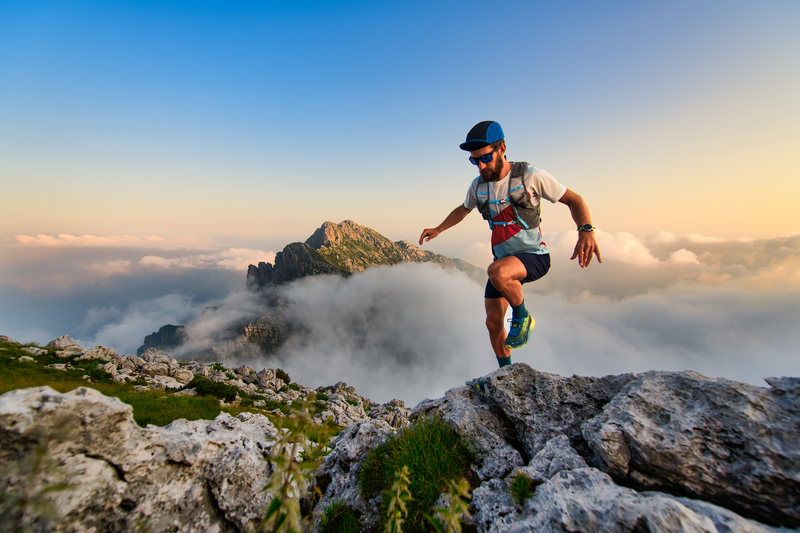
95% of researchers rate our articles as excellent or good
Learn more about the work of our research integrity team to safeguard the quality of each article we publish.
Find out more
ORIGINAL RESEARCH article
Front. Sustain. Cities , 29 September 2022
Sec. Health and Cities
Volume 4 - 2022 | https://doi.org/10.3389/frsc.2022.876945
This article is part of the Research Topic Insights in Health and Cities: 2021 View all 5 articles
Social vulnerability is directly addressed by contemporaneous health research to improve social equity. Existing disadvantage indices capture vulnerability to COVID-19 pandemic less accurately. Health-related studies apply the indices to relate overall vulnerability to health outcomes including severe COVID-related health outcomes leading to hospitalization and death. The multi-variable indices are based on various attributes, some unrelated to COVID-19 severity. Some studies that use multi-attribute indices don't find meaningful association with COVID-19 health outcomes. Additionally, current research is lacking on health impacts of social disadvantage during various COVID-19 stages. Thus, due to variations in inequality in exposure and pandemic susceptibility, community health should be assessed at different time points. To fill this gap, we develop an index using six indicators capturing pandemic vulnerability. We apply it in a retrospective case study of a relationship between social disadvantage and morbidity and mortality due to COVID-19 focusing on disadvantaged communities set in Tennessee with a concentration of vulnerability and rurality. COVID-19-related health outcomes were tested for associations with a social disadvantage index (SDI) for earlier (2021) and later (2022) pandemic stages. First, we describe a methodology to create a social disadvantage index to identify communities at risk for severe COVID-19-related health outcomes based on the CDC guidance. Second, we highlight the differences in COVID-19 health outcomes over time as we examine health disparities associated with social disadvantage during various pandemic stages. To understand how the findings would differ if the same analyses were to be carried out using other vulnerability indices, we apply analyses to areas based on the CDC's-based Social Vulnerability Index and find strong statistical association between the two indices and substantial overlap regarding disadvantaged areas identified and similar health outcome findings. A better understanding of whether residents of socially disadvantaged areas experience worse COVID-related health outcomes can help determine whether policies need to target not only individuals but entire communities to improve outcomes. The developed index based on six indicators is thus especially suitable during the COVID-19 disaster to efficiently identify vulnerable communities and address social equity.
Social vulnerability should be addressed explicitly to improve social equity. In the United States, more than 2,000 COVID-19 deaths have been reported each day for February 2022. As of the week of February 14 through 20, 2022, the World Health Organization (WHO) reported over 422 million confirmed cases and over 5.8 million deaths globally, while the United States of America was among the regions with the highest numbers of new weekly cases (746,129 new weekly cases, or 225.4 new cases per 100, 000) only following the Russian Federation, Germany, and Brazil; while reporting the highest number of new weekly deaths globally (14,723 new deaths, or 4.4 new deaths per 100,000) (WHO, 2022). Policy- and decision-makers need guidance on how to justify the scarce resource allocation decisions (Scully, 2020) including identification of areas that might become local infection epicenters requiring support such as where to place a clinic, a field hospital, testing sites or construct make-shift health centers and hospitals while making decisions that are just and fair. Recent study reveals that vaccinations alone using the current vaccines will likely be insufficient to offer some protection against household transmission and decrease COVID-19 transmission over a long term within a population requiring use of other restrictions to control transmission (Lyngse et al., 2021). According to the February 2022 COVID-19 Weekly Epidemiological Update by WHO (WHO, 2022), while Delta variant still has substantial reported circulation, the Omicron variant of SARS-CoV-2 is predominant circulating variant globally and the overall global risk related to it is high due to the shorter generation time (the period between getting infected to the time of infecting another person) and a higher risk of reinfection with Omicron compared to other SARS-CoV-2 variants (Lyngse et al., 2021). With many people being sick simultaneously, healthcare systems and local economies (due to absences from work across different sectors and education) even in communities with a high vaccination coverage may have substantial pressure and strain (European Centre for Disease Prevention and Control, 2022).
Accurate identification of the areas more susceptible to COVID-19 using consistent criteria is thus important for decision makers in informing the most appropriate strategies for epidemic control in various scenarios. This paper contributes to the current literature by addressing the issue of social and health equity and developing the consistent methodology for identification of socially vulnerable communities and applying it in the COVID-19 health case study in Tennessee. As of February 23, 2022, Tennessee ranked the fourth highest in total cases per 1 mln people (292,261) and the 6th highest in total number of deaths per 1 mln people (3,562) among other states (https://www.worldometers.info/coronavirus/).
This study has three objectives. First, it applies a methodology using a social disadvantage index (SDI) created to locate vulnerable communities using simple and yet robust measures of vulnerability especially suitable during the COVID-19 disaster. The index is based on a combination of several known COVID-19 risk drivers. Thus, it simultaneously captures multiple risk factors mediating the COVID-19-related outcomes. Second, it examines health disparities during COVID-19. Studies identifying socially vulnerable areas are needed for the following reason. Since resources are still limited, the more socially vulnerable communities can be higher prioritized regarding support due to their residents' greater social vulnerability to COVID-19 (Karaye and Horney, 2020). Second, we study whether living in vulnerable communities significantly impacts the COVID-19-related health outcomes including morbidity and mortality. For this, we will use case rates to compare the disease sickness (measured as case rates or cases per 100,000 people, and as case rates 7-day average), disease severity (measured by case fatality ratio, or CFR) and mortality (measured by death rates or deaths per 100,000 people, and rates 7-day average) between communities with “vulnerable demographics” and other neighborhoods. Third, we need to understand how the findings would differ if the same analyses were to be carried out using other vulnerability indices such as the CDC-based Social Vulnerability Index (SVI) (described in the Background section). Accordingly, we identify high-disadvantaged communities in the study area applying the CDC's-based SVI, carry out analyses to these areas comparing the COVID-19-related health outcomes, and look for statistical association between the two indices (the SDI proposed here and the SVI developed by the CDC) by conducting Pearson correlation between the SDI and the CDC-based SVI. A methodological flowchart for this study is presented in Figure 1. Finally, the impact of social disadvantage on economic wellbeing grew stronger as the pandemic progressed compared with the pre-pandemic period (Antipova and Momeni, 2021), thus, health assessment at different time points particularly for disadvantaged communities is needed due to likely variations in inequality in exposure and pandemic susceptibility (Karaye and Horney, 2020).
Motivated by our research questions, we tested the following four hypotheses:
(1) COVID-19-related sickness is higher for socially disadvantaged communities compared to other communities.
(2) The severity of COVID-19-related disease measured by the case-fatality ratio (CFR) is higher in socially disadvantaged communities.
(3) COVID-19-related mortality is higher in socially disadvantaged communities.
(4) The role of social disadvantage may change as society develops health-promoting strategies of population protection (e.g., COVID-19 vaccination).
The paper is organized as follows. Section Introduction is the Introduction with the problem statement, significance and objectives of the research. Section Background Information, provides literature review and describes the various vulnerability indices developed previously. Section Risk factors of severe COVID-19 health outcomes lists recently identified risk factors contributing to a greater severity from COVID-19 which could be used to develop the Social Disadvantage Index (SDI) to identify communities with a high percent of population-at-risk, followed by the Methodology presenting study area, representations periods, development of the Social Disadvantage Index (SDI), data and their sources, and statistical analyses in Section Methodology. Section Results presents and explains the results. Finally, sections Discussion and Conclusion offer Discussion and Conclusion.
Literature is increasingly in consensus on the critical role played by social and environmental factors including socioeconomic status, education, employment in both determining health and health-related outcomes (Marmot et al., 1991; WHO Commission on Social Determinants of Health and WHO, 2008), and contributing to health inequity (Schroeder, 2007; Committee on the Recommended Social and Behavioral Domains and Measures for Electronic Health Records, Board on Population Health and Public Health, Practice, Institute of Medicine, 2015). The World Health Organization termed these factors “social determinants of health” because the various conditions of places surrounding an individual from the moment a person is born and where they are growing, living, working, and aging, have the ability to shape their health during various life stages. Social determinants of health play increasingly greater role in who suffers the most severe outcomes from COVID-19 especially after the Omicron virus spread rapidly across the US and became so widespread affecting disadvantaged communities particularly hard. Low socioeconomic status and educational attainment, unemployment, and other factors of disadvantage can make individuals less resilient and more vulnerable including worse health outcomes. Accordingly, attempts have been made to quantify social vulnerability (interchangeably termed disadvantage) by developing various vulnerability indices generally termed Social Vulnerability Indices (SVIs) based on analyses at multiple geographies for various purposes (Clark et al., 1998; Cutter et al., 2003; Saltelli, 2007; Tate, 2012; Birkmann, 2013).
Disaster studies explored the relationships between hazard exposure, resident demographics, and social vulnerability to improve disaster outcomes (Horney et al., 2015). Vulnerable populations have greater susceptibility and are affected disproportionately by disasters (Horney et al., 2015). After the disastrous event strikes, these groups are likely to experience structural and non-structural losses, have impaired coping capacity to the adverse effects of various hazards, and suffer the most severe adverse outcomes often requiring external assistance. As vulnerability modeling expanded from academic research into hazard mitigation and recovery planning (Drakes et al., 2021), social vulnerability measures are widely used to gauge the differential susceptibility of populations to disaster outcomes including mortality (Johnson et al., 2012), economic loss (Yoon, 2012), displacement (Esnard et al., 2011; Hamideh and Rongerude, 2018), and public assistance (Lue and Wilson, 2017).
Indices are used by policymakers and hazard vulnerability research to better understand the proportion of the population at risk and develop optimal strategies for various purposes (Flanagan et al., 2011; CDC, 2018). Using the SVIs, the communities impacted by hazardous events such as a tornado, or a chemical spill, or a disease outbreak and that need assistance may be mapped. For disaster management (Horney et al., 2015) and hazard planning (Flanagan et al., 2011), the Centers of Disease Control and Prevention (CDC) developed the Social Vulnerability Index (SVI) for mitigation of disaster vulnerability in communities as certain categories remain vulnerable at all stages of a disaster including people in poverty, racial and ethnic minorities, children, elders, disabled people and people living in certain types of housing. Using the 2016 and 2018 American Community Survey (ACS) 5-year data, the CDC has created the SVI available at the US tract- and county-level.
The CDC's SVI index determines social vulnerability of a community based on 15 variables grouped into four related themes including socio-economic status, household composition and disability, minority status and language, and housing and transportation. The variables include unemployment, minority status, disability, and others. The top 10% of the areal units are assigned high vulnerability, that is, a value of 1 is given when their values are at the 90th percentile. Areal units in the bottom 90% are given a value of 0 to indicate low vulnerability.
