- School of Engineering and Innovation, Faculty of Science, Technology, Engineering and Mathematics, The Open University, Milton Keynes, United Kingdom
Autonomous urban robots were introduced in Milton Keynes (MK), UK, in 2018 to automate on-demand grocery delivery. Two years later the COVID-19 pandemic rendered routine activities such as delivering groceries or visiting the supermarket unexpectedly unsafe for humans. The ensuing disruption provided opportunities to investigate the potentialities of robotic and autonomous systems to provide cities with resources for coping with unexpected situations such as pandemics, heatwaves and blizzards and ultimately to transform and reinforce urban flows, leading to new ways of living in the city that arise as a result of emerging human-robot constellations. The crisis accelerated the ongoing transformation in human-robot relationships and made its tensions and potentials visible. The case of MK suggests that the cognitive capabilities of urban AIs are not to be found exclusively in computer bits and human neurons but arise from encounters and contexts, with institutions, policies, practices and even the materiality of the city itself being crucial to the emergence of urban AI.
1. Introduction
In 2018 artificial intelligence (AI) enabled robots were introduced in Milton Keynes (MK), UK, to automate on-demand grocery delivery. The demonstrator was the first of its kind in the UK, introducing unsupervised robots in public spaces and complex urban environments. Starship Technologies, the company that designed and operated the robots, was one of several commercial organizations investigating the technological, legal and logistic challenges of last-mile delivery robots, with similar technologies being developed and tested inter alia by Nuro and Kiwibot in the US and White Rhino in China (Hoffmann and Prause, 2018; Figliozzi and Jennings, 2020; Zhao et al., 2022). By using AI to perform a task that would otherwise be performed by humans, robots were expected to reduce carbon emissions associated with urban logistics while also changing the cost structure of on-demand deliveries by substituting labor costs with a one-off capital expense (Ackerman, 2015; London Assembly, 2017).
In 2020, 2 years after the introduction of urban robots in MK, the COVID-19 pandemic rendered routine activities such as delivering groceries or visiting the supermarket unexpectedly unsafe. Delivery robots were reframed as a safer contact-free alternative to in-person deliveries and the autonomous fleet in MK was rapidly expanded. Robots, by virtue of not being susceptible to viral infection, were able to undertake a task potentially too dangerous for humans to perform. As demand for contactless deliveries arose and the use of robots in MK intensified, their role went beyond that of convenience or novelty (Hern, 2020). Robots became part of a multi-layered response to a crisis and after the pandemic they remained as a widespread and even mundane infrastructure (Dempsey, 2022).
The case of MK is emblematic of the ongoing introduction of AIs in urban settings, which remains largely experimental even as such intelligences are applied to automate and optimize critical urban functions such as transport, policing and security, the delivery of goods and food, healthcare, maintenance and repair (While et al., 2021). The introduction of robots in MK was explicitly intended to facilitate learning in advance of deployment in other locations (Pinsent Masons, 2021), with the pandemic subsequently accelerating such processes of learning and diffusion (Starship, 2022). The robotic presence in MK may thus be seen as a prefiguration of robotically augmented futures elsewhere. A quote famously attributed to science fiction author William Gibson states that “the future is already here- it is just not evenly distributed”. The quote alludes to the fact that technologies that will be mundane to those living in the future already exist for some today (Chatterton and Newmarch, 2017). The quote also draws attention to inequality—specifically to the risk that the future will be defined by inequalities deliberately or inadvertently reproducing those of the present (Chatterton and Newmarch, 2017). Thus, critical attention to the tensions and potentials prefigured by such early trials of urban robots is required as they reconfigure urban flows, reshape the city and are reshaped by them and potentially introduce new forms of non-human intelligences and agencies into heterogeneous urban constellations.
This article presents a case study tracing the introduction of artificially intelligent delivery robots in an urban context, their role in response to a crisis and the subsequent stabilization and diffusion of the robotic delivery service here conceptualized as a Robotic and Automated System (RAS). The research presented in this article, focusing on the tensions, potentialities and new ways of living in the city that arise as humans and artificially intelligent robots encounter each other and jointly respond to shared contexts and contingencies, is thus driven by three questions to support a broad, open-ended exploratory enquiry:
1) What new forms of intelligence, if any, emerged in the city following the introduction of the RAS?
2) How did the RAS interact with the urban constellation?
3) What difference did the RAS make to humans in the city (during the crisis and beyond it) and how were the benefits and externalities of the RAS (if any) distributed?
2. Analytical framework and method
Robots of various forms (e.g., delivery robots, drones, driverless cars) make it possible for artificial intelligences (AIs) to be physically present in the city and interact with it. The tensions, potentials and implications of the introduction of artificially intelligent robots in urban settings is a matter of concern and subject of ongoing debates in urban studies (e.g., Cugurullo, 2019; Macrorie et al., 2020; Sumartojo et al., 2021; While et al., 2021; Del Casino Jr et al., 2022). Robots have the potential to augment human capabilities and to automate jobs that humans find repetitive, tedious or even dangerous but there is also a risk that they will reinforce or automate inequalities (for example, due to unequal access to robotic services, or due to displacement of labor). Robots in cities can provide measures to address pressing global risks (for example, as discussed in the case study, by supporting the response to a pandemic) but they can also give rise to new risks as cities becomes subject to code errors or cyber-security attacks by malicious actors (Kitchin and Dodge, 2019; Guenat et al., 2022).
The presence of robots in cities is a recent phenomenon. Robots have been predominantly deployed in controlled or semi-controlled environments with controls to protect human safety and limited human interaction (While et al., 2021). Consequently, research about urban robotics has often relied on proxy approaches including modeling (Figliozzi and Jennings, 2020), scenarios (Pani et al., 2020), and deployments in semi-controlled setting such as hospitals, airports and schools (Sumartojo et al., 2022). Recent advances in AI make it feasible to deploy autonomous robots in complex and unpredictable contexts including urban environments, with this article contributing to a small but expanding body of case studies grounded on such deployments (e.g., Chen et al., 2020; While et al., 2021).
In this article, a case study is used to trace and narrate the processes through which humans and robots learned to live with each other in a complex urban context subject to change and contingency. The COVID-19 pandemic was one of such contingencies encountered during research. The disruption caused by the pandemic inspired a twofold exploration. One strand of research acknowledges the pandemic as an unprecedented crisis and an opportunity to investigate how robots may make cities better able to cope with disasters. A parallel strand frames the pandemic as a revelatory but by no means unique example of how human and artificial intelligences coexist and adapt to each other in cities that are inherently complex and unpredictable. Analysis therefore draws on two somewhat overlapping lenses.
First, we apply a lens informed by literature on urban resilience and the governance of crisis. Although this article does not aspire to make a contribution to the field, the use of such a lens in needed because the role of robots in response to disaster has become a matter of concern as a result of the global COVID-19 pandemic (Chen et al., 2020; Wang et al., 2021; Lin, 2022). Other urban crises may be expected and robots can be expected to play ever-increasing roles in crisis responses (Yigitcanlar et al., 2021). For instance, global climate change is causing increasingly unpredictable extreme weather events including for example floods, blizzards, storms and heat waves. In consequence, cities need a large array of options for coping with the unexpected (Roe, 2020).
The case of MK contributes to a growing body of evidence on how artificial intelligences may have a role to play in the governance of urban crisis, here studied in terms of formal and informal institutions undertaking coordinated action when unknown and unknowable shocks push the operations of urban systems into unstudied conditions. Contemporary governance arrangements increasingly shift away from traditional state-centric approaches to multi-level governance systems and multi-actor alliances (Renn et al., 2011; McGuirk et al., 2021). We investigate how the addition of artificial intelligences to urban constellations in MK might have inflected such multi-institutional and experimental arrangements.
Following MacKinnon and Derickson (2013) and Roe (2020) we adopt a language of coping rather than one of resilience to analyse the role of robots in times of crisis. Resilience, referring to the stability of a system against interference, is a notion derived from ecology and systems theory but conservative when applied to the social sphere (MacKinnon and Derickson, 2013). A conservative rather than transformative response to a shock brings with it a return to the same position of risk that made the system vulnerable in the first place (Roe, 2020). With the reservations above, we borrow a conceptual toolkit associated with resilience for analytical purposes and thus study how AI may have improved urban ability to cope in terms of the “4 Rs of resilience”: redundancy, robustness, rapidity and resourcefulness (Bruneau et al., 2003; Zona et al., 2020). Here redundancy refers to the ability of a system to provide alternative options for effective and efficient management; robustness, to the ability to withstand a certain level of stress while preserving functionality; rapidity, to the rate at which pre-event functionality can be attained and resourcefulness to the community's capacity to identify problems, establish priorities and mobilize resources.
A second analytical lens informing this research draws on recent debates in the field of urban studies to investigate the tensions and potentials that arise when robotic and automated systems (RAS) are deployed in cities to automate, manage, sustain and augment urban functions ranging from surveillance to healthcare to logistics and transport (Cugurullo, 2019; Macrorie et al., 2020; While et al., 2021) and is also inflected by research on cyborg urbanisms (Gandy, 2005; Swyngedouw, 2006) which foresaw the need for a framework for studying the interaction of human and non-human intelligences in cities long before technology developments made empirical study of the issue possible. We therefore investigate the robots in their context- first, robots are studied in the context of a robotic and automated system, a distributed configuration of sensors, processors and actuators spread across a physical environment and possessing the ability to purposefully and autonomously sense it and manipulate it (Marvin et al., 2018). Robots are thus seen as mechatronic actuators of non-human intelligences that may be embedded in the robot, remotely present in a control center or distributed across the system. The RAS, in turn, is investigated in an urban context where human and non-human intelligences participate in contingent unscripted encounters, interacting with and learning from each other.
