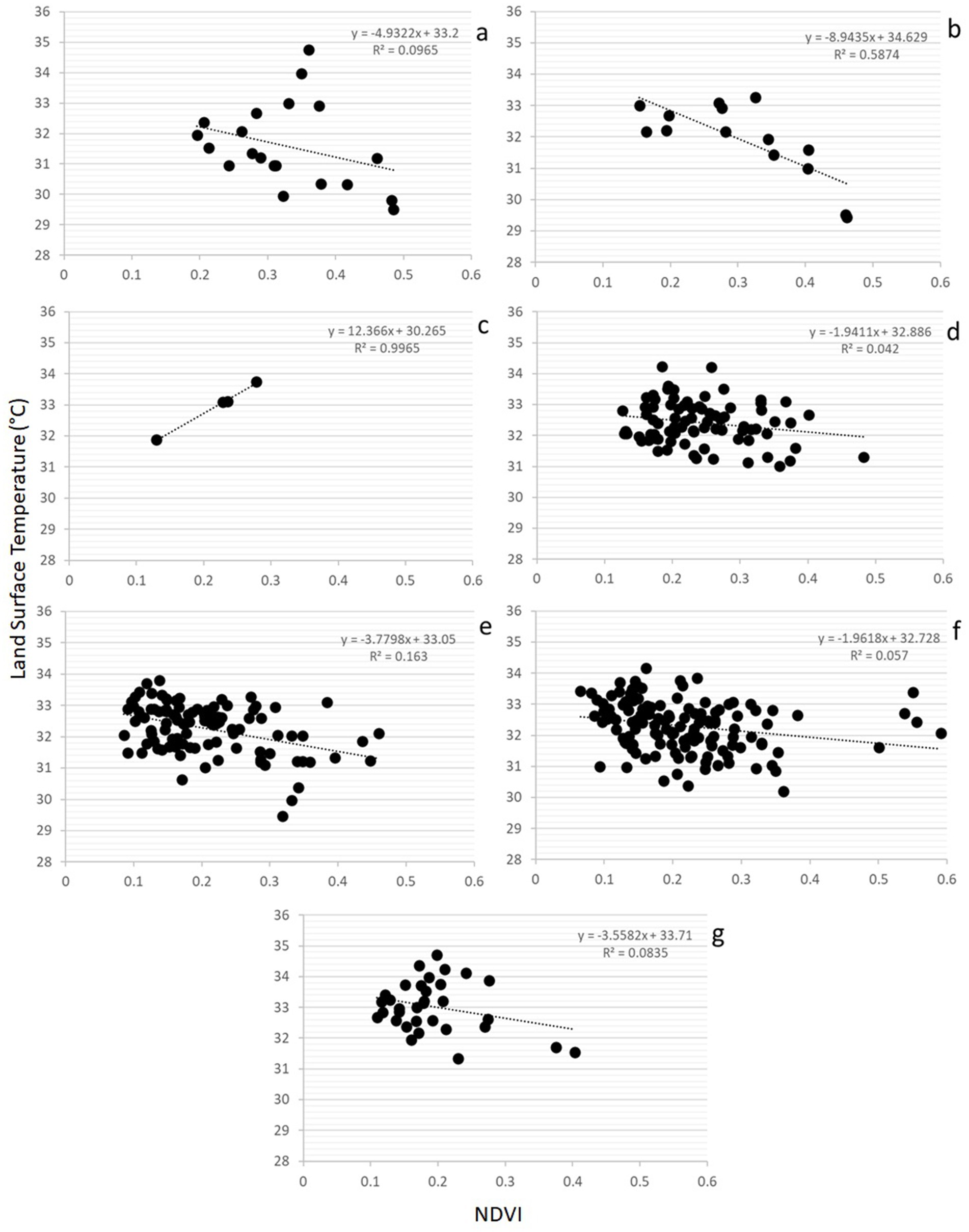
Figure 6. Linear regression between the land surface temperature (LST) and the Normalized Difference Vegetation Index (NDVI) in park with hydrological potential (A), urban park (B), linear park (C), Neighborhood park (D), local garden (E), residual green spaces (F), and sports area (G).
On the one hand, the proportion of the EGC was corresponding with the above-mentioned parameters. The highest values in the EGC percentage were 79.5 and 69.5% in parks with hydrological potential and urban park, with NDVI values of 0.32 and 0.3, respectively. On the other hand, the lowest values of the EGC percentage and NDVI were 34 and 0.19 in the sports area, respectively. Therefore, the lowest percentage values of PGS without green cover were 20.5 and 30.4% in parks with hydrological potential and urban parks, respectively, and the highest was 65% in sports area. Thus, the PGS in the metropolitan area exhibited an overall NDVI of 0.23 and an EGC average percentage of 55.1 and 44.4% of non-green cover. The urban park values were 5.3 and 4.8 m2 of total green cover per inhabitant and specific green cover per inhabitant, respectively. In contrast, in linear park, the lowest values were 0.06 and 0.05 m2 of total green area per inhabitant and specific green cover per inhabitant, respectively. In these two indicators, all PGS presented values below 1 m2, except for urban parks. Therefore, the PGS in the metropolitan area summed 6.7 and 5.8 m2 of total green area per inhabitant and specific green cover per inhabitant, respectively (Table 3).
3.2 Urban parks in the metropolitan areas: national and international perspective
Given the environmental relevance of the urban parks, their density and dimensions can be observed in metropolitan areas, with a population range between 1.0 and 1.3 million inhabitants, as in the studied city (Figure 7). In this group, generally, the density provided by large-sized urban parks was higher than the sum of medium- and small-sized parks. In contrast, the number of small parks was higher, followed by medium-sized parks, and finally by large parks in most urban systems. Despite this, no statistical relationship was found neither between the density and the number of parks nor between these variables and park size. According to these results, large-sized parks exhibited a higher density in comparison with the other two sizes in 15 of the 24 cities observed. In contrast, the metropolitan areas without large-sized parks were Da Nang, Vietnam; Dallas, USA; and Merida and Mexicali, both in Mexico. In addition, the density of medium-sized parks was higher than that of small-sized parks in 19 cities. The only metropolitan areas without medium-sized parks were Maceio, Brazil, and Zapopan, Mexico; and the lowest values were 2.8% in Mendoza, Argentina; and 2.7% in San Luis Potosí, Mexico. The latter city also had low density (1.6%) in small-sized parks, as well as Antwerp, Belgium (1.4%) and Gdansk, Poland (1.2%). In addition, considering the three sizes, the metropolitan areas with the lowest, medium, and highest urban park density were Mexicali, Mexico (M = 0.02, SD = 0.037), Aguascalientes, Mexico (M = 0.2, SD = 0.28), and New Orleans-Metairie, Louisiana, USA (M = 0.4, SD = 0.9), respectively. After this last metropolitan area, the one that exhibited the highest density was San Luis Potosi, Mexico (M = 0.37, SD = 0.9); nevertheless, it only reached 4.3% density when adding its medium- and small-sized parks. This value exhibits the 11 small- and 1 medium-sized parks that the metropolitan area has the reduced area of their polygons. Thus, the density in this city is mainly due to the two registered large-sized parks.