Hazards and Vulnerability Research Institute (2016) of the University of South Carolina created a different Social Vulnerability Index (SoVI) for the United States using twenty-eight variables from the 2010–2014 American Community Survey (ACS) data at the county level including % population under 5 years, median age, % female, % Asian, and other variables. In health research, similarly, understanding the contextual factors related to decreased morbidity and mortality is key to address future threats over the long-term (Bollyky et al., 2022), however, collecting socially determined health records data electronically is time-consuming and resource intensive (Chakravarthy et al., 2021). Thus, yet another index, an Area Deprivation Index (ADI) (ADI, University of Wisconsin School of Medicine and Public Health, 2019; 2013 version) uses readily available metrics of social determinants of health conveniently summarized into a single geocoded index (Chakravarthy et al., 2021). It has been created as a socially based health index which uses sociodemographic information aggregating data at census tracts. An ADI is a comprehensive socioeconomic deprivation index consisting of 17 indicators reflecting a diverse set of socioeconomic variables (Kind and Buckingham, 2018). The foundational work in this area has been used at a finer spatial unit (ZIP code area). Uniformly across various indices, higher scores indicate greater vulnerability.
The indices are used by the various hazard-related policies and studies. Thus, population health studies use various SVIs relating overall social vulnerability and health outcomes to explore the spatial and statistical association between social vulnerability and various outcomes. Recently, a Social Vulnerability Index (SoVI) was employed to study the links between short-term disaster assistance and social vulnerability finding a greater concentration of socially vulnerable populations underserved in regard to disaster assistance in certain areas across the contiguous US (Drakes et al., 2021). Drakes et al. (2021) scaled all input variables by using z-score standardization, then reduced a large variable set using principal components analysis to seven principal components, and next summed up the latent factors into an index.
Some health studies relied on the CDC-based Social Vulnerability Index due to its relatively higher accuracy compared with other models while conforming with other social vulnerability indices (Tate, 2012; Bakkensen et al., 2017; Karaye and Horney, 2020). However, past research noted inherent limitations of the CDC's SVI including its lower precision and weaker internal validity (Tate, 2012; Rufat et al., 2019; Karaye and Horney, 2020). Using the Area Deprivation Index (ADI) at residential zip codes, Unger et al. (2021) examined survival outcomes for major cancers finding a continuous connection between increase in risk of all outcomes and increase in the ADI with the patients from areas in the highest ADI quintile having worse survival. Yet other studies found weak associations using other multi-attribute vulnerability indices. A recent study assessed a patient's risk for HPV-related cancer by looking at the relationship between HPV-related cancers and the Area Deprivation Index (ADI) in Tennessee, finding no association between the stage of human papillomavirus (HPV)-related cancer at diagnosis and either aggregate or individual census variables (Chakravarthy et al., 2021). Recent disaster-related research by Drakes et al. (2021) using the index developed by the Hazards & Vulnerability Research Institute of the University of South Carolina and based on 28 American Community Survey (ACS) variables, found only the weak explanatory power of most social vulnerability indicators suggesting that social characteristics do not significantly influence disaster aid disbursement. The indices have been applied by the COVID-19 research. The Lancet-published study found no meaningful association between COVID-19 health outcomes including infection rates and fatality and pandemic-preparedness indices capturing health security capacity (Bollyky et al., 2022).
Thus, despite their obvious usefulness in disaster research and policies, the existing social vulnerability indices may have limitations regarding their applicability to the current pandemic. It is important to accurately identify vulnerable communities which are slower to recover from adversity and have a larger share of their citizens needing quicker protection from COVID-19 than counties that are better resourced and able to recover more readily. As the Tennessee Department of Health made efforts to identify communities at higher risk for poor COVID-19 outcomes using the CDC-based SVI, the index underestimated disadvantaged counties and thus requiring re-evaluation. Counties were manually added after underestimation by the national social vulnerability index in its COVID-19 vaccination efforts (Tennessee Department of Health, 2021). The counties in TN that have been missed by the CDC-based SVI proved at higher risk for poor outcomes from COVID-19 than more than two-thirds of counties in the United States, some already designated as economically distressed by the State of Tennessee (Tennessee Department of Health, 2021). As mentioned earlier, the CDC-based SVI is primarily targeted for hazard-related studies and policies, while it may be less applicable for the current COVID-19 pandemic.
Another recent COVID-related study found that the SVI index variables do not explain well the variability in COVID-19 case counts even when other factors are controlled for including demographic and environmental factors (such as population size and density, people tested, mean daily sunlight, precipitation, air temperature, heat index, and PM2.5), with SVI variables accounting for under 39% warranting other studies examining more relevant factors to help understand the burden of the pandemic (Karaye and Horney, 2020). Additionally, various stages in the pandemic may exhibit variations in inequality in exposure and pandemic susceptibility warranting studies further to examine the rate of increase in confirmed case counts at different time points for counties (Karaye and Horney, 2020).
One issue related to applicability to the COVID-19 pandemic may be inclusion of some variables critical in evacuation efforts and thus, relevant to hazardous events but less related to severe health outcomes of the COVID-19 pandemic. Some demographic variables that have been used in creating the SVI metric are not included into the current CDC list of conditions carrying higher risks to severe illness from COVID-19 infection. The latter is defined as admission to hospital or the intensive care unit, mechanical ventilation, or death (CDC, 2022a). For example, included to the variables used in creating the SVI metric is a number of young people (defined as people younger than 17 years of age). However, young people who comprise 22% of the U.S. population (U. S. Census Bureau, 2021), have been shown to be less affected by COVID-19. Thus, the current CDC criteria do not include this category as an increased risk factor predisposing population to severe illness from the COVID-19 infection, with fewer cases and lower hospitalization rates reported in children (age 0–17 years) compared with adults (although there is limited evidence that children with certain underlying conditions have a suggestive higher risk for severe COVID-19 outcomes). Another age-related demographic variable that was used in creating the vulnerability indices was people who are aged 65 or older, however, despite the increased risk of death from COVID, after vaccines became available, older people have higher vaccination rates in the US (at about 90% of seniors 65 and older who are fully vaccinated), thus, may not capture well community vulnerability that are at higher risk for severe outcomes. Other variables included into the vulnerability indices calculation are estimates of female and Asian (used by the SoVI), while both categories are not included by the current CDC criteria as increased risk conditions predisposing population to severe illness from the COVID-19 infection.
Past studies relate separate census-based area-level measures such as poverty, education, racial/ethnic minority status to health outcomes. These studies report a high variation across findings for people in the lowest socioeconomic categories. As explained above, the existing social disadvantage indices that identify vulnerable communities are based on multi variables while not all variables may contribute to the severe COVID-19-related health outcomes including more severe infection leading to hospitalization and death. This research is motivated by a desire to develop a method based on CDC risk factors and current research findings that would identify communities at higher risk to COVID-19 severity and that might be more susceptible to the negative impacts of the current COVID-19 epidemic. The method also uses fewer variables and still efficiently identifies such communities. The method is then applied to identify socially disadvantaged communities in Tennessee to understand the proportion of the population at risk of severe COVID-19 infection, based on current CDC guidance (Ajufo et al., 2021), and compares health outcomes across communities. Specifically, our research questions are the following: (1) where are the communities that might be most susceptible to the negative impacts of the COVID-19 epidemic? (2) what is the impact of social disadvantage upon health outcomes? and (3) does the impact evolve? As we answer these questions, we compare health outcomes in the identified disadvantaged communities to those in other more privileged communities.
A better understanding of whether residents of socially disadvantaged areas experience worse COVID-related and other health outcomes can help determine whether policies needed target not only individuals but entire communities to improve outcomes (Unger et al., 2021) and ensure social equity. Accordingly, this research contributes to the development of COVID-19-related guidance for various purposes, for example, establishing where efforts are needed to allocate the COVID-19 testing, develop community engagement strategies that are culturally sensitive to increase the confidence that individuals have in public health guidance to increase vaccination coverage to better protect especially disadvantaged communities (Bollyky et al., 2022). An understanding of the concentration of the most vulnerable population at risk of severe COVID-19 infection prior to and following vaccination, could contribute to this and other health promoting efforts in a meaningful way.
The study seeks to explore the impacts of social disadvantage on public health in unprecedented times of the COVID-19 pandemic. For this, we first reviewed the literature of the drivers of the COVID-19 infection as well as factors of social disadvantage because disadvantaged communities suffer a greater burden due to being already vulnerable prior to the COVID-19 pandemic and may have higher numbers of confirmed and probable cases and deaths in the community. Second, we group the identified risk factors into the broader risk categories to help guide the selection of factors for each category.
In a growing body of research on COVID consequences published during the past several months, a recent study set in NY analyzed health outcomes among five NYC boroughs, found that among these communities, the highest rates of COVID-19-related testing, hospitalizations and deaths were in the Bronx which also has the highest shares of racial and ethnic minorities, persons in poverty, and the lowest educational levels, while the most affluent borough, Manhattan had the lowest rates for hospitalizations and deaths (Wadhera et al., 2020). While both areas had the highest number of per capita hospital beds, and the highest population density in Manhattan, other factors may explain the disparate outcomes among communities including chronic underlying medical conditions, potential workplace exposures, socio-economic attributes, and race-based structural inequities. Figure 2 presents variation in population density, morbidity and mortality rate in New York City boroughs using the COVID-19 data for July 5, 2020. Within a particular city, in various parts of the city (not necessarily the densest, but with lower population density) such as Brooklyn or Queens in NYC there are more people per household and renters living in overcrowded conditions and have higher rates of infection, while in areas with higher population density including Manhattan (Figure 2) where more people had college degrees fewer people got sick and have lower rates of infection, thus, the important thing is choosing an appropriate small scale such as the number of people per unit of housing allowing the virus an easy transmission when people share an enclosed cramped space and are in close contact with an infected person for prolonged time. Due to unequal socio-spatial residential distribution, the lockdown may disproportionately negatively affect already vulnerable social groups than more affluent residents translated in differences in the quantity of cases of coronavirus. Where more people share housing and confined spaces and where families of five or six per room can be found, cases of coronavirus are most frequent (McDonald and Spotswood, 2020). At the same time, infection clusters emerged where workers are shoulder-to-shoulder for extended period of time across less-dense, rural America including meatpacking or poultry processing plants, or state prisons.
Figure 2. Population density, morbidity and mortality rate in New York City boroughs using the COVID-19 data for July 5, 2020.
A recent paper (Kontis et al., 2020) examines an excess mortality and relative increase in death across 21 industrialized countries in Europe and Australia and New Zealand identifying key determinants that affected the total death toll for the first wave of the COVID-19 pandemic in a country which include the socio-economic and demographic attributes of individuals and the baseline features of communities people live in, the response policies such as a lockdown, isolation of cases and their contacts, and movement restrictions that interrupt transmission and positively affect mortality, however, may negatively impact deaths due to social isolation and denial of essential services, and preparedness and resilience of the public health and social care infrastructure (whether the public health and health and social care systems are well-prepared, and resilient) (such as poverty rate, health expenditures as percent of GDP, the number of hospital beds per 1,000 people, the number of critical care beds per 1,000 people, the number of beds in nursing and residential facilities per 1,000 people). Individual- and community-level characteristics comprising baseline demographic and health features include percent population with diabetes, obesity, high blood pressure, life expectancy, social networks and inequalities in social and material resources employment status and occupation, and access to transport and housing. These can make individuals and communities either vulnerable to the virus spread and adverse health consequences of infection, or resilient. Regarding individual-level characteristics, increasing age, resource deprivation and underlying long-term health conditions such as obesity, diabetes and vascular and kidney diseases increase the risk of death from COVID-19 (Kontis et al., 2020).
