- 1Department of Civil and Environmental Engineering, Stanford University, Stanford, CA, United States
- 2Department of Psychiatry and Behavioral Sciences, Stanford University, Stanford, CA, United States
- 3Graduate School of Education and the Woods Institute for the Environment, Stanford University, Stanford, CA, United States
- 4Department of Psychology, Stanford University, Stanford, CA, United States
- 5School of Engineering, Dartmouth College, Hanover, NH, United States
- 6Department of Psychology and Child Development, California Polytechnic State University, San Luis Obispo, CA, United States
- 7Department of Computer Science, Stanford University, Stanford, CA, United States
There has been growing interest among scholars regarding the role of the built environment on occupant wellbeing. Across five studies conducted online from January 2018 to July 2021, we investigate the impact of design interventions (materials, light, and decor representing diverse identities) on several constructs indicative of wellbeing (sense of belonging, self-efficacy, and environmental efficacy), using self-reported metrics. We hypothesize that natural materials, natural light and diverse representations lead to higher self-reported scores compared to artificial materials, no natural light and non-diverse representations. We find that, while our results vary across individual experiments, the synthesized effects of materials and light on all three dependent measures hold consistent across studies, supporting our hypothesized outcomes. We also examine the influence of seasonality, survey platform and design, and independent variables' dosage on survey results. We conclude with a discussion on the challenges associated with researching the psychological as well as behavioral impacts of design interventions in indoor spaces.
Introduction
Urbanization and modern day lifestyles have caused the majority of Americans to spend 87% of their day indoors (Klepeis et al., 2001). As a result, buildings have acquired a strong potential to promote human wellbeing through physical design interventions. Recent years have seen an increase in the number of research studies exploring the impact of indoor built spaces on occupants. For example, studies show that built features in work environments can influence employees' physical health (Bornehag et al., 2001), productivity (O'Neill, 2010) and psychological wellness (Thatcher and Milner, 2014). Natural light and views to nature in the workplaces has been proven to boost creativity (Dul et al., 2011) as well as cognitive performance (Heschong, 2003). Presence of natural light and windows in hospital workspaces has also been shown to improve mood and communication (Zadeh et al., 2014). Presence of biophilic design elements in the workplace has been linked to lower stress levels (Yin et al., 2019). There is also limited research demonstrating the link between presence of natural material (wood) and preference for a space in a hospital setting (Nyrud et al., 2014). However, a deeper understanding of how specific indoor environment design interventions affect wellbeing is desirable.
Studying human subjects in realistic physical spaces may be time-intensive and costly. Online, survey-based studies offer a quicker, lower-cost method of investigating the potential impact of physical spaces on wellbeing, and they provide preliminary results that can be used to design human-subjects experiments with targeted design interventions in laboratory and field settings. The use of online crowdsourcing platforms in particular has many benefits, such as data reliability and scalability, as well as access to a more demographically diverse participant sample compared to student subjects, who can sometimes be overrepresented in some lab-based human subject experiments (Sears, 1986; Behrend et al., 2011; Wazny, 2018). Increased participants' diversity means that difficult-to-reach populations or populations with more work experiences compared to a typical university sample, for e.g., are more likely to be included in the data collection process.
In this paper, we compare results from five online studies focused on evaluating the impact of various physical interventions on wellbeing outcomes. Our work focuses on exploring two key dimensions of human wellness: social and psychological wellness (Hettler, 1976). In the studies discussed in this publication, we focus on investigating the effects of materials (natural vs. artificial), light (natural vs. no natural), and representation (diverse vs. non-diverse) on three specific constructs of wellbeing: sense of belonging and self-efficacy, which explore social and psychological dimensions of wellbeing (Hettler, 1976), as well as environmental efficacy, which is also correlated with human wellbeing (Kaida and Kaida, 2016). Sense of belonging refers to seeing oneself as socially connected, and it has been shown to promote stress reduction (Bolger et al., 2000). Self-efficacy relates to an individual's capability to exercise control over his/her behavior and actions (Bandura, 1997). Environmental efficacy is an individual's belief of how able they perceive themselves of reducing their negative impact on the environment by engaging in pro-environmental behavior (Sellers et al., 2014). Materials (Nyrud and Bringslimark, 2010) and light (Edwards and Torcellini, 2002) have been studied in the context of indoor spaces and wellbeing before, but, to our knowledge, not in relation to their impact on sense of belonging and environmental efficacy. Additionally, the selection of representation as an independent variable was motivated by prior work on sense of belonging and symbols in the physical environment (Cheryan et al., 2009). With these variables, we hypothesize that natural materials (vs. artificial materials), natural light (vs. no natural light), and diverse representations (vs. non-diverse representations) will lead to higher self-reported scores on the wellbeing metrics of belonging, self-efficacy, and environmental efficacy.
An additional objective of our work is to assess the advantages as well as challenges of conducting research using online platforms to provide guidelines and recommendations for effectively executing and evaluating future work using similar methodological approaches. We selected two online crowdsourcing recruitment platforms for our research, Amazon Mechanical Turk (MTurk) and Prolific. In recent years, MTurk has emerged as a prominent crowdsourcing platform for research (Paolacci and Chandler, 2014), and several studies report MTurk as a source of representative data and reliable results (Buhrmester et al., 2016). However, there are growing concerns of participant non-naivety when using MTurk to conduct scholarly research (Chandler et al., 2015) as well as concerns about poor data quality as many MTurk workers have become “professional survey takers” and may no longer pay as much attention to individual surveys. Prolific is a relatively newer platform that provides similar capabilities to MTurk and offers access to more naive populations (Peer et al., 2017). In addition to exploring different online study platforms, we also administered the study at different points of time over a longitudinal period and examined our research questions using different experimental designs.
Materials and Methods
As mentioned, a key goal in undertaking these studies was to employ a variety of methodological setups (e.g., recruitment platform, timeframe, experimental design) in order to explore how these different choices may impact outcomes. This section reviews such differences, commonalities, and other details of the five studies we performed.
All five studies were developed on the cloud-based software platform Qualtrics, and were completed by respondents located in the USA and satisfying a 95% approval rate by other researchers. Table 1 provides an overview of the studies, with more details about characteristics of the studies given in the subsequent sections. All studies were covered by the same Institutional Review Board (IRB) protocol (#48481), and participants were paid $1.80 for Studies 1–4 (10–15 min), and $1 for Study 5 (2–3 min). Table 2 shows the demographic distributions of the respondents for each survey, after exclusions (exclusions are described in Section Studies 1, 2, and 3: independent conditions design).
Studies 1, 2, and 3: Independent Conditions Design
Studies 1, 2, and 3 had identical experimental designs with independent conditions and were conducted on MTurk at different times of the year, thus enabling us to test our hypotheses across different seasons. Study 1 was conducted before the COVID-19 outbreak while Studies 2 and 3 were conducted during the pandemic.
The survey for the studies was framed as a research study on the modern workplace. These surveys used a within-subject design approach, meaning that each participant was shown all six study conditions in a randomized order: artificial materials, natural materials, no natural light, natural light, diverse representations, and non-diverse representations. For each condition, five pictures were shown. The pictures were photographs taken with a mobile device on the lead authors' university campus, in Northern California (Figure 1). The pictures were taken in various types of spaces found across a university such as co-working spaces, communal areas, conference rooms, and corridors. Pictures selected for the materials and light conditions were chosen so that all sets contained a similar balance of different types of spaces. Additionally, for the natural light set, the size and resolution of the pictures did not allow our participants to clearly distinguish the content of the views through the windows. Picture selection involved a careful review of the interactions between our different variables of interest. For instance, between the artificial and natural materials sets, we sought to have a similar balance of photographs with and without natural light in both sets.
For each condition, participants were given the following prompt: “These images depict spaces and decorations in your new workplace. Please imagine yourself in this workplace and answer the questions that follow.” They then answered a fixed set of eight questions: three for sense of belonging in the space, two for self-efficacy, and three for environmental efficacy. All questions were in the form of 7-point Likert scales, ranging from 1 = “Strongly Disagree” to 7 = “Strongly Agree.” The eight questions were:
“I feel like I belong in this space.”
“I am similar to the kind of people who succeed in this space.”
“I feel like other people in this space have the same values as me.”
“If someone opposed me in this space, I could find means and ways to get what I want.”
“It would be easy for me to stick to my aims and accomplish my goals in this space.”
