- 1Digital Geography Lab, Department of Geosciences and Geography, University of Helsinki, Helsinki, Finland
- 2Helsinki Institute of Urban and Regional Studies, University of Helsinki, Helsinki, Finland
- 3Helsinki Inequality Initiative, University of Helsinki, Helsinki, Finland
- 4Helsinki Institute of Sustainability Science, University of Helsinki, Helsinki, Finland
- 5Department of Geography, University of Tartu, Tartu, Estonia
The activity space approach is increasingly mobilized in spatial segregation research to broaden its scope from residential neighborhoods to other socio-spatial contexts of people. Activity space segregation research is an emerging field, characterized by quick adaptation of novel data sources and interdisciplinary methodologies. In this article, we present a methodological review of activity space segregation research by identifying approaches, methods and data sources applied. First, our review highlights that the activity space approach enables segregation to be studied from the perspectives of people, places and mobility flows. Second, the results reveal that both traditional data sources and novel big data sources are valuable for studying activity space segregation. While traditional sources provide rich background information on people for examining the social dimension of segregation, big data sources bring opportunities to address temporality, and increase the spatial extent and resolution of analysis. Hence, big data sources have an important role in mediating the conceptual change from a residential neighborhood-based to an activity space-based approach to segregation. Still, scholars should address carefully the challenges and uncertainties that big data entail for segregation studies. Finally, we propose a framework for a three-step methodological workflow for activity space segregation analysis, and outline future research avenues to move toward more conceptual clarity, integrated analysis framework and methodological rigor.
Introduction
The vast body of spatial segregation research focuses on residential patterning of social groups across urban neighborhoods (Massey and Denton, 1988; Tammaru et al., 2015). This has provided valuable knowledge on the social fabric of our cities, and on how social and physical characteristics of neighborhoods affect the life outcomes of their residents (Galster and Sharkey, 2017). Just as individuals are influenced by their residential neighborhoods, they are also exposed to and shaped by the other socio-spatial contexts of their daily life. However, the non-residential context is seldom covered in segregation studies that lead to socially, spatially and temporally limited understanding of individuals' exposure to or isolation from others. This also obscures the true nature of social inequalities and integration in society. This has been confirmed by a number of recent studies indicating that most individuals' daily activities and interactions take place in spaces with social compositions that are considerably different from their residential neighborhoods (Jones and Pebley, 2014; Toomet et al., 2015).
The scholarly focus on “night-time” segregation in residential neighborhoods over “the degree to which daytime population distributions display segregation” (Boal, 1987) is driven mainly by data availability. Segregation studies have primarily relied on census and register data (Boal, 1987; Petrović et al., 2019) that are easily accessible, at least on the spatially coarse and aggregate level. However, these data seldom include spatial information other than where people live (and in some cases where people work), which excludes the capture of individuals' other important activity locations (e.g., schools, leisure time activity sites) and mobility between those locations. Moreover, as census and register data are often made available at the administrative unit level (such as census tract or block group), the prevailing understanding of segregation and the approach to it has become residential neighborhood centered; that is, examining how segregated a place is, per se. In contrast, much less theoretical and empirical attention has been applied to the question of how segregated individuals' everyday lives in all their activity spaces are.
Inspired by the wider trends in social sciences several scholars have responsively called for researchers to incorporate new perspectives in studying segregation (Wang et al., 2012; Järv et al., 2015; Wissink et al., 2016; Sampson, 2017). The activity space approach to segregation (Wong and Shaw, 2011; Palmer, 2013) that builds on the concept of activity space (Golledge and Stimson, 1997) proposes that segregation is (re)produced across all locations that a person visits (for both social and asocial activities), and routes and areas the person travels through and around. The approach highlights the importance of both activity locations and spatial mobility in shaping people's segregation experiences. The idea is supported by the understanding brought by the mobility turn in social sciences (Sheller and Urry, 2006)—not only presence in but also mobility between activity locations entails embodied experiences with a cultural and social meaning (Cresswell, 2010). Mobility is also crucial for understanding interdependencies between segregation in residential neighborhoods, schools, workplaces and during leisure time.
More recent conceptualisations of segregation beyond residential neighborhoods are the domains approach by Tammaru et al. (2021), and spatiotemporal approach to examining multi-contextual segregation by Park and Kwan (2018). While having their own focuses, both approaches stress that, besides various spatial contexts, also temporal contexts are important for understanding segregation. Both approaches rely on time geography (Hägerstrand, 1970) that provides a conceptual framework for studying the spatial and temporal constraints of and exposures across an individual's space-time paths that reflect social inequalities across the population (Farber et al., 2015). For clarity, we hereafter refer to research that captures segregation beyond residential neighborhoods across individuals' multiple activity locations and/or mobility broadly as activity space segregation without limiting it to any conceptual approach.
Until two technological advancements made it feasible, research on activity spaces and space-time paths was long limited due to the constraints on collecting and analyzing detailed data on individuals' spatiotemporal behavior. First, increased computational capacities and new tools of geographic information systems have broadened opportunities for data-rich spatial analysis (Miller, 2005). Second, the emergence of big data sources such as mobile phones, social media platforms, smart cards, and GPS-enabled mobile devices provide novel and cost-efficient ways to capture detailed spatial and temporal information on our daily activities (Shelton et al., 2015; Wang et al., 2018). Despite the challenges (Zook et al., 2017), big data sources are increasingly mobilized in many social sciences (Kitchin, 2014; Halford and Savage, 2017) for “supplementing existing data sources and providing a richer understanding of the multiple social and spatial processes” in our cities (Zook et al., 2019).
These conceptual and methodological advancements make possible the paradigm shift from a residential neighborhood-based to an activity space-based approach to segregation. The new strand of activity space segregation research can be characterized by three main shifts on how segregation is comprehended and examined. First, studying segregation from the perspective of people by placing the dynamic life of an individual at the center of the analysis is gradually being recognized and considered to be as important as the conventional perspective of residential neighborhoods (Kwan, 2009; Farber et al., 2015; Petrović et al., 2019; Musterd, 2020). Second, this has led to the acknowledgment and incorporation of the wide variety of socio-spatial contexts from residential neighborhoods to the work and travel environments that people are exposed to during their spatiotemporally complex everyday lives, in segregation studies (Schnell, 2002; Järv et al., 2015; Zhang et al., 2019). Third, recognizing that the social composition of places is constantly changing due to individuals' mobility in space and time shifts the static notion of neighborhoods toward studying dynamic segregation levels in neighborhoods on one hand (Mooses et al., 2016; Le Roux et al., 2017), and individuals' exposure to their dynamic socio-spatial contexts on the other (Kwan, 2013; Östh et al., 2018).
An overview by Wong (2016) on the state of measuring spatial segregation highlights that to advance activity space segregation research “[e]ither existing measures have to be modified or new measures have to be developed to utilize individual-level data” (p. 94). Moreover, high hopes are set for the emergence of and constantly improving skills to apply big data sources in activity space segregation research (Järv et al., 2015; Bettencourt et al., 2019; Petrović et al., 2019). In recent years, several reviews have been published to advance activity space segregation research forward. Yao et al. (2019) focused on the development of spatial segregation measures, including indices for individual activity spaces. Cagney et al. (2020) discussed the incorporation of the activity space concept in sociological research (e.g., social inequality and segregation), and a systematic review by Bettencourt et al. (2019) focused on the micro-ecology of segregation in local spatial practices. Yet, a systematic overview on the methodological developments of the existing activity space segregation research is still lacking.
Against this backdrop, we present a methodological review of the studies that capture segregation beyond residential neighborhoods across individuals' multiple activity locations and/or mobility. With this review we aim to make a conceptual and methodological contribution to the evolving activity space segregation field in particular, and to spatial segregation research in general. Our three objectives are first, to identify what approaches, methods and data sources have been applied to study segregation in activity spaces; second, to assess how different data sources have contributed to spatially, temporally and socially more comprehensive understanding of activity space segregation, while giving special attention to the role of novel big data sources; and third, to draw attention to a number of challenges in the activity space segregation field to outline the avenues for future research.
Methods
Definition of Key Concepts
Our systematic literature search relied on the following two concepts: segregation and activity space. We understand segregation to be spatially uneven distributions and relationships—spatial arrangements and patterning and/or spatial interactions—between people belonging to different population groups (Yao et al., 2019). Often, the term spatial integration is used instead to refer to spatially even distributions of population groups and the relationships between them. Inspired by Golledge and Stimson (1997), we understand activity space as a geographic space that captures an individual's activity locations and mobility over a period of time.
When presenting results, we make a distinction between traditional data sources and big data sources. These are ambiguous terms that lack clear definition, yet are different from each other in terms of the qualities and characteristics of data (Kitchin, 2014). While the most common characteristics of big data are velocity (being produced continuously) and exhaustivity (n = all samples), traditional data sources are beyond some exceptions, such as register and census data, collected with sampling techniques, and have therefore limited volume, and spatial and temporal scope (Kitchin, 2014; Kitchin and McArdle, 2016). Here, we consider digitally produced data sources, such as mobile phone, social media, and smart card data, and data produced via mobile phone applications and collected via GPS tracking studies, to constitute big data. We consider census and register data, and data collected with surveys and interviews as traditional data.
Search Strategy
We conducted a systematic literature search in the Scopus database on 25 March 2019 to identify potential original and peer-reviewed activity space segregation studies published in English. The keywords identified around the following themes were used to search for potentially relevant studies: (1) segregation, and (2) activity space. Table 1 summarizes all the combinations of keywords, and the detailed search query is presented in Supplementary Material S1.