The above factors have been documented by recent studies contributing to the spread of the infection and higher rates of morbidity and mortality that broadly can be grouped as COVID-19-related epidemiological vulnerability, virus transmission vulnerability, and overall wellbeing vulnerability (Wilkinson et al., 2020) (see below). Knowing where these drivers concentrate, might help identify “high-priority areas” and support priority-based local actions to mitigate community spread in a timely fashion and might prove relevant to future humanitarian and health crises while taking community-based actions may prevent catastrophes (Sharifi and Khavarian-Garmsir, 2020).
Ecological characteristics: include population density as importantly associated with COVID-19 due to its relation to virus transmission vulnerability (Wilkinson et al., 2020) as high-density settings contribute to the faster spread of COVID-19. Cities which concentrate large shares of population and economic activities, are vulnerable to higher concentrations of COVID-19 infections. The spatial pattern of high COVID-19 mortality generally tracks those of population density. The acceleration of dissemination is higher in high-density urban areas due to the potential for seeding the broader community (Rocklöv and Sjödin, 2020; Schuchat, 2020). Therefore, neighborhoods that have high population density and which generate a substantial urban footprint due to high-rise buildings or apartment blocks belong to factors associated with the pandemic transmission. Population density might also play a significant role in the acceleration of transmission since COVID-19 is primarily transmitted by respiratory droplets [Morbidity and Mortality Weekly Report (MMWR), 2020]. For example, the New York metropolitan area characterized by high density, population reliance on mass transit where close contact occurs on a frequent and prolonged basis, and household spread due to high prevalence of apartment living, had the very high numbers of COVID-19 cases (Schuchat, 2020).
Population density may function as a useful indicator for a greater vulnerability for COVID transmission at certain spatial scales and have a role in per capita infection rates at smaller scales (such as interstate level) and a transit network including subways for urban areas which seem to help to transmit virus among passengers. However, there are other factors related to transmission vulnerability that hinder social distancing including multi-generational households, and a number of incarcerated population. An appropriate scale is needed such as the rooms where people live and work. Multi-generation households can contribute to high case-fatality rates (Bayer and Kuhn, 2020). Reitsma et al. (2021) define “high-exposure-risk” households as those with one or more essential workers and fewer rooms than inhabitants.
Epidemiological vulnerability: Multiple studies have reported characteristics linked with severe COVID-19 infection with consistent findings (Ioannou et al., 2020; Kabarriti et al., 2020). Underlying health conditions represent epidemiological vulnerability since both hospitalizations and fatality rates due to COVID-19 may be impacted by this factor, with obesity, hypertension, and diabetes (in this order) being the top risks of poor health outcomes among over 900,000 people hospitalized in the US due to coronavirus disease over 2019 through November 2020 (O'Hearn et al., 2021). Obesity is among the twelve medical conditions identified by the CDC that indicate with sufficient evidence an increased risk of severe COVID-19 infection including hospitalization, need for intensive care, or death among adults of any age (CDC Risk Criteria). Using current CDC guidance, a recent study examined the prevalence of twelve medical risk factors of severe COVID-19 infection and estimated the share of the US population at increased risk and identified obesity as the most prevalent risk condition (96.4 mln, or 41.3%) among 233.8 million of the U.S. adults (≥18) (Ajufo et al., 2021). The 2022 Lancet-based study used mean body-mass index as a measure of obesity as a factor to estimate cumulative case fatality ratio using multivariate linear modeling: the factor explains variation in COVID-19 (Bollyky et al., 2022). Obesity prevalence is a leading factor related to COVID-19 infection among most predictive covariates in a study modeling infection-fatality rate and infection-hospitalization rate models (COVID-19 excess mortality collaborators, 2022). Other underlying conditions, including hypertension, cardiovascular disease, chronic obstructive pulmonary disease, and cancer, are already known to significantly decrease the chance of recovery from the severest form of COVID-19 (Wu and McGougan, 2020).
These poor outcomes are preventable if these medical conditions are minimized. Many of the already existing disparities unfold during COVID-19. Many disadvantaged communities are disproportionally impacted by health inequalities. The various direct and indirect factors including underlying health conditions such as advanced age, sex (male), obesity (Cariou et al., 2020; Simonnet et al., 2020) and multimorbidity (Chow et al., 2020; Richardson et al., 2020) make these individuals of these communities more vulnerable to COVID-19. Huge health disparities are evident by race/ethnicity reflected in both COVID-19 mortality and burdens of health conditions: Black communities are disproportionately affected by higher death rates due to the COVID-19 virus (Thebault et al., 2020), unemployment, and financial troubles. Thus, identifying communities as future “hot spots” where disadvantage exists and therefore where disadvantage and increased risk of COVID-19 intersect is of upmost importance.
Economic stability: Poverty is an indicator of economic stability which is a Social Determinant of Health (Healthy People, 2030). Recent studies identify low socioeconomic status (as a measure of economic stability) a risk factor for poor health outcomes due to poor access to resources such as medical, technical, and financial (Arpey et al., 2017; McMaughan et al., 2020). Prior research reports the association between low socioeconomic status and poor health outcomes for many diseases (Cutler et al., 2008; Chen and Miller, 2013). Residents in socioeconomically deprived areas have limited access to healthcare and other resources (Unger et al., 2021), and residents of economically distressed counties have higher risk for poor COVID-19 outcomes (Tennessee Department of Health, 2021). Socioeconomic attributes strongly impact health outcomes even under normal circumstances with socially disadvantaged groups more likely having worse health outcomes compared to those experienced among more affluent populations groups (Antipova, 2020). Some racial groups disproportionally work jobs that cannot be done from home leading to higher risks of exposure. A greater proportion of the US overall and younger population with less education and lower income were found to be at increased-risk of severe COVID-19 infection (Ajufo et al., 2021). Individuals who reside in a lower socioeconomic area more likely to practice high-risk health behaviors, including smoking, obesity, physical inactivity, alcohol use, and poor diet (Blackwell et al., 2014; Heymach et al., 2018).
Similarly to minorities, those in poverty represent a vulnerable group disproportionately affected by the COVID-19 as they have been hard hit making urban poor a disadvantaged category. A study by the New York Times found that low-income city residents are affected by an inequality in hospital care making low income a risk factor for higher mortality among those with confirmed SARS-CoV-2 infection. The disparities in mortality appeared among community hospitals (with lower staffing, worse equipment and less access to advanced treatments) and the private, well-financed academic medical centers in the wealthiest parts of Manhattan, with patients' deaths three times greater in the former during the peak of the pandemic in April (Rosenthal et al., 2020). Using daily SARS-CoV-2 cases and deaths for 177 countries and territories and 181 subnational locations between Jan 1, 2020, and Sept 30, 2021, GDP per capita (as a measure of wealth) was among the factors that explained the most variation in cumulative case rates (Bollyky et al., 2022). Generally, low-income residents suffer from a combination of various factors including housing crowdedness as they tend to reside in smaller apartments, insufficient access to basic resources, and precarious livelihoods. In poorer neighborhoods where more people of color live in multiunit structures and in multigenerational households, the ability to practice social distancing is compromised and few options exist for protecting both health and economic wellbeing.
Further, employment helps people achieve economic stability (Healthy People, 2030). Besides health impacts, many disadvantaged communities are disproportionally impacted by the side effects of COVID-19 like forced unemployment, loss of income and social isolation. Thus, unemployment is identified as a vulnerability indicator of economic stability.
General risk factors for coronavirus are minority status including race and ethnicity due to persisting disparities in COVID-19 cases for Hispanic people and deaths for Black people (CDC, 2022b) even after accounting for age differences across racial and ethnic groups (Hill and Artiga, 2022), though it decreased over time. Minorities continue to carry a disproportionate share of cases and deaths relative to their share of the population (Centers for Disease Control and Prevention, 2020b) of the health burden and economic pain. Due to long-standing systemic health and social inequities, racial and ethnic minorities have a greater risk of getting COVID-19 or a more severe disease regardless of age. Additionally, some communities with greater shares of racial and ethnic minorities have higher exposure to pollution and other environmental hazards. African Americans and other minority populations tend to live in counties with elevated levels of PM 2.5 compared with other racial groups (Wu et al., 2020). Compared with non-Hispanic white persons, racial and ethnic minorities experience higher rates of hospitalization or death from COVID-19: Black and Hispanic Americans are hospitalized at five times and four times the rate of white one, respectively (Golestaneh et al., 2020). Further, due to institutional racism in the form of residential housing segregation, racial and ethnic minorities tend to live in densely populated areas. Minorities and lower-income groups often live in multiunit structures and in multigenerational households which inhibit the ability to practice social distancing which may impact disease occurrence and deaths (Qualls et al., 2017) and have fewer options for protecting both their health and economic wellbeing (Gould and Wilson, 2020). This form of segregation is related to health and medical conditions increasing the risk of getting severely ill or dying from COVID-19. Additionally, studies are concerned with an increased risk of both contracting and transmitting the disease among undocumented migrants who may not report COVID-19 symptoms or get tested due to potential retaliation and deportation (Daniels, 2020; Page et al., 2020; Resnick et al., 2020). Latino people compared with members of other racial/ethnic minority groups have worse risks and outcomes in California who are 8.1 times more likely to live in high-exposure-risk households than Whites people, and have as much higher burden of infection measured by cumulative cases per 100,000 people (Reitsma et al., 2021). Race is associated with other aspects of inequality including lower levels of housing assistance in places where assistance was otherwise high (Drakes et al., 2021).
Our study area is Tennessee which is part of the US southeastern region where counties with high morbidity and mortality rates similarly have high social vulnerability (Drakes et al., 2021). Determining the unit of analysis used in a case study and to which coding procedures are applied, or “bounding of the case,” represents an important action in the establishment of a case study protocol (Yin, 2013). The boundaries of a case can be delineated across space, time, or other representation (Yin, 2013). Next, we therefore selected the scale of analysis the smallest geographic unit for which health data were publicly available: county. This is a potential study limitation as the characteristics of households affected by COVID-19 may differ from the population characteristics in the associated county. However, spatial data are often neither available in the necessary spatial extent, spatial detail, the needed accuracy and consistency, nor the desired up-to-datedness. Thus, we used counties in Tennessee as a case study.
The virus that causes COVID-19 is constantly mutating leading to emergence and predominance of new variants (CDC, 2021). Different variants may impact transmission and disease spread, severity (e.g., increased hospitalizations or deaths), treatment, and natural and vaccine-induced immunity (Paul et al., 2021). The number of new cases and deaths varied substantially across phases and waves during the COVID-19 pandemic. Understanding the difference between the various phases is needed to develop better public health intervention efforts and preventive measures including lockdown measures and others to reduce the spread of the virus. This study is an effort to characterize the public health impact of variants across various communities with a focus on socially disadvantaged areas, its understanding can help guide interventions to mitigate the COVID-19 pandemic in the United States by informing prevention strategies (e.g., enhanced vaccination coverage efforts) and management decisions (Paul et al., 2021).
The following sections briefly describe two representations periods (January 1–31, 2021 and January 1–31, 2022) and state differences in COVID-related health outcomes observed during the earlier and later periods and note that the key difference between these two sampling periods with the surge in cases during the latter period may impact the findings, thus, requiring some caution in interpreting the results. Then we describe our use of measures to represent COVID-19-related health outcomes, a creation of a social vulnerability index, and spatial and statistical models to discern the relationship between social vulnerability and health outcomes.
Characteristics of two representations periods (January 1–31, 2021 and January 1–31, 2022): Contemporaneous studies examine empirical data to compare various health outcomes across COVID-19 phases with big variation in contagiousness, transmissibility and lethality caused by various strains (Iftimie et al., 2021; Kunno et al., 2021; Seong et al., 2021). We build upon these existing studies in that public health data analyzed here covers two representations periods (January 1–31, 2021 and January 1–31, 2022), the latter is Omicron predominance, however, both are high–COVID-19 transmission periods (Iuliano et al., 2022). We briefly provide differences in health outcomes due to COVID observed during the earlier and later periods below.