As AI and robots are introduced to cities there is growing interest in investigating the extent to which RAS might generate new and unanticipated forms of urban life and social organizations, thus (re)configuring the contextual, contingent constellations of places, institutions, infrastructures and the myriad of entities and encounters that constitute the urban (Macrorie et al., 2020; While et al., 2021; Yigitcanlar et al., 2021; Mintrom et al., 2022). We therefore investigate the tensions and potentialities of the RAS and discuss how its deployment in MK may prefigure similar deployments elsewhere. Here, disruption reconfigures the city (McGuirk et al., 2021) and reveals aspects of urban life that would otherwise remain invisible, with breakdowns bringing fleeting visibility to the complex practices and technologies that continuously bring urban life into being (Graham, 2010). Following the geographical sensitivity of this body of research, the following themes directed our analysis: Encounters and interfaces, distributed learning and cognition, (re)configuration, and splintering.
Initial data collection focused on six expert interviews of volunteers and representatives of community organizations supporting vulnerable populations during the crisis, as well as representatives from vulnerable and at-risk groups. This selection of interviewees responded to the original scope of the research, which sought to investigate the role of robots in supporting vulnerable populations (Valdez et al., 2021). As the scope expanded to include broader tensions and potentials of urban AI, data collection and analysis moved predominantly toward non-intrusive observational and documentary approaches. Owing to COVID-19 regulations on research by the Open University, researchers engaged in socially distanced observation (~30 h) through various stages of the pandemic, attending to the interactions of robots, pedestrians, vehicles, pets and other heterogeneous elements that constitute the urban. Desk research included news items, with 50 articles selected for analysis drawing from national publications (The Guardian, BBC News) as well as local MK publications (MK Citizen, MKFM 106.3). Ten technical briefs and press releases published by Starship Technologies were also selected for analysis as well as 20 policy documents including minutes and agendas published by the UK Parliament, the London Assembly and Milton Keynes Council.
When relevant, documents identified through desk research were incorporated into the corpus for thematic analysis, with the critical skepticism required for study of what are often promotional materials. Documents also provided quantitative data used through the case study—e.g., regarding the number of robots in the fleet and deliveries completed at various points in time. Representatives of Starship Technologies were contacted by the authors but interviews could not be secured. Such difficulties in gaining access to data are often associated to research of the urban-digital interface on account of the proprietary nature of algorithms, the value of secrecy and commercial sensitivity in highly competitive fields, and the emphasis on confidentiality and privacy in the venture capital industry (Fields et al., 2020). The study of AIs introduces an additional layer of black-boxing or opacity as machine learning processes do not naturally accord with human logics or explanations, so that machines make meaningful decisions that cannot be fully assessed or explained (Burrell, 2016). Fields et al. (2020) suggest that, rather than “lifting the black box” researchers can usefully look for the meaning achieved through the relations of networked humans and non-humans. Tracing and narratives approaches providing a sense of the sites of transformation, for instance, can be usefully applied to attend to the messiness that the notion of the black box helps to hide (Fields et al., 2020).
The case study was produced to narrate results of a thematic analysis (Braun and Clarke, 2012) pursued through an iterative engagement between data and matters of concern identified in the developing agenda for research on urban AI and robotics (Macrorie et al., 2020; Yigitcanlar and Cugurullo, 2020; While et al., 2021), with a focus on themes across two strands as discussed above—the RAS contribution to the city's ability to cope was studied in terms of Redundancy, Robustness, Rapidity and Resourcefulness, while the urban geography of the RAS was studied in terms of encounters and interfaces, distributed learning and cognition, (re)configuration, and splintering.
3. Case study
The case study will be organized in four sections corresponding to distinct phases of the deployment in MK. First, we will discuss the background to demonstrator including the characteristics of the robots, the AI driving them and the RAS in which they were deployed. Secondly, we will discuss the introduction of robots on a commercial basis to MK, covering the period between the launch of the autonomous delivery service in 2018 and an announcement of how the 30-robot fleet completed its 100,000th delivery in MK, issued in March 2020. The third section of the case study begins with the announcement of the first COVID-19 lockdown in the UK, which coincidentally took place days after the RAS in MK met the 100,000-delivery milestone. This period was characterized by a rapid growth in demand for contactless deliveries, matched by a corresponding increase in the size of the robotic fleet. The size of the fleet in MK went from 30 to ~120 robots during the period covered in this phase, which concludes in August 2020, when most restrictions arising from the first lock-down came to an end. The fourth section of the case study will cover the period of stabilization that followed, when the demand for robotic deliveries and the size of the fleet in MK remained stable despite the end of the crisis, as well as the dissemination that followed when the RAS was introduced to five new urban locations with 500 robots operating across the UK.
3.1. Background—The robots and their RAS
Starship Technologies was founded in 2014 and produced its first prototype robot the same year. The current iteration of the 6-wheeled robot (Figure 1) measures ~56 × 68 × 56 cm (or 125 cm on account of the bright antenna added for visibility), weights ~27 kg and can transport up to 10 kg in its lockable cargo compartment. With its speed of 6 km/h it is suitable for on-demand last-mile deliveries.
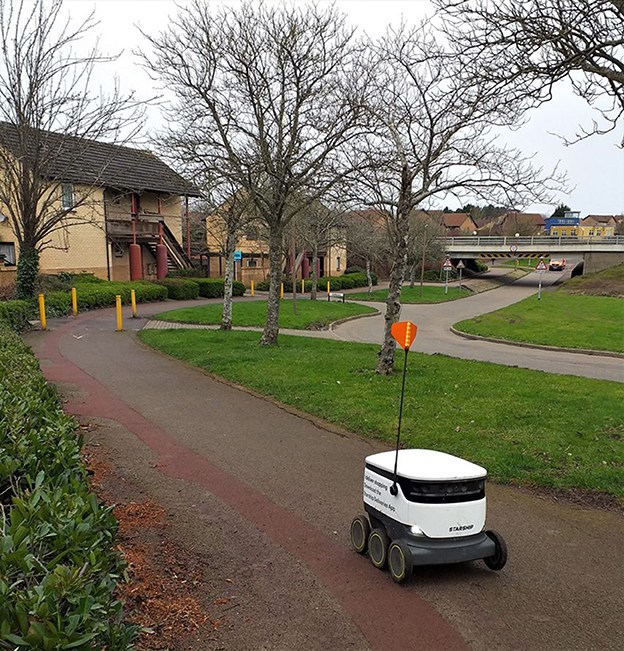
Figure 1. Starship robot in MK, traveling along a segregated path for pedestrians and cyclists colloquially known as a “Redway”.
Autonomous operation of the robots is made possible by machine learning through neural networks (Pärnamaa, 2018) which allow them to navigate their surroundings, detect real-time changes (including the change of traffic lights as well as the movement of pedestrians and cars) and adapt to major changes in their environment (for example, road closures or new constructions). Ten cameras and radars provide inputs to the trainable neural network, which over time becomes better able to create an internal representation of the locations in space of entities such as pedestrians, cars, cyclists, dogs on leashes and navigational landmarks. Individual robots thus sense their environment and then use neural networks to structure low level data into high-level information (Pärnamaa, 2018). Urgent information (e.g., about cars and pedestrians) is processed by the robot while less time-sensitive information related to navigation and operations is transferred to remote servers and aggregated, with robots collectively creating a picture of the city and its flows (Kosonen, 2020; Lääne, 2020). Robots therefore learn collectively and pool their collective knowledge to create a unified three-dimensional map of a given area, which they use to identify and navigate the shortest and the safest path between their hub and their destinations. Collective artificial intelligence is also used to orchestrate fleet operations—deciding which robot should do which delivery based on predictions about the expected demand, the availability of robots and the expected battery state after each journey (Kosonen, 2020).
Customers order groceries through a mobile phone application. The robots are loaded at the local hub and then navigate autonomously to the destination indicated by the user. Once the robot arrives, users receive an alert through the mobile application so they can meet the robot and unlock its cargo compartment. Delivery robots therefore perform a similar function to that of a bicycle courier but they are expected to operate at a fraction of the cost of other last-mile delivery alternatives when deployed at scale (Ackerman, 2015; London Assembly, 2017). Consequently, there is a risk that robots will displace human workers- with some predictions warning that ultimately 50% of the jobs that currently exist may be replaced by robots AIs (Bissell and Del Casino, 2017). Such automated labor futures are full of complexities and ambivalences as artificial intelligences still requires the (frequently backgrounded) support of human intelligence and labor (Sadowski, 2022). Initially, human support for the RAS was intensive as well as overt—two or even three people were needed to remotely monitor every single robot, including a human minder walking with it. As time progressed human support was backgrounded and the RAS moved asymptotically toward full autonomy- according to the company, robots can operate autonomously 99% of the time and one human can now oversee the operations of ten robots remotely, confirming the decision of the AI or taking control if needed. Robots can send a request for human control if their path is blocked, if a wheelchair is identified or if the robot is unable to move. In some cases, if the operator cannot solve the problem remotely, a field assistant is dispatched from an operation center in the area. On occasion, robots also receive unscripted assistance—e.g., when passers-by encounter a robot that has fallen off the pavement, is stuck in a tight space or is struggling to climb an icy incline (Dempsey, 2022; Dobrosovestnova et al., 2022).
3.2. MK—Early demonstrator
When a RAS for grocery deliveries was first envisioned, the legal status of unaccompanied autonomous robots in urban environments was unclear owing to a lack of regulations and legal precedent (Ackerman, 2015). Early demonstrators preceding that of MK took place in corporate and academic campuses in the United States (Jennings and Figliozzi, 2019). Benefits of campus deployments included the ease of navigating orderly, pedestrianized spaces and a high concentration of generally affluent and time-poor consumers. Importantly, campuses are not subject to regulations (or lack thereof) regarding the use of autonomous robots in public space. In order to set precedent and capture knowledge regarding the operation of robots in public spaces, Starship representatives cultivated connections with industry bodies, becoming embedded within the autonomous and connected vehicle community in the UK, as well as with innovation-friendly local authorities (Pinsent Masons, 2021). Milton Keynes, a city in the southeast of England with a population of ~250,000 residents and located some 100 km North of London was selected for the first commercial deployment of delivery robots operating autonomously in an urban setting. Operations started in April 2018.