“I would recycle newspaper in this space”
“I would recycle cans or bottles in this space”
“I would pick up litter that is not my own in this space”
These questions were adapted from previously established instruments; i.e., the Sense of Social Fit Scale (Walton and Cohen, 2007) for the sense of belonging construct; the General Self-Efficacy Scale (Schwarzer and Jerusalem, 1995) for the self-efficacy construct, and the Environmental Attitudes Inventory (Milfont and Duckitt, 2010) for the environmental efficacy construct. The Cronbach's alpha values for all studies are reported in Table 3.
Data exclusions for Study 1 and Study 2 were based on data integrity assessed by looking at unnatural answer patterns and self-reported attention issues. Unnatural answer patterns included the same score repeated across all questions, alternating values, and increasing or decreasing scores. Additionally, three concluding questions were added to the survey to gauge attention and ask if the participant had given the survey their full attention, if they read each question carefully, and if they believed we should use their data and responses. Participants who answered “no” to any one of the three final questions were excluded.
This exclusion approach was introduced to reduce the likelihood of using bots' data in our analyses (Chmielewski and Kucker, 2020). The data exclusion approach was updated in Study 3 to consist of three attention check questions systematically included at different points in the survey, and participants who failed two or more attention checks were discarded from the analysis. Attention checks were questions with evident answers such as “Please select “Slightly Disagree,” “Please select the color blue,” or “What was this survey about?” Participants were also checked for patterned answers and excluded using a similar criteria as Studies 1 and 2.
Studies 4 and 5: Factorial Design
For Studies 4 and 5, we produced a new set of images that feature a single, realistic office environment. The new pictures were photographs taken from a human-subjects laboratory experiment conducted on the lead authors' university campus (Figure 2). This experiment involved eight different room layouts, each containing a different combination of material, light, and representation type. Variations in material types were seen with different furniture and frame materials (natural wood for natural materials, and white or black laminate for artificial materials). Variations in light were represented by either the absence or presence of a large window in the room. We note that the natural light conditions included a window with a view of nature, as shown in Figure 2. For parsimony, we refer to this condition as natural light, but we are aware that the presence of a window could be a confounding factor impacting wellbeing outcomes. Variations in representations were shown with framed photographs, displaying either only white men (non-diverse), or more mixed gender and racial identifications (diverse). To enhance visibility of the representations in the wall photographs, these images were shown both in the room photograph and separately, up close. Figure 3 shows two examples of a participant's view during the online experiment and Figure 4 shows the non-diverse and diverse photographs used. The same eight questions for our measures from Studies 1–3 were used for Studies 4 and 5 with an additional qualitative question added at the end asking respondents what stood out between the different conditions they saw, in order to evaluate the efficacy of our manipulations in these new photographs.
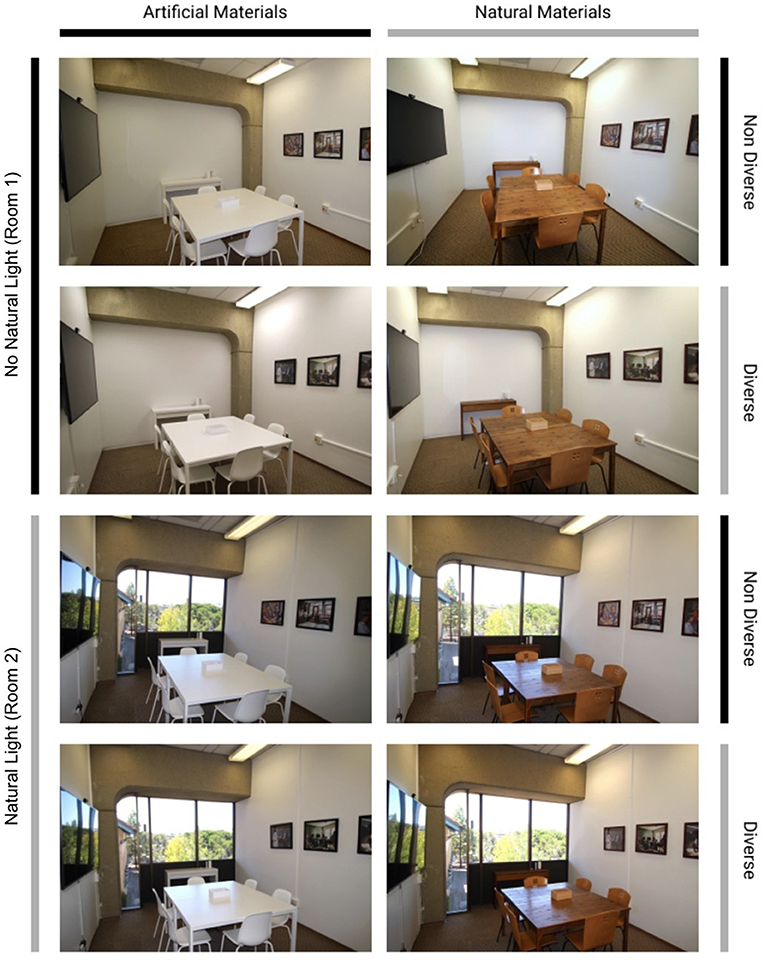
Figure 2. Pictures of the two laboratory rooms located on the lead authors' university campus that were used in studies 4 and 5.
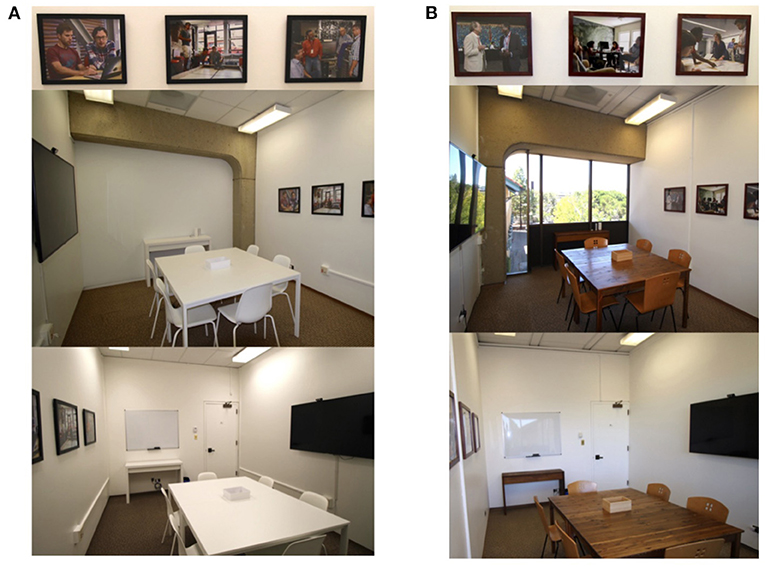
Figure 3. Comparison of participants' view for two of the eight laboratory room conditions: (A) artificial materials, artificial light and non-diverse representation, and (B) natural materials, natural light and diverse representation.
Studies 4 and 5 were conducted using the online platform Prolific in an effort to reach a more attentive, and naive pool of participants (Peer et al., 2021). Exclusions were based on the same criteria as for Survey 3, excluding participants who failed more than one out of three attention checks for Study 4, or the one attention check for Study 5 (because of between-subjects design), or who provided unnatural, patterned answers (refer to Table 1 for exclusion data).
Within-Subjects Design (Study 4a)
Study 4a was a within-subject design wherein each participant was shown all eight study conditions in a randomized order and asked to answer our eight questions after each set of photographs. Cronbach's alpha ranges for this survey are reported in Table 3.
Between-Subjects Design (Study 4b and Study 5)
A concern regarding survey fatigue arose when designing Study 4a, as we speculated that the repetition of our measurements eight times (as opposed to six times in Studies 1–3), in conditions with similar room pictures, could lead to inattentive participants, increasing the chances of survey satisficing. To assess whether this issue impacted our results, we began by analyzing data from Study 4a using a between-subject approach, which we refer to as Study 4b. A between-subjects design refers to studies where participants only see one condition, randomly assigned. For Study 4b, we therefore extracted survey data for only the first room that each participant saw in Study 4a (maintaining the same exclusions from Study 4a), and repeated the analyses. The number of participants who had seen each condition as their first room ranged from 45 respondents in the condition least often seen first (artificial materials, natural light, non-diverse representation), to 69 participants in the most common condition seen first (artificial materials, no natural light, diverse representation). Cronbach's alpha values for Study 4b are also reported in Table 3.