During 2020–2021, there were reductions in death rates disparities by race and ethnicity, but large disparities in mortality remained in 2021 (Truman et al., 2022). According to the CDC's provisional report, in 2021, COVID-19 was the third major cause of death following heart disease and cancer, with 60,000 more people dying of COVID-19 in the US compared with 2020, while old age (≥85 years) and males were among the demographic factors contributing to highest COVID-19 death rates (Ahmad et al., 2022).
During the second period analyzed in this study, Omicron, classified as a Variant of Concern in the US in November 2021, spread more easily (CDC, March 29, 2022) causing a surge in daily case numbers in the U.S. of over a million (Katella, 2022). Nationwide, during the Omicron-related period, emergency department visits grew by 137% compared with the similar period during the winter 2020–21, and admissions grew by 31%, both driven by high case counts and not by increased disease severity (Iuliano et al., 2022). Compared with winter 2020–21, during winter 2021–2022 various indicators of disease severity decreased including a lower highest daily 7-day moving average of deaths, decreased average length of hospital stay [5.5 days during Omicron vs. 8.0 days during winter 2020–2021), less ICU admittance] (Iuliano et al., 2022), decrease of severe cases (Kim et al., 2022; Maslo et al., 2022; Wolter et al., 2022), and lower mortality (Houhamdi et al., 2022). Due to differences in characteristics across the two periods covered in this study including greater virus contagiousness during the Omicron variant, the results on the role of social disadvantage during various pandemic stages may be impacted, more so regarding the COVID case-related requiring some caution in interpreting the results.
In the study of the impacts of social disadvantage on public health, after we identified major drivers of the COVID-19 infection, and factors of social disadvantage, we develop an index consisting of multiple risk factors to identify vulnerable communities. As explained in the Background section, we also overcome the issues identified inherent to the previously constructed vulnerability indices (such as the CDC-based SVI) and address the issues by using less variables and focusing on only those factors related to the severity of COVID-19. We develop the methodology that identifies the vulnerable communities that most likely need support before, during, and after major health emergencies such as pandemics that might inform healthcare planners and practitioners facing an unfamiliar situation of providing extremely rationed life-saving critical care to residents of the areas that may get extremely sick or die without, using more recent Census data.
Based on the thorough literature review, we have identified the following categories composing a multi-dimensional social disadvantage (also referred to as vulnerability): (1) minority population, (2) hispanic ethnicity (race and ethnicity are vulnerability metrics used in studies on various aspects of health disparities including the COVID-19-related outcomes and environmental justice), (3) poverty (a measure of economic stability). (4) education: the current analysis used lower educational attainment and income levels as additional risk criteria based on recent research findings which included both factors as increased risk conditions (Ajufo et al., 2021). Accordingly, high rates of limited education (% of population with less than high school diploma, an indicator of poverty) have been included, (5) adult obesity based on current CDC criteria which includes it as an increased risk condition for severe COVID [% adult population estimated to be obese defined based on body mass index of 30 and over. Supported by several lines of evidence, both domestically and internationally, obesity may predispose to more severe COVID-19 outcomes (O'Hearn et al., 2021). Thus, obesity is used as epidemiological vulnerability to COVID-19 that may put a person with obesity at higher risk and lead to worse outcomes], and (6) unemployment (a vulnerability indicator of economic stability and overall wellbeing due to its link with all-cause mortality).
Thus, the factors we decided to use to construct the Social Disadvantage Index are the following six variables grouped into four categories: (1) general risk factors of overall vulnerability commonly applied by social justice and health disparity studies including the COVID-19 (race and ethnicity measured by % African American and % Hispanic), (2) epidemiological vulnerability (obesity measured as % estimated obese adults reporting to be obese with the BMI 30 or greater), (3) low socioeconomic status (lower educational attainment measured as % less than high school graduates), (4) economic stability (poverty level measured as % below 100% federal poverty level, and unemployment measured as % unemployment) (see the Background section). We did not include ecological characteristics related to virus transmission such as population density and multi-generation households due to their low variability at the county level, though as noted earlier at certain spatial scales both may serve useful indicator for a greater vulnerability for COVID transmission.
Once a decision was made on the risk factors for severe COVID-19, data was collected and used to compute a composite variable as summed-up z-scores of the above variables to represent social disadvantage (Antipova and Momeni, 2021).
Prior to summing up z-scores, original variables were standardized. Standardization of the original variables was dictated by different variances of the original variables to avoid “unduly” influence of an original variable with a large variance upon the association between the composite variable and outside variables and ensure equal contributions of the original variables (Song et al., 2013). The measurements were converted into standardized z-scores after ensuring that they follow a normal distribution (Gerstein et al., 2021). One variable, % African American, was transformed to a normal distribution by logarithmic transformation. While there are no official rules about cut-off values for skewness (that measures asymmetry) to indicate non-normality, the data is considered normal if skewness is between −2 to +2 (Hair et al., 2010); here, the distributions of the original variables are considered normal (measured with skewness as follows: Ln_% African American: −0.1, % Hispanic: 1.6, % BelowPoverty 100%: −0.16, % Less Than High School Graduates: 0.04, % Estimated Obese Adults: 1.29, % Unemployment: 0.25), and the distribution of the resulting composite variable capturing social disadvantage is deemed normal with the skewness = 0.59.
The higher the value of the social disadvantage index (SDI), the greater the disadvantage. SDI was used to identify vulnerable communities that are at greater risk for more severe disease outcomes (refer to Figure 1). We mapped the SDI with the values ranging from −8.27 to 10.01 across the surface of the study area (Figure 3). Figure 3 presents the distribution of socially disadvantaged communities in Tennessee at the county level. For this, we used interpolation to produce the surface presented in Figure 3. Specifically, it was derived from an Inverse Distance Weighted technique (Watson and Philip, 1985). The concentration of disadvantage can be found in the southwestern corner of the state around Memphis and also in the middle and northeastern part in the counties which are mostly rural. Based on recent studies (Unger et al., 2021), we split the computed SDI values into quintiles and applied a percentile-based approach to group counties by social disadvantage with those counties with the SDI index in the lowest percentile (the lowest 25%) (the SDI of <-2.09; N = 24) as “Low Disadvantage”. Counties in the following quantile with the SDI index of −2.09 < x < −0.25 (N = 28) as “Low Medium Disadvantage”. Counties in the third quantile with the SDI index of −0.25 < x < 1.46 (N = 20) have been labeled as “Medium Disadvantage”. Finally, counties in the highest quantile with the SDI index of >1.46 (N = 23) as “High Disadvantage”. We map these communities in Figure 3 which presents communities by their social disadvantage status. For mapping, we used the Tennessee State Plane Coordinate System of 1983 which is the Lambert Conformal Conic Projection recognized and utilized in Tennessee (Stem, 1995). The projection is best suited for the US states that are long in east-west direction such as Tennessee. The map was created using ArcMap 10.7 version software.
Comparison with the CDC-based SVI index: in order to understand how the findings would differ if the same analyses were to be carried out using other vulnerability indices, such as the existing CDC's-based Social Vulnerability Index (SVI) which ranks percentile of social vulnerability from 15 social factors grouped into four related themes (an overall ranking is represented with RPL_THEMES), we identified the disadvantaged areas according to the CDC's SVI index. The SVI 2018 was downloaded in shapefile format from https://www.atsdr.cdc.gov/placeandhealth/svi/data_documentation_download.html. For consistency, we used the same scale (county) and the highest 25% (RPL_THEMES >0.751) to identify counties in the highest percentile as “High Disadvantage” (Ncdc = 24). We mapped those areas (presented in Figure 4 in red color) where location of disadvantaged areas using both methods (proposed here— the SDI and the CDC-based SVI) can be compared. The visual examination reveals substantial spatial overlap between the two indices as many counties identified as “Disadvantaged” using the SDI method proposed in this paper (Figure 4A) are also disadvantaged using the methodology developed by the CDC (Figure 4B), although differences exist as not all highly socially disadvantaged counties located with the SDI method have been captured when using the SVI index available from the CDC. We conducted Pearson correlation between the SDI index proposed here and the CDC-based SVI index finding strong positive statistical association between both indices (Pearson correlation coefficient = 0.75, p-value < 0.000; N = 95). Next, we ran statistical analyses for areas identified using both indices and show the results in the Results section.
Figure 4. Disadvantaged areas in Tennessee shown in red color using (a) the SDI and (b) the CDC-based SVI methods.
It is important to identify “high-priority areas” where resources including testing kits and facilities need to be provided in a timely fashion to mitigate community spread. Then, we map geographical inequalities in coronavirus disease morbidity (using case rates), disease severity (using case-fatality ratio) and mortality (using death rates) in Tennessee. We also assess the association between preexisting social vulnerability and health outcomes across the study area and test the hypothesis of the link between social disadvantage and the burden from COVID-19-related morbidity and mortality (the primary health outcomes) in Tennessee. For this, we examined spatial association between health outcomes and social vulnerability (Figures 3, 4). Next, we plotted various health outcomes against the SDI values both for the earlier period (here, 2021) and later pandemic stage (2022) (presented in Figures 5–8). Finally, we computed odds ratios of various health outcomes for disadvantaged communities for the later pandemic stage (2022) compared with the earlier period in 2021 (Tables 2, 3).
Figure 6. Social disadvantage and case rate 7-day average for (A) January 1, 2021; (B) January 1, 2022.
Figure 8. Social disadvantage and case-fatality ratios for (A) January 1, 2021; (B) January 1, 2022.
Broadly, this research examines the COVID-19-related health outcomes including morbidity and mortality. We used county-level morbidity and mortality data by day for each county in Tennessee from the local public health department. Due to potential variations in inequality in exposure and pandemic susceptibility during different COVID-19 stages requiring analysis at different time points for counties (Karaye and Horney, 2020), we analyzed data for two representative periods in the pandemic. To understand how social disadvantage impacted disease outcomes through the pandemic (if any), we used January 1–31, 2021, as an earlier period when vaccination was hardly available, and to be consistent, January 1–31, 2022, as a later post- vaccination stage. We extracted total cases and deaths data, as published for Tennessee (at: https://www.tn.gov/health/cedep/ncov/data/downloadable-datasets.html).
Specifically, we measure morbidity by the sickness [measured by the total confirmed cases per 100,000 people (case rate), and the total confirmed cases per 100,000 people 7-day average (case rate in the last 7 days). We also use the metric of disease severity (measured by Case Fatality Rate, CFR, or the ratio of the total confirmed cases to deaths). CFR represents the proportion of people who die from a specified disease (here, COVID-19) among all individuals with COVD-19. Finally, we analyze mortality (measured by the total deaths, and the total deaths per 100,000 people (death rate). We compare these health outcomes between communities with “vulnerable demographics” and more privileged neighborhoods based on the values of the SDI. The figures and tables provide info as of January 1, 2021, and January 1, 2022. Other figures and tables plot all daily data for January 1–31, 2021, and January 1–31, 2022 (available in Appendices and on request).
In detail, using reported metrics of total case and deaths counts (tca, tde), the author calculated (1) the total average number of COVID-19 cases in last 7 days (tca7av), (2) COVID-19 case rate per 100,000 people (the total number of COVID-19 cases per 100,000 people (tcaK), (3) the average number of COVID-19 cases per 100,000 people in last 7 days (tca7avK), (4) new daily cases (ndca), (5) new daily cases per 100,000 people (ndcaK), (6) the total number of COVID-19 deaths (tde), (7) COVID-19 death rate per 100,000 people (the total number of COVID-19 deaths per 100,000 people tdeK), (8) the average number of COVID-19 deaths per 100,000 people in last 7 days (tde7avK), (9) case-fatality ratio (CFR) (listed in Table 1).