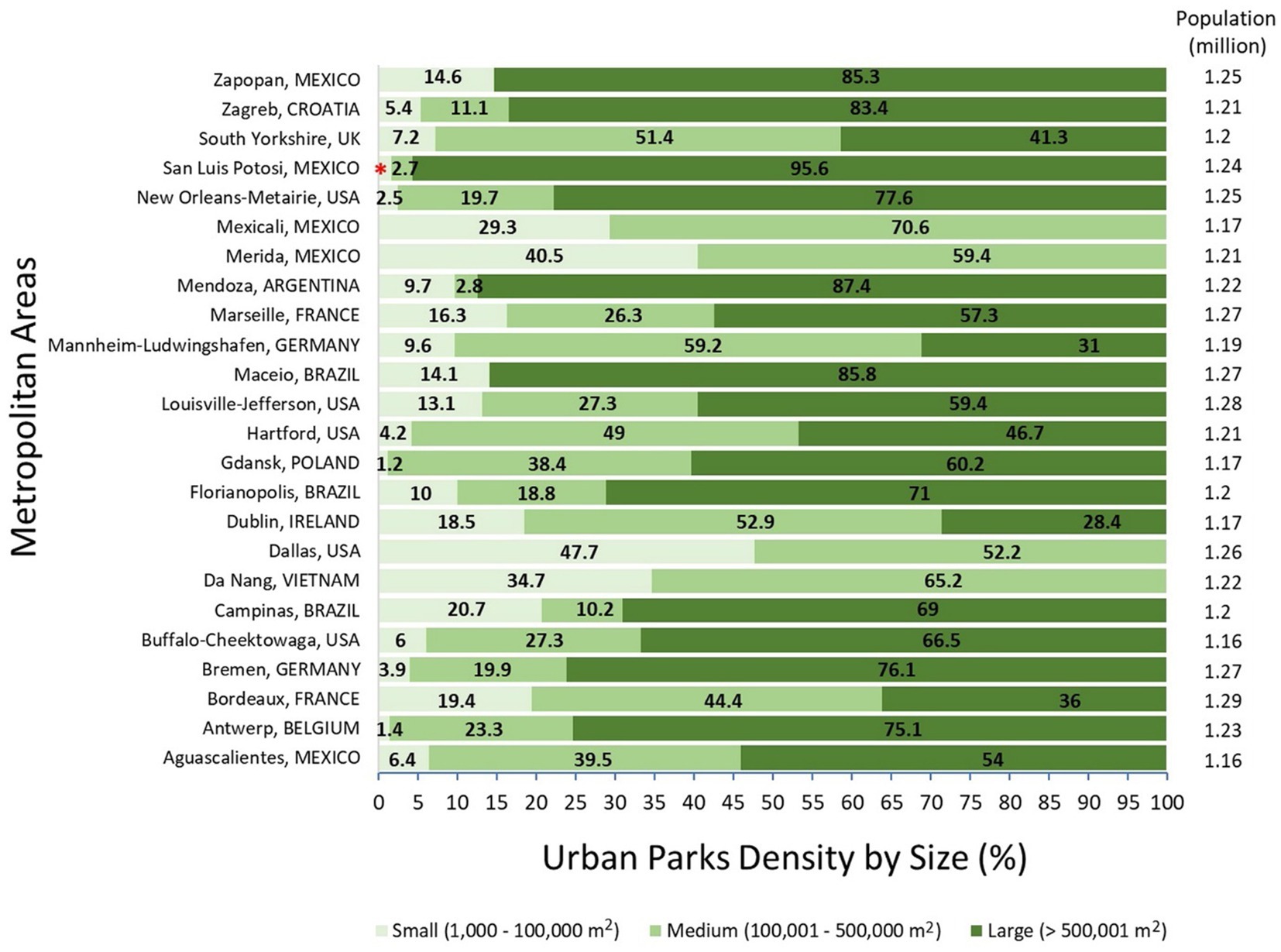
Figure 7. Density of urban parks per capita presented as a percentage, according to three size scales and the population size of 24 metropolitan areas of different latitudes. The right margin of the graph indicates the population size of each area, and the red asterisk corresponds to the 1.6% of small-sized parks in the metropolitan area of San Luis Potosí, Mexico.
3.3 Equipment and infrastructure attributes
Equipment–infrastructure response was differential among the different PGS. According to the score to evaluate these components, only three polygons in urban parks, one in linear parks, and one in sports area obtained a value of 10. In contrast, the highest value was 5 in three residual green space polygons and 7 in two parks with hydrological potential; however, these two PGSs were the ones with the lowest scores. The categories with intermediate scores were neighborhood park and local garden. In this way, according to the test of variance, three groups were differentiated in equipment–infrastructure: park with hydrological potential–residual green spaces, urban park–linear park–sports area, and neighborhood park–local garden (Figure 8A). Regarding the Wilcoxon rank sum test, equipment–infrastructure attributes score showed high variability.
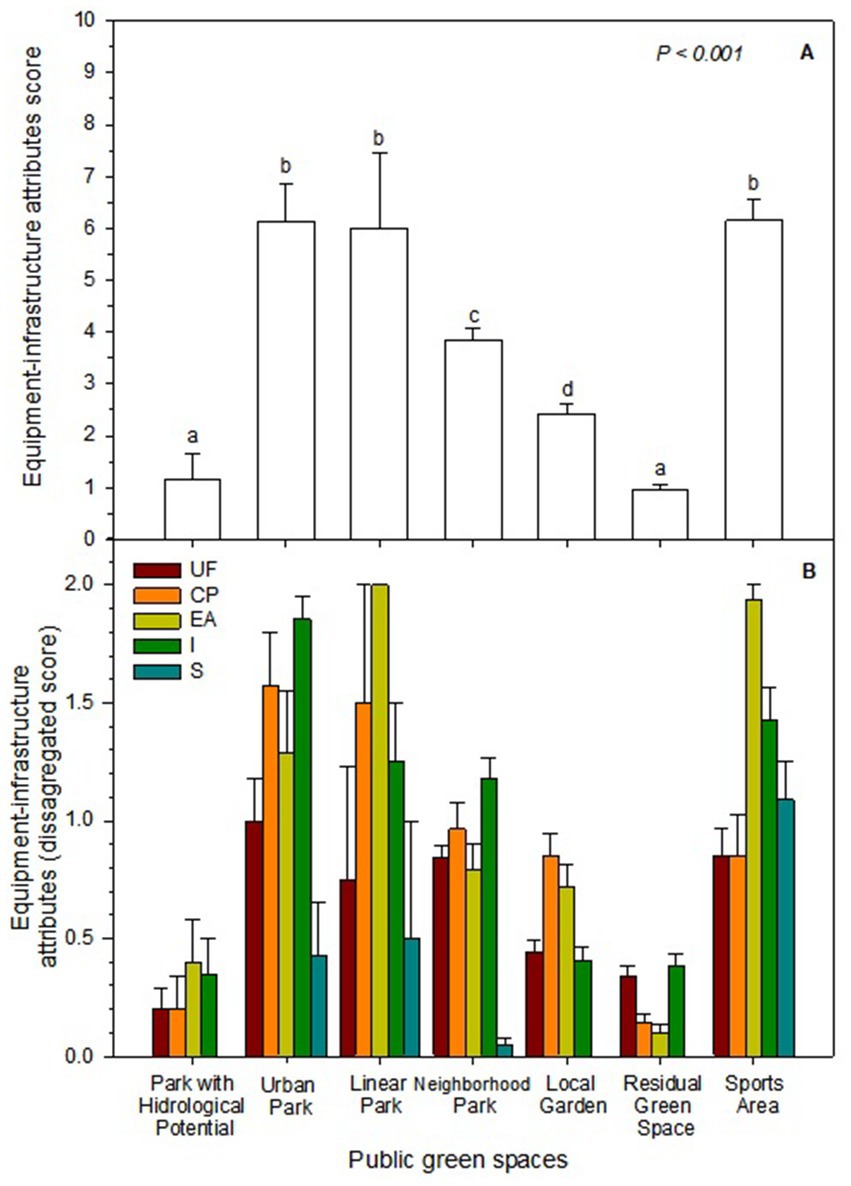
Figure 8. Equipment–infrastructure attributes per PGS category, separated into four different groups according to their statistical significance, indicated by a, b, c, and d (A). Disaggregated equipment–infrastructure attributes per PGS category, where the acronyms correspond to urban furniture (UF), children’s playground (CP), exercise area (EA), infrastructure (I), and services (S) (B).