MK council was willing to work with a technology which they acknowledged was not yet fully proven because of their potential to support their agenda for the economy (by stimulating innovation and job creation), environment (by replacing car trips or van deliveries) and inclusion, as they anticipated that the robots would be useful for providing deliveries to households that suffered from reduced personal mobility (Milton Keynes Council, 2018). MK was also a suitable location for the pioneering deployment of autonomous robots in an urban environment on account of its low density and its extensive network of segregated roads for pedestrians and cyclists (see Figure 1) which were relatively safe and easy for AIs to navigate (CMK Town Council, 2018).
MK was founded in 1967 as part of the “new towns” movement that followed the second world war. As the intellectual successor of the “garden cities” movement of the late 19th and early 20th century, the new town movement was characterized by a modernist, rationalist approach to planning, and incorporated garden city principles such as enforcing a separation between pedestrians and road traffic (Clapson, 2017). Consequently, the development of MK followed a masterplan that was meant to result in a pedestrian-friendly city and incidentally made streets easier to transverse for robots as well. The masterplan also advised that every house in MK should be within 500 meters of a “local center” where amenities such as shops, pubs, and schools would be concentrated. Such local centers work well with the logistics of short-distance on-demand robotic deliveries, as robots can reach households within walking distance of such local centers in a reasonable time. The autonomous delivery service in MK was thus organized around local hubs where the robots were loaded, recharged, and cleaned between deliveries.
The RAS initially delivered groceries on behalf of partner supermarkets which would not have provided on-demand deliveries otherwise, and consequently there was not an obvious displacement of workers. The partner organizations had human-operated vans suitable for making next-day deliveries of a week's worth of groceries and robots complemented their capabilities by making it possible to arrange, for example, the cost-effective delivery of a pint of milk within 30 min. While one of the supermarkets supported by the RAS also offered 1-h delivery without robots by relying on human motorcyclists, this service was only available in London, where the high density and the affluent customer base made human deliveries feasible. In effect, human deliveries made sense for areas with high density and complexity while robot deliveries thrived in the opposite.
In principle, the use of delivery robots in dense urban centers would also benefit from high customer density, with more potential customers living within the area served by a delivery hub (Figliozzi and Jennings, 2020). However, further developments in AI are needed before the deployment of robots in high-density urban centers can be realistically considered (Loke and Rakotonirainy, 2021; Mavrogiannis et al., 2022). Low-density footpaths such as those available in MK make it possible for people to make their way around robots when they stop, as they often do when faced with an uncertain or unexpected situation. However, if a robot were to stop in a crowded footpath in London or a sidewalk in New York it would cause considerable aggravation and risk for pedestrians (Salvini et al., 2021). Classical mapping and navigation algorithms are considered insufficient for safe operation of robots in high-density urban settings which are likely to require consideration of the sometimes random and sometimes linear flows of pedestrians (Du et al., 2019). Narrow AI driven by neural networks may achieve the sophistication needed to monitor and identify pedestrian trajectories (Nasr Esfahani et al., 2022) so that robots can learn to either “go with the flow” or “get out of the way”. However, the ability to navigate crowded urban settings such as those that might be found in London or New York requires capacities approaching those of general AI, such as the ability to understand the social and psychological constraints on pedestrian behavior and cultural conventions of behavior in public space (Bera et al., 2017; Woo et al., 2020; Gao and Huang, 2021). In contrast, the low density of MK, which is comparable to that of suburban areas of larger cities, made it possible for pedestrians to accept the limited social intelligence of the robots and adapt to their behavior, which is seen as non-threatening, helpful and endearing even as they occasionally struggle to complete their assigned task (Sumartojo et al., 2021). As the wide footpaths of MK make it easy for robots and pedestrians to coexist, 70% of pedestrians do not pay any attention to the robots, with most of the rest of street-goers reacting positively to them (Jennings and Figliozzi, 2019). Consequently, the robots were rapidly accepted by users in MK and they were considered part of its everyday life, as even non-users would encounter them as they made their way along pavements or footpaths and kids would try to pet them or feed them (Hamilton, 2021). This is consistent with recent research emphasizing the power of affect in robot-human interaction, as well as role that anthropomorphism plays in the production of such affective responses (Chuah and Yu, 2021; Spatola and Wudarczyk, 2021; Sumartojo et al., 2021).
Initially, the RAS was only available in a relatively affluent neighborhood of MK. As its operations expanded its geography became more socially inclusive. Particularly, expansion of the RAS to the city center made autonomous deliveries available to several offices including corporate headquarters, but also to some of the most deprived areas of MK. A barrier to access remained in the form of the delivery fee of £1. Although the company acknowledged that some early users were attracted by the novelty of the service, they also identified regular users who appreciated the convenience and were willing to pay a premium for it. By April 2019 50,000 deliveries had been completed and the company was aware of users who had placed more than 100 orders through the year (Starship, 2019). 11 months later, on March 13th, 2020, the company met its next milestone, with a 30-robot fleet having completed 100,000 deliveries across MK, leading to a major expansion in the service area which would include the city center. Three days later the United Kingdom unexpectedly entered its first COVID-19 lockdown, with the UK Health Secretary announcing that all unnecessary social contact should cease. The British population was instructed to stay home, all non-essential shops and services were ordered to close and those at the highest risk of severe complications from COVID-19 were advised to follow special shielding measures. MK, like so many other cities across the world, was faced with a major disruption of key aspects of urban life including transport and food provision (Boons et al., 2021).
3.3. MK crisis—The role of robots during the pandemic
Uncertainty about the duration of the stay-at-home orders and the capacity of supply chains to overcome the disruptions caused by COVID-19 provided a rationale for consumers to build up their stocks of groceries and basic household supplies, engaging in what can be described as hoarding and panic buying (O'Connell et al., 2021). Traditional online grocery delivery services were affected by the disruption. Delivery drivers worked extended ours while supermarkets implemented restricted queueing systems and released additional delivery slots but struggled to cope with the sudden growth in demand (UK Department for Environment, Food and Rural Affairs, 2020; BBC News, 2021). Customers faced waiting times of several weeks for scheduled deliveries (Hobbs, 2020). The disruption to online grocery deliveries was problematic for vulnerable individuals including older adults, people with underlying health conditions or those less able to move (Hobbs, 2020), who often rely on home deliveries to remain independent (Jesus et al., 2021). Despite attempts by supermarkets to allocate additional slots, some vulnerable or at-risk individuals were unable to secure deliveries when services were inundated by requests (Gleason et al., 2020). Consequently, 55% of disabled adults surveyed by the Office for National Statistics reported difficulties accessing groceries, medication and essentials (Office for National Statistics, 2020)—this included those who were not considered vulnerable enough to receive government or community support, as well as people who qualified for assistance but struggled to secure support (Eskyte et al., 2020). Over three million people reported going hungry in the first three weeks of the UK's COVID-19 lockdown (YouGov, 2020) and ~8% of the adults in the UK advised to self-isolate experienced food insecurity because they were unable to go out and did not have any other way to get the food they needed (Loopstra, 2020).
In contrast to conventional online-delivery services, the RAS proved to be sufficiently robust as to meet the sudden spike in demand and continued operations in MK during the pandemic with very little disruption. Company executives state that the number of orders doubled virtually overnight. The fleet of autonomous robots was working non-stop 14 h a day (Shirbon, 2020) but the RAS remained able to support same-day deliveries. Although the delivery service had not been specifically designed for that purpose, robots which replaced humans and thus prevented face-to-face interactions proved to be well suited for delivering groceries to those housebound, shielding or self-isolating on account of the pandemic. Disability advocacy groups included deliveries by Starship robots, where available, in guidance for clinically vulnerable and extremely vulnerable groups (Disability Horizons, 2020). A decision was made to expand the coverage and capacity of the fleet as quickly as possible, so that the number of robots available for deliveries in Milton Keynes doubled from 30 at the beginning of the pandemic in March 2020 to 70 by April and 100 by July of the same year.
Achieving a 300% increase in capacity in a matter of weeks would have been difficult for car-based and human-based services on account of the cost and challenge of acquiring new vehicles in the middle of a pandemic which severely affected supply chains in addition to the difficulties of recruiting and training staff on a short notice. The case of MK suggests that AI-driven robotic systems can be reconfigured and can rapidly adapt and respond to crisis situations. The RAS in MK benefited from a fortuitous contingency—delivery robots in other locations were predominantly deployed in university campuses which were closed due to the pandemic, leaving them available for redeployment to MK. Such a rapid redeployment still required considerable human effort [for example, for the staff loading the robots at the delivery hub (Bird, 2021)] but was facilitated by the AI powering the robots: new additions to the fleet could be readily connected to the servers storing the aggregated navigational knowledge of the collective intelligence and could be readily handled by the AI system orchestrating fleet operations.