This section describes the geographic distribution of social vulnerability and health outcomes across the study area in Tennessee. We identified areas with a greater burden of the disease and which drive a spread of the infection due to higher rates of morbidity, contributed by a concentration of the known risk factors (a high share of obesity, minority, Hispanic ethnicity, poverty, unemployment, low education), to be considered “high-priority areas” for timely resource provision (such as testing kits and facilities) as well as targeted for a promotion of health policies (such as vaccination) and other strategies to mitigate community spread and reduce disease severity in disadvantaged communities.
Both Figures 3, 4 suggest spatial association between health outcomes and social vulnerability where counties which are both disadvantaged and rural seem to have more cases per 100,000 people, higher severity and mortality, especially communities around Nashville, Knoxville, and Chattanooga. Conceptually, places suffering higher adverse health outcomes will need more assistance such as increased access to vaccination and other resources and health-promoting strategies to cope with this disproportionate impact (Drakes et al., 2021).
Figures 5–8 illustrate the statistical association of health outcomes (morbidity and mortality) and social vulnerability. Visual examination of the maps in Figures 5–8 indicates positive spatial correlation, where counties with extreme levels of the negative health outcomes tend to have high levels of social vulnerability. Figure 5 shows the distribution of case rates by social disadvantage [shown for (a) January 1, 2021, and (b) January 1, 2022. All monthly case rates for January 1–31, 2021, and January 1–31, 2022, are provided in the Figure A1]. Figure 6 presents social disadvantage and the case rate 7-day average for (a) January 1, 2021; (b) January 1, 2022 (similarly, monthly case rate 7-day averages for January 1–31, 2021 and January 1–31, 2022 are provided in the Figure A2). Figures 7, 8 present the distribution of death rates and case-fatality ratios by social disadvantage for (a) January 1, 2021; (b) January 1, 2022 (similarly, monthly death rates and case-fatality ratios for January 1–31, 2021 and January 1–31, 2022 are provided in Figures A3, A4, respectively).
We tested the null hypothesis that there is no difference in the health outcomes against an alternative hypothesis that the health outcomes are worse in the areas with high social disadvantage using the non-parametric Wilcoxon rank-sum test. To test for differences in location, we performed the PROC NPAR1WAY procedure using SAS software. The results of this two-sample (as there are two classification levels) one-sided test can be found in Table 2. The results obtained based on using the vulnerability index proposed in this study (SDI) and those with the CDC-based SVI index, are largely similar and support each other, with some differences.
Table 2. The results of Wilcoxon Two-Sample Test (1-sided) using January 1, 2022 (top of the table) and January 1, 2021 (bottom) data.
In detail, after we used the SDI index to identify highly vulnerable areas, and then carried out statistical analyses regarding disease morbidity, we show in 2021 (the results are in the lower part of the table), there is no difference in measures of crude morbidity (measured with total cases and total cases 7-day average) between areas with high and low disadvantage, while in 2022 (in the upper part of the table), the total number of cases and total cases 7-day average are higher in areas with low disadvantage. However, accounting for population size (shown in total case rates per 100,000 population), the direction changes with the disadvantaged areas having statistically significantly greater disease burden in both 2021 (p-value <0.001) and 2022 (p-value <0.01). Using other measures of disease morbidity, new daily cases and new daily case rates are not different between areas with different exposure to disadvantage in either 2021 or 2022. Regarding disease severity, measured as mortality (total deaths and total deaths rate), there are more deaths in areas with low social disadvantage (though statistically insignificantly in 2021, while statistically significantly higher in 2022). Again, taking into account population size and considering rates, disadvantaged areas are burdened with higher total death rates in both 2021 and 2022 at 0.05 significance level. Case fatality rate (CFR) is greater in high-disadvantaged areas but not significantly different from low-disadvantaged areas during both periods.
Using the CDC-based SVI index to identify high-disadvantaged areas, the results are somewhat similar to those above obtained for vulnerable areas identified using the SDI-based method of identification. Similarities include total case rate (also 7-day average) which are higher in disadvantaged areas in both periods and total death rates are higher in high-disadvantaged areas in 2021 (but not 2022). Other similarities to the results above include new daily case rates that are not different between areas with different exposure to disadvantage in either 2021 or 2022. The differences to the results above include new daily cases that appear statistically significantly higher in low-disadvantaged areas. Other measures of crude morbidity that are different from those above include total cases and total cases 7-day average (both insignificant when using the SDI-based method of identification of vulnerable areas in 2021) which seem statistically significantly higher in low-disadvantaged areas. Total deaths (an indicator of disease severity) are statistically significantly higher in low-disadvantaged areas in both 2021 and 2022 (while not statistically significant when carrying out analyses on areas found with the SDI-based method), while total death rates which account for population size are higher in high-disadvantaged areas (p-values are insignificant in 2022).
Overall, it appears that despite results similarity and apparent strong positive statistical association between both indices, we show greater inconsistencies when analyzing areas identified using the CDC-based index, such as finding more often greater disease burden in low-disadvantaged areas including total cases, total case 7-day average, new daily cases, and total deaths in 2021, the year when vaccination was still largely unavailable, and when many residents of more privileged communities could afford work from home while their counterparts from high-disadvantaged areas were more likely to hold essential jobs and thus had a greater exposure to the virus due to inability to practice social distance at work and home due to crowded conditions. The SDI-index that is based on a combination of several known COVID-19 risk drivers, seems to perform better in analyzing pandemic-related results.
While there is association between social disadvantage and COVID-19-related health outcomes, the role of social disadvantage appears to be weaker in 2022 compared with 2021 (using the magnitude of the R2 values which are consistently lower for 2022 data of the disease morbidity, severity, and mortality), with other unexplained factors driving the negative outcomes such as premature relaxation of social distancing and mask requirements with the disease continuing to cause an outsized impact on the population. Compared with pre-pandemic, as of February 16, 2022, present all-cause mortality in the United States is much higher. Other recent research supports this statement and observes that majority of variation in cumulative infection rates could not be explained (Bollyky et al., 2022). However, an earlier study noted that nationwide COVID-19 case rates could be explained by minority status, language, household composition and transportation, and housing and disability (Karaye and Horney, 2020). Additionally, we assessed how disparities have evolved over the course of the COVID-19. For this, we computed odds ratios of COVID-19 case count 7-day average, case rate, and case rate 7-day average for disadvantaged communities for the later pandemic stage (2022) compared with the earlier period in 2021 (in Table 3). For COVID-19 cases (measured as case count 7-day average and case rate and case rate 7-day average (Table 3), odds ratios (OR) show that since the OR is >1, counties with high disadvantage had higher odds of having higher infection load during later stage of the COVID-19 compared with those disadvantaged counties during the earlier stage of COVID-19. This was in agreement with recent research that shows that overall, compared to the Delta, the Omicron variant is generally 2.7–3.7 times more infectious among vaccinated individuals, and unvaccinated individuals have been reported to have an increased transmission of the variant fully compared to vaccinated individuals (Lyngse et al., 2021).
Table 3. Odds ratio of case count (7-day average) and case rate (7-day average) for counties with high disadvantage: Results comparison for areas identified with SDI and SVI indices.
The odds ratios (ORs) have been calculated in this cross-sectional study (Setia, 2016). We use a 2 × 2 table (Table 3) for odds ratios calculation to understand the association between exposure to social disadvantage and health outcomes of COVID-19. Thus, from Table 3, for case count and case rate (both 7-day average), we interpret these ORs computed with both using the SDI and the CDC-based SVI index, that since the OR is >1 for both total case count and case rate (both 7-day average), counties with high disadvantage had higher odds of having higher infection load during later stage of the COVID-19 compared with disadvantaged counties during earlier stage of COVID-19.
Given the high disease burdens in the United States, and consistent evidence for strong associations between the major risk factors and COVID-19 severity, with severe outcomes preventable if these conditions are absent (O'Hearn et al., 2021), the extent to which multi-dimensional disadvantage may be contributing to COVID-19 morbidity and mortality and associated health disparities, needs to be quantified to better protect at-risk communities. We develop a systematic and a straightforward approach to identify communities at a higher risk for a more severe COVID-19 impact and apply it to a case study in Tennessee. Overall, high social vulnerability is clustered in the southeastern United States which also tend to be rural (Drakes et al., 2021). Places that are both rural and socially vulnerable are therefore more likely to require federal assistance. Rurality is a dominant feature of the high social vulnerability clusters. Accordingly, we studied the impact of social disadvantage on COVID-19-related health outcomes in communities in a region that is characterized by a concentration of high vulnerability and rural areas (Drakes et al., 2021).
Socioeconomic impacts remain relatively underexplored in studies on the impacts of COVID-19 on cities (Sharifi and Khavarian-Garmsir, 2020). Communities may have very contrasting experiences regarding health outcomes due to pandemics. Generally, the historical evidence provides many examples when social and economic inequalities interact and impact the course of epidemics. Past research on the urban impacts of various disasters considered inequalities the main issue rendering people in poverty more susceptible to pandemics (Wade, 2020). Those already marginalized have often the greatest risks documented earlier in history such as during the Black Death in the medieval times when wealthy and poor had very different mortality rates, while the Native Americans had a much greater mortality burden during the colonial times not entirely attributed to a lack of acquired immunity (Wade, 2020). At the beginning of the COVID-19 epidemic, in New York City, Latino and African Americans suffered twice as a great mortality from COVID-19 as Whites, with clusters of cases observed in poorer zip codes due to more people who live in crowded conditions and inability to do work from home or relocate to vacation homes. Practicing social distancing is a challenge when more dwellers live per apartment accelerating disease transmission in the community. The unequal socio-economic conditions render people of some communities more susceptible to epidemics, damage people's health, and make the effects more devastating. When people are already experiencing extreme poor health, poverty, people have more deadly effects when diseases sweep through, however, the conditions making people more vulnerable to the disease outcomes can be changed.
We assessed the association between preexisting social vulnerability and health outcomes across the study area. For this, we first reviewed factors identified by the recent studies associated with a greater severity from COVID-19. Accordingly, we proceeded by identifying key factors associated with general vulnerability for diseases and social justice, COVID-19 epidemiological vulnerability, and overall wellbeing vulnerability. People seem to be at higher risk of severe illness from COVID-19 if they have serious underlying chronic medical conditions like chronic lung disease, a serious heart condition, or a weakened immune system. Some underlying conditions, including hypertension, cardiovascular disease, chronic obstructive pulmonary disease, and cancer, are already known to significantly decrease the chance of recovery from the severest form of COVID-19 (Wu and McGougan, 2020). People with disabilities might be at higher risk of COVID if underlying medical conditions are involved (Centers for Disease Control and Prevention, 2020a).
These key attributes have been used to construct the Social Disadvantage Index (SDI) and locate counties in Tennessee with a greater burden of the disease due to a concentration of the contributing factors. These include obesity, minorities/Hispanic ethnicity, poverty, low education, and unemployment. We did not include other potential risk factors contributing to disease severity from COVID-19 including exposure to air pollution, crowded household conditions, and population density due to either data unavailability or their weak variation across the spatial units used in this study. The areas with high shares of the above-listed risk factors have been designated as “high social disadvantage”, while areas with low shares have been designated as “low social disadvantage”. We tested the relationship between socio-economic deprivation and the burden from COVID-19-related morbidity and mortality (the primary health outcomes) in Tennessee.
The pattern of reported COVID-19-related infection and mortality is different from that of many other communicable diseases. To illustrate, the recent study set out to estimate excess mortality from COVID-19 noting that wealthier countries have lower share of the world population (48%) yet have higher national cumulative mortality rates (53% of the total estimated excess mortality-adjusted COVID-19-related deaths as of Sept 30, 2021), despite higher COVID-19 vaccination coverage since December 2020, compared with those in lower-income countries (COVID-19 excess mortality collaborators, 2022). Recent studies also note that having trust in the government and interpersonal trust, less government corruption, have large associations that are statistically significant with lower standardized infection rates (Bollyky et al., 2022). Overall, research reveals the impact of the scale effect: at the global level, there is association between Social Vulnerability Index, minority status and language, and greater COVID-19 case rates, however, finer-scale modeling at the county level reveals varied association between social vulnerability and COVID-19 health outcomes (Karaye and Horney, 2020).