Our final study, Study 5 was an intentional between-subjects experiment with the same photograph sets from Study 4 where only one condition was shown to each participant. As this study was designed with the purpose of being a between-subjects survey (unlike Study 4b), the number of participants per condition after exclusions was more balanced than for Study 4b. The two least seen rooms (having natural materials, no natural light, and diverse representation and having natural materials, natural light and non-diverse representation) had 56 participants each, and the two most viewed rooms (having artificial materials, no natural light, diverse representation and having natural materials, no natural light, non-diverse representation) had 60 participants each.
For this between-subject experiment, Cronbach's alpha values ranges are given in Table 3. We confirmed with our statistical analysis that the low Cronbach's alpha for self-efficacy scores in studies 4b and 5 did not affect the validity of our results as noted in Table 3.
Results
Analysis
Individual Studies
Data was analyzed using analysis of variance (ANOVA) to compute the main effects of our different intervention treatments. For within-subject designs (Studies 1, 2, 3, and 4a), we used repeated measures ANOVA to account for participants seeing all the conditions. For the factorial design experiments (Studies 4a, 4b, and 5), we conducted three-way ANOVA to integrate the interaction effects between our independent variables. In order to control for the influence of covariates on our results, we also conducted analysis of covariance (ANCOVA) on all study datasets. The covariates were gender identification (man or woman), racial identification (white or non-white), and highest level of education achieved (above or below a bachelor's degree or equivalent) based on findings from prior research (Steiner et al., 2010). We then used these covariates to conduct mixed-model ANOVA analyses for all the studies to identify significant interactions between the covariates and each one of our independent variables. As per standard practice, respondents with non-binary gender identifications, or those who had answered “Prefer Not to Say,” were excluded from the ANCOVA and mixed ANOVA analyses. All of our statistical models were carried out using the R-4.0.2 computing environment. To compute the sense of belonging, self-efficacy, and environmental efficacy scores, we generated the mean score for the three items for belonging, the two for self-efficacy, and the three for environmental efficacy, respectively.
Meta-Analysis
We ran a meta-analysis on the five studies (excluding Study 4b) to synthesize the effect of each one of the three independent variables (materials, light, and representation) on our three dependent variables (belonging, self-efficacy, and environmental-efficacy). Study 4b was not included in the meta-analysis as it was not an independent study and the data for that study was taken from Study 4a. We combined all within-subjects studies (Studies 1–3 and 4a) and the between-subject study (Study 5) for the meta-analysis. The decision to include all studies in the same meta-analysis was based on the argument presented by Morris and DeShon (2002) that it is acceptable to combine within-subjects and between-subjects study designs when (a) a common metric is used for the effect sizes across within-subjects and between-subjects study designs, (b) the same treatment effect is estimated by effect sizes in different study designs1, and (c) sampling variance estimates used for the meta analysis are design-specific.
The common effect size metric used for all studies was change-score metric, as four of the studies had repeated measures design. The raw-score effect size for between-subjects study (Study 5) was therefore converted to change-score metric. The effect size estimates were computed using t-statistics. The sampling variances were calculated accounting for the effect size metric as well as the study design used. The meta-analysis was carried out using R version 4.0.2, with a random-effects model and restricted maximum likelihood for the estimation of variance-weighted mean effect sizes. A moderation analysis was also performed to test if the study design (within-subjects vs. between-subjects) was acting as a moderator of the effect size to ensure that the effect sizes did not differ significantly across the different study designs used, after they were transformed into a single effect size metric (i.e., change-score metric).
Results Per Independent Variable
Our results are organized for each independent variable with subsections for each dependent variable. The ANOVA results are reported using p-value (p), F ratio (F), degrees of freedom (df) and effect size (η2). Significant thresholds for p-value are < 0.05 (*), < 0.01 (**) and < 0.001 (***). Unless otherwise specified in the text, our results held after controlling for covariates with the ANCOVA model. Table 1 in the Supplementary Materials submission provides the detailed results from analysis of covariance. Table 2 in the Supplementary Materials submission provides additional statistical details from analysis of variance with interaction effects.
Materials
Table 4 presents the main effects obtained with ANOVA, as well as significant interactions for materials. The interactions reported in the tables are those produced by the mixed ANOVA, whereas the text sections discuss the post-hoc analysis results detailing the directions of the significant interactions. Table 5 shows the mean scores, standard deviations, and 95% confidence intervals for natural and artificial materials. Possible scores ranged from 1 (“Strongly Disagree”) to 7 (“Strongly Agree”).
Sense of Belonging
Participants' self-reported sense of belonging scores were higher for natural materials across Study 1, Study 2, and Study 3, with both our ANOVA models showing significant results, as shown in Table 4. In Study 1, participants who identified as white women reported particularly higher belonging scores in the natural materials condition (M = 5.462, SD = 1.227) over its artificial counterpart (M = 4.059, SD = 1.625, p < 0.001). An interaction with participants' race was also observed, leading white people to express a strong preference for natural materials (M = 5.258, SD = 1.367) over artificial materials (M = 4.291, SD = 1.580, p < 0.001).
Data from Study 2 revealed that white women again reported stronger feelings of belonging with natural materials (M = 5.529, SD = 0.988) compared to artificial materials (M = 4.954, SD = 1.437, p < 0.001). Participants who reported having achieved less than a bachelor's degree also scored higher with natural materials (M = 5.140, SD = 1.306) over artificial materials (M = 4.473, SD = 1.432, p = 0.008). Additionally, Study 2 showed that white women who reported having achieved less than a bachelor's degree scored higher with natural materials (M = 5.381, SD = 1.02) over artificial materials (M = 3.714, SD = 1.709, p = 0.006). There was a similar result for white identifying women with bachelors or more reporting higher belonging scores in the natural materials condition (M = 5.561, SD = 0.986) compared to the artificial materials condition (M = 5.217, SD = 1.234, p = 0.028).
In Study 3, participants who had indicated having achieved less than a bachelor's degree were driving the main effect by showing a strong preference (p < 0.001) for natural materials (M = 5.08, SD = 1.33) over artificial materials (M = 4.36, SD = 1.59).
Analyses for Study 4a, Study 4b, and Study 5 revealed that, although participants' self-reported belonging scores were higher for natural materials than artificial materials, this difference was not statistically significant. A single significant interaction was found in Study 4a between race and materials, with white people self-reporting higher belonging scores with natural materials (M = 4.434, SD = 1.540) than artificial materials (M = 4.29, SD = 1.61, p < 0.001), while people of color preferred the artificial materials condition (M = 4.375, SD = 1.582) over natural materials (M = 4.26, SD = 1.58, p = 0.031).
Self-Efficacy
Participants' self-reported self-efficacy scores were statistically higher for the natural materials scenario compared to artificial materials in Study 1, with p-values below 0.001. However, in Study 2, although our ANOVA model produced significant results, this significance was not maintained when controlling for covariates, and the gender of participants was identified as the moderating covariate leading to null ANCOVA results. Study 3 returned significant results even after controlling for covariates with, once more, a preference for natural materials.
In Study 1, white women were driving the main effect by scoring significantly higher for natural materials (M = 5.441, SD = 1.066) than artificial ones (M = 4.401, SD = 1.321, p < 0.001).
In Study 2, white women once more expressed more self-efficacy with natural materials (M = 5.538, SD = 1.158) than they did with artificial materials (M = 4.963, SD = 1.321, p < 0.001). Also, in Study 2, women expressed higher self-efficacy scores in natural materials condition (M = 5.583, SD = 1.105) than they did with artificial materials (M = 5.117, SD = 1.282, p < 0.001). Further, Study 2 showed that people who associated as white expressed higher self-efficacy scores in the natural materials condition (M = 5.217, SD = 1.277) than they did with artificial materials (M = 5.079, SD = 1.172, p = 0.013). There was also a significant race by education by materials interaction where people who associated as whites and had less than a bachelor's degree expressed higher self-efficacy scores in the natural materials condition (M = 5.375, SD = 1.091) than they did with artificial materials (M = 4.528, SD = 1.270, p = 0.005). A gender by race by education by materials significant interaction was found where white women with less than (p = 0.004) as well as more than (p = 0.010) a bachelor's degrees reported higher levels of self-efficacy in the natural materials condition (M = 5.786, SD = 0.893) and (M = 5.484, SD = 1.206) compared to the artificial materials condition (M = 4.036, SD = 1.562) and (M = 5.159, SD = 1.187).
Data from Study 3 revealed that participants with an education level below the bachelor's degree were driving the main effect by showing a strong preference (p < 0.001) for natural materials (M = 5.14, SD = 1.31) over artificial materials (M = 4.54, SD = 1.45).