Inclusion and Exclusion Criteria
We sought to include in the current review all empirical activity space segregation studies. For that, we assessed each article, which was identified through database searching, against the following criteria: (1) investigated spatial segregation or spatial integration as defined in Section Definition of Key Concepts; (2) studied segregation across individuals' activity spaces as defined in Section Definition of Key Concepts with the conditions specified in the following criteria; (3) empirical investigation of activity spaces was based on individual-level location data capturing at minimum two activity locations or mobility between them; (4) activity space measurement included quantitative measurement of realized movement, behavior, activity or locations visited in geographical space. We excluded studies that: (1) used qualitative methods for examining activity spaces and segregation; and (2) studied micro-scale mobility in one activity location or socio-spatial context, e.g., limited to a dining hall. When assessing studies against inclusion and exclusion criteria, we treated segregation and activity space as umbrella terms. Thus, the inclusion of the study did not depend on the theoretical definition or concept used in the article reviewed, but on whether the empirical case study met the criteria listed above.
Study Selection and Data Extraction
We used a four-phase selection process to identify relevant articles, following the Preferred Reporting Items for Systematic Reviews and Meta-Analyses (PRISMA) Statement (Moher et al., 2009). The initial literature search resulted in 420 publications. After a careful assessment of the full texts, i.e., the contents of the articles, we identified 44 articles that were relevant for our methodological review (Figure 1). See Supplementary Material S2 for the results of literature search and article selection decisions.
We analyzed approaches, methods and data sources used in articles from the three dimensions of segregation: social, spatial and temporal. We paid particular attention to big data sources. In the case of studies using mixed methods, we reviewed only parts that use quantitative methods. The studies using both big data and traditional sources are classified as big data studies in the review as the latter sources have a supporting role. This study does not review the empirical findings, per se, nor assess the quality of each methodology. The list of reviewed articles with data extraction is provided in Supplementary Material S2.
Results
Data Sources and General Characteristics
Research on activity space segregation is relatively young (Figure 2) compared to the long history of residential segregation research (van Kempen and Özüekren, 1998). The first empirical studies on the role of everyday life spaces for understanding socio-spatial integration and isolation of members of social groups were conducted at the start of the 2000s (Scheiner, 2000). Back then, research on segregation in activity spaces was still uncommon—only a few articles were published in 2000–2010 and half of these by Schnell and colleagues (Schnell and Yoav, 2001; Goldhaber and Schnell, 2007). The yearly publication numbers have increased since then (Figure 2) totalling 44 quantitative activity space segregation articles published in various journals listed in the Scopus database by the time of conducting this review (see articles by journal in Supplementary Material S3).
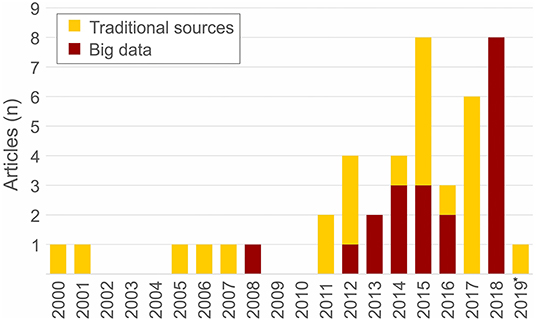
Figure 2. The number of articles on quantitative activity space segregation research in the Scopus database by year and data source type (n = 44). The year 2019 includes articles published until March 25.
Activity space segregation studies have used various data sources to analyse individuals' realized mobility and activity locations quantitatively (Figure 3). The studies rely exclusively on self-reported location data (n = 24) or on automatically collected location data (n = 15) or a combination of these two data sources (n = 5).
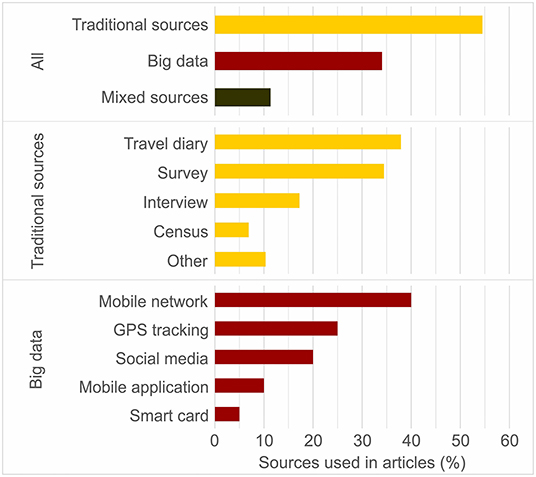
Figure 3. Distribution of articles by data source type used for analyzing activity spaces quantitatively (n = 44, top section). Distribution of traditional sources (n = 29, middle) and big data sources (n = 20, bottom) used in articles (n = 44).
Self-reported location data were collected using traditional methods, mainly travel diaries (n = 11) and surveys (n = 10), as shown in Figure 3. The earlier studies relied on specifically designed small-scale surveys, asking about respondents' everyday activity locations (Scheiner, 2000) or time spent in different sociocultural spaces (Schnell and Yoav, 2001). In more recent studies (Wang et al., 2012; Li and Wang, 2017), respondents were asked to fill in travel diaries. In addition, scholars have made use of extensive neighborhood/household (travel) surveys, conducted by state or regional authorities, that include information on respondents' key destinations (Jones and Pebley, 2014; Browning et al., 2017) or daily mobility (Wong and Shaw, 2011; Le Roux et al., 2017). To a lesser extent, interviewing (n = 5), mapping activities, deriving census data, and other statistical data sources were used.
Automatically collected location data were drawn from several novel big data sources in nearly half of the activity space segregation studies (n = 20; Figure 3). The first study on spatial mobility and social integration using big data was published by Licoppe et al. (2008), in which the location data of people, collected via a mobile phone application, was examined. Since 2012, the use of big data has been increasing (Figure 2). However, the diversity of big data sources is significant, e.g., in terms of how data are generated, and what social, spatial and temporal elements they include. The most widely applied big data source in activity space segregation studies was mobile phone data (n = 10; Figure 3), specifically using call detail records (n = 7) as described by Järv et al. (2015), network-driven 5-mins interval data (Östh et al., 2018) and data collected via mobile phone applications (Licoppe et al., 2008). Other data sources included GPS tracking data (Shdema et al., 2018), and social media data such as geographically located Twitter (Netto et al., 2018; Wang et al., 2018) and Flickr datasets (Li et al., 2018). One study used public transport smart card data (Lathia et al., 2012).
However, activity space segregation studies do not rely only on location data but also incorporate other quantitative and qualitative data to contextualize location data, characterize activity spaces and explain segregation. Additional data were more often used in studies employing big data than in studies using traditional data sources—80 and 54% of studies, respectively. Almost half of those studies used census data in addition, for example, to obtain neighborhood characteristics for examining individuals' exposure to different socio-economic contexts within one's activity space (Jones and Pebley, 2014; Li and Wang, 2017). Eight studies applied mixed methods in examining activity space segregation, e.g., by combining quantitative activity space analysis based on GPS tracking or survey data with a qualitative analysis based on interview data (Scheiner, 2000; Shdema et al., 2018).
The case studies on activity space segregation cover a range of geographical contexts, 15 countries in total (Figure 4). However, most of the studies are from Global North countries (75% of all case studies) such as the United States and Israel (Shdema et al., 2018; Wang et al., 2018), where integration and residential segregation between racial or ethno-sectarian groups has historically been a much-examined topic. Interestingly, China (n = 6) and Estonia (n = 5) stand out with a number of studies (Figure 4). In China, studies focused on income groups (Zhou et al., 2015), and people residing in a range of housing types (Li and Wang, 2017) or neighborhoods (Wang et al., 2012). The research in Estonia focused on the difference between language groups as proxies for ethnicity, based on mobile phone data (Järv et al., 2015; Mooses et al., 2016; Silm et al., 2018).
In terms of the size of the research population, the studies are highly heterogeneous, ranging from 12 people (Roulston and Young, 2013) to 5.1 million people (Lathia et al., 2012). The median size of the research population in activity space segregation studies was around 1,200 people. A clear distinction emerges between studies relying on novel big data sources and on traditional data sources (Figure 5). That is, most studies (58%) in the latter group tend to be based on 100 to 10,000 people. This is due to the limitations of the dominant data source in this group: surveys (median sample size 683 people) and travel diaries (median sample size 1,100 people). In contrast, most of the big-data-based studies (70%) are either based on very small (<100) or large (>10,000) research populations. The largest datasets used include public transport smart card data (Lathia et al., 2012), mobile phone data (Östh et al., 2018) and social media data (Wang et al., 2018). Big data studies based on small research populations tend to use GPS tracking (Roulston and Young, 2013; Shdema et al., 2018) or mobile phone applications (Licoppe et al., 2008). This group also includes several pilot studies with the aim to test and introduce new methodologies (Greenberg Raanan and Shoval, 2014) and data collection methods to segregation research (Licoppe et al., 2008; Roulston and Young, 2013). Three studies did not reveal their research population size.
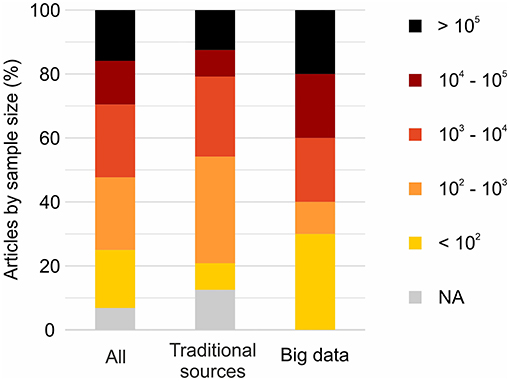
Figure 5. Distribution of articles by the size of the research population of all activity space segregation studies (n = 44), and separately of studies using traditional data sources (n = 24) and big data sources (n = 20).