Despite availability of multiple vulnerability indices, some variables used in creating the existing indices may be less relevant to the COVID. Our research questions are motivated by a desire to develop an improved social disadvantage index (SDI) that might be used efficiently for identification of vulnerable communities that are at a higher risk for disease severity during the COVID-19 pandemic and might need external assistance. The social disadvantage index (SDI) created for the purpose of the study uses the concept of a multi-dimensional social disadvantage. It is based on a combination of several COVID-19 risk factors identified by the current literature. We applied the SDI in the study on the relationship between disadvantage and the COVID-19-related outcomes.
This study has three objectives. First, it develops a methodology to create a social disadvantage index (SDI) consisting of variables contributing to greater COVID-19 severity. For this objective, it uses a simple and yet robust measures of COVID-19- and other aspects of vulnerability. Second, it addresses disparities associated with the social determinants of health. For this, we apply a multi-dimensional index to examine the relationship between social disadvantage and health focusing on disadvantaged communities. The approach we rely on uses quantitative spatial parameters. The developed index is especially suitable during the COVID-19 disaster to identify vulnerable communities. The method follows a few simple and readily implemented guidelines yet generating robust and precise knowledge based on a case study in Tennessee. We apply this method to study COVID-19 health outcomes in disadvantaged communities. Third, we identify high-disadvantaged communities in the study area using another vulnerability index such as the existing social vulnerability index (SVI) developed by the CDC and compare the findings (here, the COVID-19-related health outcomes) after we carry out analyses to these areas. We find strong statistical association between the two indices. However, despite a substantial spatial overlap between the two indices including the SDI and SVI, with most disadvantaged communities captured with both indices, differences remain as some counties identified with the SDI method as highly socially disadvantaged do not have the same status when using the SVI index available from the CDC. The difference may be due to using some variables that are not risk factors for a greater disease severity due to COVID-19 in the construction of vulnerability indices that have been developed in the previous decade including the SVI index developed by the CDC.
Among individuals who are fully vaccinated against SARS-CoV-2 infection, transmission of the Omicron variant has been high, and unvaccinated individuals have been reported to have an increased transmission of the variant compared to fully vaccinated individuals (Lyngse et al., 2021). Globally, the most recent decreasing trend reflected in both the number of new COVID-19 cases and the number of new deaths during February 14–20, 2022, as compared to the previous week, may have been observed as some countries decreased their testing and sequencing policies during the presented period (WHO, 2022). Further, recently, older people had experienced an increase in the number of cases potentially leading to a delayed increase of severe cases and deaths (European Centre for Disease Prevention and Control, 2022).
The study is subject to the following limitations. Health studies assessing a patient's risk for certain health outcomes note that simplifying social determinants of health into a single index may be not a reliable surrogate (Chakravarthy et al., 2021). Next, the scale of analysis is at the county level since it is the smallest geographic unit for which health data were publicly available. As noted earlier, the characteristics of households affected by COVID-19 may differ from the population characteristics in the associated county as well as population size. Further, we did not account for healthcare access which was less available during the early pandemic stages, representing an area of potential future research. Omitting access to health care may lead to overestimated results of mortality risk for some health outcomes such as cancer survival (Hastert et al., 2015; Singh and Jemal, 2017; Unger et al., 2021). Further, vaccinations have still been largely unavailable in January 2021, while many people had been vaccinated in January 2022, potentially impacting the comparability of our estimates for the two periods. However, disadvantaged communities have a lower vaccination coverage, thus, potentially subject to severe infections among these populations and high-risk groups (European Centre for Disease Prevention and Control, 2022). A greater share of Hispanic, non-Hispanic Black had not been vaccinated or had not received a third COVID-19 vaccine dose (Thompson et al., 2022). As stated earlier, because characteristics across different pandemic periods differ including greater virus contagiousness during the latest Omicron variant, the results on the role of social disadvantage may impact COVID case-related findings requiring some caution in interpreting the results. Further, research findings indicate that the rapid spread of the Omicron variant is largely attributed to the immune evasiveness (that is, a higher share of vaccinated or priorly infected individuals being infected) and not greater basic transmissibility (Lyngse et al., 2021) reducing vaccination effectiveness over the short and medium term (Atlani-Duault et al., 2021), thus, our results should be robust to this potential limitation.
Further research may address the limitations of the current study. Future work could mitigate the issue of cross-county wide variability in population size by (1) constructing analysis unit of comparable size as in Wang and Robert (2015), or (2) using a weighted OLS regression model (using population size as weight). Additionally, future work should consider the variability of health outcomes by urbanicity of a county e.g., as classified by the National Center for Health Statistics (Ingram and Franco, 2012) or by urban ratio derived by smaller area units as in Xu and Wang (2015). Similarly, regarding healthcare access, future research should examine whether the health disparity is related to reduced access to health care during the COVID-19. Next, our research might be logically extended by comparing other social vulnerability indices (SVIs). Future studies may also analyze how application of different SVI indices across various-sized areal units may affect the resulting differences in health outcomes.
The raw data supporting the conclusions of this article will be made available by the authors, without undue reservation.
AA designed the study, obtained, analyzed, visualized data, researched the topic, and wrote the manuscript.
UTHSC/University of Memphis SARS-CoV-2/COVID-19 Research CORNET (Collaboration Research Network) grant.
The author declares that the research was conducted in the absence of any commercial or financial relationships that could be construed as a potential conflict of interest.
All claims expressed in this article are solely those of the authors and do not necessarily represent those of their affiliated organizations, or those of the publisher, the editors and the reviewers. Any product that may be evaluated in this article, or claim that may be made by its manufacturer, is not guaranteed or endorsed by the publisher.
The Supplementary Material for this article can be found online at: https://www.frontiersin.org/articles/10.3389/frsc.2022.876945/full#supplementary-material
Figure A1. Social disadvantage and case rates for (A) January 1–31, 2021; (B) January 1–31, 2022.
Figure A2. Social disadvantage and case rate 7-day average for (A) January 1–31, 2021; (B) January 1–31, 2022.
Figure A3. Social disadvantage and death rates for (A) January 1–31, 2021; (B) January 1–31, 2022.
Figure A4. Social disadvantage and case-fatality ratios for (A) January 1–31, 2021; (B) January 1–31, 2022.
Ahmad, F. B., Cisewski, J. A., and Anderson, R. N. (2022). Provisional mortality data — United States, 2021. MMWR Morb. Mortal. Wkly. Rep. 71, 597–600. doi: 10.15585/mmwr.mm7117e1
Ajufo, E., Rao, S., Navar, A. M., Pandey, A., Ayers, C. R., and Khera, A. (2021). U.S. population at increased risk of severe illness from COVID-19. Am. J. Prev. Cardiol. 6, 100156. doi: 10.1016/j.ajpc.2021.100156
Antipova, A. (2020). “Analysis of exposure to ambient air pollution: case study of the link between environmental exposure and children's school performance in Memphis, TN,” in Spatiotemporal Analysis of Air Pollution and Its Application in Public Health, eds L. Li, X. Zhou, and W. Tong (Cambridge, MA: Elsevier), 217–275.
Antipova, A., and Momeni, E. (2021). Unemployment in socially disadvantaged communities in Tennessee U.S. during the COVID-19. Front. Sustain. Cities 3, 726489. doi: 10.3389/frsc.2021.726489
Arpey, N. C., Gaglioti, A. H., and Rosenbaum, M. E. (2017). How socioeconomic status affects patient perceptions of health care: a qualitative study. J. Prim. Care Commun. Health 8, 169–175. doi: 10.1177/2150131917697439
Atlani-Duault, L., Lina, B., Chauvin, F., Delfraissy, J. F., and Malvy, D. (2021). Immune evasion means we need a new COVID-19 social contract. Lancet 6, e199–e200. doi: 10.1016/S2468-2667(21)00036-0
Bakkensen, L. A., Fox-Lent, C., Read, L. K., and Linkov, I. (2017). Validating resilience and vulnerability indices in the context of natural disasters. Risk Anal. 37, 982–1004. doi: 10.1111/risa.12677
Bayer, C., and Kuhn, M. (2020). Intergenerational Ties and Case Fatality Rates: A Cross-Country Analysis. IZA Discussion Papers 13114, Institute of Labor Economics (IZA).
Birkmann, J. (2013). Data, Indicators and Criteria for Measuring Vulnerability: Theoretical Bases and Requirements. Measuring Vulnerability to Natural Hazards Towards Disaster Resilient Societies. New York, NY: United Nations University Press, 80–106.
Blackwell, D. L., Lucas, J. W., and Clarke, T. C. (2014). Summary health statistics for U.S. Adults: National Health Interview Survey. Vital Health Stat. 10, 1–161.
Bollyky, T. J., Hulland, E. N., Barber, R. M., Collins, J. K., Kiernan, S., Moses, M., et al. (2022). Pandemic preparedness and COVID-19: an exploratory analysis of infection and fatality rates, and contextual factors associated with preparedness in 177 countries, from Jan 1, 2020, to Sept 30, 2021. Lancet. 399, 1489–1512. doi: 10.1016/S0140-6736(22)00172-6
Cariou, B., Hadjadj, S., Wargny, M., Pichelin, M., Al-Salameh, A., Allix, I., et al. (2020). Phenotypic characteristics and prognosis of inpatients with COVID-19 and diabetes: the CORONADO study. Diabetologia 63, 1500–1515. doi: 10.1007/s00125-020-05180-x
CDC (2018). CDC Social Vulnerability Index 2018 Database. Centers for Disease Control and Prevention/Agency for Toxic Substances and Disease Registry/Geospatial Research, Analysis, and Services Program. Available online at: https://www.atsdr.cdc.gov/placeandhealth/svi/data_documentation_download.html (accessed September 20, 2021).
CDC (2021). SARS-CoV-2 Variant Classifications and Definitions. Atlanta, GA: US Department of Health and Human Services, Centers for Disease Control and Prevention. Available online at: https://www.cdc.gov/coronavirus/2019-ncov/cases-updates/variant-surveillance/variant-info.html (accessed April 26, 2022).
CDC (2022a). Science Brief: Evidence Used to Update the List of Underlying Medical Conditions Associated with Higher Risk for Severe COVID-19. Centers for Disease Control and Prevention. Available online at: https://www.cdc.gov/coronavirus/2019-ncov/hcp/clinical-care/underlyingconditions.html (accessed June 15, 2022).
CDC (2022b). Demographic Trends of COVID-19 Cases and Deaths in the US Reported to CDC. Centers for Disease Control and Prevention. Available online at: https://covid.cdc.gov/covid-data-tracker/#demographics (accessed September 04, 2022).
Centers for Disease Control and Prevention (2020a). Others at Risk: People with Disabilities. Available online at: https://www.cdc.gov/coronavirus/2019-ncov/need-extra-precautions/people-with-disabilities.html (accessed April 9, 2020).
Centers for Disease Control and Prevention (CDC) (2020b). Health Equity Considerations and Racial and Ethnic Minority Groups. Available online at: https://www.cdc.gov/coronavirus/2019-ncov/community/health-equity/race-ethnicity.html?CDC_AA_refVal=https%3A%2F%2Fracial-ethnic-minorities.html (accessed July 24, 2020).