Company executives framed contactless delivery as one of the most reliable ways to protect vulnerable populations, preventing face-to-face contact and reducing the risk of contagion (Starship, 2021c). The robots completed over 1.5 million deliveries through the duration of the pandemic (Starship, 2021c). The rapid growth in demand suggests that the robots performed a useful role during the crisis although, like so many other urban technologies, robots also demonstrated potential to reinforce social and spatial inequalities (Macrorie et al., 2020). According to interviews with local community organizations and support groups, such inequalities were present to some degree in MK. Spatial inequalities were present because the robots were only available in selected areas of MK, with a tendency to cover more affluent areas and neglect poorer ones and those that were somewhat marginalized. The ability to cope depended on managing scarce resources and therefore entailed some degree of exclusion. Before the pandemic, the delivery service operated under a flat fee of £0.99. The pricing scheme was temporarily changed at the height of the pandemic to prevent demand from exceeding the capabilities of the robotic fleet, with the service operating under a variable pricing scheme. The company lowered service fees when fewer people requested deliveries and increased it during periods of high demand (Starship, 2021b). The variable pricing scheme, which was by design a barrier to use intended to manage an excess of demand, had the disadvantage of privileging those better able to pay the additional fee while marginalizing the economically disadvantaged. Members of vulnerable or marginalized groups such as those subsisting on a disability allowance would not be able to pay the variable delivery fee on a regular basis. Other vulnerable individuals who would have liked to rely on the robots during the pandemic were unable to use them because they were not able to use the mobile phone application. Vulnerable populations are often less connected to the internet and less able to use online resources, particularly if they are older, have lower incomes, or live alone (Eskyte et al., 2020; Gleason et al., 2020). The robots are also unsuitable for some users with physical and mobility impairments. For instance, wheelchair users may find it difficult to reach and unload the robot while those with visual impairments may be unable to use the mobile phone application.
Nonetheless, even if a RAS was not a suitable replacement for voluntary organizations, community groups and other components of the social safety net, they usefully complemented it—the availability of robots and human volunteers increased the redundancy and resourcefulness of the support network, and therefore made the city better able to cope with an unexpected crisis. Various initiatives and responses by governmental, commercial and community organizations demonstrated distinct capabilities, strengths and weaknesses and served (or failed to serve) distinct groups at various points through the crisis. Although the safety network provided by robots was not equally available to everyone in need of grocery deliveries, it had the distinct advantage of being robust and rapidly able to adapt and respond to an unexpected situation in real time, thus providing a crucial safety net during the early weeks of the pandemic when many vulnerable individuals experienced food insecurity (Loopstra, 2020; YouGov, 2020).
In contrast, national and local authorities were not well suited to take rapid action when faced with an unprecedented situation. Weeks after the beginning of the lockdown, local authorities were still waiting for guidance from national government and did not have access to the full lists of people identified as extremely vulnerable in their area (Local.gov.uk, 2020). Community and volunteer organizations were also largely caught by surprise by the rapidly changing situation, with a study conducted by the Research Institute for Disabled Consumers (2020) finding that 50% of the respondents were unable to receive support previously available to them during the early weeks of the pandemic (Eskyte et al., 2020). Interviews of MK-based volunteers confirms that some organizations were able to respond in a matter of days but for others it was a matter of weeks before they could adapt to the new situation and provide safe and effective support to those who needed it. Volunteer and community organizations benefited from their pre-existing knowledge of vulnerable individuals (Chevée, 2021) and were able to reach and support people that robots could not reach, but they could not easily identify and reach people who had not considered themselves vulnerable before the pandemic. Those same individuals were potentially excluded from government initiatives intended to ensure that vulnerable people could get food (UK Parliament, 2020), which were subject to triage (Department for Environment and Rural Affairs, 2020) and consequently excluded some individuals who considered themselves vulnerable but were not considered a priority. People with manageable health conditions (e.g., immune deficiencies, asthma) who were able to live independently under ordinary circumstances unexpectedly found themselves vulnerable, housebound and unfamiliar with the support networks that could have helped them, but many of them were able to rely on the autonomous robot delivery system. Urban responses to the developing crisis can therefore be conceptualized as a multi-layered safety net, with each layer having different gaps or potential points of failure. For some vulnerable households, access to autonomously delivered groceries provided a first safety net before the other layers could be set in place. In other cases, the robots provided one last extra layer for vulnerable individuals who had slipped through the cracks in all the others, for example because they were not eligible for government support and were not aware of community organizations.
3.4. Stabilization and diffusion
The first lock down was eased in August 2020. The size of the RAS in MK had largely stabilized by then with a fleet of ~120 robots. Public acceptance and demand for autonomous deliveries continued well beyond the end of the lock-down. The robotic delivery service, initially positioned as purely commercial, benefited from its framing as part of a pandemic response. This is reflected in PR materials made available by Starship (2021b), in announcements by adopting local authorities and commercial partners (Milton Keynes Council, 2018; Hutton, 2022), investors (Lienert and Lee, 2020) and commercial partners (Co-op, 2020).
In November 2020, the RAS was introduced in a second location in the UK, with the service becoming available to 5,000 households in a suburban area of Northampton. A second lock-down was announced by UK government shortly afterwards and the Northampton RAS was once again framed as a safer option in a time of crisis (Northamptonshire Council, 2021). No new locations were announced in the UK for the duration of the restrictions (although the robots gradually returned to university campuses in the US). Following the easing of restrictions in the UK in September 2021 Starship announced a plan to bring the RAS to 5 new urban areas across and increase the size of the fleet to 500 robots across the UK (Starship, 2022). By the end of 2022, following the expansion of the RAS and its post-pandemic return to university campuses, 4 million autonomous deliveries had been completed globally.
The urban layout of successive locations, initially similar to MK but gradually departing from its suburban qualities, suggests that the reach of robots was inflected by learning processes pertaining to the navigation spaces of increasing complexity. Deployments in Cambourne (May 2022), Bedford and Northamptonshire (July 2022), Cambridge and Leeds (November 2022) suggest that navigation of university campuses was a steppingstone toward navigation of the segregated pedestrian footpaths of a suburban setting, which in turn lead to crossing urban roads and navigating the pavements of historic market towns.
Despite the diffusion of the robots to other urban settings (or, perhaps, on account of it) the robots have remained closely associated with MK, to the point that one of them was tasked with delivering a bid for MK to be granted formal city status by Queen Elizabeth on occasion of her platinum jubilee. At the same time, familiarity made the robots appear mundane- a resident reported that his younger child, too young to remember life without robots, considered them to be somewhat less interesting than buses.
4. Discussion and conclusions
As stated in the introduction, the case study above supported parallel explorations of a revelatory episode in the introduction of urban robots to MK with potential to prefigure similar deployments elsewhere. We acknowledge the pandemic as an unprecedented crisis and an opportunity to investigate how robots and cities respond together to disruption. At the same time, we consider disruption, contingency and unpredictability as defining characteristics of urban life. The case can thus be considered as a revelatory but by no means unique example of how human and artificial intelligences coexist and adapt to each other in cities. We now return to the three questions driving this open-ended exploratory enquiry of artificial intelligences, of how they may reconfigure urban constellations and of what the impact of such reconfigurations may be.
First, what new forms of intelligence, if any, emerged in the city following the introduction of the RAS? The intelligence demonstrated by individual robots was limited and based on repetition, a consequence of the nature of machine learning (Sumartojo et al., 2022). Repeated journeys along the footpaths and pavements of MK trained them to navigate more efficiently, identifying and avoiding obstacles and choosing more reliable routes. However, such repetitions could not lead to new behaviors by the robots. Consequently, the intelligence demonstrated by them was limited to navigation and to the optimisation of urban flows. Qualitatively, the intelligent behaviors demonstrated by robots were not significantly different before, during or after the pandemic.
Nonetheless, the RAS demonstrated rapid, intelligent adaptation. Where services that predominantly relied on human labor and human intelligence were overwhelmed by a sudden increase in demand, the RAS proved to be robust and adaptable, continuing operations without disruption. In a sense, however, the RAS did not adapt, but was adapted—human intelligence was behind the decision to expand the fleet by 300% and human operators had to institute new practices such as regular disinfection of the robots. However, such an adoption would not have been possible without the AI, as the intelligence of the robots, limited as it may have been, was built on a logic of connection (Sumartojo et al., 2021) and therefore made it possible for 90 new robots to join the fleet and immediately have the knowledge to intelligently navigate the city and maintain its flows.
Through the case study, the effective operation of the robots before, during and after the crisis was revealed as a result of hybrid intelligences—with the narrow intelligence of the robots acting as a multiplier, enhancing and opening new possibilities of action to human intelligences. Thus, the robotically enhanced response to the pandemic could not be attributed decisively to human or non-human intelligences but (following McGuirk et al., 2021) was revealed to be a form of hybrid cognition and governance by experiment collectively produced by actors in the private sector, civic groups, philanthropies, users and even passers-by.
Here, the intelligence of urban AI is seen again as emerging not from the machine alone but from its use in context. Sumartojo and Lugli (2022) see the robots not as purely mechanical but as lively and unfinished—active, but never quite completed as their potentialities exceed those of their design process. The case illustrated how robots, or rather, the RAS in which they are embedded, can be quickly hacked or redesigned in response to unprecedented demands, and such hacks are not necessarily implemented as changes to the hardware or software. Adaptive capacity and intelligence exceeding that actually present in the RAS emerged from reconfigurations of the ongoing robotic relationalities with people, environments and other technologies.
Second, how did the RAS interact with the urban constellation? Cities are heterogeneous, and the urban response to the crisis in the case study was similarly heterogeneous and multi-layered. Robots could be readily incorporated into the shifting and heterogeneous constellations coalescing around the distribution of groceries to households before, during and after the pandemic. Their narrow intelligence and the capabilities of their physical bodies did not exceed those of a human in any way, but nevertheless they opened up new potentialities. The case of MK suggests that the RAS became one more actor readily integrated to already present de-centralized and multi-layered networks for crisis governance and management (Renn et al., 2011). When a critical societal function (e.g., ensuring that groceries can reach households) is structured by a small number of practices, capabilities and technologies (e.g., buyers driving to stores or by couriers from the stores driving to households) there is a risk that when faced with a shock in their environment they will all experience highly correlated and destabilizing failures (Bronk and Jacoby, 2016; Beckert and Bronk, 2018). The case thus suggests that urban robots can make cities better able to cope with the unexpected when they initiate a shift away from technical and institutional monocultures. To achieve that end, it is important that they are not framed as the sole solution to a given urban challenge (potentially becoming a monoculture of their own) but they can usefully become part of a network of complementary commercial, governmental and volunteer-led approaches for supporting key urban functions such as that of getting groceries to households.