Social Dimension
Activity space segregation studies, like residential segregation research, focus mainly on socioeconomic, and ethnic, racial, religious and linguistic dimensions (Figure 6). The studies on socioeconomic dimensions examined segregation in various geographical contexts, mainly based on income (Netto et al., 2018; Östh et al., 2018), occupational (Shen, 2019) and educational (Le Roux et al., 2017) groups. The studies conducted in China also investigated population groups defined by the type of housing (Zhang et al., 2019) and residential neighborhood (Wang et al., 2012). Studies on ethnic segregation are geographically more concentrated—the majority of studies investigated Arabs and Jews in Israel (Shdema et al., 2018), Estonian- and Russian-speakers in Estonia (Järv et al., 2015; Mooses et al., 2016), and racial groups in the United States (Wong and Shaw, 2011; Farber et al., 2015). Some studies also examined segregation by age (Li and Wang, 2017) and by place of residence (Li et al., 2018). Most of the studies have taken the perspective of one social dimension, however a few studies formed population groups by intersecting background characteristics such as age and language (Silm et al., 2018), and race and income level (Wang et al., 2018).
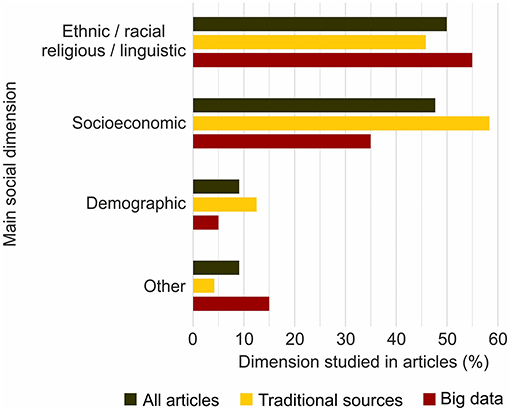
Figure 6. Distribution of main social dimensions along which activity space segregation was studied in all articles (n = 44), and in articles using traditional data sources (n = 24) and big data sources (n = 20).
There were no significant differences in the main social dimensions investigated between the studies applying big data sources and traditional data sources, except that the socioeconomic dimension is more examined based on traditional data sources (Figure 6). However, there was a clear difference between these two data source categories in terms of “social richness”, i.e., what background information is known about the individuals who are examined. In particular, one-third of big-data-based studies (n = 6) used a complementary data source such as a census (Netto et al., 2018), register (Östh et al., 2018) or community survey data (Wang et al., 2018) to derive social attributes along which segregation was studied. This was done by estimating each individual's residential location based on data themselves and spatially linking them with corresponding residential neighborhood characteristics. In contrast, only two articles relying on a traditional data source used complementary data sources for deriving social attributes (Wong and Shaw, 2011; Cordoba Calquin et al., 2017). In addition, individuals' various other background characteristics were included, mainly in statistical analysis, often in studies that relied on survey and travel diary data.
Spatial Dimension
Activity space segregation studies vary considerably in terms of the extent to which activity spaces are examined. Most studies (75%, n = 33) were aimed at investigating an individual's entire activity space, although the spatial resolution varied between data collection methods. For example, some studies were based on self-reporting addresses of frequently visited activity locations in a survey (Browning et al., 2017), some on recording a person's precise GPS coordinates every 10 s for a week (Greenberg Raanan and Shoval, 2014), and others on analyzing a mobile network operator's base station coverage areas where a person had made phone calls and sent messages during 1 year (Järv et al., 2015). When comparing data source types, the proportion of studies focusing on the whole activity spaces of people is the same for studies using big data and studies using traditional data sources. The rest of the studies (25%, n = 11) examined some parts of an activity space. From these studies, seven focused on individuals' daily mobility between the main anchor points of their lives—home and work (Farber et al., 2015), or home and school (Cordoba Calquin et al., 2017). Four other studies focused on some parts of the activity space, such as out-of-home activities (Zhou et al., 2015), out-of-home non-employment activities (Silm and Ahas, 2014) or out-of-home non-employment activities on public and national holidays (Mooses et al., 2016).
Another spatial aspect that limits capturing and examining activity spaces, and therefore activity space segregation, is the geographical extent of a case study, i.e., the geographical coverage of the data used (Figure 7). From the articles we reviewed, the spatial extent of the case studies ranges from a city (Wang et al., 2018) to transnational level (Silm and Ahas, 2014). However, most articles (66%, n = 29) focused on a city or a metropolitan area, whereas in particular, these studies relied more often on traditional data sources like travel diaries (Le Roux et al., 2017) and questionnaire surveys (Schnell and Yoav, 2001). Instead, studies using big data tended to be geographically less limited in capturing an individual's activity space—almost half of the big data studies (n = 9) captured individual activity spaces on a country or even transnational level. Some of these studies made use of the wider geographical coverage of the data to analyse segregation simultaneously at multiple spatial scales such as at a district scale in a city, at a municipality scale in a country, and at a country scale transnationally (Silm and Ahas, 2014). Here, the strength of big data sources is clearly visible as automated data collection is not geographically limited as traditional data collection methods. For example, mobile phone-based calling data (Silm and Ahas, 2014) and specific phone application data (Licoppe et al., 2008) allow an individual's whereabouts to be captured globally.
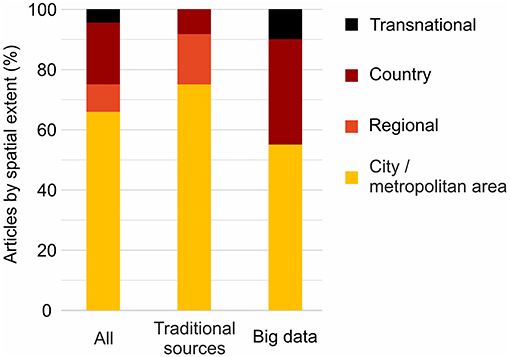
Figure 7. Distribution of articles by the spatial extent of the case study in all activity space segregation studies (n = 44), and separately in studies using traditional data sources (n = 24) and big data sources (n = 20).
Temporal Dimension
Activity space segregation studies vary considerably in terms of whether and how the time dimension is considered, i.e., what is the length of the study period and whether segregation is studied as a dynamic phenomenon. In general, activity space segregation studies cover a range of time periods when an individual's mobility and activities are captured (Figure 8). The data used for examining activity spaces range from 1 day (Östh et al., 2018) up to several years, whereas the maximum period studied was about 10 years (Li et al., 2018). The latter study relied on social media data with infrequent spatial locations that was compensated for by having a longer study period. However, 25% of the studies (n = 11) were atemporal—they did not define any study period nor time units. In these studies, an individual's activity space did not capture mobility and activities from a certain period, but it represented one's “routine” activity space. For example, Browning et al. (2017) relied on a neighborhood survey in which respondents were asked to report on their commonly visited activity locations.
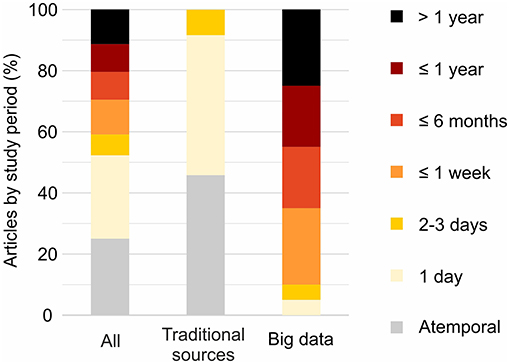
Figure 8. Distribution of articles by the length of the study period of all activity space segregation studies (n = 44), and separately of studies using traditional data sources (n = 24) and big data sources (n = 20).
There is a clear distinction between big data sources and traditional data sources regarding temporality – big data sources enable better integrated temporality in activity space segregation research (Figure 8). Several studies using traditional data sources (46%, n = 11) were atemporal (Scheiner, 2000; Jones and Pebley, 2014). The studies relying exclusively on traditional sources covered the longest only two consecutive days (Wang et al., 2012; Tan et al., 2017). In contrast, only one big data based study was limited to 1 day (Östh et al., 2018). Some 90% of the big data based studies captured activity spaces at least for 1 week (Shdema et al., 2018), and 45% of the studies at least for 1 year (Järv et al., 2015; Shelton et al., 2015).
Having information about an individual's whereabouts and activities in time allows the segregation phenomenon to be examined dynamically (Kwan, 2013)—how segregation in space changes over time. Interestingly, only one-third (32%, n = 14) of the activity space segregation studies examined segregation dynamically (Table 2). Once again, there is a clear distinction between big data sources and traditional data sources. About 40% of the big-data-based studies (n = 8) examined segregation dynamically, whereas the proportion is 25% for studies using traditional data (n = 6). These studies in the latter group relied predominantly on travel diary data (n = 5) such as Zhou et al. (2015) and Tan et al. (2017).
Dynamic activity space segregation studies address temporality in several ways. It can be a repeated segregation measurement at points of time with a constant time unit such as each hour of the day (Le Roux et al., 2017), different weekdays (Wang et al., 2012), different seasons (Shelton et al., 2015) or comparing regular work days against public holidays (Mooses et al., 2016). Also, temporality can be regarded as the segregation measurement of the same research population at different time scales such as measuring segregation regarding an individual's daily, monthly and yearly activity space (Järv et al., 2015). While these highlighted studies calculate both activity space and segregation measures separately for each period, one can also provide a global segregation metric based on activity spaces of people from several time frames. For example, Östh et al. (2018) measure the mobility of people at 5-min intervals and Toomet et al. (2015) measure locations of people with 1-h interval, which are the input data for calculating a generic metric to describe dynamic segregation. These two studies demonstrate the value of operationalising the co-presence concept—people being in the same location at the same time—in segregation research.