Chakravarthy, R., Stallings, S. C., Velez Edwards, D. R., Zhao, S. K., Conway, D., Rao, J. S., et al. (2021). Determinants of stage at diagnosis of HPV-related cancer including area deprivation and clinical factors. J. Public Health. 44, 18–27. doi: 10.1093/pubmed/fdaa246
Chen, E., and Miller, G. E. (2013). Socioeconomic status and health: mediating and moderating factors. Annu. Rev. Clin. Psychol. 9, 723–749. doi: 10.1146/annurev-clinpsy-050212-185634
Chow, N., Fleming-Dutra, K., Gierke, R., Hall, A., Hughes, M., Pilishvili, T., et al. (2020). Preliminary estimates of the prevalence of selected underlying health conditions among patients with coronavirus disease 2019 — United States, February 12–March 28, 2020. MMW Morbid. Mortal. Wkly Rep. 69, 382–386. doi: 10.15585/mmwr.mm6913e2
Clark, G. E., Moser, S., Ratick, S., Dow, K., Meyer, W., Emani, S., et al. (1998). Assessing the vulnerability of coastal communities to extreme storms: the case of revere, MA., USA. Mitigat. Adapt. Strat. Global Change 3, 59–82. doi: 10.1023/A:1009609710795
Committee on the Recommended Social and Behavioral Domains Measures for Electronic Health Records, Board on Population Health and Public Health, Practice, Institute of Medicine. (2015). Capturing Social and Behavioral Domains and Measures in Electronic Health Records: Phase 2. National Academies Press. Available online at: http://www.ncbi.nlm.nih.gov/books/NBK268995/ (accessed February 20, 2022).
COVID-19 excess mortality collaborators. (2022). Estimating excess mortality due to the COVID-19 pandemic: a systematic analysis of COVID-19-related mortality, 2020-21. Lancet. 399, 1513–1536. doi: 10.1016/S0140-6736(21)02796-3
Cutler, D.M., Lleras-Muney, A., and Vogl, T. (2008). Socioeconomic status and health: dimensions and mechanisms. National Bureau of Economic Research Working Paper Series, No. 14333. doi: 10.3386/w14333
Cutter, S., Boruff, B., and Shirley, L. (2003). Social vulnerability to environmental hazards. Soc. Sci. Q. 84, 242–261. doi: 10.1111/1540-6237.8402002
Daniels, J. P. (2020). Venezuelan migrants “struggling to survive” amid COVID-19. Lancet 395, 1023. doi: 10.1016/S0140-6736(20)30718-2
Drakes, O., Tate, E., Rainey, J., and Brody, S. (2021). Social vulnerability and short-term disaster assistance in the United States. Int. J. Disast. Risk Reduct. 53, 102010. doi: 10.1016/j.ijdrr.2020.102010
Esnard, A. M., Sapat, A., and Mitsova, D. (2011). An index of relative displacement risk to hurricanes. Nat. Hazards 59, 833. doi: 10.1007/s11069-011-9799-3
European Centre for Disease Prevention and Control (2022). Assessment of the Further Spread and Potential Impact of the SARS-CoV-2 Omicron Variant of Concern in the EU/EEA, 19th Update - 27 January 2022. Stockholm: ECDC. Available online at: https://www.ecdc.europa.eu/sites/default/files/documents/RRA-19-update-27-jan-2022.pdf (accessed February 20, 2022).
Flanagan, B. E., Gregory, E. W., Hallisey, E. J., Heitgerdand, J. L., and Lewis, B. (2011). A Social vulnerability index for disaster management. J. Homeland Sec. Emerg. Manag. 8, 3. doi: 10.2202/1547-7355.1792
Gerstein, H. C., Ramasundarahettige, C., and Bangdiwala, S. I. (2021). Creating composite indices from continuous variables for research: the geometric mean. Diabetes Care 44, e85–e86. doi: 10.2337/dc20-2446
Golestaneh, L., Neugarten, J., Fisher, M., Billett, H. H., Gil, M. R., Johns, T., et al. (2020). The association of race and COVID-19 mortality. Eclin. Med. 25, 100455. doi: 10.1016/j.eclinm.2020.100455
Gould, E., and Wilson, V. (2020). Black Workers Face Two of the Most Lethal Preexisting Conditions for Coronavirus - Racism and Economic inequality. Washington, DC: Economic Policy Institute. Available online at: https://www.epi.org/publication/black-workers-covid/
Hair, J. F., Black, W. C., Babin, B. J., and Anderson, R. E. (2010). Multivariate Data Analysis: A Global Perspective, Upper Saddle River, NJ: Pearson Education.
Hamideh, S., and Rongerude, J. (2018). Social vulnerability and participation in disaster recovery decisions: public housing in Galveston after Hurricane Ike. Nat. Hazards 93, 1629–1648. doi: 10.1007/s11069-018-3371-3
Hastert, T. A., Beresford, S. A., Sheppard, L., and White, E. (2015). Disparities in cancer incidence and mortality by area-level socioeconomic status: a multilevel analysis. J. Epidemiol. Community Health 69, 168–176. doi: 10.1136/jech-2014-204417
Hazards and Vulnerability Research Institute (2016). U.o.S.C. Social Vulnerability Index for the United States – 2010–2014. Available online at: http://artsandsciences.sc.edu/geog/hvri/sovi%C2%AE-0 (accessed February 20, 2022).
Healthy People (2030). U.S. Department of Health and Human Services, Office of Disease Prevention and Health Promotion. Available online at: https://health.gov/healthypeople/objectives-and-data/social-determinants-health (accessed February 20, 2022).
Heymach, J., Krilov, L., Alberg, A., Baxter, N., Chang, S. M., Corcoran, R. B., et al. (2018). Clinical cancer advances 2018: annual report on progress against cancer from the American Society of Clinical Oncology. J. Clin. Oncol. 36, 1020–1044. doi: 10.1200/JCO.2017.77.0446
Hill, L., and Artiga, S. (2022). COVID-19 Cases and Deaths by Race/Ethnicity: Current Data and Changes Over Time. Available online at: https://www.kff.org/coronavirus-covid-19/issue-brief/covid-19-cases-and-deaths-by-race-ethnicity-current-data-and-changes-over-time/# (accessed August 22, 2022).
Horney, J., Simon, M., Grabich, S., and Berke, P. (2015). Measuring participation by socially vulnerable groups in hazard mitigation planning, Bertie County, North Carolina. J. Environ. Plann. Manag. 58, 802–818. doi: 10.1080/09640568.2014.892870
Houhamdi, L. P., Hoang, V. T., Fournier, P.-E., Colson, P., and Raoult, D. (2022). Characteristics of the first 1119 SARS-CoV-2 omicron variant cases, in Marseille, France. J. Med. Virol. 94, 2290–2295. doi: 10.1002/jmv.27613
Iftimie, S., López-Azcona, A. F., Vallverdú, I., Hernández-Flix, S., De Febrer, G., Parra, S., et al. (2021). First and second waves of coronavirus disease-19: a comparative study in hospitalized patients in Reus, Spain. PLoS ONE 16, e0248029. doi: 10.1371/journal.pone.0248029
Ingram, D. D., and Franco, S. J. (2012). NCHS urban-rural classification scheme for counties. National Center for Health Statistics. Vital Health Stat. 2. Available online at: https://www.cdc.gov/nchs/data/series/sr_02/sr02_154.pdf
Ioannou, G. N., Locke, E., Green, P., Berry, K., O'Hare, A. M., Shah, J. A., et al. (2020). Risk factors for hospitalization, mechanical ventilation, or death among 10 131 US veterans with SARS-CoV-2 infection. JAMA Netw Open 3, e2022310. doi: 10.1001/jamanetworkopen.2020.22310
Iuliano, A. D., Brunkard, J. M., Boehmer, T. K., Peterson, E.;,Adjei, S., Binder, A. M., Cobb, S., et al. (2022). Trends in disease severity and health care utilization during the early omicron variant period compared with previous SARS-CoV-2 high transmission periods — United States, December 2020–January 2022. MMWR Morb. Mortal. Wkly. Rep. 71, 146–152. doi: 10.15585/mmwr.mm7104e4
Johnson, D. P., Stanforth, A., Lulla, V., and Luber, G. M. (2012). Developing an applied extreme heat vulnerability index utilizing socioeconomic and environmental data. Appl. Geogr. 35, 23–31. doi: 10.1016/j.apgeog.2012.04.006
Kabarriti, R., Brodin, N. P., Maron, M. I., Guha, C., Kalnicki, S., Garg, M. K., et al. (2020). Association of race and ethnicity with comorbidities and survival among patients with COVID-19 at an urban medical center in New York. JAMA Netw. Open 3, e2019795. doi: 10.1001/jamanetworkopen.2020.19795
Karaye, I. M., and Horney, J. A. (2020). The impact of social vulnerability on COVID-19 in the U.S.: an analysis of spatially varying relationships. Am. J. Prev. Med. 59, 317–325. doi: 10.1016/j.amepre.2020.06.006
Katella, K. (2022). Omicron, Delta, Alpha, and More: What To Know About the Coronavirus Variants. Yale Medicine. Available online at: https://www.yalemedicine.org/news/covid-19-variants-of-concern-omicron (accessed August 31, 2022).
Kim, M.-K., Lee, B., Choi, Y. Y., Um, J., Lee, K. S., Sung, H. K., et al. (2022). Clinical characteristics of 40 patients infected with the SARS-CoV-2 omicron variant in Korea. J. Korean Med. Sci. 37, e31. doi: 10.3346/jkms.2022.37.e31
Kind, A. J. H., and Buckingham, W. R. (2018). Making neighborhood-disadvantage metrics accessible - the neighborhood atlas. N. Engl. J. Med. 378, 2456–2458. doi: 10.1056/NEJMp1802313
Kontis, V., Bennett, J. E., Rashid, T., Parks, R. M., Pearson-Stuttard, J., Guillot, M., et al. (2020). Magnitude, demographics and dynamics of the effect of the first wave of the COVID-19 pandemic on all-cause mortality in 21 industrialized countries. Nat Med. 26, 1919–1928. doi: 10.1038/s41591-020-1112-0
Kunno, J., Supawattanabodee, B., Sumanasrethakul, C., Wiriyasivaj, B., Kuratong, S., and Kaewchandee, C. (2021). Comparison of different waves during the COVID-19 pandemic: retrospective descriptive study in Thailand. Adv. Prev. Med. 8, 5807056. doi: 10.1155/2021/5807056
Lue, E., and Wilson, J. P. (2017). Mapping fires and American Red Cross aid using demographic indicators of vulnerability. Disasters 41, 409–426. doi: 10.1111/disa.12198
Lyngse, F. P., Mortensen, L. H., Denwood, M. J., Christiansen, L. E., Møller, C. H., Skov, R. L., et al. (2021). SARS-CoV-2 omicron VOC transmission in danish households. medRxiv [Preprint]. doi: 10.1101/2021.12.27.21268278
Marmot, M. G., Smith, G. D., Stansfeld, S., Patel, C., North, F., Head, J., et al. (1991). Health inequalities among British civil servants: the Whitehall II study. Lancet 337, 1387–1393. doi: 10.1016/0140-6736(91)93068-K
Maslo, C., Friedland, R., Toubkin, M., Laubscher, A., Akaloo, T., and Kama, B. (2022). Characteristics and outcomes of hospitalized patients in South Africa during the COVID-19 omicron wave compared with previous waves. JAMA 327, 583–584 doi: 10.1001/jama.2021.24868
McDonald, R., and Spotswood, E. (2020). Cities Are Not to Blame for the Spread of COVID-19—nor Is the Demise of Cities an Appropriate Response. The Nature of Cities (2020). Available online at: https://www.thenatureofcities.com/2020/04/14/cities-are-not-to-blame-for-the-spread-of-covid-19-nor-is-the-demise-of-cities-an-appropriate-response/ (accessed September 21, 2020).
McMaughan, D. J., Oluyomi, O., and Lee, S. M. (2020). Socioeconomic status and access to healthcare: interrelated drivers for healthy aging. Front. Public Health. 8, 231. doi: 10.3389/fpubh.2020.00231
Morbidity and Mortality Weekly Report (MMWR) (2020). Centers for Disease Control and Prevention (CDC). Available online at: https://www.cdc.gov/mmwr/index.html (accessed September 15, 2020).