The RAS was intended to facilitate urban flows by inexpensively transporting groceries from hubs to households, and the case suggests that it did so effectively. However, the case also suggests that affects and effects were not independent, so that affective factors inflected the (re)configuration of the RAS. In terms of operation and institutional logic, the case of MK would seem to be similar to that of San Francisco (While et al., 2021), comprising a small-scale commercial initiative providing a robotic platform for grocery deliveries within an experimental space made available by a local authority. However, the affective response to robots in MK appears to be entirely different to the one in San Francisco, where opposition resulted in a temporary ban. Potential reasons behind the different affective responses are multiple and attributable, for instance, to culture, to place or to contingency. For an example regarding place, the case suggests that the layout of MK allows humans and robots to share public spaces without getting in each other's way and may even allow room for encounters where humans antropomorphise robots and have generally positive affective responses toward them (Dempsey, 2022). Regarding contingency, the case of MK is consistent with the observation by Yigitcanlar et al. (2020, 2022) suggesting that the use of AI for “greater good” areas such as disaster management is perceived uncritically in comparison to for-profit applications. Although further research would be needed to identify factors inflecting the differences in affective responses in MK and SF, the case strongly suggests that such responses are impactful.
Observation of the interactions between robots and urban constellations also draws attention to their mutually constitutive nature. RAS and related technologies such as driverless cars are expected to become more prevalent in the future, raising the possibility that urban forms may be altered and simplified to make it easier for robots to operate effectively in them (Sumartojo et al., 2022). The case suggests that such alterations to the urban fabric were not needed in MK because the city, designed according to a rational masterplan, was already repetitive, compartimentalised and generally amenable to robotic ways of seeing and acting. Later, as the RAS was deployed in other locations across the UK, the case may appear to suggest that robots, through a process of training and repetition, gradually learned to navigate urban forms that were somewhat different to those of MK and considerably more challenging than those of academic and corporate campuses. Therefore, it may appear that robots changed but cities remained obdurate and static, as no new roads were built for the robots and the operations of the RAS were run from already existing distribution centers. Nevertheless, the case suggests that although robots did not alter urban forms, they altered urban flows in ways that were not entirely determined by the materiality of the city but were rather constrained by the physical and computational possibilities of the RAS. The RAS acted as infrastructure for handling the flow of groceries with such flows determined by the location of distribution hubs and the reach of robots with a speed of 6 km/h. Consequently, although the city appeared to remain the same, some of its flows and institutions were altered to fit robotic logics of repetition and compartmentalization.
Third, what difference did the RAS make for humans in the city (during the crisis and beyond it) and how were the benefits and externalities distributed? Urban robots are expected to have complex beneficial and detrimental impacts on society, with potential to support and augment human capabilities but also to replace them altogether. Likewise, robots have potential to foster innovation, enhance access and provide data for improved decision-making but there is also a risk that they will reproduce and automate inequality or result in more fragmented and opaque urban governance (Guenat et al., 2022). Further research is needed to critically assess the long-term impacts of the RAS in MK. Ultimately, robots have become a mundane infrastructure and a normal part of the streetscape, but the scale of their deployment has not had a transformational impact. Data suggests that there are environmental benefits arising from the substitution of car journeys by electrically powered robot deliveries. A study commissioned by MKC suggests that 70% of the journeys undertaken by robots would otherwise have been undertaken by a car (Starship, 2021a). However, even with a 500-hundred robot fleet the reductions (estimated to be equivalent to 137 tons of CO2 across the UK as of 2021) remain limited, if welcome. Meanwhile, further research is needed to assess the impact and distribution of externalities such as the displacement of human workers. Urban scholars have emphasized the need to shed light on the new socio-spatial relations of production and consumption enabled by AI, and on the emerging human-machine relations that the presence of urban AI is fostering in the city (Bissell and Del Casino, 2017). However, by accident or by design, robots in MK were deployed in niches where they did not overtly displace or compete with human workers. Consequently, the case did not provide conclusive data regarding the impact of robots on employment.
The difference made by the RAS is clearer when focusing on the response to the crisis. The case of MK suggests that autonomous robot systems can effectively provide cities with resources to better cope with future pandemics. The RAS made groceries available to some vulnerable or at-risk persons who would not have been able to get them otherwise and allowed many others to avoid exposure and potential contagion.
However, the case strongly suggests that the RAS would have failed if it had been expected single-handedly support the relevant urban function of delivering groceries in times of crisis, and indeed it was not intended to do so. The impact of robots has often been limited in scope and reliability when they have been pushed to their limits in crisis scenarios (Wang et al., 2021). The case study does not suggest otherwise but reveals that robots provide cities with more options, if deployed as part of a heterogeneous and multi-layered constellation. Robots made the city better able to cope with the unexpected not because they were inherently more effective or less prone to failure than other systems, but because they acted as a redundant system with different points of failure. A city that relied exclusively on robots for all its logistics would not necessarily be more robust nor better able to rapidly adapt than one relying exclusively on humans and automobiles. Urban robots, like other urban systems that rely on networked computation, are subject to malfunction as well as to forms of vandalism, disruption and criminal exploitation that risk making city infrastructures insecure and brittle (Kitchin and Dodge, 2019). However, even if the robots could not single-handedly address the crisis, they usefully complemented MK's response to it as the increased resources and options available increased its ability to cope.
Importantly, the ability of the RAS to rapidly adapt was not planned. The case suggests that it emerged as a result of the open-ended nature of urban RAS as lively technologies (Sumartojo et al., 2021). This matters because the potential of RAS to be hacked or adapted may allow them to support key urban functions when faced with unknown and unknowable crisis scenarios (Figure 2). Global climate change is causing increasingly unpredictable extreme weather events including for example floods, blizzards, storms and heat waves. Hence cities need a large array of options for coping with the unexpected (Roe, 2020) as resourceful communities can better respond to disaster events as they have more options available to come with solutions (MacKinnon and Derickson, 2013; Zona et al., 2020). For instance, the RAS operated without interruption during the major weather disruption caused by Storm Emma in 2018 (Morris et al., 2018; Pärnamaa, 2018). However, it must be noted that the RAS was not specifically designed for adverse conditions- robots are currently unable to transverse flooded areas (MKFM, 2020) and they sometimes required human assistance to complete their task in icy weather (Dempsey, 2022; Dobrosovestnova et al., 2022).
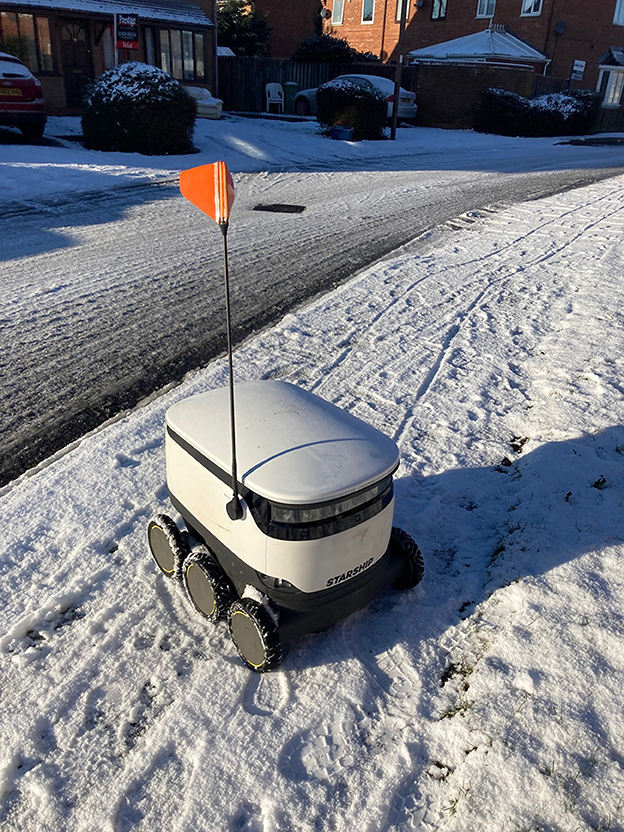
Figure 2. Starship robots have proven able to operate under a variety of conditions including heatwaves, storms and blizzards.
The case of MK also suggests that the RAS was useful to support some vulnerable sectors of the population but not others—in this case, housebound or at risk-individuals without access to a human support network benefited from the robots, but such support depended on users living in areas covered by the RAS, being able to pay the delivery fee, and being physically able to interact with the robots. The case thus confirms that the introduction of robots in urban environments risks reproducing or reinforcing various forms of inequality, fragmentation, splintering and injustice, as has also been the case for digitally augmented smart and cybernetic cities (Martin et al., 2018; Clark, 2020; Macrorie et al., 2020; Odendaal, 2021).