The results from Study 4a, Study 4b, and Study 5 showed no significant main effect for materials and self-efficacy (Table 4), despite natural materials leading to higher self-efficacy scores than artificial ones (Table 5). One statistically significant interaction was identified during Study 4a between race and materials, with white-identifying participants reporting higher self-efficacy in the natural materials setting (M = 4.58, SD = 1.33) over the artificial materials condition (M = 4.51, SD = 1.38, p = 0.025), and people of color in the artificial materials condition (M = 4.46, SD = 1.35) over natural materials (M = 4.36, SD = 1.37, p = 0.033).
Environmental Efficacy
Environmental efficacy results for Study 1, 2, and 3 showed a statistically significant difference between the effect of natural and artificial materials, with natural materials leading to higher self-reported scores.
In terms of significant interactions, participants from Study 1 who identified as white women reported higher levels of environmental efficacy when shown pictures of natural materials (M = 5.937, SD = 1.207) over artificial materials (M = 5.182, SD = 1.478, p < 0.001). White men also expressed higher environmental efficacy in the natural material condition (M = 5.633, SD = 1.428) over the artificial one (M = 5.408, SD = 1.369, p = 0.043). Similarly, men of color reported higher environmental efficacy in the natural material condition (M = 5.657, SD = 1.560) over the artificial one (M = 4.899, SD = 1.452, p = 0.007).
Similarly, for Study 2, higher levels of environmental efficacy in the natural materials condition were reported by both women (M = 5.807, SD = 1.099) and men (M = 5.589, SD = 1.199) over the artificial materials condition (M = 5.444, SD = 1.225) and (M = 5.435,SD = 1.224, p < 0.001) and (p = 0.024). Additionally, for Study 2, people of color with a bachelor's degree or more reported higher levels of environmental efficacy in the natural materials condition (M = 5.861, SD = 1.129) over the artificial materials condition (M = 5.592, SD = 1.257, p = 0.006). Finally, people who self-identified as white and had less than a bachelor's degree also reported higher levels of environmental efficacy in natural materials condition (M = 5.778, SD = 1.247) over the artificial materials condition (M = 5.111, SD = 1.168, p < 0.001).
An interaction between gender and race was observed in Study 3 with both men of color (p = 0.006) and white women (p = 0.005) preferring natural materials (Men of color: M = 5.75, SD = 0.98; White women: M = 5.65, SD = 1.13) over artificial materials (Men of color: M = 5.42, SD = 1.22; White women: M = 5.37, SD = 1.36).
The data from Study 4a, Study 4b, and Study 5 showed no significant difference in self-reported environmental efficacy between natural and artificial materials. While the ANOVA models reported null results, the mixed ANOVA analyses showed a significant interaction between gender and race for Study 4b only, with men of color reporting higher environmental efficacy scores with natural materials (M = 5.80, SD = 0.97) over artificial materials (M = 5.11, SD = 1.29) [p = 0.01, t(71) = 2.65, CI = (0.17, 1.22)].
Light
Table 6 shows the main effects and significant interactions obtained for light type. The interactions in the tables are those revealed by the mixed ANOVA, whereas the text discusses the post-hoc results and details the directions of the interactions. Table 7 shows the mean scores, standard deviations, and 95% confidence intervals for natural and artificial light. Possible scores ranged from 1 (“Strongly Disagree”) to 7 (“Strongly Agree”).
Sense of Belonging
The data from Study 1 and Study 3 led to significant results for belonging and light, with participants self-reporting higher scores in the natural light settings than the settings with no natural light. However, these results were not replicated in Study 2.
Data from Study 1 revealed a significant interaction with participants' gender, with women preferring presence (M = 5.265, SD = 1.317) over absence of natural light (M = 4.392, SD = 1.551, p < 0.001). Study 2 showed that people with less than a bachelor's degree self-reported higher levels of belonging in the natural light condition (M = 5.073, SD = 1.439) over the no natural light condition (M = 4.607, SD = 1.225, p = 0.042). We also found, for Study 2, that white women reported higher levels of belonging in the natural light condition (M = 5.504, SD = 1.156) over the condition without natural light (M = 5.113, SD = 1.289, p = 0.018).
Upon running a mixed ANOVA model on Study 3, it appeared that participants not having achieved a bachelor's degree preferred natural light (M = 4.97, SD = 1.52) over absence of natural light (M = 4.37, SD = 1.51, p < 0.001). More specifically, women with less than a bachelor's degree showed strong preference for natural light (M = 5.36, SD = 1.52) over no natural light (M = 4.26, SD = 1.54, p < 0.001).
Data from Study 4a and Study 5 revealed that participants' self-reported belonging scores were significantly higher for natural light even after controlling for covariates. However, Study 4b, which analyzed only the first condition that participants had seen in Study 4a, revealed null results. One interaction between education level and race was observed when running a mixed ANOVA on Study 4b, but our post-hoc analysis did not confirm the significance of this observation, p = 0.129.
Self-Efficacy
Participants' self-efficacy scores in Study 1 showed a statistically significant difference between natural and no natural light, with the natural light setting resulting in higher reported self-efficacy. While Survey 2 returned null results for the main effect, Study 3 showed a statistically significant preference for natural materials, but null results after controlling for covariates. A moderation analysis for Study 3 revealed that participants' education level was acting as moderator.
Women participating in Study 1 reported higher levels of self-efficacy when presented with pictures of natural light (M = 5.199, SD = 1.205) over no natural light (M = 4.567, SD = 1.364, p < 0.001). Similarly, Study 2 also showed a trend with women reporting higher self-efficacy scores in the natural light condition (M = 5.248, SD = 1.109) compared to the no natural light condition (M = 5.107, SD = 1.242). However, no significance was found in the post-hoc analysis, p = 0.271. We also found in Study 2 that people with less than a bachelor's degree reported higher levels of self-efficacy when presented with pictures of natural light (M = 5.220, SD = 1.221) over absence of natural light (M = 4.810, SD = 1.293, p = 0.046). More particularly, white women with less than a bachelor's degree reported higher levels of self-efficacy when presented with pictures of natural light (M = 5.50, SD = 1.345) over no natural light (M = 4.179, SD = 1.552, p = 0.017). Men of color with a bachelor's degree or more reported higher levels of self-efficacy when presented with pictures of natural light (M = 5.658, SD = 0.979) over no natural light (M = 5.870, SD = 0.890, p = 0.037).
An interaction during Study 3 was observed with participants' education level, leading people who had achieved less than a bachelor's degree to score higher with natural light (M = 4.99, SD = 1.30) than without natural light (M = 4.56, SD = 1.34, p < 0.001). An interaction with gender was also returned by our mixed ANOVA model, with women preferring natural light (M = 5.03, SD = 1.32) over absence of natural light (M = 4.74, SD = 1.36, p = 004).
The data from Study 4a and Study 5 showed that participants' self-efficacy scores increased significantly in the natural light setting, while null results were obtained for Study 4b. A mixed ANOVA model conducted on Study 4b identified an interaction between participants' education level and race, although this was not confirmed by post-hoc tests, p = 0.210. However, Study 5 revealed that men of color preferred natural light to be present (M = 4.81, SD = 1.28) rather than absent (M = 4.10, SD = 1.24, p = 0.03).
Environmental Efficacy
Environmental efficacy scores reported by participants from Study 1 revealed a statistically significant difference in scores, with natural light leading to a higher sense of environmental efficacy. Significant results in the same direction were also obtained for Study 4a. However, all other studies returned null main effects. Moreover, no significant interaction effects were highlighted between light and participants' covariates at any point during our studies of the environmental efficacy scores.
Representation
The main effects and significant interactions obtained for representation type are given in Table 8 below. The interactions in the tables were obtained with a mixed ANOVA model, and the text details the post-hoc results as well as the directions of these interactions. Table 9 shows the mean scores, standard deviations, and 95% confidence intervals for diverse and non-diverse representation. Self-reported scores ranged from 1 (“Strongly Disagree”) to 7 (“Strongly Agree”).