Activity Space Measurement
The three previous subsections described how social, spatial and temporal dimensions are addressed in activity space segregation studies. Yet, it is important to understand how people's activity spaces are measured as an input for studying segregation. We broadly categorize whether characteristics of activity spaces are calculated at the individual level for each person (individual-level measures) or at the aggregate level—measured by social group, spatial unit or movement flows between locations (aggregate-level measures).
In general, most studies (70%, n = 31) calculated individual-level activity space measures, whereas half of these studies also included activity space measurement at the aggregate level (Figure 9). For example, Schnell et al. (2015) and Li and Wang (2017) measured activity spaces solely at the individual level, while Wang and Li (2016) and Silm et al. (2018) examine activity spaces both at the individual and at the aggregate level. Studies relying on traditional and big data sources apply individual-level measures equally often.
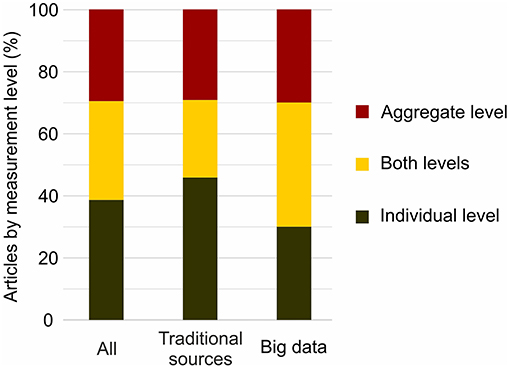
Figure 9. Distribution of articles by activity space measurement level in all studies (n = 44), and separately in studies using traditional data sources (n = 24) and big data sources (n = 20).
In the articles we reviewed, various individual-level activity space metrics were calculated (Figure 10A). Half of the studies examining an individual's activity spaces focused on (a number of) locations visited, whereas this was more often based on big data sources (Toomet et al., 2015; Wang et al., 2018). Almost equally often, movement behavior between activity locations (Wong and Shaw, 2011) and time spent in spatial units (Goldhaber and Schnell, 2007; Schnell et al., 2015) was used to characterize activity spaces. The spatial extent (area) of an individual's activity space (Järv et al., 2015) was studied less often. Additionally, one study calculated the configuration of an individual's activity space from the spatial structure of visited activity locations using the entropy metric (Silm et al., 2018).
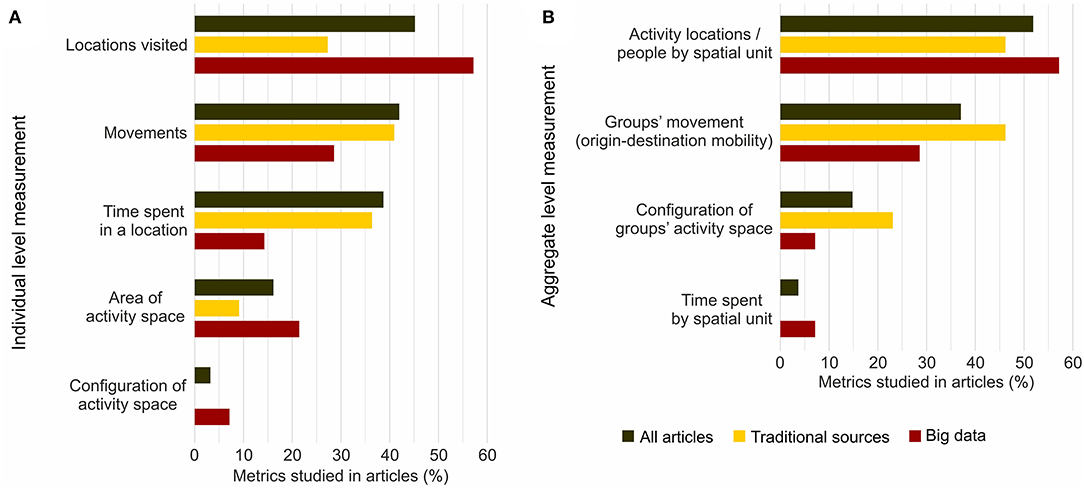
Figure 10. Distribution of individual (A) and aggregate (B) level activity space metrics used in all studies (n = 44), and separately in studies using traditional data sources (n = 24) and big data sources (n = 20).
In terms of aggregate level activity space measures, two metrics were predominantly used (81%; Figure 10B). First, locations visited by people were aggregated to predefined spatial units such as city districts and municipalities (Mooses et al., 2016), or census areas (Lathia et al., 2012). Second, movement of people between activity locations was aggregated to a physical street network (Netto et al., 2018) or to an origin-destination type of flow matrix (Shen, 2019). The aggregations to spatial units tend to be applied more with big data sources, and aggregation to movement flows in studies relying on large-scale (travel) surveys, census data and other statistical data products.
Segregation Measurement
The final aspect to understanding how activity space segregation is studied is to examine how segregation measures are calculated. That is, whether a segregation metric per se is calculated for a spatial unit (place-based), a movement flow (flow-based), an individual's or a group's activity space (people-based), or is a mix of these approaches (combined measure). Most studies (82%, n = 36) calculated people-based segregation measures (Figure 11). These are studies that either calculated a segregation metric for a social group being studied (Zhou et al., 2015) or for each individual. The latter approach is data-demanding and poses methodological challenges for making generalizations. However, Schnell and Yoav (2001) calculated individual-level segregation indices, Li and Wang (2017) used statistical regression measurements, and Greenberg Raanan and Shoval (2014) applied a geovisual map comparison method.
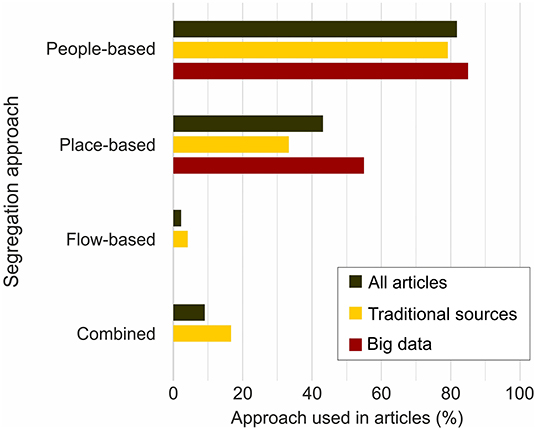
Figure 11. Distribution of approaches used to study activity space segregation in all studies (n = 44), and separately in studies using traditional data sources (n = 24) and big data sources (n = 20).
However, as data on individual activity spaces were often aggregated into predefined spatial units, 43% of studies calculated place-based segregation metrics based on activity locations of people. This tends to be more common in studies using big data sources (Figure 11). For example, Silm et al. (2018) calculated a dissimilarity index based on the distribution of activity locations across study districts, Farber et al. (2015) applied a social interaction potential metric to identify and map spatial patterns in social contact opportunities, and Östh et al. (2018) used a co-presence metric to examine and compare exposure to poverty and wealth in different urban areas at different times.
Only one study used a clear flow-based segregation metric (Shen, 2019), where a segregation indicator was calculated for each movement flow between two spatial units. Additionally, a few studies (n = 4) combined people-based and place-based, and flow-based and place-based approaches. For instance, Wong and Shaw (2011) calculated one aggregate activity space segregation measure for people living in the same census tract, based on their activity spaces. Interestingly, more than one-third of articles (36%, n = 16) applied two segregation measurement approaches in a case study. For example, Järv et al. (2015) relied mainly on the people-based approach while providing additional contextualization by using a place-based measure. Nevertheless, half of all studies (n = 22) relied solely on people-based segregation measures.
Discussion and Conclusions
The incorporation of the activity space perspective has opened new avenues for segregation research. To provide a comprehensive overview about the methodological developments in this field, we systematically reviewed 44 quantitative segregation analyses that relied on individual-level data on activity locations and/or in-between mobility. We identified the methodological approaches employed in studying activity space segregation to date, and assessed the contribution of different data sources to examining its spatial, temporal and social dimensions.
Our methodological review has some limitations. First, the review does not include all articles on activity space segregation as we focused on the ones published in the journals listed in the Scopus database. Still, compared to Web of Science, Scopus covers considerably more scientific journals, including in social sciences, and around 99% of the journals indexed in Web of Science are also listed in Scopus (Singh et al., 2021). Second, some relevant articles for the review may not have been detected due to keyword mismatches, despite carefully listing keywords for the database search to cover the research topic (Table 1). Nevertheless, we believe that the database search resulted in a broad set of publications that is not biased and therefore our review is sufficiently representative to provide a cross-cutting overview on the approaches, methods and data sources used. Finally, our review does not assess the rigor of methodologies, as this crucial issue requires separate investigation and should therefore be addressed in future research.
Notwithstanding the limitations, this paper provides the first comprehensive overview about the methodological status of the activity space segregation research to feed into much-needed conceptual and methodological debates and developments in future. We draw particular attention to the increasing use of big data sources in segregation research to initiate the discussion about their appropriateness, and about the opportunities and challenges that these novel data sources entail. Next, we discuss our conclusions around the four central topics of the review: (1) high conceptual and methodological heterogeneity; (2) the contribution of different data sources to examining the spatial, temporal and social dimension of segregation; (3) opportunities and challenges introduced by big data; (4) avenues for future research.