Meanwhile, in Figure 8B, the disaggregated score exhibits the variability of attributes as well. The attributes with the most statistical significance P (<0.05) were street furniture, children’s playgrounds, and the exercise area. According to this statistical test, the major differences are indicated for each category and for the most outstanding PGS. The statistical significance occurred between green areas whose difference was wide in equipment values. In this direction, the green area with the highest urban furniture was urban park, and conversely, park with hydrological potential exhibited the lowest score. Children’s playgrounds in urban parks and linear parks had a high score, while in parks with hydrological potential and residual green spaces, the score was very low. On the other hand, it can be said that every green area of linear park and sports area contains an exercise area given its high score, while residual green spaces indicated the lowest score for equipment attribute. Regarding infrastructure attributes, the highest result was found in urban park and the lowest in park with hydrological potential and residual green spaces. Regarding the service attributes, the green area with the highest value was sports area, with low scores in the other PGS categories; however, no score was recorded in park with hydrological potential, local garden, and residual green spaces. Due to this result, the services in the sports area were the attributes that presented significant differences in comparison with the majority of the PGS categories. Therefore, the statistical significance was presented between PGS whose difference was wide, for example, park with hydrological potential and residual green spaces were below 0.5, while the values for urban park and sports area were greater than 1. Other PGS with differences in the attributes, but less contrasting, were linear park, neighborhood park, and local garden, in which some values were also below 1.
4 Discussion
The green spaces number was a parameter with notable differences for the PGS as a totality, and by category. According to this, it can be said that the PGS of the metropolitan area are numerous, but small in area. This is confirmed because residual green spaces, local garden, and neighborhood park encompass 82% of the studied green spaces, suggesting that green infrastructure is mainly composed of leftover surfaces from urban development and road works. The remaining 17% corresponds to urban park, park with hydrological potential, linear park, and sports area, which indicates that large-sized green areas are a minority in the city. Thus, the green infrastructure is composed of numerous small and residual spaces, in contrast to the few large and planned green areas. This represents uneven planning in the number and types of green spaces in the city. A large number of studies indicate that these spaces should preferably be either of a larger area or, if they have a small area, they should be numerous and with high plant density (Breuste et al., 2008; Pickett and Cadenasso, 2008; Nor et al., 2017; Zhang et al., 2019).
4.1 Environmental and ecological parameters in public green spaces
According to the linear regression analysis, the vegetation cover quality increased in proportion to the EGC in parkspark with hydrological potential, urban park, local garden, and sports area; though it cannot be stated conclusively. Even though the larger-sized green spaces were park with hydrological potential and urban park, the points cloud in the graphs were not completely linearly distributed, although their regression models have shown the best data fit and symmetry of residuals. Despite this, park with hydrological potential and urban park resulted in the highest average NDVI values. In linear park, it is not possible to define correspondence between the variables, as there are few observed data and the shape of the polygon can only be longitudinal in this type of green area. Another category whose linear regression model showed proportionality between NDVI and EGC was neighborhood park, albeit the results cannot be conclusive. Despite showing acceptable values in the regression model, the data in the graph do not align to indicate a strong association between the variables. On the other hand, sports area was a space with favorable results in the linear regression; nonetheless, it is important to clarify that the EGC can be permeable surfaces of bare soil detected in the GIS. In the studied city, sports areas frequently contain non-green surfaces such as sports fields and areas without grass, rather than places with large and dense vegetated cover.
In addition to the weak association between the NDVI and EGC variables in each category, total green infrastructure, with an NDVI value of 0.23, can be considered low with respect to estimations in different cities. For example, in Sheffield, South Yorkshire, England, the proportionality was direct between the average NDVI value and the urban green area portion, in a range of 0.05 and 6 ha (Davies et al., 2008). In another study, 10- to 60-hectare urban parks reported NDVI values between 0.2 and 0.4 in Ankara, Turkey (Bilgili et al., 2013). In addition, in Bucharest and Athens, smaller green areas showed values of 0.5 and 0.3, respectively, while larger ones—such as parks—reached NDVI values of 0.6 (Popa et al., 2022).
In green spaces, the vegetated cover quality and the size are two variables, whose relationship is clear, and which have to be enhanced in the studied city. This is especially important because the NDVI is a crucial indicator to determine the photosynthetic activity of vegetation, which is of great value from the urban and landscape ecology perspective. Moreover, Chen et al. (2018) used NDVI to map the social functions of urban green spaces in the city of Beijing in China. This is the reason why this work aims at increasing the literature of PGS and their current status in San Luis Potosí, as a means of assisting the governmental entities in effective urban planning.
Another variable estimated—based on the specific green portion in each category—was land surface temperature (LST). Despite this, the association between variables was not decisive in establishing the decrease in LST due to this cause. Although the R2 value in urban park was the highest, in neighborhood park and local garden, it exhibited the lowest values, as data did not reflect a good fit to the regression model. Regarding the linear park, the adjusted line showed a contrary association between the variables; thus, it is likely that the LST was more influenced by the adjacent conditions, than by the effect of the green cover in its polygons. Therefore, the decrease in LST as a result of an increase in EGC is not conclusive, although the urban park showed a moderate effect when the area of the polygon increased. In turn, the categories of urban park and park with hydrological potential showed lower average LST in their polygons, which were larger and more densely vegetated. In this manner, this corresponds to several studies, in which the cooling effect is evident, as city’s green spaces offer a greater area (Lin et al., 2015; Zhu et al., 2021; Zhang et al., 2022). In addition, green spaces may have a more significant cooling effect, if their vegetated cover increases (Gomez-Martinez et al., 2021); this is also true for small green areas, due to the great influence that the surrounding built space represents (Liu et al., 2022).
As the coverage variation in green areas is a factor of significant environmental influence, the degree of association between NDVI and LST allowed us to analyze the performance of each PGS category. When evaluating the LST as a function of NDVI, only urban park was statistically significant in the regression model. A similar trend was observed in the park with hydrological potential, but it exhibited several points away from the adjusted line. Even though this last green space showed polygons of great green coverage, their forms were irregular and longitudinal. In the other green spaces, it was observed that the distribution of points is closer to the adjusted line, but the model predictors did not allow to establish any relationship between the variables. In linear park, the response was contrary to the expected relationship; in other words, it is likely that its low plant density does not counteract the high warming of the surrounding urban space, detected by image processing. Therefore, the category that indicated a clear effect of decreasing LST due to the quality of its green cover was the urban park. Several studies have reported a negative correlation between LST values and NDVI, especially in urban park (Feyisa et al., 2014; Yu et al., 2017; Xiao et al., 2020). Finally, the surface thermal response was influenced by the greenness level, but the evidence is stronger in larger green spaces. In addition, due to their characteristics and properties, it is likely that only urban park and park with hydrological potential can provide mitigation of the air temperature around them. Lemoine-Rodríguez et al. (2022) point out that green spaces larger than two hectares can decrease air temperature by approximately 2°C. Similarly, the cooling effect can be noticeable in green spaces of 2.8 hectares or more, with at least 21% of tree cover.
The area of the polygon and the greenery degree determined the net vegetated cover. Regarding the first parameter, the area without vegetated cover was high in local garden, residual green spaces, and sports area, while the percentage of EGC dominated in park with hydrological potential, urban park, and neighborhood park. Even though the deficit in the first three above-mentioned green spaces is more important, any category could contain a higher percentage of EGC, as indicated by the average total values in Table 3. In this context, it is interesting to determine what is the ideal green fraction that each of the PGS categories should have. Although this may be a research topic of great interest, its study does not correspond to the objective of this work. In general, PGS require more ecological management, especially conservation strategies in parks with hydrological potential. For example, Zingraff-Hamed et al. (2022) indicate that these spaces, named Urban River Parks, can improve ecosystem values and reduce water scarcity in cities.