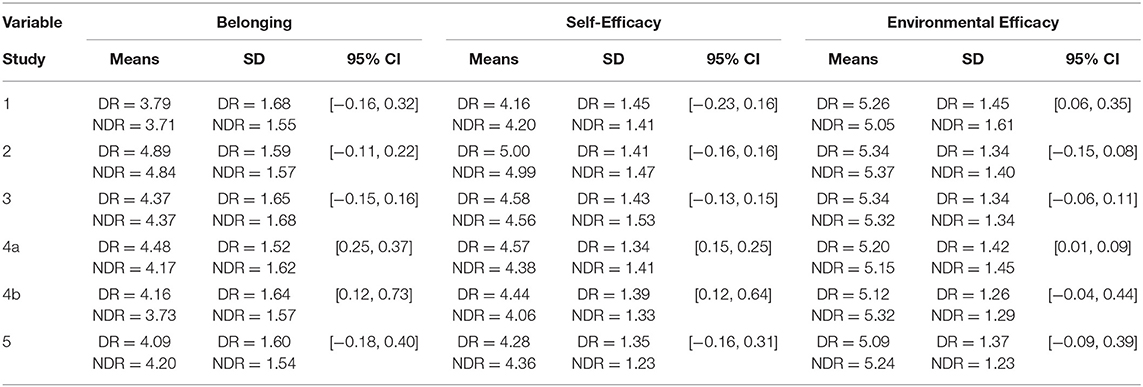
Table 9. Mean, standard deviation, and 95% CI scores obtained for representation for all the studies.
Sense of Belonging
Self-reported belonging scores for Studies 1, 2 and 3 showed no statistically significant difference between the impact of diverse and non-diverse representation. In Study 1, women expressed strong preference for diverse representation (M = 4.269, SD = 1.70) over non-diverse (M = 3.589, SD = 1.563, p < 0.001), whereas men reported stronger feelings of belonging with non-diverse representation (M = 3.842, SD = 1.527) instead of diverse (M = 3.280, SD = 1.496, p < 0.001).
Similarly, post-hoc tests confirmed that women participants from Study 2 reported higher belonging scores in the diverse representation (M = 5.136, SD = 1.464) than the non-diverse setting (M = 4.531, SD = 1.692, p < 0.001), whereas men expressed more belonging in the non-diverse setting (M = 5.009, SD = 1.475) than they did in the diverse setting (M = 4.755, SD = 1.644, p = 0.006). People with less than a bachelor's degree showed a trend with higher belonging scores in diverse representation (M = 4.10, SD = 1.750) vs. non-diverse representation settings (M = 3.780, SD = 1.604). However, no significance was found in the post-hoc breakdown, p = 0.227. Additionally, in Study 2, women with less than a bachelor's degree reported higher levels of belonging in diverse representation (M = 4.90, SD = 1.697) than non-diverse representation settings (M = 3.183, SD = 1.543, p < 0.001). Similarly, men with less than a bachelor's degree reported higher levels of belonging in diverse representation (M = 3.567, SD = 1.598) than non-diverse representation settings (M = 4.178, SD = 1.543, p = 0.020).
Although our mixed ANOVA model for Study 3 returned a significant interaction with participants' racial identification, upon completing a post-hoc analysis, this result was revealed to be not statistically significant, p = 0.079. However, pairwise comparisons confirmed the validity of the interaction with participants' gender observed during the mixed ANOVA. Our post-hoc test showed once again that men preferred non-diverse representations (M = 4.55, SD = 1.62) over diverse representations (M = 4.26, SD = 1.67, p = 0.001), whereas women preferred the diverse condition (M = 4.58, SD = 1.60) over its non-diverse counterpart (M = 4.03, SD = 1.74, p < 0.001).
Study 4a and Study 4b revealed significantly higher belonging scores in the diverse condition. In Study 4a, women significantly preferred diverse (M = 4.32, SD = 1.52) over non-diverse (M = 3.87, SD = 1.62) representation (p < 0.001). In that same study, participants of color expressed strong preference (p < 0.001) for diverse representation (M = 4.53, SD = 1.49) over non-diverse representation (M = 4.10, SD = 1.64). Similarly, in Study 4b, participants who identified as women were driving the main effect by scoring significantly higher (p = 0.004) in the diverse setting (M = 3.92, SD = 1.63) than in the non-diverse setting (M = 3.30, SD = 1.55).
Finally, Study 5 revealed that participants' self-reported scores were higher in the non-diverse condition, but our statistical models did not return any significant difference between the two conditions, and no interactions were found.
Self-Efficacy
As with belonging, self-efficacy scores did not significantly vary with representation types for either Studies 1, 2, or 3. However, a significant interaction with participants' gender was identified during our mixed ANOVA analysis for Study 1. A post-hoc test confirmed this interaction and showed that, while women preferred diverse representation (M = 4.397, SD = 1.458) over non-diverse (M = 4.067, SD = 1.448, p = 0.015), men had a different preference, and expressed stronger self-efficacy with non-diverse representation (M = 4.336, SD = 1.352) than diverse (M = 3.905, SD = 1.392, p = 0.002).
An interaction with participants' gender was again identified in Study 2. Upon post-hoc analysis, a significant preference for diverse representation (M = 5.112, SD = 1.312) over non-diverse (M = 4.689, SD = 1.666) was found for women participants (p = 0.008), whereas participants who identified as men reported higher scores in the non-diverse setting (M = 5.166, SD = 1.330) than they did in the diverse one (M = 4.932, SD = 1.467, p = 0.007). We also found that women with less than a bachelor's degree reported higher levels of self-efficacy in the diverse representation condition (M = 4.825, SD = 1.558) compared to non-diverse representation condition (M = 3.65,SD = 1.850, p = 0.007). On the contrary, men with less than a bachelor's degree reported higher self-efficacy scores in the non-diverse condition (M = 4.60, SD = 1.329) compared to the diverse condition (M = 3.917, SD = 1.474, p = 0.011).
As with previous studies, data from Study 3 revealed that men preferred non-diverse representation (M = 4.73, SD = 1.46) over diverse representation (M = 4.48, SD = 1.45, p = 0.003), whereas women preferred diverse representation (M = 4.75, SD = 1.40) over non-diverse representation (M = 4.25, SD = 1.62, p < 0.001).
Study 4a and Study 4b showed significant results for the main effect, with diverse representation leading to higher self-efficacy. Additionally, Study 4a revealed a significant interaction with participants' race [p < 0.001, t(567) = 5.91, CI = (0.21, 0.42)], leading people of color to drive the main effect observed in favor of diverse representation (M = 4.57, SD = 1.30) over non-diverse representation (M = 4.25, SD = 1.41). However, all models for Study 5 returned null results, with participants scoring higher in the non-diverse condition.
Environmental Efficacy
Participants' self-reported environmental efficacy scores were significantly higher in the diverse condition compared to the non-diverse condition in Study 1. However, after controlling for covariates, the difference in the mean of the two groups was no longer statistically significant, although no moderating covariate was identified. Studies 2 and 3 showed no main effect.
In Study 1, an interaction with race was identified, with people of color significantly preferring the diverse condition (M = 4.922, SD = 1.584) over the non-diverse condition (M = 4.397, SD = 1.762, p = 0.005). Women of color with a bachelor's degrees or more reported higher levels of environmental efficacy in the diverse representation condition (M = 5.693, SD = 1.154) compared to the non-diverse representation condition (M = 4.507, SD = 1.738, p = 0.003).
For Study 2, people with less than bachelors degree had a trend for higher environmental efficacy scores in the diverse condition (M = 5.053, SD = 1.544) relative to the non-diverse condition (M = 4.793, SD = 1.706). However, no significance was found in the post-hoc breakdown, p = 0.057.
As observed in Studies 1 and 2, Study 3 revealed that participants who identified as women preferred diverse representation (M = 5.38, SD = 1.37) over non-diverse representation (M = 5.22, SD = 1.40, p = 0.02).
Study 4a revealed significantly higher scores for diverse representation, but this result was not maintained after controlling for covariates, with no moderator found. On the other hand, both the ANOVA and ANCOVA analyses conducted for Study 4b returned null results, and no significant interactions were observed.
Finally, Study 5 showed null main effects, but the non-diverse condition seemed to be preferred by participants. Furthermore, a mixed ANOVA revealed an interaction between gender and race, with men of color reporting higher environmental efficacy with non-diverse representation (M = 5.46, SD = 1.21) over the diverse condition (M = 4.70, SD = 1.66, p = 0.019, t(66) = −2.40, CI = (−1.39, −0.13)].
Interactions Between Independent Variables
Prior literature has demonstrated the combined impact of built features on wellbeing outcomes. For example, the interactions between different lighting conditions and different types of natural wood materials were studied with reference to their impact on participants' appreciation of the space, visual comfort, and wellbeing (Poirier et al., 2019). We, therefore, expected seeing interactions between our independent variables of interest and analyzed them for Studies 4a onwards.