Conceptual and Methodological Heterogeneity
Our review highlights that activity space segregation research is an emerging field that is characterized by high conceptual and methodological heterogeneity, driven by several factors. First, the activity space approach to segregation implies that both activity locations and human mobility are considered to be an integral part of segregation research. Moreover, an increasing number of segregation studies aim to examine the temporal dimension, be it segregation over hours, days, months, or seasons. To capture the various forms of spatial differentiation, its causes and consequences, segregation is studied from various disciplinary as well as interdisciplinary perspectives. This disciplinary diversity that now also includes computer sciences makes it challenging to develop coherent and commonly agreed methodologies that successfully integrate all components. Second, as almost half of the quantitative activity space segregation studies rely on big data sources, we also have to bear in mind that big data research is itself a new way of producing knowledge and entails a number of uncertainties (Kitchin, 2014; see Section Big Data Bring New Challenges). Third, the field has emerged within the last decade, and we are only at the beginning of the development toward a more coherent research field.
High heterogeneity is not necessarily unfavorable, yet different studies often endorse different conceptualisations and operationalisations to examine same aspects. We noticed two main areas of conceptual fuzziness: how (1) segregation, and (2) various socio-spatial contexts are conceptualized and captured. The latter has been addressed by proposing and advancing various approaches, such as the activity space approach (Wong and Shaw, 2011), the domains approach (Tammaru et al., 2021), and the spatiotemporal approach (Park and Kwan, 2018). However, there are alternative views on what qualifies as segregation in this context. Our review shows that the concept of segregation has been operationalised by measuring and comparing differences in people's activity space measures, or by employing traditional segregation indices. The strategy chosen often depends on the disciplinary background of authors: geographers, demographers, sociologists, transport researchers, computer scientists or social psychologists. Certainly, disciplinary diversity has always been fundamental to segregation research, but the incorporation of the activity space approach and big data sources requires new types of collaboration to move toward more conceptual clarity, integrated analysis framework, and methodological rigor.
One valuable contribution of the activity space approach to segregation is that it enables us to comprehend and capture segregation beyond residential neighborhoods from the perspectives of places, people and movement flows (Kwan, 2009; Palmer, 2013). Different perspectives enrich our understanding on how segregation may manifest itself and be experienced. To date, the perspective of places has attracted most attention in segregation research, and consequently most segregation policies focus on residential neighborhoods and social mixing. Our review shows that the increasing application of the activity space approach has brought more attention also to the perspective of people, i.e., segregation patterns regarding people's daily lives (see Section Segregation Measurement). Several studies from Asia, Europe and the United States have demonstrated the importance of this approach by showing that even though people's activity spaces are often more socially heterogeneous than their residential neighborhoods, many remain substantially segregated throughout their daily lives (Jones and Pebley, 2014; Wang and Li, 2016; Östh et al., 2018; Wang et al., 2018). The perspective of movement flows, which has been studied the least, is equally important, as it uncovers segregation regarding the mobility of people that remains otherwise invisible. Having an extended overview on how spatially uneven patterns and relationships between people unfold from different angles enables policy-makers to develop more targeted integration policies, by focusing on places (e.g., housing mix), people (e.g., education and employment), and connections (e.g., public transport) (van Ham et al., 2018).
Different perspectives—place-, people- and flow-based segregation—are also useful for guiding our methodological choices in this heterogeneous field. We propose a three-step methodological workflow (Figure 12) as a framework to facilitate thinking on the various methodological steps for more coherent quantitative activity space segregation analysis. The initial research problem and study objectives determine whether segregation should be investigated as a place-, people- or flow-based phenomenon, or a combination of those. This guides us to select the most fit-for-purpose individual-level dataset, be it a traditional or a big data source. While a dataset comprising spatial (and temporal) information on activity locations and/or in-between mobility is a prerequisite for any activity space segregation analysis, some data sources are more suitable than others for exploring different perspectives (see Section Traditional and Big Data Sources—Valuable in Their Own Ways).
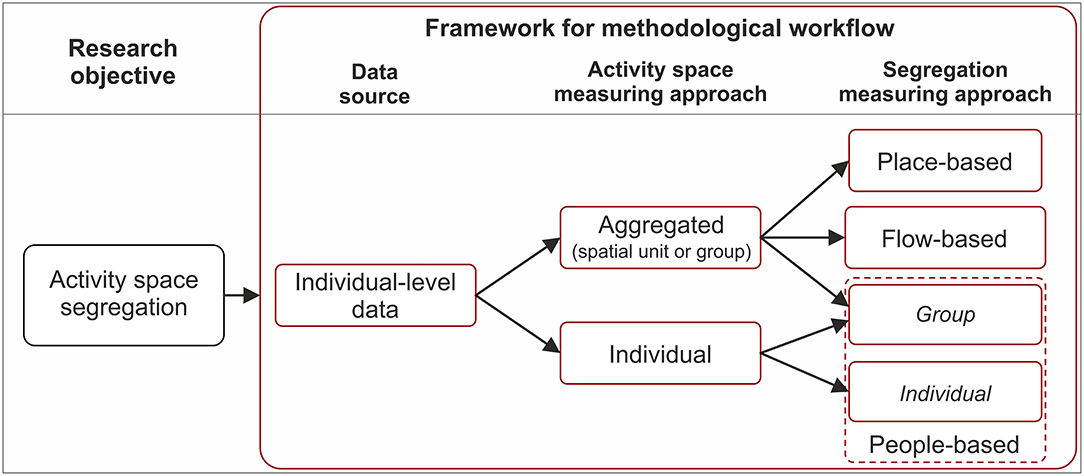
Figure 12. Framework for a three-step methodological workflow for quantitative activity space segregation analysis.
After selecting the most fit-for-purpose dataset, there are two options for measuring activity space—whether to measure activity spaces at the individual level or at the aggregated level (Figure 12). The former means calculating a metric for every individual (e.g., the extent of an individual's daily activity space) and the latter provides a metric for a population group or spatial unit (e.g., distribution of population group's activity locations across neighborhoods). This is dependent on and determines the options for measuring segregation in the final step. Measuring activity spaces at individual level allows a segregation metric to be calculated, either for each individual or for a population group. In both cases, this reveals how segregated are people's lives across their activity spaces, i.e., provide insights into people-based segregation (Kwan, 2009). In contrast, once individual activity spaces are aggregated to predefined study units such as census tracts, one can only calculate place-based segregation metrics and assess how segregated a study area is. Similarly, flow-based segregation metrics (Shen, 2019) can be calculated for home-work commuting flows once all individuals' movements are aggregated to connections between spatial units.
Traditional and Big Data Sources—Valuable in Their Own Ways
Current studies on activity space segregation have mobilized a wide range of data sources that are seen as “holding promise for maximizing urban mobility and activity space contribution” in urban sociological research (Cagney et al., 2020). Our review shows that traditional and big data sources have contributed equally to activity space segregation field by broadening the analysis focus from residential neighborhoods to other socio-spatial contexts of people (Petrović et al., 2019). However, data sources have distinctive characteristics—benefits and limitations—that define and restrict the ways in how to measure segregation. Thus, some data sources are better for examining certain dimensions of segregation—spatial, temporal, or social—than others (Figure 13).
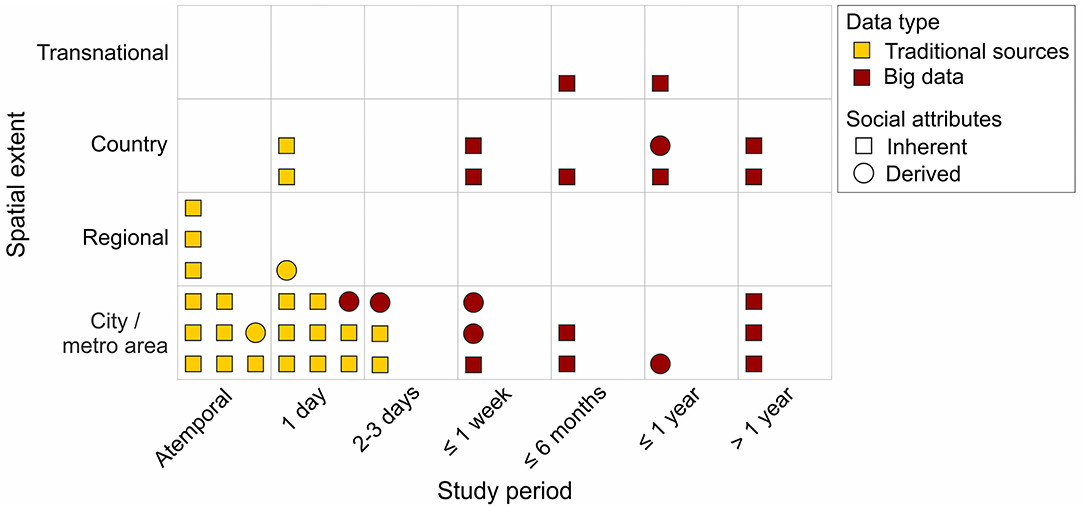
Figure 13. Distribution of activity space segregation studies by spatial and temporal dimension (n = 44). Studies are further divided by whether social attributes for segregation analysis are inherent in data or derived from a complementary data source.
Big data, such as mobile phone and social media data, have the potential to untie segregation research from its current spatial and temporal boundaries. From the spatial perspective, these sources allow us to study segregation across individuals' whole activity spaces and stretch the geographical extent of segregation studies from conventional administrative borders (neighborhoods, cities) to national and transnational dimensions (Figure 13). This is important, since an individual's life is not confined to a residential neighborhood (Piekut et al., 2019) nor often to a single city or a country (Mooses et al., 2020). Moreover, a wider geographical coverage of data allows us to uncover segregation processes that occur at different spatial scales. A study by Silm and Ahas (2014), for example, found that the spatial differentiation between ethnic groups' out-of-home non-employment activities increased when the spatial extent of analysis was extended from respondents' home city to the whole country. Then again, advancements regarding traditional sources have also broadened our opportunities to provide new insights beyond residential neighborhoods. For instance, a relational database that connects various registers enables us to link individuals' main habitual activity locations such as residences, schools and workplaces over their life course (Tammaru et al., 2021).