In terms of the green area density as a function of the number of inhabitants, the resulting value of the urban park was higher than an order of magnitude in each of the other categories. In other words, urban parks represent the dominant type of green space in the city. For example, if the area of the largest urban park called “Tangamanga I” were eliminated, the total green cover value would be 3.4 m2, whereas the EGC value would be 2.8 m2 per inhabitant. Consequently, the density of green area per inhabitant is dependent on urban parks. In addition, as social use includes the non-green portion of the polygons, the density of green infrastructure in the city can be considered to correspond to the value of 6.7 m2 of total green cover. This result is distant from the minimum parameter of 9 m2 of green area per inhabitant according to the WHO (2016). Whereas this is the benchmark parameter for most cities, in Europe, the maximum value of this indicator is 50 m2 per inhabitant, which is easily achieved and overcome, depending on their population density (European Commission, 2015). Other regions, such as East Asia, are also presenting important values, such as the city of Xi’an with 27.8 m2 per inhabitant in China (Pang et al., 2023). On the other hand, in Latin American cities, green space values are reported below 9 m2 where its distribution is mainly inequitable and inaccessible in different areas of the city (Sorensen et al., 1998; Reyes-Päcke and Figueroa-Aldunce, 2010; Gómez and Velázquez, 2018). Similarly, cities in Mexico reach an average value of 8.2 m2 of green area per inhabitant (UN-Habitat, 2019). Even if the capital, Mexico City, has 14.4 m2 of green space per inhabitant, it is not equitable among its municipalities, and its calculation may include private areas and other sites, that are not necessarily green infrastructure (PAOT, 2010). However, the calculation of the green areas’ density requires a precise analysis due to differences in the criteria for estimating green portions in a city or at least a method for assessing green spaces by category (Badiu et al., 2016). In this regard, López Torrero and Navarro Navarro (2023) point out that the determination of this indicator is variable according to the nature and conduction of the study and report a value of 2.14 m2/inhabitant of green space for the city of Hermosillo, Mexico (López Torrero and Navarro Navarro, 2023). Nevertheless, this was the same value obtained in the study by the Inter-American Development Bank, Harvard University, and the Municipal Institute of Urban Planning and Public Space for the same city (IDB, Harvard University and IMPLAN, 2017). In another semi-arid city in the country, Durango, the density of green spaces was 3.7 m2 per inhabitant (Blancarte-Siqueiros et al., 2019). Given this scenario, the value of 6.7 m2 of green space that resulted in the city of San Luis Potosí is higher than the mentioned cities in the country, albeit it is important to emphasize the difference in the methods to determine the calculation. In the UN-HABITAT report (2019), the values of these three Mexican cities are not the same as those reported in the above-mentioned studies, but they do support the low values of green area per inhabitant, and congruently highlight the density pattern of these cities.
It is important to highlight that there are few studies regarding this indicator in semi-arid cities globally (Meerow et al., 2021), few studies related to green areas in Latin American cities (Breen et al., 2020; Bille et al., 2023), and even fewer which study the vegetated cover in this context. An example of decreased proportion of vegetated cover in green spaces is found in Santiago de Chile, Chile, where the variable observed values of less than 5% in three districts, which equals to 4,6 m2 per inhabitant in just one of them (de la Barrera et al., 2016). Thus, the resulting indicator of the present study can be added to the scarce reports of semi-arid cities, compared to abundant literature on cases of green infrastructure in cities located in high latitudes and temperate environments, as Parker and Zingoni de Baro (2019) indicate. In addition, it has been reported that in semi-arid cities the inequity of green areas is even stronger (Chamberlain et al., 2020), as it can also be observed in Figure 1.
4.2 Urban parks in the metropolitan areas: national and international perspective
In addition, the density, number, and size of parks in the observed urban systems imply important differences in the planning of such green spaces. The cities that exhibited the highest sample variation were Mendoza, Argentina; San Luis Potosí, Mexico; and New Orleans-Metairie, USA; that is to say, where density showed wide differences between the three park sizes of each metropolitan area. While the contribution was 5 m2 of large-sized urban park per inhabitant in San Luis Potosi, the density of small- and medium-sized parks was less than 1 m2 per inhabitant in both cases. Therefore, it can be said that the availability of public green space for the population is limited exclusively to the large-sized parks offered by the city, which has important social and maintenance implications.
Just as the EGC can be a measure that specifies the vegetation composition of each polygon, the density of urban parks allows us to evaluate them in relation to their dimensions. In the present study, the analysis between these variables did not show a significant statistical relationship, as the sample size of large, medium, and small parks was very low. However, they highlight the differences in the size and number of parks in the metropolitan area under study. For this reason, a sustainable planning idea would be the increase of medium-sized parks and the incorporation of at least another large park for the city. In the literature, parks with at least 2 hectares acquire great acceptance by visitors, especially if they present connectivity between them by means of pedestrian walkways (Coles and Bussey, 2000). Moreover, large green spaces such as urban parks are more likely to provide ecosystem services. For example, for a study on urban landscape metrics, green spaces of approximately 10 ha were more favorable for carbon storage and the proliferation of pollinating organisms (Grafius et al., 2018).
Although this ecological connectivity parameter was not the objective of the present study, the density and size of the green spaces suggest a first step for future landscape ecology studies. A possible topic to be developed is to evaluate the increase in the green density of local garden and neighborhood park to facilitate connectivity between large urban park and park with hydrological potential. In a green infrastructure study, urban river and forest areas indicated greater functionality and degree of naturality than urban parks, especially with community gardens and smaller green areas in the middle-sized city of Zaragoza, Spain (Hanna et al., 2023). A salient theme of ecological connectivity is that of functional form within urban areas (LaPoint et al., 2015; Schütz et al., 2017), in addition to including abiotic factors such as temperature or the water issue for future connectivity projects (Lookingbill et al., 2022). Therefore, the shape, size, and distribution of the polygons—as well as their vegetation density and the presence of water bodies—are important parameters, when analyzing every PGS category. This approach is relevant because of the green infrastructure buffering against the semi-arid climate and also because San Luis Potosi is a growing city. This is critical, because after large cities on the global scale, medium-sized cities are expected to have high growth (UN-Habitat, 2022), and a decrease in their ecological values (Parivar et al., 2021; Okour and Shaweesh, 2024).
4.3 Equipment and infrastructure attributes
In addition to their environmental and landscape properties, the green spaces of the city under study presented variations in equipment–infrastructure attributes. It makes sense that urban parks, linear parks, and sports areas have shown higher values than other PGS, due to the functions that the former fulfill. Except for the linear park, the areas of the PGS polygons allow sports, cultural and entertainment activities, and offer high social value, as they have the capacity to receive numerous visitors. Urban parks can have high-quality standards by offering relaxation, leisure, psychological, emotional, and social benefits (Chiesura, 2004; Taylor et al., 2020), and thus, elements of equipment and infrastructure become important. In the case of sports areas, whose score in the category of services was the highest, it can be justified by its public function. In contrast, the decrease in equipment–infrastructure in the neighborhood park and local garden reflects less spatial capacity but also because these PGS are designed for a local target audience. Notwithstanding, there are certain factors that explain the differences between these last two green spaces. As the neighborhood park is a larger green space than the local garden, it acquires greater capacity for visits and also equipment and, in some cases, services. Even if the local garden meets the needs of a smaller group of people, site visits showed that residents are actively involved in its maintenance and care.
