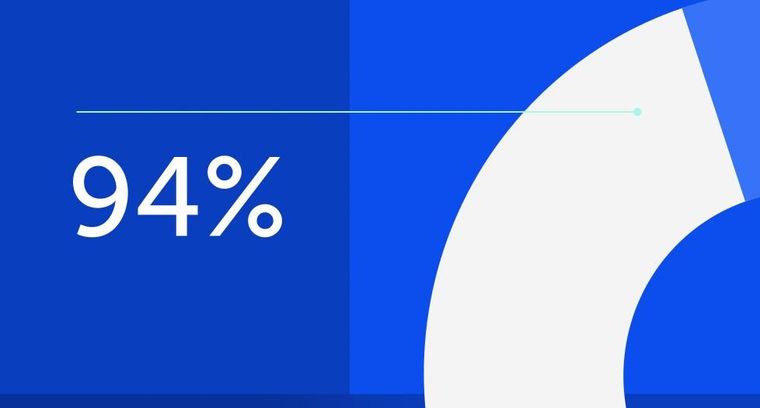
94% of researchers rate our articles as excellent or good
Learn more about the work of our research integrity team to safeguard the quality of each article we publish.
Find out more
ORIGINAL RESEARCH article
Front. Sustain. Cities, 16 November 2022
Sec. Smart Technologies and Cities
Volume 4 - 2022 | https://doi.org/10.3389/frsc.2022.1063067
This article is part of the Research TopicTowards 2030: A Sustainable Cities Perspective on Achieving Sustainable Development Goal 9 – Industry, Innovation, and InfrastructureView all 4 articles
Air pollution, climate change, and chemical exposure constitute the world's most significant environmental health concern, resulting in the early deaths of 6. 5 million people annually. Reducing child mortality from preventable causes, primarily pneumonia and other respiratory illnesses, would have contributed to the united nation's sustainable development goals (SDG). Some significant goals are sustainable cities, industry innovation, green and resilient infrastructure, good health, and well-being. Non-ventilator hospital-acquired pneumonia (NV-HAP) is a severe but preventable cause of morbidity and mortality in hospitalized patients. Despite being the most frequent and fatal hospital-acquired infection (HAI), NV-HAP is not tracked, documented, or avoided in most hospitals. The success of NV-HAP prevention and monitoring initiatives relies on reliable, up-to-date surveillance data. Surveillance offers the information needed to target, analyze, and quantify the efficacy of preventative activities by identifying patients at the highest risk for NV-HAP. However, pneumonia monitoring is complex due to the clinical criteria's subjective, imprecise, inconsistently recorded, and labor-intensive nature. Non-ventilator hospital-acquired pneumonia must be monitored and standardized, which demands cutting-edge technologies and the deployment of advanced sensors. In the framework of this research, initially, a wireless body area networks (WBANs) architecture has built by making use of wearable biosensors, and then real-time sensor data were uploaded to a cloud platform. Researchers have devised a wireless sensor network (WSN) to track volatile organic compounds (VOC) and other atmospheric characteristics in real time to curb the spread of NV-HAP. The ESP32 Internet of Things (IoT) and Raspberry Pi 4B graphical processing unit platforms host the finalized WBAN and WSN network. To reduce the mortality rate of NV-HAP, this research aims to investigate clinics' and hospitals' indoor and outdoor air quality. The developed biosensor-assisted IoT enabled framework is used in hospitals to keep tabs on the conditions of individual patient rooms, treatment areas, and critical care units in real time. The research found the suggested technique achieves better results than existing state-of-the-art methods regarding computing cost, communication overhead, storage cost, and energy utilization.
More fabulous resources and energy efficiency are potential long-term solutions to economic and environmental concerns, but only if these solutions are founded on innovation and technological progress. Policymakers will require cutting-edge information and communication technology (ICT) and infrastructure expenditures to speed up economic recovery, generate employment, decrease poverty, and boost productive investment when the Covid'19 crisis has passed its severe phase. Sustainable development goal no. 9 (SDG9) focuses on three interrelated pillars: infrastructure, industry, and innovation, with the ultimate goal of creating economic development that is both socially equitable and ecologically sustainable. These tenets aim to create an economy that benefits everyone and doesn't harm the environment. Seventeen objectives and indicators relating to its three pillars and other SDGs related to better health, food security, green technology, etc., are necessary to achieve SDG9 by 2030. However, indoor and outdoor air pollution remains a major cause of death, especially in low- and middle-income countries. Carbon monoxide (CO), nitrogen oxides, benzene, particulate matter (PM), and other hazardous organic compounds are only some of the many pollutants released when Biomass fuels are burned. As a result of the widespread use of polluting fuels and technologies, the health risks posed to women and children are disproportionately high. In addition to causing lower respiratory infections (21%), COPD (19%), and lung cancer (11%), smoking also raises the risk of having a stroke (23%), heart disease (32%), and other cardiovascular problems.