Big data sources are also valuable for incorporating temporality in segregation analysis and capturing co-presence. Activity space segregation studies relying on traditional data sources (e.g., surveys) are either atemporal or cover 1 or 2 days in people's lives. Instead, big data sources can cover weeks, months and even years (Figure 13), and thus allow us to reveal both individuals' daily activity spaces and less routine spatial behavior related to multi-local living or business and holiday trips (Järv et al., 2015). For example, a year-long study from Estonia compared the daily, monthly and annual activity spaces of the country's two biggest ethno-linguistic groups and found that the differences were the most profound not between daily routine activities but between annual activity spaces that include leisure-related activities and trips that take place over a longer periodicity (Järv et al., 2015). Further, combining spatial and temporal dimensions uncovers the actual co-presence of people (Toomet et al., 2015), which allows us to investigate individuals' experienced exposure to their dynamic socio-spatial contexts across their activity spaces.
The spatiotemporal accuracy of examining individuals' exposure to their actual socio-spatial contexts depends on input data and determines the scale(s) at which segregation can be measured. On one hand, mobile phone call detail records and social media data with lower spatiotemporal resolution can capture segregation at coarser spatial scales, but from longer study periods. And even though macro-scale segregation analyses entail more uncertainties due to spatial non-stationarity, they are important for obtaining a more comprehensive understanding about segregation processes that occur at higher spatial scales (Manley et al., 2015). On the other hand, data collected with GPS devices, mobile phone applications or travel diaries can uncover individuals' hourly and daily segregation experiences at the micro-scale, yet from a shorter period. For instance, high-resolution GPS data have been used to define person-specific neighborhoods (Park and Kwan, 2018) which allow individuals' segregation experiences to be captured more adequately than administrative units. Moreover, avoiding aggregation of location data to predefined spatial units enables us to address the modifiable areal unit problem (MAUP)—a methodological challenge well-known among spatial segregation researchers. Any individual-level data with high spatiotemporal resolution is a prerequisite for this.
The social dimension is the third inherent dimension of segregation that concerns the background characteristics of people, and information on perceptions, experiences and reasons. Traditional sources such as surveys and travel diaries usually include rich background information on study participants, whereas this information is limited or missing from several big data sources (Figure 13). In order to use big data to analyse segregation, several scholars have derived people's background characteristics using data fusion, by spatially linking social media data with census data (Netto et al., 2018), or mobile network data with register data (Östh et al., 2018). When the objective is to examine perceptions and experiences besides segregation patterns, traditional sources such as surveys and interviews are crucial. For example, combining a GPS tracking study with interviews provides socially rich data with high spatiotemporal resolution (while having a limited study period and sample size). A study by Greenberg Raanan and Shoval (2014) demonstrated this well—a combination of data from in-depth interviews, mental maps and GPS tracking enabled the authors to find a strong relationship between the perceptions of the segregated city and the actual spatial behavior of three conflicting cultural groups in Jerusalem. Finally, the potentials of rich social media content including text and images for examining segregation remain undiscovered.
To conclude, the wide variety of traditional and big data sources provide opportunities for capturing activity space segregation from different perspectives and for giving more attention to particular dimensions of segregation. Being aware of the specific benefits and limitations of data sources is crucial for selecting the most fit-for-purpose dataset for each specific segregation study (see Figure 12). For instance, individual-level census or register data on home and work locations might be the best for studying segregation across commuting flows, but detailed GPS tracking data reveal individuals' actual space-time paths and exposure over 24 h. One potential way forward is to combine traditional and big data sources to benefit from the strengths of both sources (Järv et al., 2020).
Big Data Bring New Challenges
Segregation researchers are generally aware of the limitations of traditional data sources, whereas using big data sources for scientific knowledge production entails new kinds of challenges and uncertainties. Therefore, in addition to the challenges related to the conceptual and methodological heterogeneity of the emerging activity space segregation field (see Section Conceptual and Methodological Heterogeneity), one has to be aware of the big-data-specific challenges to make the best use of various data sources. Here, we briefly reflect on some challenges relevant to segregation studies.
Using individual-level data with high spatiotemporal resolution and extensive social content requires careful consideration of possible ethical and privacy issues, especially when dealing with vulnerable groups and sensitive aspects of human behavior. Yet, ethical standards and privacy protection regulations have significant differences between countries—even in the Global North (Pernot-Leplay, 2020), where most activity space segregation studies are conducted. However, in any context, researchers have the ultimate responsibility to minimize potential harm of their research (Zook et al., 2017).
Another challenge of using big data relate to the uncertainties of whom and what the data represent. First, as big data do not represent the whole population (e.g., age and gender biases), nor all activity locations and mobility, this has to be acknowledged and dealt with care to avoid making over-simplistic interpretations and amplifying existing inequalities (Toivonen et al., 2019). One way to mitigate this is to make big data small and meaningful (Poorthuis and Zook, 2017). Second, various algorithmic uncertainties are introduced when collecting, generating, processing and analyzing big data (Kwan, 2016). In the context of segregation studies, this is evident when deriving people's background characteristics using indirect data fusion (see Section Spatial Dimension). This entails uncertainties regarding the identification and division of study groups. That said, various uncertainties should be addressed when using traditional data. For example, respondents' subjectivity and selective memory are inherent in survey and travel diary data (Järv et al., 2020). Overall, scholars have to be critical about the representativeness of and uncertainties related to any data source and methodology used, and articulate these clearly when publishing and presenting research results.
Despite various challenges, we need to acknowledge that big data analytics, similar to activity space segregation research, is a rapidly developing field that is searching for a coherent research tradition from ontological (Kitchin and McArdle, 2016), methodological (Toivonen et al., 2019) to ethical perspectives (Markham et al., 2018). This also unfolds in our review as it includes several big-data-based studies that are methodological showcases or explorative in nature. However, we can expect that the realization of the potential of big data in segregation research improves in line with the developments in big data research more broadly. At the same time, following the principles of responsible big data research is utterly important for realizing the potential of big data in segregation research while avoiding the harm it may cause (Zook et al., 2017; Poom et al., 2020).
Future Research Avenues
Our methodological review highlights that despite the short history and high conceptual and methodological heterogeneity, the activity space approach provides new valuable perspectives to comprehend and capture segregation. However, further conceptual and methodological debates and developments are necessary to move toward more conceptual clarity, integrated analysis framework, and methodological rigor.
First, a more coherent understanding of the concept of segregation and its operationalisation is needed within the activity space segregation field, to avoid further blurring of the concept. For instance, what is the difference between studying segregation and differences in people's activity spaces? Secondly, a number of methodological challenges should be addressed to be able to better navigate in the highly diverse field of activity space segregation research, and make informed decisions on the appropriateness of different data sources, methods and measures for addressing specific research questions. As a number of methods and measures are proposed, tested and developed, the field would benefit from further scrutiny on the effectiveness of these methods and measures. Moreover, the appropriateness of different data sources, and especially the challenges and implications of big data, should be further examined. For instance, the uncertainties of whom and what the data represent, and the performance of different techniques used for deriving social attributes require in depth investigation.
We propose that an effective development of a more integrated conceptual and methodological framework requires tight interdisciplinary capacity-building, as the approaches, methods and measures mobilized originate from different disciplines and discourses. Although segregation research has always been an interdisciplinary field, the emerging trend to incorporate the activity space approach and big data sources to segregation research requires new collaborations between segregation scholars, transport/mobility researchers and data scientists. This sounds trivial, yet we noticed that some studies suffered from a weak linkage to segregation theory from being conducted by the scholars of one field only.
Given that activity space segregation research involves data sources, methods and measures that are often unfamiliar to traditional segregation research, ensuring methodological transparency of each study is of paramount importance. Our review indicated that this is often not the case. Thus, besides clarifying a methodological workflow (Figure 12), we highly recommend implementing open science practices—sharing codes, tools and data openly, if possible. This will allow us to advance toward a more coherent research framework and conduct comparative research in different contexts. Even if data cannot be published openly due to privacy concerns, opening metadata as comprehensively as possible allows for a better understanding of the analysis undertaken, especially in the case of big data sources.
Finally, we stress the value of examining segregation from the perspective of places, people and movement flows (Figure 12). By answering the three questions—“how segregated are neighborhoods?”, “how segregated are individuals' activity spaces?” and “how segregated are human mobility flow patterns between activity locations?”—, we gain a fuller understanding of segregation in society from several angles and are able to develop more effective policy interventions. Although all perspectives require further investigation, we would like to emphasize two prospective directions that are greatly overlooked. First, bringing people- and place-based perspectives together enables us to study people's experienced exposure based on their constantly changing co-presence with other people across their activity space. Second, although societies are much about movements and spaces of flows (Sheller and Urry, 2006), the flow-based perspective has received minimal attention (see Shen, 2019). This is a critical direction to advance with, as segregation across movement flows reflects and reinforces mobility injustice (Cook and Butz, 2018). Certainly, subsequent systematic reviews on the empirical results of activity space segregation studies would further enrich our understanding on the contribution of different perspectives to capturing segregation.
Data Availability Statement
The original contributions presented in the study are included in the article and Supplementary Material, further inquiries can be directed to the corresponding author.