In Study 4a, a significant interaction between material type and light type was found (p = 0.03, F = 4.757, ges ≤ 0.001). No main effect was obtained for materials in this study, but our interaction analysis revealed that natural materials (M = 4.10, SD = 1.59) led to significantly higher belonging scores than artificial materials (M = 3.98, SD = 1.63) in the conditions with no natural light [p = 0.004, t(875) = −2.892, CI = (0.04, 0.20)].
A significant interaction between material and light type was observed with survey Study 4b as well (p = 0.037, F = 4.400, ges = 0.010). Once again, although no main effect was obtained for materials in this study, our multiway ANOVA showed that natural materials (M = 4.11, SD = 1.58) lead to higher sense of belonging than artificial materials (M = 3.60, SD = 1.72) in the conditions with no natural light [p = 0.021, t(215) = −2.33, CI = (0.08, 0.95)]. Additionally, despite no main effect observed for light, this interaction revealed that natural light (M = 4.17, SD = 1.58) promoted a sense of belonging over no natural light (M = 3.60, SD = 1.72) in the artificial materials settings specifically [p = 0.009, t(227) = −2.64, CI = (0.10, 0.14)].
Finally, Study 4b also revealed a significant interaction between light type and representation type (p = 0.017, F = 5.746, ges = 0.013), leading participants to report significantly higher environmental efficacy scores for non-diverse (M = 5.46, SD = 1.20) than diverse (M = 4.96, SD = 1.34) representation when they were in the natural light conditions (p < 0.001, t(204) = 2.80, CI = (0.15, 0.84)].
Interactions between several dependent and independent variables acting simultaneously together were also observed for these studies when running our mixed ANOVA model. Although some of these were confirmed following post-hoc tests, no boundary cases or repetitions across more than one study were observed, hence these interactions were considered sample-specific artifacts.
Meta Analysis
The results of the meta-analysis are summarized in Table 10. The explanation of the results is broken down by the independent variables of interest, i.e., materials, light, and representation.
Materials
The meta-analysis reveals that all the studies show a similar effect of natural vs. artificial materials on the sense of belonging, with the Q-test yielding a non-significant chi-square result2, Q = 1.175, p = 0.882. Being in the natural materials condition led to greater sense of belonging than artificial materials, d = 0.214, p = 0.032, 95% confidence interval (CI) = [0.019, 0.410]. The meta-analysis also shows that the effects of natural materials vs. artificial materials on self-efficacy scores were homogenous across the five studies, Q = 1.595, p = 0.810. Being in the natural materials condition led to higher self-efficacy scores than artificial materials, d = 0.172, p = 0.037, 95% confidence interval (CI) = [0.011, 0.332]. Similarly, the effects of natural materials vs. artificial materials on environmental efficacy were consistent across the five studies, Q = 2.128, p = 0.712. Like belonging and self-efficacy, being in the natural materials condition also led to higher environmental efficacy scores than artificial materials, d = 0.182, p = 0.033, 95% confidence interval (CI) = [0.015, 0.349].
Light
The meta-analysis shows that the effects of natural light vs. no natural light on the sense of belonging were homogenous across the five studies, Q = 3.583, p = 0.465. Being in the natural light condition led to greater sense of belonging than in the condition without natural light, d = 0.354, p = 0.028, 95% confidence interval (CI) = [0.039, 0.669]. The meta-analysis also confirms that all studies show the same effect of natural light vs. absence of natural light on self-efficacy, Q = 4.918, p = 0.296. Being in the natural light condition led to marginally higher self-efficacy scores than no natural light, d = 0.263, p = 0.051, 95% confidence interval (CI) = [0.001, 0.526]. Additionally, the effects of absence vs. presence of natural light were also consistent on environmental efficacy scores, Q = 3.776, p = 0.437. Being in the natural light condition led to significantly higher environmental efficacy scores, d = 0.194, p = 0.029, 95% confidence interval (CI) = [0.020, 0.368].
Representation
The meta-analysis shows that the effects of diverse representation vs. non-diverse representation on sense of belonging were not consistent across the five studies, Q = 8.496, p = 0.075. Being in the diverse representation condition did not lead to greater sense of belonging than non-diverse representation, d = 0.129, p = 0.161. The meta-analysis also shows that the effects of diverse representation vs. non-diverse representation on self-efficacy scores were not homogenous across the studies tested, Q = 9.006, p = 0.061. Being in the diverse representation condition did not lead to greater self-efficacy scores than non-diverse representation, d = 0.095, p = 0.201. Additionally, the effects of diverse representation vs. non-diverse representation on environmental efficacy score were also not the same across the five studies, Q = 1.220, p = 0.875. Being in the diverse representation condition did not improve environmental efficacy scores compared to non-diverse representation, d = 0.097, p = 0.086.
Moderation Analysis
The moderation analysis indicated that the study design (within-subjects vs. between-subjects) did not act as a significant moderator of the effect sizes in any of the meta-analyses. Hence, transforming all effect sizes into a single metric is an acceptable way of combining these studies into a single meta-analysis to estimate the overall effect of independent variables on dependent variables.
Discussion
Despite changes in survey design and analysis methods across all six studies, our meta-analysis reveals homogeneous effects of both material and light interventions on our three dependent variables. In the next section, we examine several parameters that varied across our different online surveys that could have impacted our results.
Seasonality
As highlighted in Table 1, Studies 1 and 2 were conducted during the winter months (January, February), whereas all other studies were conducted in the summer (June, July). As participants were recruited across the USA only, it can be assumed that a large proportion of our subjects were exposed to significantly different climates between the summer and the winter surveys. Across different seasons, people spend different amounts of time indoors and outdoors, which can affect how they respond to reflecting on different indoor spaces. Additionally, seasonal phenomena such as seasonal affective disorder or circannual cycles have shown that changes in seasons can affect human biology (Rosen et al., 1990).
In Study 1, during the winter, we found that natural materials and natural light led to improved sense of belonging, self-efficacy, and environmental efficacy. When we replicated this study in the summer (Study 2), significant results were obtained for natural materials only across all dependent variables, while our light variable returned null results across all dependent variables. One potential explanation is that, in the summer months, our participants might have been less sensitive to access to natural light due to extended access to daylight during longer summer days. However, the data from Study 3 did not lead to results similar to those obtained with Study 1, although both were conducted in the winter. In Study 3 we were able to reproduce significant results for natural materials across all dependent variables, whereas natural light only led to statistically significant results for the sense of belonging construct.
The impact of seasonality on our results therefore appears to be complex, and is likely mediated by other factors that varied across our studies. For example, although Study 1 and Study 3 were both conducted in the winter and on the MTurk platform, the 3 year gap between the surveys had led to significant changes in the demographics of participants (see Table 2). Since our results highlighted many interactions between the influence of our independent variables and demographic variables (participants' gender, race, and education level), changes in demographics could have potentially contributed to significant variations in our results.
Additionally, Study 1 was conducted before the COVID-19 outbreak in early 2020, which resulted in significant behavioral changes in the USA and worldwide, with many people spending more time indoors. Despite differences in results between individual studies, which could potentially be attributed to effects of seasonality and/or COVID-19, our synthesized results from the meta-analysis show that the positive impacts of natural materials and natural light on all three dependent variables of belonging, self-efficacy, and environmental efficacy hold consistent overall across all five studies.
Dosage
As our studies were carried out with two different sets of pictures, the level of exposure to each independent variable, referred to here as “dosage,” changed. Studies 1, 2, and 3 were conducted using the same set of photographs taken at different locations on the university campus (Figure 1), whereas the Studies 4 and 5 displayed different photographs taken during a controlled laboratory study involving two rooms, in which the variations in material, light, and representation types were more limited. Comparing the results obtained with different pictures can show the impact of different dosages of our independent variable manipulations that the participants received.
Natural materials appeared to consistently lead to higher scores for all 3 constructs of belonging, self-efficacy and environmental efficacy across Studies 1, 2, and 3. When we lowered the dosage for the subsequent studies by using pictures taken in the lab, null main effects were systematically obtained for materials. However, as the dosage changed, new significant results appeared for light and representation.