O'Hearn, M., Liu, J., Cudhea, F., Micha, R., and Mozaffarian, D. (2021). Coronavirus disease 2019 hospitalizations attributable to cardiometabolic conditions in the United States: a comparative risk assessment analysis. J. Am. Heart Assoc. 10, e019259. doi: 10.1161/JAHA.120.019259
Page, K. R., Venkataramani, M., Beyrer, C., and Polk, S. (2020). Undocumented U.S. immigrants and Covid-19. N Engl J Med. 382, e62. doi: 10.1056/NEJMp2005953
Paul, P., France, A. M., Aoki, Y., Batra, D., Biggerstaff, M., Dugan, V., et al. (2021). Genomic surveillance for SARS-CoV-2 variants circulating in the United States, December 2020–May 2021. MMWR Morb. Mortal. Wkly. Rep. 70, 846–850. doi: 10.15585/mmwr.mm7023a3
Qualls, N., Levitt, A., Kanade, N., Wright-Jegede, N., Dopson, S., et al. (2017). Community Mitigation Guidelines to Prevent Pandemic Influenza — United States, 2017. MMWR Recomm. Reports 66, 1–34. doi: 10.15585/mmwr.rr6601a1
Reitsma, M. B., Claypool, A. L., Vargo, J., Shete, P. B., McCorvie, R., Wheeler, W. H., et al. (2021). Racial/ethnic disparities in COVID-19 exposure risk, testing, and cases at the subcounty level in California. Health Aff. 40, 870–878. doi: 10.1377/hlthaff.2021.00098
Resnick, A., Galea, S., and Sivashanker, K. (2020). Covid-19: the painful price of ignoring health inequities. BMJ Opin. Available online at: https://blogs.bmj.com/bmj/2020/03/18/covid-19-the-painful-price-of-ignoring-health-inequities/
Richardson, S., Hirsch, J. S., Narasimhan, M., Crawford, J. M., McGinn, T., Davidson, K. W., et al. (2020). Presenting characteristics, comorbidities, and outcomes among 5700 patients hospitalized with COVID-19 in the New York City Area. J. Am. Med. Assoc. 323, 2052–2059. doi: 10.1001/jama.2020.6775
Rocklöv, J., and Sjödin, H. (2020). High population densities catalyse the spread of COVID-19. J. Travel Med. 27, taaa038. doi: 10.1093/jtm/taaa038
Rosenthal, B. M., Goldstein, J., Otterman, S., and Fink, S. (2020). Why Surviving the Virus Might Come Down to Which Hospital Admits You. The New York Times. Available online at: https://www.nytimes.com/2020/07/01/nyregion/Coronavirus-hospitals.html?utm_source=ig (accessed July 1, 2020).
Rufat, S., Tate, E., Emrich, C. T., and Antolini, F. (2019). How valid are social vulnerability models? Ann. Am. Assoc. Geogr. 109, 1131–1153. doi: 10.1080/24694452.2018.1535887
Saltelli, A. (2007). Composite indicators between analysis and advocacy. Soc. Indicat. Res. 81, 65–77. doi: 10.1007/s11205-006-0024-9
Schroeder, S. A. (2007). lecture. we can do better–improving the health of the American people. N. Engl. J. Med. 357, 1221–1228. doi: 10.1056/NEJMsa073350
Schuchat, A. (2020). Public Health Response to the Initiation and Spread of Pandemic COVID-19 in the United States. MMWR Morb. Mortal. Wkly. Rep. 69, 551–556. doi: 10.15585/mmwr.mm6918e2
Scully, J. L. (2020). Disability, disablism, and COVID-19 pandemic triage. J. Bioeth. Inq. 17, 601–605. doi: 10.1007/s11673-020-10005-y
Seong, H., Hyun, H. J., Yun, J. G., Noh, J. Y., Cheong, H. J., Kim, W. J., et al. (2021). Comparison of the second and third waves of the COVID-19 pandemic in South Korea: importance of early public health intervention. Int. J. Infect. Dis. 104, 742–745. doi: 10.1016/j.ijid.2021.02.004
Setia, M. S. (2016). Methodology series module 3: cross-sectional studies. Ind. J. Dermatol. 61, 261–264. doi: 10.4103/0019-5154.182410
Sharifi, A., and Khavarian-Garmsir, A. R. (2020). The COVID-19 pandemic: Impacts on cities and major lessons for urban planning, design, and management. Sci. Total Environ. 749, 142391. doi: 10.1016/j.scitotenv.2020.142391
Simonnet, A., Chetboun, M., Poissy, J., Raverdy, V., Noulette, J., Duhamel, A., et al. (2020). High prevalence of obesity in severe acute respiratory syndrome coronavirus-2 (SARS-CoV-2) requiring invasive mechanical ventilation. Obesity 28, 1195–1199. doi: 10.1002/oby.22831
Singh, G. K., and Jemal, A. (2017). Socioeconomic and racial/ethnic disparities in cancer mortality, incidence, and survival in the United States, 1950-2014: Over six decades of changing patterns and widening inequalities. J. Environ. Public Health 2017, 2819372. doi: 10.1155/2017/2819372
Song, M. K., Lin, F. C., Ward, S. E., and Fine, J. P. (2013). Composite variables: When and how. Nurs. Res. 62, 45–49. doi: 10.1097/NNR.0b013e3182741948
Stem, J. E. (1995). State Plane Coordinate System of 1983 (NOAA Manual NOS NGS 5). Rockville, MD: National Geodetic Information Center. Available online at: https://www.ngs.noaa.gov/library/pdfs/NOAA_Manual_NOS_NGS_0005.pdf
Tate, E. (2012). Social vulnerability indices: a comparative assessment using uncertainty and sensitivity analysis. Nat. Hazards 63, 325–347. doi: 10.1007/s11069-012-0152-2
Tennessee Department of Health (2021). ERSION 6.0 COVID-19 Vaccination Plan. Tennessee. Vaccination Plan. Available onlin at: https://www.tn.gov/content/dam/tn/health/documents/cedep/novel-coronavirus/COVID-19_Vaccination_Plan.pdf (accessed February 20, 2022).
Thebault, R., Tran, A. B., and Williams, V. (2020). African Americans are at Higher Risk of Death from Coronavirus. The Washington Post. Available online at: https://www.washingtonpost.com/nation/2020/04/07/coronavirus-is-infecting-killing-black-americans-an-alarmingly-high-rate-post-analysis-shows/?arc404=true (accessed September 15, 2020).
Thompson, M. G., Natarajan, K., Irving, S. A., Rowley, E. A., Griggs, E. P., Gaglani, M., et al. (2022). Effectiveness of a third dose of mRNA vaccines against COVID-19–associated emergency department and urgent care encounters and hospitalizations among adults during periods of delta and omicron variant predominance — VISION Network, 10 States, August 2021–January 2022. MMWR Morb. Mortal. Wkly. Rep. 71, 139–145. doi: 10.15585/mmwr.mm7104e3
Truman, B. I., Chang, M., and Moonesinghe, R. (2022). Provisional COVID-19 age-adjusted death rates, by race and ethnicity — United States, 2020–2021. MMWR Morb. Mortal. Wkly. Rep. 71, 601–605. doi: 10.15585/mmwr.mm7117e2
Unger, J. M., A. B., Moseley, Cheung, C. K., Osarogiagbon, R. U., B., Symington, Ramsey, S. D., et al. (2021). Persistent disparity: socioeconomic deprivation and cancer outcomes in patients treated in clinical trials. J. Clin. Oncol. 39, 1339–1348. doi: 10.1200/JCO.20.02602
U. S. Census Bureau (2021). Quick Facts United States. Available online at: https://www.census.gov/quickfacts/fact/table/US/AGE295219#AGE295219 (accessed February 20, 2022).
Wadhera, R. K. Y., Figueroa, J. F., Dominici, F., Yeh, R. W., and Joynt Maddox, K., E. (2020). Mortality and hospitalizations for dually enrolled and nondually enrolled medicare beneficiaries aged 65 years or older, 2004 to 2017. J. Am. Med. Assoc. 323, 961–969. doi: 10.1001/jama.2020.1021
Wang, F., and Robert, K. (2015). Constructing geographic areas by REDCAP and MLR for analysis of homicide rates: a case study of New Orleans, Louisiana. Pap. Appl. Geogr. 1, 295–306. doi: 10.1080/23754931.2015.1084355
Watson, D. F., and Philip, G. M. (1985). A refinement of Inverse distance weighted interpolation. Geoprocessing 2, 315–327.
WHO (2022). COVID-19 Weekly Epidemiological Update. World Health Organization. Available online at: https://www.who.int/emergencies/diseases/novel-coronavirus-2019/situation-reports (accessed August 31, 2022).
WHO Commission on Social Determinants of Health and WHO (2008). Closing the Gap in a Generation: Health Equity through Action on the Social Determinants of Health: Commission on Social Determinants of Health Final Report. Geneva: World Health Organization. Available online at: https://apps.who.int/iris/bitstream/handle/10665/43943/9789241563703_eng.pdf (accessed September 4, 2022).
Wilkinson, A., Ali, H., Bedford, J., Boonyabancha, S., Connolly, C., Conteh, A., et al. (2020). Local response in health emergencies: key considerations for addressing the COVID-19 pandemic in informal urban settlements. Environ. Urban. 32, 503–522. doi: 10.1177/0956247820922843
Wolter, N., Jassat, W., Walaza, S., Welch, R., Moultrie, H., Groome, M., et al. (2022). Early assessment of the clinical severity of the SARS-CoV-2 omicron variant in South Africa: a data linkage study. Lancet 399, 437–446. doi: 10.1016/S0140-6736(22)00017-4
Wu, X., Nethery, R. C., Sabath, M. B., Braun, D., and Dominici, F. (2020). Air pollution and COVID-19 mortality in the United States: Strengths and limitations of an ecological regression analysis. Sci. Adv. 6, eabd4049. doi: 10.1126/sciadv.abd4049
Wu, Z., and McGougan, J. (2020). Characteristics of and important lessons from the coronavirus disease 2019 (COVID-19) outbreak in China. Summary of a report of 72 314 cases from the Chinese Center for Disease Control and Prevention. J. Am. Med. Assoc. 323, 1239–1242.
Xu, Y., and Wang, F. (2015). Built environment and obesity by urbanicity in the US. Health Place 34, 19–29. doi: 10.1016/j.healthplace.2015.03.010
Keywords: social disadvantage index (SDI), disadvantaged areas, vulnerability, health outcomes, COVID-19
Citation: Antipova A (2022) Analysis of disease burden in socially disadvantaged areas: Mapping of geographical inequalities in COVID-19 morbidity and mortality using a social disadvantage index in Tennessee. Front. Sustain. Cities 4:876945. doi: 10.3389/frsc.2022.876945
Received: 16 February 2022; Accepted: 23 August 2022;
Published: 29 September 2022.
Edited by:
Patrick Lott Kinney, Boston University, United StatesReviewed by:
Courtney Page-Tan, Embry–Riddle Aeronautical University, United StatesCopyright © 2022 Antipova. This is an open-access article distributed under the terms of the Creative Commons Attribution License (CC BY). The use, distribution or reproduction in other forums is permitted, provided the original author(s) and the copyright owner(s) are credited and that the original publication in this journal is cited, in accordance with accepted academic practice. No use, distribution or reproduction is permitted which does not comply with these terms.
*Correspondence: Anzhelika Antipova, YW50aXBvdmFAbWVtcGhpcy5lZHU=
Disclaimer: All claims expressed in this article are solely those of the authors and do not necessarily represent those of their affiliated organizations, or those of the publisher, the editors and the reviewers. Any product that may be evaluated in this article or claim that may be made by its manufacturer is not guaranteed or endorsed by the publisher.
Research integrity at Frontiers
Learn more about the work of our research integrity team to safeguard the quality of each article we publish.