Pneumonia is a bacterial infection of the lungs that is very deadly. If sepsis is brought on by pneumonia, the patient will almost undoubtedly need to be admitted to the intensive care unit (ICU) and be supported with mechanical ventilation. Intensive care unit patients, in-patients, and healthcare workers might suffer from poor air quality, which could influence their health and the degree of comfort in the hospital. Non-pharmacological strategies for reducing hospital-acquired infections (HAI) include maintaining healthy indoor air quality. The three most common types of HAIs are ventilator-associated pneumonia (VAP), community-acquired pneumonia (CAP), and non-ventilator hospital-acquired pneumonia (NV-HAP). Non-ventilator-associated pneumonia is one of the most frequent and deadly illnesses in hospitals across the globe (Munro et al., 2021). Yet, it isn't recorded, reported, or actively avoided by most of them. The prevention of NV-HAP can enhance both the quality of treatment and the safety of the patient. A nosocomial infection or HAI is caused by viral, bacterial, or fungal pathogens obtained in a hospital, ICUs, outpatient clinic, or diagnostic laboratory. Health care personnel, in-patients, outpatients, visitors, an infectious environment, contaminated equipment, airborne, and droplet transmission can convey infection to a patient in the clinical setting. The microorganism might come from various sources, including the patient's microbiota, exhaled breath, and volatile organic compounds (VOC), which also contribute to HAIs especially hospital-acquired pneumonia (HAP) (Pantoja et al., 2016; Liao et al., 2019; Bartley et al., 2021).
Nosocomial pneumonia, or HAP is the most prevalent cause of pneumonia among critical care unit patients and those on mechanical ventilation. As much as 47% of all nosocomial infections in the ICU are caused by HAP, which occurs when bacteria from the upper respiratory tract are aspirated into the lower respiratory tract and then ascend from the stomach (Vignari, 2020). Non-ventilator-associated pneumonia accounts for about 68% of all HAP, appear within 48 h after admission; the remainder is VAP. Although HAP is an exogenous infection with nosocomial bacteria acquired from the hospital environment, limited information is known on the risk factors for HAP. Despite the fact that NV-HAP affects more people than any other healthcare-associated virus, most institutions do not monitor, lack both recordkeeping and preventative policies, or have policies to aggressively prevent NV-HAP (Baker and Quinn, 2018).
Most cases of HAP are brought on by the aspiration of bacteria from the patient's nasal or stomach flora. Aspiration pneumonia is the primary cause of mortality in stroke patients; it may occur in people with neuromuscular illnesses, chronic obstructive lung disease, radiation therapy, and neurologic abnormalities (Hayashitani et al., 2019; Yu et al., 2019). Even if a patient is quite healthy, aspiration may lead to pneumonia when they are hospitalized and their health state is weakened. Pathogens spread by healthcare personnel and hospital equipment may also cause HAP infection. Patient pneumonia is more common in acute care settings; thus healthcare staff must closely adhere to infection prevention guidelines while treating patients. Patients may develop NV-HAP on any unit in the hospital, which may need transfer to critical care. Nurses should be on the alert for potential of aspiration and respond swiftly and effectively to avoid HAP. To detect HAP in its early stages, tests including pulse oximetry, chest radiography, and sputum culture are often used (Youssef et al., 2020; Ayan et al., 2022; Skrvan et al., 2022). Some of the symptoms of NV-HAP including pus filled secretions, fever, shortness of breath, coarse breath sounds, etc. Long hospital stays, high medical costs, and admission in a long-term care institution are all potential consequences of NV-HAP. In order to minimize the HAI and mortality rate for thousands of hospital patients, NV-HAP must be the primary focus of attention. In light of the fact that NV-HAP has been linked to a wide variety of sociodemographic, clinical, and hospital-related parameters, an all-encompassing preventative strategy is required.