Ultimately, the case study provided an opportunity to critically investigate the role of disaster as a productive catalyst for the accelerated development of urban AI. As Lin (2022) observed, COVID-19 accelerated research into new form of advanced automation with potential to achieve more resilient but also less inclusive urban flows. The resulting urban (re)configuration can be seen as confirmation of a familiar pattern, as the relationship between disruption, shock and innovation is well known in the literature (e.g., Schroeder et al., 2000; Bessant et al., 2015). However, what is unexpected is the speed of the response—Robots adapted (or were adapted) to the crisis in a matter of days or even hours, with the sudden rupture opening up potentials and creating an opening for AI-driven reconfigurations (Lin, 2022). Although robots possessed only a narrow intelligence based on repetition and compartmentalisation, they benefited from their ability to connect data and coordinate action, as well as from a logic based on real-time adaption to changes in urban flows which can be usefully described as smart (Kitchin, 2018) or cybernetic (Gandy, 2005; Swyngedouw, 2006). In the case of MK the outcome of such reconfiguration was simultaneously transformative and reinforcive. It was transformative because it made human-robot interactions appear mundane and because those robotic infrastructures, mundane in MK but largely unseen elsewhere, are now inflecting multiple urban sites (where they may then become mundane). Nevertheless, the reconfiguration was also reinforcive as it provided the city (or rather, commercial organizations in the city) with means to maintain pre-existing urban flows in the face of disruption.
Current debates about the impact of AI and their relationship with humans have generally coalesced around three broad perspectives (Peeters et al., 2021)—a technology-centric perspective claiming that AI will outperform and potentially displace humans, a human-centric perspective claiming that humans will always remain superior to AI and lastly a perspective centered on collective intelligence- ultimately, the case of MK suggests that assemblages of human and non-human intelligences have potential to lead to the emergence of cognitive capabilities greater than the sum of its parts (Ha and Tang, 2022). Crucially, the resulting forms of collective intelligence cannot be reduced to some combination of bits in a computer and synapses in human brains. Instead, urban intelligence is expanded ecology of intelligence that includes bits and brains but also institutions, cultural memories, communities, bits of paperwork and protocol, and even the materialities of the city itself (Mattern, 2020). The resulting constellation unexpectedly and almost incidentally, provided a city with more options to cope with an uncertain, unpredictable future, with robots simultaneously reconfiguring and sustaining urban logistics. A RAS faced with the unexpected was able to support some members of vulnerable populations but also replicated some pre-existing socio-spatial inequalities and exclusions. Ultimately, the case suggests that AI in cities unlocks potentials for more inclusive, socially just and sustainable cities, but such potentials cannot be taken for granted as they depend on a constant and open-ended interplay of human and non-human intelligences.
Data availability statement
The datasets presented in this article are not readily available because they contain information that could compromise the privacy of study participants. Participants of this study did not agree for their data to be accessed publicly. Requests to access the datasets should be directed to bWlndWVsLnZhbGRlekBvcGVuLmFjLnVr.
Ethics statement
The studies involving human participants were reviewed and approved by Human Research Ethics Committee at The Open University—reference number 3756/Valdez. The patients/participants provided their written informed consent to participate in this study.
Author contributions
All authors listed have made a substantial, direct, and intellectual contribution to the work and approved it for publication.
Funding
This paper results from a research project funded by the Open University's Coronavirus rapid response fund.
Acknowledgments
The content of this manuscript has been presented in part at the IEEE International Conference on Systems, Man, and Cybernetics (SMC) (Valdez et al., 2021).
Conflict of interest
The authors declare that the research was conducted in the absence of any commercial or financial relationships that could be construed as a potential conflict of interest.
Publisher's note
All claims expressed in this article are solely those of the authors and do not necessarily represent those of their affiliated organizations, or those of the publisher, the editors and the reviewers. Any product that may be evaluated in this article, or claim that may be made by its manufacturer, is not guaranteed or endorsed by the publisher.
References
Ackerman, E. (2015). Startup Developing Autonomous Delivery Robots That Travel on Sidewalks. Available online at: https://spectrum.ieee.org/automaton/robotics/industrial-robots/starship-technologies-autonomous-ground-delivery-robots (accessed February 21, 2023).
BBC News (2021). Supermarket Websites Struggle Amid New Lockdown. Available online at: https://www.bbc.co.uk/news/business-55540485 (accessed February 21, 2023).
Beckert, J., and Bronk, R. (2018). Uncertain Futures—Imaginaries, Narratives and Calculation in the Economy. Oxford: Oxford University Press. doi: 10.1093/oso/9780198820802.001.0001
Bera, A., Randhavane, T., Prinja, R., and Manocha, D. (2017). “Sociosense: Robot navigation amongst pedestrians with social and psychological constraints,” in 2017 IEEE/RSJ International Conference on Intelligent Robots and Systems (IROS), p. 7018–25. doi: 10.1109/IROS.2017.8206628
Bessant, J., Rush, H., and Trifilova, A. (2015). Crisis-driven innovation: the case of humanitarian innovation. Int. J. Innovat. Manage. 19, 1540014. doi: 10.1142/S1363919615400149
Bird, C. (2021). “It's international youth day,” Co-op Colleague Stories. Available online at: https://colleaguestories.coop.co.uk/2021/08/12/its-international-youth-day/ (accessed February 21, 2023).
Bissell, D., and Del Casino, V. J. (2017). Whither labor geography and the rise of the robots? Social Cultural Geography. 18, 435–442. doi: 10.1080/14649365.2016.1273380
Boons, F., Doherty, B., Köhler, J., Papachristos, G., and Wells, P. (2021). Disrupting transitions: qualitatively modelling the impact of COVID-19 on UK food and mobility provision. Environ Innovat Soc Transit. 40, 1–19. doi: 10.1016/j.eist.2021.04.003
Braun, V., and Clarke, V. (2012). “Thematic analysis,” in Cooper H,. E., Camic, P. M., Long, D. L., Panter, A. T., Rindskopf, D. E., and Sher, K. J. APA handbook of research methods in psychology, Vol. 2. Research designs: Quantitative, qualitative, neuropsychological, and biological (Washington D.C.: American Psychological Association), p. 57–71.
Bronk, R., and Jacoby, W. (2016). Uncertainty and the dangers of monocultures in regulation, analysis and practice—Discussion Paper 16/6. Cologne: Max Planck Institute for the Study of Societies.
Bruneau, M., Chang, S. E., Eguchi, R. T., Lee, G. C., O'Rourke, T. D., Reinhorn, A. M., et al. (2003). A framework to quantitatively assess and enhance the seismic resilience of communities. Earthquake Spectra. 19, 733–752. doi: 10.1193/1.1623497
Burrell, J. (2016). How the machine “thinks”: Understanding opacity in machine learning algorithms. Big Data Soc. 3, 2053951715622512. doi: 10.1177/2053951715622512
Chatterton, T., and Newmarch, G. (2017). The future is already here: it's just not very evenly distributed. Interactions. 24, 42–45. doi: 10.1145/3041215
Chen, B., Marvin, S., and While, A. (2020). Containing COVID-19 in China: AI and the robotic restructuring of future cities. Dialogues Hum Geogr. 10, 238–241. doi: 10.1177/2043820620934267
Chevée, A. (2021). Mutual Aid in north London during the COVID-19 pandemic. Soc. Mov. Stud. 21, 1–7. doi: 10.1080/14742837.2021.1890574
Chuah, S. H., and Yu, J. (2021). The future of service: the power of emotion in human-robot interaction. J. Retail. Consumer Serv. 61, 102551. doi: 10.1016/j.jretconser.2021.102551
Clapson, M. (2017). The English new towns since 1946: what are the lessons of their history for their future?. Histoire Urbaine. 3, 93–111. doi: 10.3917/rhu.050.0093
Clark, J. (2020). Uneven Innovation: The Work of Smart Cities. NY: Columbia University Press. doi: 10.7312/clar18496
CMK Town Council (2018). Have you seen a Starship? Available online at: http://cmktowncouncil.gov.uk/cmk-news/have-you-seen-a-starship/ (accessed February 21, 2023).
Co-op (2020). Rise of the Robots as Co-op and Starship Roll-out Autonomous Delivery Expansion. Available online at: https://www.co-operative.coop/media/news-releases/rise-of-the-robots-as-co-op-and-starship-roll-out-autonomous-delivery (accessed February 21, 2023).
Cugurullo, F. (2019). Urban artificial intelligence: from automation to autonomy in the smart City. Front. Sustain. Cities 2, 38. doi: 10.3389/frsc.2020.00038
Del Casino Jr, V. J., House-Peters, L., Crampton, J. W., and Gerhardt, H. (2022). “The social life of robots: The politics of algorithms, governance, and sovereignty,” in Machine Learning and the City: Applications in Architecture and Urban Design, ed S. Carta. p. 603–613. doi: 10.1002/9781119815075.ch49
Dempsey, M. (2022). Are we falling in love with robots? BBC News. Available online at: https://www.bbc.co.uk/news/business-62007675 (accessed February 21, 2023).
Department for Environment and Rural Affairs (2020). Non Shielded Food Vulnerable Program. Available online at: https://fooddeliveryreferrals.defra.gov.uk/help (accessed 14 May 2021).
Disability Horizons (2020). Coronavirus shopping safely: how to get your food if you're disabled. Available online at: https://disabilityhorizons.com/2020/04/coronavirus-uk-how-to-get-your-food-shopping-if-youre-disabled-people/ (accessed February 21, 2023).