On the other hand, the sites with less equipment corresponded to contrasting green spaces such as park with hydrological potential and residual green spaces. This last green space stood out for the small area of its polygons and being surrounded by roads, which may explain its lack of equipment. Additionally, if they have some attributes, these are very scarce or sometimes host improvised elements by citizens. As for the park with hydrological potential, the only values found correspond to a short stretch of the Españita River equipped with a children’s playground and sports fields. Even though only a fragment of the river provides this type of equipment, all the other parks with hydrological potential are not officially declared as such or recognized as green areas. As it is a green space with riparian vegetation, it generally does not have the infrastructure of an urban park. Nonetheless, the form, vegetation, and functions are important factors for these spaces with nature to be proposed and included in the green infrastructure. Even though they are areas of intermittent rivers, with the presence of water flow in the few rainy months, their location on the city limits could encourage projects to rescue urban rivers. This is expected to promote ecological and hydrological conservation, bringing together the natural properties of a river and the benefits of a green space with a certain degree of equipment and social use. The quantification of urban equipment and infrastructure in different green spaces is important due to their social implications, influence on visitors and presence in the city (Zheng et al., 2023), their use for physical activities (Stewart et al., 2018), and perception of safety (Min et al., 2022). Despite the fact this study does not analyze the quality of equipment and infrastructure, it would be important to consider it in future studies, as this could influence users’ attendance in green spaces (Englund and Thorpert, 2021). Therefore, the degree of equipment and infrastructure was quantitatively variable according to the PGS type, which determines the activities offered.
5 Conclusion
Given the question posed by the current study, it could be said that a larger green space increases environmental benefits; however, only in urban parks this aspect showed significant results. One of these benefits was the mitigation of surface temperature by increasing the NDVI indicator in urban park, despite the reduced number of such spaces in the metropolitan area. Regarding the vegetated cover quality, urban park, and park with hydrological potential were highlighted, as these can potentially offer valuable environmental, as well as ecosystem services. This suggests that parks with hydrological potential should be legally considered as green infrastructure; thus, through ecological conservation, this PGS category could reinforce the natural water system. Although the other green spaces showed a lower environmental performance, it can be said that their conditions could improve, if their vegetation cover increases in density and quality.
Accordingly, EGC suggests evaluating the net green composition according to the different kinds of urban green spaces. While the amount of green space was larger than non-green cover in urban parks and parks with hydrological potential, this proportion was inverse in local gardens, residual green spaces, and sports areas. For this reason, the proposed parameter—EGC allowed assessing the net plant density, discarding the built components in each green space.
Another key outcome is the composition of green infrastructure of the city, which encompasses many small sites, few large-sized urban parks, and only one medium-sized park. Our results indicated a total value of 6.7 m2 total green cover and 5.8 m2 EGC in the metropolitan area of San Luis Potosí, Mexico. Thus, the contribution of large-sized urban parks was so significant that, without Tangamanga 1 and 2, the indicator would be 3.4 m2 per inhabitant. In any case, the first value is lower than the minimum of 9 m2 per capita recommended by WHO; and thus, insufficient when compared to other metropolitan areas, at national and international scales. Among the metropolitan areas studied, San Luis Potosí displayed the greatest variation in park dimensions, with up to 5 m2 of large-sized parks density, but less than 1 m2 of combined medium- and small-sized parks per resident. Therefore, while urban parks are the elements with the highest environmental value, only large ones are the green areas with the highest availability for the population.
Nevertheless, the green spaces with the most significant contribution of equipment–infrastructure attributes were urban park, linear park, and sports area in contrast to park with hydrological potential, local garden, and residual green spaces. Green spaces have a high added value due to their potential to develop a variety of social activities, and this can increase with infrastructure–equipment, especially for less-favored sites such as smaller ones.
This study highlights the importance of considering the EGC as a parameter which can determine the vegetated cover deficit in terms of ecological functions. However, the planning and design of these areas face latent challenges, such as rapid urban growth, lack of territorial planning, scarce implemented projects, and low economic investment, among others. Public green space planning could not only reach the WHO’s minimal recommended standard but also ensure the provision of ecosystem services. In addition, another important aspect is the limited number of such studies in urban systems located in semi-arid climate regions, which are central to guiding public policies. The current Urban Development Plan does not consider the green capital and its deficiencies as a problem, and thus improvements in their maintenance and revegetation are not even in discussion. A plantation strategy for the non-vegetated areas inside the PGS is to use tree species, which are not only adapted to semi-arid conditions but also provide ecosystem services to the metropolitan area. Finally, the urban warming of the growing metropolitan area, the semi-arid condition of the region, and the drought phenomena in the north-central part of the country will be addressed not only with the improvement of existing green infrastructure but also with a significant increase of urban green spaces.
Data availability statement
The raw data supporting the conclusions of this article will be made available by the authors, without undue reservation.
Author contributions
CR-P: Investigation, Project administration, Supervision, Writing – original draft, Writing – review & editing, Conceptualization, Data curation, Formal analysis, Methodology, Resources, Validation, Visualization. MB-E: Data curation, Formal analysis, Investigation, Methodology, Project administration, Validation, Writing – original draft, Writing – review & editing, Software. CB-R: Formal analysis, Methodology, Supervision, Writing – review & editing, Investigation, Writing – original draft. AC-V: Formal analysis, Investigation, Methodology, Project administration, Validation, Writing – review & editing, Writing – original draft, Supervision. JR-H: Formal analysis, Methodology, Supervision, Writing – review & editing, Data curation, Software.
Funding
The author(s) declare that no financial support was received for the research, authorship, and/or publication of this article.
Acknowledgments
The authors would like to acknowledge the CONAHCYT institution for funding two master’s scholarships: 745471 and 750314. They would also like to express their gratitude to Andrés Gerardo Castro Ovalle, Carolina Suvea Calvillo, Leonardo Daniel García Torres, and Heidi Cedeño Gilardi for their valuable help in different stages of the study method.
Conflict of interest
The authors declare that the research was conducted in the absence of any commercial or financial relationships that could be construed as a potential conflict of interest.
Publisher’s note
All claims expressed in this article are solely those of the authors and do not necessarily represent those of their affiliated organizations, or those of the publisher, the editors and the reviewers. Any product that may be evaluated in this article, or claim that may be made by its manufacturer, is not guaranteed or endorsed by the publisher.
References
Armson, D., Stringer, P., and Ennos, A. R. (2012). The effect of tree shade and grass on surface and globe temperatures in an urban area. Urban For. Urban Green. 11, 245–255. doi: 10.1016/j.ufug.2012.05.002
Badiu, D. L., Iojă, C. I., Pătroescu, M., Breuste, J., Artmann, M., Niță, M. R., et al. (2016). Is urban green space per capita a valuable target to achieve cities’ sustainability goals? Romania as a case study. Ecol. Indic. 70, 53–66. doi: 10.1016/j.ecolind.2016.05.044
Barradas, V. L., Miranda, J. A., Esperón-Rodríguez, M., and Ballinas, M. (2022). (Re)designing urban parks to maximize urban Heat Island mitigation by natural means. Forests 13:1143. doi: 10.3390/f13071143
Bartesaghi-Koc, C., Osmond, P., and Peters, A. (2017). Towards a comprehensive green infrastructure typology: a systematic review of approaches, methods and typologies. Urban Ecosyst. 20, 15–35. doi: 10.1007/s11252-016-0578-5
Bascuñán-Walker, F., Walker-Fernández, P., and Mastrantonio-Freitas, J. (2007). Modelo de cálculo de áreas verdes en planificación urbana desde la densidad habitacional. Urbano 10, 97–101.
Bilgili, B. C., Şatır, O., and Müftüoğlu, V. (2013). Using NDVI values for comparing parks in different scales. J. Food Agric. Environ. 11, 2451–2457.