This curated research identifies risk factors and derived a bundle of strategies for preventing the chance of getting of non-hospital-acquired pneumonia. A wireless sensor network (WSN) and wireless body area network (WBAN) enabled Internet of Things (IoT) assisted bio-sensor framework is presented in this research. The following is a summary of the primary contributions that the research has made, including improvements to nursing practices and infection prevention standards that make use of contemporary technical breakthroughs and biosensors.
1. Developed a WSN for indoor air quality monitoring and tracking of harmful VOC in ICUs, inpatient room, and other hospital buildings.
2. Deployed a WBAN enabled wearable gadget for the incessant monitoring of heath parameters of ICU patients, in-patients, and hospital care workers and deploy it to AWS IoT cloud platform.
3. Developed a mobile application to monitor the developed IoT enabled WBAN and WSN assisted biosensor parameters and avoid NV-HAP by early detection of pneumonia symptoms and by preventing the risk of airborne and droplet borne diseases.
Wearable biosensors will allow diagnostic equipment to continually monitor a patient's physiological and biochemical parameters under natural physiological settings and in any location. Wearable device networks called WBANs and WSNs are integral parts of the healthcare IoT. Three key areas of respiratory healthcare research are being explored in WBAN, including body temperature, heart rate, and blood oxygen level (SpO2). Wireless sensor network measures and reports on air quality parameters like temperature, humidity, PM2.5, and VOC such as ozone, carbon dioxide (CO2), CO, benzene, and acetone. Wireless sensor network assisted smart wearable technologies will present unique prospects for the future of tailored respiratory treatment; early detection and prevention of NV-HAP is promising by the VOC mentioned earlier, even though various concerns still need to be resolved.
Hospital-acquired infections can be prevented by adequate hand hygiene, air quality monitoring, and face masks to avoid the transmission of airborne and droplet borne diseases. Staphylococcus aureus is the most common cause of HAP worldwide. Standardized oral hygiene reduces the number of bacteria in the mouth, hence lowering HAP to a more manageable level. Bacteria may bring pneumonia to the mouth, often inhaled into the lungs as human's sleep. As a basic guideline, improved dental hygiene will reduce the risk of pneumonia by 40–60%. Elevating the head of the bed by 30°-45° may decrease aspiration risk (Güner and Kutlutürkan, 2022). The majority of patients still spend more than 80% of their time in bed owing to a lack of early movement, while early mobilization is beneficial in reducing HAP (Garvey et al., 2021). It has long been established that coughing, deep breathing, and incentive spirometry all have a role in preventing NV-HAP in patients who have had surgery. Since more than half of NV-HAP patients are treated in medical units, these nursing practices may benefit patients who do not need surgical procedures. Coughing and deep breathing help expand the chest wall, and incentive spirometry-based inspiration regulation may improve oxygenation and reduce pulmonary issues and NV-HAP (Davila, 2020; Quinn et al., 2020).
Every hospital unit has a higher mortality rate from pneumonia that is not related to the use of a ventilator than from pneumonia that is acquired via the use of a ventilator. Non-ventilator hospital-acquired pneumonia is the most frequent kind of HAI. For patients diagnosed with NV-HAP, the average length of hospitalization is almost four times longer, and the fatality rate is nearly eight times higher. Non-ventilator hospital-acquired pneumonia is the cause of rising expenditures associated with long-term care, which places an additional strain on patients. The mortality rate is close to being the same for ventilator and non-ventilator acquired pneumonia.
Figure 1 depicts the proposed model of a biosensor network using WBANs and WSNs to identify NV-HAP early symptoms and monitor air quality. The patient's wearable device, built using the TTGO T ESP 32 IoT module, is equipped with various biosensors. The biosensor nodes are equipped with minimal storage and processing power, and they use the IEEE 802.15.6 protocol to transfer information with the base station (BS). The BS has a powerful Raspberry Pi 4 B computer with Graphical Processing Unit (GPU) capabilities and IoT connectivity. The Raspberry Pi 4B is a 64-bit Quad-core Cortex-A72 (ARM v8) system-on-a-module that features dual-band wireless LAN and Bluetooth and is certified for modular compliance. This enables the board to be designed into end products with significantly reduced compliance testing, reducing costs, and shortening development times. The whole WBAN database, including all patient information, is stored on an NVIDIA 128 Core Jetson Nano server computer. Using a smartphone app and IoT connectivity, physicians, medical analysts, and other authorized users can access patients' medical histories stored on a server. The server system in the proposed WBAN network paradigm provides data protection via password-based authentication.