Dobrosovestnova, A., Schwaninger, I., and Weiss, A. (2022). “With a little help of humans. an exploratory study of delivery robots stuck in snow,” In 2022 31st IEEE International Conference on Robot and Human Interactive Communication (RO-MAN) (IEEE), p. 1023–29. doi: 10.1109/RO-MAN53752.2022.9900588
Du, Y., Hetherington, N., Oon, C. L., Chan, W., Quintero, C. P., Croft, E., et al. (2019). “Group surfing: a pedestrian-based approach to sidewalk robot navigation,” in 2019 International Conference on Robotics and Automation (ICRA) (IEEE), p. 6518–24. doi: 10.1109/ICRA.2019.8793608
Eskyte, I., Lawson, A., Orchard, M., and Andrews, E. (2020). Out on the streets–crisis, opportunity and disabled people in the era of COVID-19: reflections from the UK. Alter. 14, 329–336. doi: 10.1016/j.alter.2020.07.004
Fields, D., Bissell, D., and Macrorie, R. (2020). Platform methods: Studying platform urbanism outside the black box. Urban Geograph. 41, 462–468. doi: 10.1080/02723638.2020.1730642
Figliozzi, M., and Jennings, D. (2020). Autonomous delivery robots and their potential impacts on urban freight energy consumption and emissions. Transp. Res. Proc. 46, 21–28. doi: 10.1016/j.trpro.2020.03.159
Gandy, M. (2005). Cyborg urbanization: complexity and monstrosity in the contemporary city. Int. J. Urban Reg. Res. 29, 26–49. doi: 10.1111/j.1468-2427.2005.00568.x
Gao, Y., and Huang, C. (2021). Evaluation of socially-aware robot navigation. Front. Robot. AI. 8, 420. doi: 10.3389/frobt.2021.721317
Gleason, C., Valencia, S., Kirabo, L., Wu, J., Guo, A., Carter, E., et al. (2020). “Disability and the COVID-19 Pandemic: Using Twitter to Understand Accessibility during Rapid Societal Transition,” in The 22nd International ACM SIGACCESS Conference on Computers and Accessibility, p. 1–14. doi: 10.1145/3373625.3417023
Graham, S. (2010). Disrupted cities: When infrastructure fails. NY: Routledge. doi: 10.4324/9780203894484
Guenat, S., Purnell, P., Davies, Z. G., Nawrath, M., Stringer, L. C., Babu, G., et al. (2022). Meeting sustainable development goals via robotics and autonomous systems. Nat. Commun. 13, 3559. doi: 10.1038/s41467-022-31150-5
Ha, D., and Tang, Y. (2022). Collective intelligence for deep learning: a survey of recent developments. Collect. Intell. 1, 26339137221114874. doi: 10.1177/26339137221114874
Hamilton, I. A. (2021). Food delivery company Starship Technologies has enjoyed explosive growth during the pandemic. It doesn't mind that kids are feeding its robots bananas. Available online at: https://www.businessinsider.com/starship-technologies-finds-kids-feeding-its-robots-bananas-2021-1?r=US&IR=T (accessed February 21, 2023).
Hern, A. (2020). Robots deliver food in Milton Keynes under coronavirus lockdown. The Guardia. Available online at: https://www.theguardian.com/uk-news/2020/apr/12/robots-deliver-food-milton-keynes-coronavirus-lockdown-starship-technologies (accessed February 21, 2023).
Hobbs, J. (2020). Food supply chains during the COVID-19 pandemic. J. Agric. Econ. 68, 171–176. doi: 10.1111/cjag.12237
Hoffmann, T., and Prause, G. (2018). On the regulatory framework for last-mile delivery robots. Machines 6, 33. doi: 10.3390/machines6030033
Hutton, L. (2022). Co-op introduces driverless delivery pods in Leeds. Highway News. Available online at: https://highways-news.com/co-op-introduces-driverless-delivery-pods-in-leeds/ (accessed February 12, 2023).
Jennings, D., and Figliozzi, M. (2019). Study of sidewalk autonomous delivery robots and their potential impacts on freight efficiency and travel. Transp. Res. Rec. 2673, 317–326. doi: 10.1177/0361198119849398
Jesus, T., Bhattacharjya, S., Papadimitriou, C., Bogdanova, Y., Bentley, J., Arango-Lasprilla, J. C., et al. (2021). Lockdown-related disparities experienced by people with disabilities during the first wave of the COVID-19 pandemic: scoping review with thematic analysis. Int. J. Environ. Res. Public Health, 18, 6178. doi: 10.3390/ijerph18126178
Kitchin, R., and Dodge, M. (2019). The (in) security of smart cities: vulnerabilities, risks, mitigation, and prevention. J. Urban Technol. 26, 47–65. doi: 10.1080/10630732.2017.1408002
Kosonen, P. (2020). Autonomous robots out in the wild—a software engineering challenge. Available online at: https://medium.com/starshiptechnologies/running-autonomous-robots-on-city-streets-is-very-much-a-software-engineering-challenge-66927869090a (accessed February 21, 2023).
Lääne, J. (2020). How Starship Delivery Robots Know Where they are Going. Starship Technologies. Available online at: https://medium.com/starshiptechnologies/how-starship-delivery-robots-know-where-they-are-going-c97d385a1015 (accessed February 21, 2023).
Lienert, P., and Lee, J. (2020). Automated Delivery Cashes in on Pandemic-Driven dEmand. Reuters. Available online at: https://www.reuters.com/article/us-health-coronavirus-delivery-robots-fo-idUKKBN22U1F8 (accessed February 21, 2023).
Lin, W. (2022). Automated infrastructure: COVID-19 and the shifting geographies of supply chain capitalism. Prog. Hum. Geogr. 46, 463–483. doi: 10.1177/03091325211038718
Local.gov.uk (2020). Shielding Package FAQs Pack: Local Authorities, Available online at: https://www.local.gov.uk/sites/default/files/documents/Shielding%20packages%20for%20FAQs%20for%20local%20authorities.pdf (accessed February 22, 2023).
Loke, S. W., and Rakotonirainy, A. (2021). “Automated vehicles, urban robots and drones: three elements of the automated City,” in The Automated City (Cham: Springer), p. 69–108. doi: 10.1007/978-3-030-82318-4_3
London Assembly (2017). Transport Committee—Transcript of Agenda Item 6—Future Transport. Available online at: https://www.london.gov.uk/about-us/londonassembly/meetings/documents/s66365/Appendix%201%20-%20transcript%20of%20item%206.pdf (accessed February 22, 2023).
Loopstra, R. (2020). Vulnerability to Food Insecurity Since the COVID-19 Lockdown—Preliminary Report. Available online at: https://foodfoundation.org.uk/wp-content/uploads/2020/04/Report_COVID19FoodInsecurity-final.pdf (accessed February 22, 2023).
MacKinnon, D., and Derickson, K. D. (2013). From resilience to resourcefulness: a critique of resilience policy and activism. Prog. Hum. Geogr. 37, 253–270. doi: 10.1177/0309132512454775
Macrorie, R., Marvin, S., and While, A. (2020). Robotics and automation in the city: a research agenda. Urban Geogr. 42, 1–21. doi: 10.1080/02723638.2019.1698868
Martin, C. J., Evans, J., and Karvonen, A. (2018). Smart and sustainable? Five tensions in the visions and practices of the smart-sustainable city in Europe and North America. Technol. Forecast. Soc. Change 133, 269–278. doi: 10.1016/j.techfore.2018.01.005
Marvin, S., While, A., Kovacic, M., Lockhart, A., and Macrorie, R. (2018). Urban Robotics and Automation: Critical Challenges, International Experiments and Transferable Lessons for the UK. EPSRC UK Robotics and Autonomous Systems (RAS) Network. doi: 10.31256/WP2018.1
Mattern, S. (2020). “A city is not a computer,” in Willis, K., and Aurigi, A. The Routledge Companion to Smart Cities (London: Routledge), p. 17–28. doi: 10.4324/9781315178387-2
Mavrogiannis, C., Alves-Oliveira, P., Thomason, W., and Knepper, R. A. (2022). Social momentum: Design and evaluation of a framework for socially competent robot navigation. ACM Trans. Hum. Robot Interaction 11, 1–37. doi: 10.1145/3495244
McGuirk, P., Dowling, R., Maalsen, S., and Baker, T. (2021). Urban governance innovation and COVID-19. Geograph. Res. 59, 188–195. doi: 10.1111/1745-5871.12456
Milton Keynes Council (2018). Minutes of the Meeting of the Cabinet Held on 6 March 2018, Made Available by MKC Democratic Services. Milton Keynes: MKC.
Mintrom, M., Sumartojo, S., Kulić, D., Tian, L., Carreno-Medrano, P., and Allen, A. (2022). Robots in public spaces: implications for policy design. Policy Design Practice 5, 123–139. doi: 10.1080/25741292.2021.1905342
MKFM (2020). Photo shows delivery robot stuck as floods continue to cause problems in Milton Keynes. Available online at: https://www.mkfm.com/news/local-news/photos-show-delivery-robot-stuck-in-water-as-rain-sweeps-through-milton-keynes/ (accessed February 21, 2023).
Morris, S., Weaver, M., and Khomami, N. (2018). Beast from the East meets storm Emma, causing UK's worst weather in years. Guardian. 2, 18.
Nasr Esfahani, H., Song, Z., and Christensen, K. (2022). A deep neural network approach for pedestrian trajectory prediction considering flow heterogeneity. Transp. Transport Sci. 19, 1–24. doi: 10.1080/23249935.2022.2036262
Northamptonshire Council (2021). In-demand delivery robot service expanding in Northampton to cover Barry Road and Upton. Available online at: https://www.northamptonshire.gov.uk/news/council-news/Pages/in-demand-delivery-robot-service-expanding-in-northampton-to-cover-barry-road-and-upton.aspx (accessed February 22, 2023).
O'Connell, M., de Paula, A. D., and Smith, K. (2021). Preparing for a pandemic: Spending dynamics and panic buying during the COVID-19 first wave. Fiscal Stud. 42, 249–264. doi: 10.1111/1475-5890.12271
Odendaal, N. (2021). Everyday urbanisms and the importance of place: exploring the elements of the emancipatory smart city. Urban Stud. 58, 639–654. doi: 10.1177/0042098020970970
Office for National Statistics (2020). Coronavirus and the Social Impacts on Disabled People in Great Britain. Available online at: https://www.ons.gov.uk/peoplepopulationandcommunity/healthandsocialcare/disability/articles/coronavirusandthesocialimpactsondisabledpeopleingreatbritain/may2020 (accessed February 22, 2023).