Bille, R. A., Jensen, K. E., and Buitenwerf, R. (2023). Global patterns in urban green space are strongly linked to human development and population density. Urban Forestry Urban Green. 86:127980. doi: 10.1016/j.ufug.2023.127980
Blancarte-Siqueiros, R. H., Perez-Verdin, G., and Cortes-Ortiz, A. (2019). The relationship between quality of life, sense of belonging, and green spaces in urban environments in the city of Durango, Mexico. Rev. Chap. Serie Cienc. Forest. Amb. 26, 97–111. doi: 10.5154/r.rchscfa.2019.03.023
Bolund, P., and Hunhammar, S. (1999). Ecosystem services in urban areas. Ecol. Econ. 29, 293–301. doi: 10.1016/S0921-8009(99)00013-0
Breen, A., Giannotti, E., Flores Molina, M., and Vásquez, A. (2020). From “government to governance”? A systematic literature review of research for urban green infrastructure Management in Latin America. Front. Sustain. Cities 2:572360. doi: 10.3389/frsc.2020.572360
Breuste, J., Artmann, M., Li, J., and Xie, M. (2015). Special issue on green infrastructure for urban sustainability. J. Urban Plann. Dev. 141, 1–5. doi: 10.1061/(ASCE)UP.1943-5444.0000291
Breuste, J., Niemelä, J., and Snep, R. P. H. (2008). Applying landscape ecological principles in urban environments. Landsc. Ecol. 23, 1139–1142. doi: 10.1007/s10980-008-9273-0
Brinkhoff, T. (2023) City population. Available at: http://www.citypopulation.de (Accessed June 13, 2023).
Brzoska, P., and Spāģe, A. (2020). From City- to site-dimension: assessing the urban ecosystem Services of Different Types of green infrastructure. Land 9:150. doi: 10.3390/land9050150
Byrne, J., and Sipe, N. (2010). Green and open space planning for urban consolidation - a review of the literature and best practice. Urban Res. Porgr. 11, 6–9.
Calaza-Martínez, P. (2016). Infraestructura verde Sistema natural de salud pública. Spain: Ediciones Mundi-Prensa.
Chamberlain, D., Reynolds, C., Amar, A., Henry, D., Caprio, E., and Batáry, P. (2020). Wealth, water and wildlife: landscape aridity intensifies the urban luxury effect. Glob. Ecol. Biogeogr. 29, 1595–1605. doi: 10.1111/geb.13122
Chen, W., Huang, H., Dong, J., Zhang, Y., Tian, Y., and Yang, Z. (2018). Social functional mapping of urban green space using remote sensing and social sensing data. ISPRS J. Photogramm. Remote Sens. 146, 436–452. doi: 10.1016/j.isprsjprs.2018.10.010
Chiesura, A. (2004). The role of urban parks for the sustainable city. Landsc. Urban Plan. 68, 129–138. doi: 10.1016/j.landurbplan.2003.08.003
Coles, R. W., and Bussey, S. C. (2000). Urban forest landscapes in the UK—progressing the social agenda. Landsc. Urban Plan. 52, 181–188. doi: 10.1016/S0169-2046(00)00132-8
Davies, R. G., Barbosa, O., Fuller, R. A., Tratalos, J., Burke, N., Lewis, D., et al. (2008). City-wide relationships between green spaces, urban land use and topography. Urban Ecosyst. 11, 269–287. doi: 10.1007/s11252-008-0062-y
de la Barrera, F., and Henríquez, C. (2017). Vegetation cover change in growing urban agglomerations in Chile. Ecol. Indic. 81, 265–273. doi: 10.1016/j.ecolind.2017.05.067
de la Barrera, F., Reyes-Paecke, S., and Banzhaf, E. (2016). Indicators for green spaces in contrasting urban settings. Ecol. Indic. 62, 212–219. doi: 10.1016/j.ecolind.2015.10.027
Dobbs, C., Escobedo, F. J., and Zipperer, W. C. (2011). A framework for developing urban forest ecosystem services and goods indicators. Landsc. Urban Plan. 99, 196–206. doi: 10.1016/j.landurbplan.2010.11.004
Englund, J.-E., and Thorpert, P. (2021). Colour perception and visitor experiences of urban parks as influenced by an intense Colourful urban artefact: An in-situ quasi-experiment. Iconarp Int. J. Archit. Plann. 9, 485–505. doi: 10.15320/ICONARP.2021.169
European Commission (2015) European cities, territorial analysis of characteristics and trends – An application of the LUISA modelling platform (EU reference scenario 2013 - updated configuration 2014). Available at: https://data.europa.eu/doi/10.2788/737963.
Feyisa, G. L., Dons, K., and Meilby, H. (2014). Efficiency of parks in mitigating urban heat island effect: An example from Addis Ababa. Landsc. Urban Plan. 123, 87–95. doi: 10.1016/j.landurbplan.2013.12.008
Geneletti, D., Cortinovis, C., Zardo, L., and Adem Esmail, B. (2020). “Towards equity in the distribution of ecosystem services in cities” in Planning for ecosystem services in cities. SpringerBriefs in environmental science (Cham: Springer).
Georgi, N. J., and Zafiriadis, K. (2006). The impact of park trees on microclimate in urban areas. Urban Ecosyst. 9, 195–209. doi: 10.1007/s11252-006-8590-9
Goličnik-Marušić, B. (2015). Social behaviour as a basis for design and development of green infrastructure. Urbaniizziv 26:46. doi: 10.5379/urbani-izziv-en-2015-26-supplement-009
Gómez, N. J., and Velázquez, G. A. (2018). Asociación entre los espacios verdes públicos y la calidad de vida en el municipio de Santa Fe, Argentina. Cuadern. Geogr. 27, 164–179. doi: 10.15446/rcdg.v27n1.58740
Gomez-Martinez, F., de Beurs, K. M., Koch, J., and Widener, J. (2021). Multi-temporal land surface temperature and vegetation greenness in urban green spaces of Puebla, Mexico. Land 10:155. doi: 10.3390/land10020155
Grafius, D. R., Corstanje, R., and Harris, J. A. (2018). Linking ecosystem services, urban form and green space configuration using multivariate landscape metric analysis. Landsc. Ecol. 33, 557–573. doi: 10.1007/s10980-018-0618-z
Haaland, C., and van den Bosch, C. K. (2015). Challenges and strategies for urban green-space planning in cities undergoing densification: a review. Urban For. Urban Green. 14, 760–771. doi: 10.1016/j.ufug.2015.07.009
Hanna, E., Bruno, D., and Comín, F. A. (2023). Evaluating naturalness and functioning of urban green infrastructure. Urban For. Urban Green. 80:127825. doi: 10.1016/j.ufug.2022.127825
IDB, Harvard University and IMPLAN (2017) Rethinking Hermosillo. Emergent sustainable cities. Available at: https://www.implanhermosillo.gob.mx/wp-content/uploads/2018/08/Rethinking-Hermosillo-2017_09_27-Spanish-1.pdf (Accessed February 16, 2024).
Ihlen, V., and Zanter, K. (2019). Landsat 8 (L8). Data users handbook. Version 5.0. South Dakota, USA: Sioux Falls, SD.
INEGI (2022) Sistema de Consulta de Información Censal (SCINCE), Instituto Nacional de Estadística y Geografía (INEGI). Available at: https://gaia.inegi.org.mx/scince2020/ (Accessed June 16, 2024).
Jayasooriya, V. M., Ng, A. W. M., Muthukumaran, S., and Perera, B. J. C. (2017). Green infrastructure practices for improvement of urban air quality. Urban For. Urban Green. 21, 34–47. doi: 10.1016/j.ufug.2016.11.007
Ju, Y., Dronova, I., and Delclòs-Alió, X. (2022). A 10 m resolution urban green space map for major Latin American cities from Sentinel-2 remote sensing images and OpenStreetMap. Sci. Data 9:586. doi: 10.1038/s41597-022-01701-y
Kargar, R., and Ghassemi, M. (2016). Urban furniture and leisure of citizens (case study of Sabzevar urban parks). J. Civil Eng. Urban. 6, 40–47.