Author Contributions
KM: conceptualization, methodology, data curation, investigation, formal analysis, visualization, writing—original draft, review and editing, project administration, and funding acquisition. OJ: conceptualization, methodology, validation, visualization, writing—original draft, review and editing, and funding acquisition. TTa: conceptualization, methodology, writing—original draft, review and editing, supervision, and funding acquisition. TTo: conceptualization, methodology, writing—original draft, review and editing, and supervision. All authors contributed to the article and approved the submitted version.
Funding
This work was supported by the Kone Foundation (201608739 and 201710415), the Academy of Finland (Grant Number 331549), the Estonian Research Council (Grant Number PUT PRG306), and the Estonian Academy of Sciences (research professorship of TTa).
Conflict of Interest
The authors declare that the research was conducted in the absence of any commercial or financial relationships that could be construed as a potential conflict of interest.
Publisher's Note
All claims expressed in this article are solely those of the authors and do not necessarily represent those of their affiliated organizations, or those of the publisher, the editors and the reviewers. Any product that may be evaluated in this article, or claim that may be made by its manufacturer, is not guaranteed or endorsed by the publisher.
.Acknowledgments
We thank the members of the Digital Geography Lab at the University of Helsinki for their comments and suggestions on this review.
Supplementary Material
The Supplementary Material for this article can be found online at: https://www.frontiersin.org/articles/10.3389/frsc.2022.861640/full#supplementary-material
References
Bettencourt, L., Dixon, J., and Castro, P. (2019). Understanding how and why spatial segregation endures: a systematic review of recent research on intergroup relations at a micro-ecological scale. Soc. Psychol. Bull. 14:33482. doi: 10.32872/spb.v14i2.33482
Boal, F. W. (1987). “Segregation,” in Social Geography: Progress and Prospect, ed M. Pacione (Kent: Croom Helm) (pp. 90-128).
Browning, C. R., Calder, C. A., Krivo, L. J., Smith, A. L., and Boettner, B. (2017). Socioeconomic segregation of activity spaces in urban neighborhoods: does shared residence mean shared routines? RSF 3, 210. doi: 10.7758/rsf.2017.3.2.09
Cagney, K. A., York Cornwell, E., Goldman, A. W., and Cai, L. (2020). Urban mobility and activity space. Annu. Rev. Sociol. 46, 623–648. doi: 10.1146/annurev-soc-121919-054848
Cook, N., and Butz, D., (eds.). (2018). Mobilities, Mobility Justice and Social Justice. London: Routledge.
Cordoba Calquin, C. A., Farris, M., and Rojas Patuelli, K. (2017). Discussing school socioeconomic segregation in territorial terms: the differentiated influence of urban fragmentation and daily mobility. Investig. Geográficas 92, 54766. doi: 10.14350/rig.54766
Cresswell, T. (2010). Towards a politics of mobility. Environ. Plan. D Soc. Space 28, 17–31. doi: 10.1068/d11407
Farber, S., O'Kelly, M., Miller, H. J., and Neutens, T. (2015). Measuring segregation using patterns of daily travel behavior: a social interaction based model of exposure. J. Transp. Geogr. 49, 26–38. doi: 10.1016/j.jtrangeo.2015.10.009
Galster, G., and Sharkey, P. (2017). Spatial foundations of inequality: a conceptual model and empirical overview. RSF 3, 1. doi: 10.7758/rsf.2017.3.2.01
Goldhaber, R., and Schnell, I. (2007). A model of multidimensional segregation in the Arab Ghetto in Tel Aviv-Jaffa. Tijdschrift Voor. Econ. En Soc. Geografie 98, 603–620. doi: 10.1111/j.1467-9663.2007.00428.x
Golledge, R. G., and Stimson, R. J. (1997). Spatial Behavior: A Geographic Perspective. New York, NY: Guilford Press.
Greenberg Raanan, M., and Shoval, N. (2014). Mental maps compared to actual spatial behavior using GPS data: a new method for investigating segregation in cities. Cities 36, 28–40. doi: 10.1016/j.cities.2013.09.003
Hägerstrand, T. (1970). What about people in Regional Science? Pap. Reg. Sci. Assoc. 24, 6–21. doi: 10.1007/BF01936872
Halford, S., and Savage, M. (2017). Speaking sociologically with big data: symphonic social science and the future for big data research. Sociology 51, 1132–1148. doi: 10.1177/0038038517698639
Järv, O., Masso, A., Silm, S., and Ahas, R. (2020). The link between ethnic segregation and socio-economic status: an activity space approach. Tijdsch. Voor Econ. Soc. Geograf. 2020, tesg.12465. doi: 10.1111/tesg.12465
Järv, O., Müürisepp, K., Ahas, R., Derudder, B., and Witlox, F. (2015). Ethnic differences in activity spaces as a characteristic of segregation: a study based on mobile phone usage in Tallinn, Estonia. Urban Stud. 52, 2680–2698. doi: 10.1177/0042098014550459
Jones, M., and Pebley, A. R. (2014). Redefining neighborhoods using common destinations: social characteristics of activity spaces and home census tracts compared. Demography 51, 727–752. doi: 10.1007/s13524-014-0283-z
Kitchin, R. (2014). The data revolution: big data, open data, data infrastructures and their consequences. Sage. doi: 10.4135/9781473909472
Kitchin, R., and McArdle, G. (2016). What makes big data, big data? exploring the ontological characteristics of 26 datasets. Big Data Soc. 3, 205395171663113. doi: 10.1177/2053951716631130
Kwan, M.-P. (2009). From place-based to people-based exposure measures. Soc. Sci. Med. 69, 1311–1313. doi: 10.1016/j.socscimed.2009.07.013
Kwan, M.-P. (2013). Beyond space (as we knew it): toward temporally integrated geographies of segregation, health, and accessibility. Ann. Assoc. Am. Geogr. 103, 1078–1086. doi: 10.1080/00045608.2013.792177
Kwan, M.-P. (2016). Algorithmic geographies: big data, algorithmic uncertainty, and the production of geographic knowledge. Ann. Am. Assoc. Geogr. 106, 274–282. doi: 10.1080/00045608.2015.1117937
Lathia, N., Quercia, D., and Crowcroft, J. (2012). “The hidden image of the city: sensing community well-being from urban mobility,” in Pervasive Computing, Volume 7319, eds J. Kay, P. Lukowicz, H. Tokuda, P. Olivier, and A. Krüger (Berlin: Springer), 91–98.
Le Roux, G., Vallée, J., and Commenges, H. (2017). Social segregation around the clock in the Paris region (France). J. Transp. Geogr. 59, 134–145. doi: 10.1016/j.jtrangeo.2017.02.003
Li, D., Zhou, X., and Wang, M. (2018). Analyzing and visualizing the spatial interactions between tourists and locals: a Flickr study in ten US cities. Cities 74, 249–258. doi: 10.1016/j.cities.2017.12.012
Li, F., and Wang, D. (2017). Measuring urban segregation based on individuals' daily activity patterns: a multidimensional approach. Environ. Plan. A 49, 467–486. doi: 10.1177/0308518X16673213
Licoppe, C., Diminescu, D., Smoreda, Z., and Ziemlicki, C. (2008). Using mobile phone geolocalisation for 'socio-geographical' analysis of co-ordination, urban mobilities, and social integration patterns. Tijdsch. Voor Econ. Soc. Geogr. 99, 584–601. doi: 10.1111/j.1467-9663.2008.00493.x
Manley, D., Johnston, R., Jones, K., and Owen, D. (2015). Macro-, meso- and microscale segregation: modeling changing ethnic residential patterns in Auckland, New Zealand, 2001–2013. Ann. Assoc. Am. Geogr. 105, 951–967. doi: 10.1080/00045608.2015.1066739
Markham, A. N., Tiidenberg, K., and Herman, A. (2018). Ethics as methods: doing ethics in the era of big data research-introduction. Soc. Med. Soc. 4, 205630511878450. doi: 10.1177/2056305118784502
Massey, D. S., and Denton, N. A. (1988). The dimensions of residential segregation. Soc. Forces 67, 281–315. doi: 10.2307/2579183
Miller, H. J. (2005). “What about people in geographic information science?,” in Re-presenting GIS, eds P. F. Fisher and D. J. Unwin (New York, NY: John Wiley and Sons, Ltd), 215–242.
Moher, D., Liberati, A., Tetzlaff, J., Altman, D. G., and The PRISMA Group. (2009). Preferred reporting items for systematic reviews and meta-analyses: the PRISMA statement. PLoS Med. 6, e1000097. doi: 10.1371/journal.pmed.1000097
Mooses, V., Silm, S., and Ahas, R. (2016). Ethnic segregation during public and national holidays: a study using mobile phone data. Geografiska Ann. Ser. B Human Geogr. 98, 205–219. doi: 10.1111/geob.12100
Mooses, V., Silm, S., Tammaru, T., and Saluveer, E. (2020). An ethno-linguistic dimension in transnational activity space measured with mobile phone data. Human. Soc. Sci. Commun. 7, 140. doi: 10.1057/s41599-020-00627-3
Musterd, S. (2020). “Urban segregation: Contexts, domains, dimensions and approaches,” in Handbook of Urban Segregation, ed S. Musterd (London: Edward Elgar Publishing), 2–17.
Netto, V. M., Meirelles, J. V., Pinheiro, M., and Lorea, H. (2018). A temporal geography of encounters. Cybergeo 43, 28985. doi: 10.4000/cybergeo.28985
Östh, J., Shuttleworth, I., and Niedomysl, T. (2018). Spatial and temporal patterns of economic segregation in Sweden's metropolitan areas: a mobility approach. Environ. Plan. A Econ. Space 50, 809–825. doi: 10.1177/0308518X18763167
Palmer, J. R. B. (2013). Activity-Space Segregation: Understanding Social Divisions in Space and Time. PhD Dissertation. Princeton, NJ: Princeton University.