As shown in Figure 4, our photographs in Studies 4 and 5 included a close-up of the representation pictures that were framed on the laboratory's walls, to ensure that participants were able to see the content of these photographs properly. Additionally, the contrast between presence and absence of natural light was clearer in our lab set of pictures, as participants were shown very similar rooms, with either a large window on the back wall, or a simple white wall. Participants' qualitative answers confirmed that this difference was notable, with more than half of respondents mentioning light when asked what built features stood out for them (some recurring words in responses were “window,” “light,” “lighting,” “outside,” and “glass”). On the other hand, no close-up image of the table was provided, which could have drawn more attention to the materials texture and highlighted the difference between artificial and natural material manipulations. Additionally, in the second set of pictures, the changes were seen through furniture changes only, and did not affect the materiality of the rooms themselves. This limited change could also have been affecting participants' responses. Finally, it is important to remember that the photographs used for the first picture set were taken in non-controlled environments, meaning external factors could also have changed between different conditions. For instance, pictures for the natural materials condition were overall more colorful than those used for the artificial materials. This is another difference between the two sets of pictures that has the potential of leading to inconsistent results.
For representation, the picture sets from Studies 1–3 (Figure 1) included photographs of influential leaders that were displayed around campus and also contained statues, sculptures and drawings. On the other hand, the photographs for Studies 4a, 4b, and 5 (Figure 4) only included pictures of humans. It can be speculated that participants are likely to react more substantially to pictures of people than they are to pictures of inanimate objects such as statues.
Additionally, the diverse representations displayed in Studies 1–3 included only one man. Interaction analysis for these surveys showed that, while women reported higher scores in all dependent variables in the diverse condition, men reported higher levels of belonging and self-efficacy in the non-diverse setting. After rebalancing the inclusion of different genders in the second set of pictures, both men and women reported higher levels of belonging, self-efficacy, and environmental efficacy in the diverse conditions in Study 4a and Study 4b. Study 5 revealed that non-diverse representations led to higher self-reported scores across all dependent variables, despite using the same pictures as those used in Study 4a and Study 4b, and being conducted on the same platform, during the same season. However, this result was not statistically significant, and was therefore considered to be specific to our survey sample.
Independent Conditions vs. Factorial Design
Interactions between our independent variables of interest were another aspect of our surveys' design that varied across studies. For all studies carried out using the picture sets from Figure 1 (pictures taken on the lead authors' university campus), the interactions between our independent variables were balanced out as much as possible in each set, so that each condition was independent. However, since the conditions were not controlled, we cannot exclude that interaction effects took place and affected the dependent variables.
When using the picture sets from Figure 2 (pictures taken in the lab), interactions between all three variables in each condition were controlled and accounted for in the analyses. Our multiway ANOVA model for Study 4a revealed an interaction between material type and light type for the belonging construct, and this finding was confirmed by a pairwise test. The test revealed that, although material type had no significant main effect for this study, natural materials were significantly preferred by our respondents in the settings with no natural light. This interaction was confirmed by Study 4b, which highlighted another significant interaction. In this study, no main effect for light type was observed. However, our analysis showed that natural light led to higher self-reported sense of belonging than absence of natural light when the participants were in an artificial materials setting.
It appears that our selected interventions can act together to promote well-being in certain combinations. Accounting for these interactions in survey design and analysis can therefore enhance insights into how physical design features impact well-being.
Within-Subjects and Between-Subjects Approaches
Unlike Studies 1–3, which were strictly treated as within-subject studies, Study 4 was analyzed using both a within-subjects approach (Study 4a) and a between-subjects approach (Study 4b). We found that, although natural light seemed to have a significant impact on heightened sense of belonging, self-efficacy, and environmental efficacy for the within-subject approach (Study 4a), those results were not seen when running a between-subjects analysis for that same study (Study 4b). On the other hand, the significant results obtained for diverse representations in our within-subjects analysis of Study 4a were maintained for the between-subject approach in Study 4b, for belonging and self-efficacy. We therefore hypothesize that the effect of natural light was emphasized when put in a comparative context (no naturall vs. natural lighting) in the within-subjects study design, whereas the impact of diverse or non-diverse representations was strong enough to be significant even when participants had only seen one condition in the between-subjects design approach.
The results from Study 5–a between-subjects only version of Study 4–were much closer to those obtained for Study 4a, than for Study 4b, despite both Study 4b and Study 5 being a between-subjects design using the same pictures, on the same survey platform, during the same season and with similar sample sizes. We note that the demographics between these two studies were slightly different in gender distribution. Proportionally, Study 4b had 10% more men than Study 4a did, and only a third of the proportion of non-binary participants. As our results show that covariates such as participants' gender reveal many interactions in mixed ANOVA models, we can assume a significant change in the gender distribution across our pool of respondents can lead to different survey results.
Future Research
Use of online platforms for testing design interventions has several benefits, such as cost-effectiveness, and speed of data collection. Future work could focus on exploring experimental ways of achieving similar benefits as provided by the online platforms but with more immersive experiences for the participants, so the in-person experience is replicated more realistically. Two avenues currently under investigation by the authors are (1) using videos in online surveys, and (2) conducting experiments in virtual reality.
Conclusion
Buildings are an under-leveraged resource to support the wellbeing of their users, and ensuring architects, engineers and interior designers understand the psychological effects of the indoor built environment is crucial to promoting health. Our online studies represent an inexpensive, scalable, and time-efficient approach to investigate the impact of design interventions such as materials, light, and representation on the wellbeing outcomes of belonging, self-efficacy and environmental efficacy. Such strategies have the potential to deliver results quickly and highlight significant patterns, such as those revealed during our meta-analysis, where we identify that natural materials and natural light lead to higher self-reported feelings of belonging, self-efficacy, and environmental efficacy.
As we have demonstrated with our suite of studies, some variables can impact the results of our online experiments, such as seasonality, dosage of the independent variables, or study design. Despite the aforementioned limitations, online surveys facilitate pilot testing to identify potentially impactful design interventions and allow for a more flexible trial and error process. Their results can thus inform the design of future laboratory or field studies and represent a valuable first step toward understanding how design interventions affect the human experience.
Data Availability Statement
The raw data supporting the conclusions of this article will be made available by the authors, without undue reservation if requested.
Ethics Statement
The studies involving human participants were reviewed and approved by Institutional Review Board (IRB) Protocol (#48481). The participants provided their written informed consent to participate in this study. Written informed consent was obtained from the individual(s) for the publication of any identifiable images or data included in this article.
Author Contributions
BA: study design revision, statistical analysis, and manuscript write-up. EB: study design revision, data collection, statistical analysis, and manuscript write-up. ID: study design conception and revision, data collection, and statistical analysis. KD, EM, and LB: study design conception and revision and statistical analysis. BB: statistical analysis. PP, JL, and SB: study design conception and revision. NA and HM: study design conception. All authors contributed to the article and approved the submitted version.
Funding
This material is based upon work supported by the National Science Foundation Graduate Research Fellowship under Grant No. DGE-1656518 and the Catalyst Grant from the School of Engineering at Stanford University. These funders had no part in the conceptualization, design, data collection, analysis, decision to publish, or preparation of the manuscript.
Conflict of Interest
The authors declare that the research was conducted in the absence of any commercial or financial relationships that could be construed as a potential conflict of interest.
Publisher's Note
All claims expressed in this article are solely those of the authors and do not necessarily represent those of their affiliated organizations, or those of the publisher, the editors and the reviewers. Any product that may be evaluated in this article, or claim that may be made by its manufacturer, is not guaranteed or endorsed by the publisher.
Acknowledgments
Sarah Lyons-Padilla, MarYam Hamedani, Alana Connor, Martin Fischer, John Barton, Gerry Hamilton, Andreas Katsanevas, Research Assistants (Yusef Ferhani, Naomi Gregorio, Selaine Rodriguez, Madeline K. Connelly), HP+DS Research Team, Stanford SPARQ team, View Inc. (materials and labor donations).
Supplementary Material
The Supplementary Material for this article can be found online at: https://www.frontiersin.org/articles/10.3389/frsc.2022.780376/full#supplementary-material
Footnotes
1. ^The difference between the participants' scores in two treatments (natural vs. artificial materials/light and diverse vs. non-diverse representations) is used as the treatment effect estimate in all studies.
2. ^A significant chi-square result from a Q-test indicates heterogeneity among effect sizes which means that the variance in the effect size estimates cannot be explained by the sampling error alone and there may be potential moderator variable/s. Studies that reveal high heterogeneity cannot be combined in a single meta-analysis (Morris and DeShon, 2002).