LaPoint, S., Balkenhol, N., Hale, J., Sadler, J., and van der Ree, R. (2015). Ecological connectivity research in urban areas. Funct. Ecol. 29, 868–878. doi: 10.1111/1365-2435.12489
Lemoine-Rodríguez, R., Inostroza, L., Falfán, I., and MacGregor-Fors, I. (2022). Too hot to handle? On the cooling capacity of urban green spaces in a Neotropical Mexican city. Urban For. Urban Green. 74:127633. doi: 10.1016/j.ufug.2022.127633
Lin, W., Yu, T., Chang, X., Wu, W., and Zhang, Y. (2015). Calculating cooling extents of green parks using remote sensing: method and test. Landsc. Urban Plan. 134, 66–75. doi: 10.1016/j.landurbplan.2014.10.012
Liu, Y., Xu, X., Wang, F., Qiao, Z., An, H., Han, D., et al. (2022). Exploring the cooling effect of urban parks based on the ECOSTRESS land surface temperature. Front. Ecol. Evol. 10:1031517. doi: 10.3389/fevo.2022.1031517
Lookingbill, T. R., Minor, E. S., Mullis, C. S., Nunez-Mir, G. C., and Johnson, P. (2022). Connectivity in the urban landscape (2015–2020): who? Where? What? When? Why? And How? Curr. Landsc. Ecol. Rep. 7, 1–14. doi: 10.1007/s40823-021-00068-x
López Torrero, J. C., and Navarro Navarro, L. A. (2023). Inventario de parques urbanos para el cumplimiento de la Agenda 2030: el caso de Hermosillo, Sonora. Frontera Norte 35, 1–25. doi: 10.33679/rfn.v1i1.2307
Mao, Q., Wang, L., Guo, Q., Li, Y., Liu, M., and Xu, G. (2020). Evaluating cultural ecosystem Services of Urban Residential Green Spaces from the perspective of residents’ satisfaction with green space. Front. Public Health 8:226. doi: 10.3389/fpubh.2020.00226
McPhearson, T., Pickett, S. T. A., Grimm, N. B., Niemelä, J., Alberti, M., Elmqvist, T., et al. (2016). Advancing urban ecology toward a science of cities. Bioscience 66, 198–212. doi: 10.1093/biosci/biw002
Meerow, S., Natarajan, M., and Krantz, D. (2021). Green infrastructure performance in arid and semi-arid urban environments. Urban Water J. 18, 275–285. doi: 10.1080/1573062X.2021.1877741
Min, Y. H., Byun, G., and Ha, M. (2022). Young women’s site-specific fear of crime within urban public spaces in Seoul. J. Asian Archit. Build. Eng. 21, 1149–1160. doi: 10.1080/13467581.2021.1941993
Morar, T., Radoslav, R., Spiridon, L. C., and Păcurar, L. (2014). Assessing pedestrian accessibility to green space using Gis. Transyl. Rev. Admin. Sci. 10, 116–139.
Nor, A. N. M., Corstanje, R., Harris, J. A., Grafius, D. R., and Siriwardena, G. M. (2017). Ecological connectivity networks in rapidly expanding cities. Heliyon 3:e00325. doi: 10.1016/j.heliyon.2017.e00325
Norton, B. A., Coutts, A. M., Livesley, S. J., Harris, R. J., Hunter, A. M., and Williams, N. S. G. (2015). Planning for cooler cities: a framework to prioritise green infrastructure to mitigate high temperatures in urban landscapes. Landsc. Urban Plan. 134, 127–138. doi: 10.1016/j.landurbplan.2014.10.018
Noyola-Medrano, M. C., Ramos-Leal, J. A., Domínguez-Mariani, E., Pineda-Martínez, L. F., López-Loera, H., and Carbajal, N. (2009). Factores que dan origen al minado de acuíferos en ambientes áridos: caso Valle de San Luis Potosí. Rev. Mex. Cienc. Geol. 26, 395–410.
Nur Hidayati, I., Suharyadi, R., and Danoedoro, P. (2019). Environmental quality assessment of urban ecology based on spatial heterogeneity and remote sensing imagery. KnE Soc. Sci. doi: 10.18502/kss.v3i21.4981
Okour, Y., and Shaweesh, H. (2024). Identifying the barriers to green infrastructure implementation in semi-arid urban areas using the DPSIR framework: a case study of Amman, Jordan. City Environ. Interact. 24:100165. doi: 10.1016/j.cacint.2024.100165
Ovalle, A. G. C., Tristán, A. C., Amador-Nieto, J. A., Putri, R. F., and Zahra, R. A. (2021). Analysing the land use/land cover influence on land surface temperature in San Luis Potosí Basin, México using remote sensing techniques. IOP Conf. Ser. 686:012029. doi: 10.1088/1755-1315/686/1/012029
Pang, B., Zhao, J., Zhang, J., and Yang, L. (2023). ‘Calculating optimal scale of urban green space in Xi’an, China. Ecol. Indic. 147:110003. doi: 10.1016/j.ecolind.2023.110003
PAOT (2010). Presente y futuro de las áreas verde y del arbolado de la Ciudad de México. D.F. México: Gobierno del Distrito Federal-Procuraduría Ambiental y del Ordenamiento Territorial del Distrito Federal.
Parivar, P., Quanrud, D., Sotoudeh, A., and Abolhasani, M. (2021). Evaluation of urban ecological sustainability in arid lands (case study: Yazd-Iran). Environ. Dev. Sustain. 23, 2797–2826. doi: 10.1007/s10668-020-00637-w
Parker, J., and Zingoni de Baro, M. E. (2019). Green infrastructure in the urban environment: a systematic quantitative review. Sustain. For. 11:3182. doi: 10.3390/su11113182
Paul, S., and Nagendra, H. (2017). Factors influencing perceptions and use of urban nature: surveys of park visitors in Delhi. Land 6:27. doi: 10.3390/land6020027
Pickett, S. T. A., and Cadenasso, M. L. (2008). Linking ecological and built components of urban mosaics: an open cycle of ecological design. J. Ecol. 96, 8–12. doi: 10.1111/j.1365-2745.2007.01310.x
Popa, A. M., Onose, D. A., Sandric, I. C., Dosiadis, E. A., Petropoulos, G. P., Gavrilidis, A. A., et al. (2022). Using GEOBIA and vegetation indices to assess small urban green areas in two climatic regions. Remote Sens. 14:4888. doi: 10.3390/rs14194888
Puliafito, S. E., Bochaca, F. R., Allende, D. G., and Fernandez, R. (2013). Green areas and microscale thermal comfort in arid environments: a case study in Mendoza, Argentina. Atmos. Clim. Sci. 3, 372–384. doi: 10.4236/acs.2013.33039
Quintero, J. D. (2012) Principles, practices, and challenges for green infrastructure projects in Latin America. Inter-American Development Bank.
Reyes-Päcke, S., and Figueroa-Aldunce, I. M. (2010). Distribución, superficie y accesibilidad de las áreas verdes en Santiago de Chile. EURE 36, 89–110.
Russo, A., and Cirella, G. (2018). Modern compact cities: how much greenery do we need? Int. J. Environ. Res. Public Health 15:2180. doi: 10.3390/ijerph15102180
Schütz, C., Reckendorfer, W., and Schulze, C. H. (2017). Local quality versus regional connectivity-habitat requirements of wintering woodpeckers in urban green spaces. J Urban Ecol. 3, 1–11. doi: 10.1093/jue/jux019
SEDATU, CONAPO and INEGI (2018). Delimitación de las zonas metropolitanas de México 2015. 1st Edn. Mexico City: Secretaría de Desarrollo Agrario, Territorial y Urbano (SEDATU), Consejo Nacional de Población (CONAPO), and Instituto Nacional de Estadística y Geografía (INEGI).
SEDEMA (2017) Inventario de áreas verdes urbanas, Secretaria del Medio Ambiente (SEDEMA). Available at: https://www.sedema.cdmx.gob.mx/programas/programa/inventario (Accessed March 28, 2021).