Park, Y. M., and Kwan, M.-P. (2018). Beyond residential segregation: a spatiotemporal approach to examining multi-contextual segregation. Comput. Environ. Urban Syst. 71, 98–108. doi: 10.1016/j.compenvurbsys.2018.05.001
Pernot-Leplay, E. (2020). China's approach on data privacy law: a third way between the U.S. and the EU? Penn State J. Law Int. Affairs 8, 49–117. Available online at: https://elibrary.law.psu.edu/jlia/vol8/iss1/6
Petrović, A., Manley, D., and van Ham, M. (2019). Freedom from the tyranny of neighbourhood: Rethinking sociospatial context effects. Prog. Hum. Geogr. 44, 1103–1123. doi: 10.2139/ssrn.3153380
Piekut, A., Pryce, G., and van Gent, W. (2019). Segregation in the twenty first century: processes, complexities and future directions: segregation in the twenty first century. Tijdschrift Voor Econ. Soc. Geogr. 110, 225–234. doi: 10.1111/tesg.12355
Poom, A., Järv, O., Zook, M., and Toivonen, T. (2020). COVID-19 is spatial: ensuring that mobile big data is used for social good. Big Data Society 7, 2053951720952088. doi: 10.1177/2053951720952088
Poorthuis, A., and Zook, M. (2017). Making big data small: strategies to expand urban and geographical research using social media. J. Urban Technol. 24, 115–135. doi: 10.1080/10630732.2017.1335153
Roulston, S., and Young, O. (2013). GPS tracking of some Northern Ireland students—patterns of shared and separated space: divided we stand? Int. Res. Geogr. Environ. Educ. 22, 241–258. doi: 10.1080/10382046.2013.817722
Sampson, R. J. (2017). Urban sustainability in an age of enduring inequalities: advancing theory and ecometrics for the 21st-century city. Proc. Nat. Acad. Sci. 114, 8957–8962. doi: 10.1073/pnas.1614433114
Scheiner, J. (2000). Activity spaces in the Western and Eastern part of Berlin: Socio-spatial integration or ongoing division? Die Erde 131, 143–160.
Schnell, I. (2002). “Segregation in everyday life spaces: a conceptual model,” in Studies in segregation and disegregation, ed I. Schnell and W. Ostendorf (Surrey: Ashgate), 39–65.
Schnell, I., Diab, A. A. B., and Benenson, I. (2015). A global index for measuring socio-spatial segregation versus integration. Appl. Geogr. 58, 179–188. doi: 10.1016/j.apgeog.2015.01.008
Schnell, I., and Yoav, B. (2001). The sociospatial isolation of agents in everyday life spaces as an aspect of segregation. Ann. Assoc. Am. Geogr. 91, 622–636. doi: 10.1111/0004-5608.00262
Shdema, I., Haj-Yahya, N., and Schnell, I. (2018). The social space of Arab residents of mixed Israeli cities. Geograf. Ann. Ser. B Human Geogr. 2018, 1–18. doi: 10.1080/04353684.2018.1428496
Sheller, M., and Urry, J. (2006). The new mobilities paradigm. Environ. Plan. A 38, 207–226. doi: 10.1068/a37268
Shelton, T., Poorthuis, A., and Zook, M. (2015). Social media and the city: rethinking urban socio-spatial inequality using user-generated geographic information. Landsc. Urban Plan. 142, 198–211. doi: 10.1016/j.landurbplan.2015.02.020
Shen, Y. (2019). Segregation through space: a scope of the flow-based spatial interaction model. J. Transp. Geogr. 76, 10–23. doi: 10.1016/j.jtrangeo.2019.02.007
Silm, S., and Ahas, R. (2014). Ethnic differences in activity spaces: a study of out-of-home nonemployment activities with mobile phone data. Ann. Assoc. Am. Geogr. 104, 542–559. doi: 10.1080/00045608.2014.892362
Silm, S., Ahas, R., and Mooses, V. (2018). Are younger age groups less segregated? Measuring ethnic segregation in activity spaces using mobile phone data. J. Ethnic Migr. Stud. 44, 1797–1817. doi: 10.1080/1369183X.2017.1400425
Singh, V. K., Singh, P., Karmakar, M., Leta, J., and Mayr, P. (2021). The journal coverage of web of science, scopus and dimensions: a comparative analysis. Scientometrics 126, 5113–5142. doi: 10.1007/s11192-021-03948-5
Tammaru, T., Ham, M. van, Marcińczak, S., and Musterd, S., (eds.). (2015). Socio-Economic Segregation in European Capital Cities: East Meets West. London: Routledge.
Tammaru, T., Knapp, D., Silm, S., van Ham, M., and Witlox, F. (2021). Spatial underpinnings of social inequalities: a vicious circles of segregation approach. Social Inclusion 9, 65–76. doi: 10.17645/si.v9i2.4345
Tan, Y., Kwan, M.-P., and Chai, Y. (2017). Examining the impacts of ethnicity on space-time behavior: Evidence from the City of Xining, China. Cities 64, 26–36. doi: 10.1016/j.cities.2017.02.003
Toivonen, T., Heikinheimo, V., Fink, C., Hausmann, A., Hiippala, T., Järv, O., et al. (2019). Social media data for conservation science: a methodological overview. Biol. Conserv. 233, 298–315. doi: 10.1016/j.biocon.2019.01.023
Toomet, O., Silm, S., Saluveer, E., Ahas, R., and Tammaru, T. (2015). Where do ethno-linguistic groups meet? how copresence during free-time is related to copresence at home and at work. PLoS ONE 10, e0126093. doi: 10.1371/journal.pone.0126093
van Ham, M., Tammaru, T., and Janssen, H. (2018). A Multi-Level Model of Vicious Circles of Socio-Economic Segregation. In Divided Cities. Berlin: OECD Publishing.
van Kempen, R., and Özüekren, A. S. (1998). Ethnic segregation in cities: new forms and explanations in a dynamic world. Urban Stud. 35, 1631–1656. doi: 10.1080/0042098984088
Wang, D., and Li, F. (2016). Daily activity space and exposure: a comparative study of Hong Kong's public and private housing residents' segregation in daily life. Cities 59, 148–155. doi: 10.1016/j.cities.2015.09.010
Wang, D., Li, F., and Chai, Y. (2012). Activity spaces and sociospatial segregation in Beijing. Urban Geogr. 33, 256–277. doi: 10.2747/0272-3638.33.2.256
Wang, Q., Phillips, N. E., Small, M. L., and Sampson, R. J. (2018). Urban mobility and neighborhood isolation in America's 50 largest cities. Proc. Nat. Acad. Sci. 115, 7735–7740. doi: 10.1073/pnas.1802537115
Wissink, B., Schwanen, T., and van Kempen, R. (2016). Beyond residential segregation: introduction. Cities 59, 126–130. doi: 10.1016/j.cities.2016.08.010
Wong, D. W. (2016). “From Aspatial to Spatial, from Global to Local and Individual: Are We on the Right Track to Spatialize Segregation Measures?,” in Recapturing Space: New Middle-Range Theory in Spatial Demography, eds F. M. Howell, J. R. Porter, and S. A. Matthews (Berlin: Springer International Publishing), 77–98.
Wong, D. W. S., and Shaw, S.-L. (2011). Measuring segregation: an activity space approach. J. Geogr. Syst. 13, 127–145. doi: 10.1007/s10109-010-0112-x
Yao, J., Wong, D. W. S., Bailey, N., and Minton, J. (2019). Spatial segregation measures: a methodological review. Tijdsch. Voor Econ. Soc. Geogr. 110, 235–250. doi: 10.1111/tesg.12305
Zhang, X., Wang, J., Kwan, M.-P., and Chai, Y. (2019). Reside nearby, behave apart? Activity-space-based segregation among residents of various types of housing in Beijing, China. Cities 88, 166–180. doi: 10.1016/j.cities.2018.10.009
Zhou, S., Deng, L., Kwan, M.-P., and Yan, R. (2015). Social and spatial differentiation of high and low income groups' out-of-home activities in Guangzhou, China. Cities 45, 81–90. doi: 10.1016/j.cities.2015.03.002
Zook, M., Barocas, S., Crawford, K., Keller, E., Gangadharan, S. P., Goodman, A., et al. (2017). Ten simple rules for responsible big data research. PLoS Comput. Biol. 13, e1005399. doi: 10.1371/journal.pcbi.1005399
Keywords: spatial segregation, activity space, human mobility, methodological review, big data, literature review
Citation: Müürisepp K, Järv O, Tammaru T and Toivonen T (2022) Activity Spaces and Big Data Sources in Segregation Research: A Methodological Review. Front. Sustain. Cities 4:861640. doi: 10.3389/frsc.2022.861640
Received: 24 January 2022; Accepted: 21 April 2022;
Published: 23 May 2022.
Edited by:
Thomas J. Vicino, Northeastern University, United StatesReviewed by:
Mei-Po Kwan, The Chinese University of Hong Kong, ChinaAte Poorthuis, KU Leuven, Belgium
Copyright © 2022 Müürisepp, Järv, Tammaru and Toivonen. This is an open-access article distributed under the terms of the Creative Commons Attribution License (CC BY). The use, distribution or reproduction in other forums is permitted, provided the original author(s) and the copyright owner(s) are credited and that the original publication in this journal is cited, in accordance with accepted academic practice. No use, distribution or reproduction is permitted which does not comply with these terms.
*Correspondence: Kerli Müürisepp, kerli.muurisepp@helsinki.fi