References
Behrend, T. S., Sharek, D. J., Meade, A. W., and Wiebe, E. N. (2011). The viability of crowdsourcing for survey research. Behav. Res. Methods 43, 800–813. doi: 10.3758/s13428-011-0081-0
Bolger, N., Zuckerman, A., and Kessler, R. C. (2000). Invisible support and adjustment to stress. J. Pers. Soc. Psychol. 79, 953–961. doi: 10.1037/0022-3514.79.6.953
Bornehag, C.-G., Blomquist, G., Gyntelberg, F., Järvholm, B., Malmberg, P., Nordvall, L., et al. (2001). Dampness in buildings and health. Indoor Air 11, 72–86. doi: 10.1034/j.1600-0668.2001.110202.x
Buhrmester, M., Kwang, T., and Gosling, S. D. (2016). “Amazon's mechanical turk: a new source of inexpensive, yet high-quality data?,” in Methodological Issues and Strategies in Clinical Research, ed A. E. Kazdin (Washington, DC: American Psychological Association), 133–139. doi: 10.1037/14805-009
Chandler, J., Paolacci, G., Peer, E., Mueller, P., and Ratliff, K. A. (2015). Using nonnaive participants can reduce effect sizes. Psychol. Sci. 26, 1131–1139.
Cheryan, S., Plaut, V. C., Davies, P. G., and Steele, C. M. (2009). Ambient belonging: how stereotypical cues impact gender participation in computer science. J. Pers. Soc. Psychol. 97, 1045.
Chmielewski, M., and Kucker, S. C. (2020). An MTurk crisis? Shifts in data quality and the impact on study results. Soc. Psychol. Pers. Sci. 11, 464–473. doi: 10.1177/1948550619875149
Dul, J., Ceylan, C., and Jaspers, F. (2011). Knowledge workers' creativity and the role of the physical work environment. Hum. Resourc. Manag. 50, 715–734. doi: 10.1002/hrm.20454
Edwards, L., and Torcellini, P. (2002). Literature review of the effects of natural light on building occupants (No. NREL/TP-550-30769). Technical Report, National Renewable Energy Lab., Golden, CO. (US). doi: 10.2172/15000841
Heschong, L.. (2003). Windows and Offices: A Study of Office Worker Performance and the Indoor Environment. California Energy Commision. 1–5.
Hettler, B.. (1976). The Six Dimensions of Wellness. National Wellness Institute. Available online at: ; http://www.Hettler.Com/sixdimen.Htm
Kaida, N., and Kaida, K. (2016). Pro-Environmental behavior correlates with present and future subjective well-being. Environ. Dev. Sust. 18, 111–127. doi: 10.1007/s10668-015-9629-y
Klepeis, N. E., Nelson, W. C., Ott, W. R., Robinson, J. P., Tsang, A. M., Switzer, P., et al. (2001). The national human activity pattern survey (NHAPS): a resource for assessing exposure to environmental pollutants. J. Expos. Anal. Environ. Epidemiol. 11, 231–252. doi: 10.1038/sj.jea.7500165
Milfont, T. L., and Duckitt, J. (2010). The environmental attitudes inventory: a valid and reliable measure to assess the structure of environmental attitudes. J. Environ. Psychol. 30, 80–94. doi: 10.1016/j.jenvp.2009.09.001
Morris, S. B., and DeShon, R. P. (2002). Combining effect size estimates in meta-analysis with repeated measures and independent-groups designs. Psychol. Methods 7, 105–125. doi: 10.1037/1082-989X.7.1.105
Nyrud, A. Q., and Bringslimark, T. (2010). Is interior wood use psychologically beneficial? A review of psychological responses toward wood. Wood Fiber Sci. 42, 202–218. Available online at: https://wfs.swst.org/index.php/wfs/article/view/1365/1365
Nyrud, A. Q., Bringslimark, T., and Bysheim, K. (2014). Benefits from wood interior in a hospital room: a preference study. Archit. Sci. Rev. 57, 125–131.
O'Neill, M. J.. (2010). A model of environmental control and effective work. Facilities 28, 118–136. doi: 10.1108/02632771011023104
Paolacci, G., and Chandler, J. (2014). Inside the turk: understanding mechanical turk as a participant pool. Curr. Direct. Psychol. Sci. 23, 184–188. doi: 10.1177/0963721414531598
Peer, E., Brandimarte, L., Samat, S., and Acquisti, A. (2017). Beyond the turk: alternative platforms for crowdsourcing behavioral research. J. Exp. Soc. Psychol. 70, 153–163. doi: 10.1016/j.jesp.2017.01.006
Peer, E., Rothschild, D. M., Evernden, Z., Gordon, A., and Damer, E. (2021). MTurk, Prolific or Panels? Choosing the Right Audience for Online Research. Available online at: https://papers.ssrn.com/sol3/papers.cfm?abstract_id=3765448
Poirier, G., Demers, C. M. H., and Potvin, A. (2019). Wood Perception in Daylit Interior Spaces: An Experimental Study Using Scale Models and Questionnaires. BioResources 14, 1941–1968.
Rosen, L. N., Targum, S. D., Terman, M., Bryant, M. J., Hoffman, H., Kasper, S. F., et al. (1990). Prevalence of seasonal affective disorder at four latitudes. Psychiatry Res. 31, 131–44. doi: 10.1016/0165-1781(90)90116-m
Schwarzer, R., and Jerusalem, M. (1995). “General self-efficacy scale (GSE)” in Measures in Health Psychology: A User's Portfolio. Causal and Control Beliefs, eds J. Weinman, S. Wright, and M. Johnston (NFER-NELSON), 35–37.
Sears, D. O.. (1986). College sophomores in the laboratory: influences of a narrow data base on social psychology's view of human nature. J. Pers. Soc. Psychol. 51, 515–530. doi: 10.1037/0022-3514.51.3.515
Sellers, B. C., Fiore, S. M., and Szalma, J. (2014). Developing a scale of environmental efficacy. Int. J. Sust. Policy Pract. 8, 169–195. doi: 10.18848/2325-1166/CGP/v08i04/55412
Steiner, P. M., Cook, T. D., Shadish, W. R., and Clark, M. H. (2010). The importance of covariate selection in controlling for selection bias in observational studies. Psychol. Methods 15, 250–267. doi: 10.1037/a0018719
Thatcher, A., and Milner, K. (2014). Changes in productivity, psychological wellbeing and physical wellbeing from working in A'green'building. Work 49, 381–393. doi: 10.3233/WOR-141876
Walton, G. M., and Cohen, G. L. (2007). A question of belonging: race, social fit, and achievement. J. Pers. Soc. Psychol. 92, 82–96. doi: 10.1037/0022-3514.92.1.82
Wazny, K.. (2018). Applications of crowdsourcing in health: an overview. J. Glob. Health 8, 010502. doi: 10.7189/jogh.08.010502
Yin, J., Arfaei, N., MacNaughton, P., Catalano, P. J., Allen, J. G., and Spengler, J. D. (2019). Effects of biophilic interventions in office on stress reaction and cognitive function: A randomized crossover study in virtual reality. Indoor Air 29, 1028–1039.
Keywords: meta-analysis, natural materials, natural light, sense of belonging, self-efficacy, diversity, environmental efficacy, design interventions
Citation: Altaf B, Bianchi E, Douglas IP, Douglas K, Byers B, Paredes PE, Ardoin NM, Markus HR, Murnane EL, Bencharit LZ, Landay JA and Billington SL (2022) Use of Crowdsourced Online Surveys to Study the Impact of Architectural and Design Choices on Wellbeing. Front. Sustain. Cities 4:780376. doi: 10.3389/frsc.2022.780376
Received: 21 September 2021; Accepted: 06 January 2022;
Published: 15 February 2022.
Edited by:
Thomas Krafft, Maastricht University, NetherlandsReviewed by:
Caren Martin, University of Minnesota Twin Cities, United StatesMichael Kent, University of California, Berkeley, Singapore
Copyright © 2022 Altaf, Bianchi, Douglas, Douglas, Byers, Paredes, Ardoin, Markus, Murnane, Bencharit, Landay and Billington. This is an open-access article distributed under the terms of the Creative Commons Attribution License (CC BY). The use, distribution or reproduction in other forums is permitted, provided the original author(s) and the copyright owner(s) are credited and that the original publication in this journal is cited, in accordance with accepted academic practice. No use, distribution or reproduction is permitted which does not comply with these terms.
*Correspondence: Basma Altaf, baltaf@stanford.edu; Eva Bianchi, ebianchi@stanford.edu
†These authors have contributed equally to this work and share first authorship