Pani, A., Mishra, S., Golias, M., and Figliozzi, M. (2020). Evaluating public acceptance of autonomous delivery robots during COVID-19 pandemic. Transp. Res. Part D Transp. Environ. 89, 102600. doi: 10.1016/j.trd.2020.102600
Pärnamaa, T. (2018). How Neural Networks Power Robots at Starship. Available online at: https://medium.com/starshiptechnologies/how-neural-networks-power-robots-at-starship-3262cd317ec0 (accessed February 22, 2023).
Peeters, M. M., van Diggelen, J., Van Den Bosch, K., Bronkhorst, A., Neerincx, M. A., Schraagen, J. M., et al. (2021). Hybrid collective intelligence in a human–AI society. AI Soc. 36, 217–238. doi: 10.1007/s00146-020-01005-y
Pinsent Masons (2021). Case Study—Paving the Way for Autonomous Last-Mile Delivery. Available online at: https://www.pinsentmasons.com/thinking/case-studies/paving-the-way-for-autonomous-last-mile-delivery (accessed February 22, 2023).
Renn, O., Klinke, A., and Van Asselt, M. (2011). Coping with complexity, uncertainty and ambiguity in risk governance: a synthesis. Ambio. 40, 231–246. doi: 10.1007/s13280-010-0134-0
Research Institute for Disabled Consumers (2020). RIDC COVID-19 Survey. Available online at: http://www.ridc.org.uk/sites/default/files/uploads/Research%20Reports/Covid19_030420/RiDC_Covid19_FullResults_030402020.pdf (accessed February 22, 2023).
Roe, E. (2020). “Control, manage or cope? A politics for risks, uncertainties and unknown-unknowns,” in The Politics of Uncertainty (London: Routledge), p. 73–84. doi: 10.4324/9781003023845-5
Sadowski, J. (2022) “Planetary Potemkin AI: The humans hidden inside mechanical minds,” in Graham, F. ed. Digital Work in the Planetary Market (Cambridge MA: MIT Press), p. 229–40.
Salvini, P., Paez-Granados, D., and Billard, A. (2021). Safety concerns emerging from robots navigating in crowded pedestrian areas. Int. J. Soc. Robot. 41, 1–22. doi: 10.1007/s12369-021-00796-4
Schroeder, R. G., Van de Ven, A. H., Scudder, G. D., and Polley, D. (2000). “The development of innovation ideas,” in Van de Ven, A. H., Angle, H. L., and Poole, M. S. Research on the Management of Innovation: The Minnesota Studies (Oxford: Oxford University Press).
Shirbon, E. (2020). These Robots are Delivering Groceries to UK Doorsteps in the Pandemic. Available online at: https://www.weforum.org/agenda/2020/04/robots-united-kingdom-uk-coronavirus-covid19-ai (accessed February 12, 2023).
Spatola, N., and Wudarczyk, O. A. (2021). Ascribing emotions to robots: Explicit and implicit attribution of emotions and perceived robot anthropomorphism. Comput. Human Behav. 124, 106934. doi: 10.1016/j.chb.2021.106934
Starship (2019). Starship Technologies celebrates 50,000 autonomous deliveries. Available online at: https://www.starship.xyz/press_releases/starship-technologies-celebrates-50000-autonomous-deliveries/ (accessed February 12, 2023).
Starship (2021a). Environmental Impact of Delivery Robots in Milton Keynes Revealed for the First Time during COP26. Available online at: https://www.starship.xyz/press_releases/environmental-impact-of-delivery-robots-in-milton-keynes-revealed-for-the-first-time-during-cop26/ (accessed February 12, 2023).
Starship (2021b). MK Community: Updates to Our Pricing and NHS Discounts. Available online at: https://starshipdeliveries.com/mkupdates/ (accessed February 12, 2023).
Starship (2021c). Starship Technologies Advances Adoption of Autonomous Delivery as Demand Quadruples in The Last Year. Available online at: https://www.starship.xyz/press_releases/starship-technologies-advances-adoption-of-autonomous-delivery-as-demand-quadruples-in-the-last-year/ (accessed February 12, 2023).
Starship (2022). Co-Op And Starship Technologies To Expand Partnership To 500 Robots Across Five New Towns and Cities To Cut Carbon On Last Mile Deliveries. Available online at: https://www.starship.xyz/press_releases/co-op-and-starship-technologies-to-expand-partnership-to-500-robots-across-five-new-towns-and-cities-to-cut-carbon-on-last-mile-deliveries/ (accessed February 12, 2023).
Sumartojo, S., and Lugli, D. (2022). Lively robots: robotic technologies in COVID-19. Social Cult. Geography 23, 1220–1237. doi: 10.1080/14649365.2021.1921245
Sumartojo, S., Lundberg, R., Kulić, D., Tian, L. Carreno-Medrano, P., Mintrom, M., et al. (2022). The Robotic Production of Spatiality: Predictability, Partitioning, and Connection. Hoboken, NJ: Transactions of the Institute of British Geographers. doi: 10.1111/tran.12574
Sumartojo, S., Lundberg, R., Tian, L., Carreno-Medrano, P., and Kulić, D. (2021). Imagining public space robots of the near-future. Geoforum 124, 99–109. doi: 10.1016/j.geoforum.2021.06.006
Swyngedouw, E. (2006). Circulations and metabolisms:(hybrid) natures and (cyborg) cities. Sci. Cult. 15, 105–121. doi: 10.1080/09505430600707970
UK Department for Environment, Food Rural and Affairs (2020). New Measures on Night Time Deliveries to Supermarkets to Support Coronavirus Response. Available online at: https://www.gov.uk/government/news/new-measures-on-night-time-deliveries-to-supermarkets-to-support-coronavirus-response (accessed February 22, 2023).
UK Parliament (2020). Unequal Impact? Coronavirus, Disability and Access to Service, Available online at: https://publications.parliament.uk/pa/cm5801/cmselect/cmwomeq/1050/105005.htm (accessed February 22, 2023).
Valdez, M., Cook, M., and Potter, S. (2021). “Humans and robots coping with crisis–Starship, COVID-19 and urban robotics in an unpredictable world,” in 2021 IEEE International Conference on Systems, Man, and Cybernetics (SMC) (IEEE), p. 2596–2601. doi: 10.1109/SMC52423.2021.9658581
Wang, R., Nakhimovich, D., Roberts, F., and Bekris, K. (2021). “Robotics as an enabler of resiliency to disasters: promises and pitfalls,” in Resilience in the Digital Age (Cham, Switzerland: Springer International Publishing), p. 75–101. doi: 10.1007/978-3-030-70370-7_5
While, A., Marvin, S., and Kovacic, M. (2021). Urban robotic experimentation: San Francisco, Tokyo and Dubai. Urban Stud. 58, 769–786. doi: 10.1177/0042098020917790
Woo, J., Whittington, J., and Arkin, R. (2020). Urban robotics: achieving autonomy in design and regulation of robots and cities. Conn. L. Re., 52, 319.
Yigitcanlar, T., Butler, L., Windle, E., Desouza, K. C., Mehmood, R., and Corchado, J. M. (2020). Can building “artificially intelligent cities” safeguard humanity from natural disasters, pandemics, and other catastrophes? An urban scholar's perspective. Sensors, 20, 2988. doi: 10.3390/s20102988
Yigitcanlar, T., Corchado, J. M., Mehmood, R., Li, R. Y., Mossberger, K., and Desouza, K. (2021). Responsible urban innovation with local government artificial intelligence (AI): a conceptual framework and research agenda. J. Open Innovat. Technol. Market Complex. 7, 71. doi: 10.3390/joitmc7010071
Yigitcanlar, T., and Cugurullo, F. (2020). The sustainability of artificial intelligence: An urbanistic viewpoint from the lens of smart and sustainable cities. Sustainability 12, 8548. doi: 10.3390/su12208548
Yigitcanlar, T., Degirmenci, K., and Inkinen, T. (2022). Drivers behind the public perception of artificial intelligence: insights from major Australian cities. AI Soc. 1–21. doi: 10.1007/s00146-022-01566-0
YouGov (2020). YouGov Survey Results—Food Foundation. Available online at: https://docs.cdn.yougov.com/otidumbhkq/YouGov%20Survey%20Results%20-%20Food%20Foundation.pdf (accessed February 22, 2023).
Zhao, Z., Ma, Y., Mushtaq, A., Rajper, A. M. A., Shehab, M., Heybourne, A., et al. (2022). Applications of robotics, artificial intelligence, and digital technologies during COVID-19: a review. Disaster Med. Public Health Prep. 16, 1634–1644. doi: 10.1017/dmp.2021.9
Keywords: urban robots, urban AI, artificial intelligence, robotic and automated systems, AI-human interaction, collective intelligence, delivery robots
Citation: Valdez M and Cook M (2023) Humans, robots and artificial intelligences reconfiguring urban life in a crisis. Front. Sustain. Cities 5:1081821. doi: 10.3389/frsc.2023.1081821
Received: 27 October 2022; Accepted: 16 March 2023;
Published: 05 April 2023.
Edited by:
Gregory Patrick Trencher, Kyoto University, JapanReviewed by:
Casey Lynch, University of Twente, NetherlandsCian McCarroll, Trinity College Dublin, Ireland
Copyright © 2023 Valdez and Cook. This is an open-access article distributed under the terms of the Creative Commons Attribution License (CC BY). The use, distribution or reproduction in other forums is permitted, provided the original author(s) and the copyright owner(s) are credited and that the original publication in this journal is cited, in accordance with accepted academic practice. No use, distribution or reproduction is permitted which does not comply with these terms.
*Correspondence: Matthew Cook, bWF0dGhldy5jb29rQG9wZW4uYWMudWs=