Shackleton, C. M., Blair, A., de Lacy, P., Kaoma, H., Mugwagwa, N., Dalu, M. T., et al. (2018). How important is green infrastructure in small and medium-sized towns? Lessons from South Africa. Landsc. Urban Plan. 180, 273–281. doi: 10.1016/j.landurbplan.2016.12.007
Sheik Mujabar, P. (2019). Spatial-temporal variation of land surface temperature of Jubail Industrial City, Saudi Arabia due to seasonal effect by using thermal infrared remote sensor (TIRS) satellite data. J. Afr. Earth Sci. 155, 54–63. doi: 10.1016/j.jafrearsci.2019.03.008
Sobrino, J. A., Jimenez-Munoz, J. C., Soria, G., Romaguera, M., Guanter, L., Moreno, J., et al. (2008). Land surface emissivity retrieval from different VNIR and TIR sensors. IEEE Trans. Geosci. Remote Sens. 46, 316–327. doi: 10.1109/TGRS.2007.904834
Sorensen, M., et al. (1998). Manejo de las areas verdes urbanas. Washington, DC: División de Medio Ambiente del Departamento de Desarrollo Sostenible del Banco Interamericano de Desarrollo.
Stewart, O. T., Moudon, A. V., Littman, A., Seto, E., and Saelens, B. E. (2018). The association between park facilities and the occurrence of physical activity during park visits. J. Leis. Res. 49, 217–235. doi: 10.1080/00222216.2018.1534073
Stewart, I. D., and Oke, T. R. (2012). Local climate zones for urban temperature studies. Bull. Am. Meteorol. Soc. 93, 1879–1900. doi: 10.1175/BAMS-D-11-00019.1
Szulczewska, B., Giedych, R., Borowski, J., Kuchcik, M., Sikorski, P., Mazurkiewicz, A., et al. (2014). How much green is needed for a vital neighbourhood? In search for empirical evidence. Land Use Policy 38, 330–345. doi: 10.1016/j.landusepol.2013.11.006
Tan, P. Y., Wong, N. H., Tan, C. L., Jusuf, S. K., Chang, M. F., and Chiam, Z. Q. (2018). A method to partition the relative effects of evaporative cooling and shading on air temperature within vegetation canopy. J. Urban Ecol. 4:juy012. doi: 10.1093/jue/juy012
Taylor, L., and Hochuli, D. F. (2017). Defining greenspace: multiple uses across multiple disciplines. Landsc. Urban Plan. 158, 25–38. doi: 10.1016/j.landurbplan.2016.09.024
Taylor, L., Leckey, E. H., Lead, P. J., and Hochuli, D. F. (2020). What visitors want from urban parks: diversity, utility, serendipity. Front. Environ. Sci. 8:595620. doi: 10.3389/fenvs.2020.595620
Tejeda-Martínez, A., and Gómez-Azpeitia, G. (2015). Prontuario solar de México. Mexico City: Universidad de Colima-Universidad Veracruzana.
Thamrin, D., Mulyono, G., and Antoni, (2018). Usability evaluation of adaptable Urban Park furniture product with cellular light-weight concrete as material. IOP Conf. Ser. 408:012033. doi: 10.1088/1757-899X/408/1/012033
Tratalos, J., Fuller, R. A., Warren, P. H., Davies, R. G., and Gaston, K. J. (2007). Urban form, biodiversity potential and ecosystem services. Landsc. Urban Plan. 83, 308–317. doi: 10.1016/j.landurbplan.2007.05.003
U.S. Geological Survey (2020) EarthExplorer. Available at: https://earthexplorer.usgs.gov/ (Accessed June 16, 2023).
UN (2018). La agenda 2030 y los objetivos de desarrollo sostenible: una oportunidad para América Latina y el Caribe. (LC/G.2681-P/Rev.3). United Nation: Santiago.
UN-Habitat (2022). World cities report 2022. Nairobi, Kenya: Envisaging the future of cities. Programa de las Naciones Unidas para los Asentamientos Humanos, ONU-Habitat.
Van Herzele, A., and Wiedemann, T. (2003). A monitoring tool for the provision of accessible and attractive urban green spaces. Landsc. Urban Plan. 63, 109–126. doi: 10.1016/S0169-2046(02)00192-5
van Leeuwen, E., Nijkamp, P., and de Noronha Vaz, T. (2010). The multifunctional use of urban greenspace. Int. J. Agric. Sustain. 8, 20–25. doi: 10.3763/ijas.2009.0466
Vásquez, A., Devoto, C., Giannotti, E., and Velásquez, P. (2016). Green infrastructure systems facing fragmented cities in Latin America - case of Santiago, Chile. Proc. Eng. 161, 1410–1416. doi: 10.1016/j.proeng.2016.08.602
WHO (2016). Urban green spaces and health a review of evidence. Copenhagen: WHO Regional Office for Europe. Technical report.
Xiao, Y., Dai, S., and Zhao, B. (2020). Mitigation of urban Heat Island effect with small-scale parks —An empirical study on community parks in Nanjing, Jiangsu Province. Landsc. Archit. Front. 8:26. doi: 10.15302/J-LAF-1-020028
Yu, Z., Guo, X., Jørgensen, G., and Vejre, H. (2017). How can urban green spaces be planned for climate adaptation in subtropical cities? Ecol. Indic. 82, 152–162. doi: 10.1016/j.ecolind.2017.07.002
Yu, X., Guo, X., and Wu, Z. (2014). Land surface temperature retrieval from Landsat 8 TIRS—comparison between radiative transfer equation-based method, Split window algorithm and Single Channel method. Remote Sens. 6, 9829–9852. doi: 10.3390/rs6109829
Zhang, L., Tan, P. Y., and Diehl, J. A. (2017). A conceptual framework for studying urban green spaces effects on health. J. Urban Ecol. 3, 1–13. doi: 10.1093/jue/jux015
Zhang, L., Wang, Z., and Da, L. (2019). Spatial characteristics of urban green space: a case study of Shanghai, China. Appl. Ecol. Environ. Res. 17, 1799–1815. doi: 10.15666/aeer/1702_17991815
Zhang, Y., Wang, Y., and Ding, N. (2022). Spatial effects of landscape patterns of urban patches with different vegetation fractions on urban thermal environment. Remote Sens. 14:5684. doi: 10.3390/rs14225684
Zheng, Y., Wang, S., Zhu, J., Huang, S., Cheng, L., Dong, J., et al. (2023). A comprehensive evaluation of supply and demand in urban parks along “luck greenway” in Fuzhou. Sustain. For. 15:2250. doi: 10.3390/su15032250
Zhu, W., Sun, J., Yang, C., Liu, M., Xu, X., and Ji, C. (2021). How to measure the Urban Park Cooling Island? A perspective of absolute and relative indicators using remote sensing and buffer analysis. Remote Sens. 13:3154. doi: 10.3390/rs13163154
Keywords: effective green cover (EGC), public green spaces (PGS), normalized difference vegetation index (NDVI), urban park, equipment–infrastructure attributes, land surface temperature (LST)
Citation: Ramos-Palacios CR, Banda-Escalante ME, Barba-Romo CF, Cisneros-Vidales AA and Rodríguez-Herrera JG (2024) Effective green cover and equipment–infrastructure attributes of public green spaces in a Mexican metropolitan area. Front. Sustain. Cities. 6:1470693. doi: 10.3389/frsc.2024.1470693
Edited by:
Wenjie Wang, Zhejiang Agriculture and Forestry University, ChinaReviewed by:
James Kevin Summers, Center for Environmental Measurement & Modeling, Office of Research and Development, United StatesLv Hailiang, Heilongjiang Bayi Agricultural University, China
Shi Xue, University of Illinois at Urbana-Champaign, United States
Copyright © 2024 Ramos-Palacios, Banda-Escalante, Barba-Romo, Cisneros-Vidales and Rodríguez-Herrera. This is an open-access article distributed under the terms of the Creative Commons Attribution License (CC BY). The use, distribution or reproduction in other forums is permitted, provided the original author(s) and the copyright owner(s) are credited and that the original publication in this journal is cited, in accordance with accepted academic practice. No use, distribution or reproduction is permitted which does not comply with these terms.
*Correspondence: Alicia Anahí Cisneros-Vidales, YWxpY2lhLmNpc25lcm9zQHVhc2xwLm14