Figure 1. The architecture of WBAN and WSN assisted biosensor network for monitoring air quality and detecting VOC compounds in hospitals.
The WSN is a sensor-based wireless network that monitors the indoor air quality and VOC components in ICUs, inpatient rooms, and other hospital facilities. This Wi-Fi-enabled healthcare WSN network was built using a Raspberry Pi 4B system on module (SoM) computer that monitors and maintains sensor networks in a specific region. As part of the WSN infrastructure, they are linked to the centrally located BS on various parts of the hospital. In a WSN network, a server station is configured with an NVIDIA Jetson Nano computer with a 128 CUDA core GPU. A GPU BS is connected to the WSNs located across the various hospital buildings through the internet to ensure that data is transmitted in a reasonable timeframe.
The deployment model of the WSN in the proposed research is depicted in Figure 2. Air pollutants and VOC such as nitrogen dioxide (NO2), benzene (C6H6), ozone (O3), CO2, CO, acetone (C3H6O), room temperature, and humidity are monitored using high-accuracy sensors that are distributed to the cloud and a local server. Physician and analysts can closely watch everything on their mobile phone with an android app. Raspberry Pi 4B nodes are the building blocks of a WSN. Over this, the computer may easily integrate more nodes or WSNs and can be accessed via a centralized monitoring system. Researchers have successfully established a WSN using the Raspberry Pi 4B platform to identify VOC and air quality metrics. It is a real-time, low-power method for tracking the potential for early detection of NV-HAP in ICU patients and the general public.
Recent technological advances have facilitated the creation of WSNs and WBAN by allowing for the widespread integration of non-invasive bio-sensors (Malik et al., 2020; Iqbal et al., 2022). Wireless sensor networks are autonomous sensors designed for use in healthcare, industry, agriculture, and the IoT. One of the most intriguing uses of WSNs is in WBANs which monitor patients' vital signs by transmitting data from various biosensor nodes either from in-body or on-body. Nowadays, WBANs are utilized in various settings, including but not limited to telemedicine, remote and continuous patient monitoring, and other clinical and non-clinical uses. Gateways and wireless technologies including 5G, Wi-Fi, WiMAX, Bluetooth, and satellite communication link various low-power biosensor nodes together (Curtis et al., 2022). Data acquired from a WBAN provide a significant problem due to the need for both secure storage and encrypted transfer. The less resource-intensive encryption methods are able to circumvent these problems. The WBAN design incorporates the Secure Hash Algorithm version 1 (SHA-1) and the Advanced Encryption Standard (AES) for protecting sensitive data.
Figure 3 shows the WBAN architecture utilized in this research for real-time monitoring of physiological parameters of in-patients, ICU patients, hospital care employees, and other hospital staff. The tiny and wearable bio-sensor array provides Wi-Fi-enabled mobility, which may serve as a means of mitigating the NV-HAP risk.
Figure 3. WBAN architecture deployed in wearable gadget on hospital care workers, ICU patients, and inpatients.
The WBAN design incorporates a contactless high precision Infrared (IR) digital temperature sensor (MLX90614); this sensor operates by a principle known as the Stefan-Boltzmann Law. Since the MAX30102 only requires a single 1.8 V supply, and module may be turned down in software to consume no standby current, the power rails can be left on at all times. The temperature is detected by an infrared thermopile detector (MLX81101), and the signal is converted to a digital value and sent over the I2C protocol via a single conditioning ASSP (MLX90302). The excellent precision and resolution of the MLX90302 sensor are made possible by its low noise amplifier, 17-bit ADC, and robust DSP. According to the research outcome, the MAX30102 has an accuracy of 97.86% when measuring heart rate and 98.43% while measuring oxygen saturation (SpO2). Because of this, it is used in fields as diverse as industry and medicine to take readings from various moving objects' temperatures. The clinical IoT framework of wearable device development employing personal activity trackers, fitness heart rate monitors, and other cardiac devices is best served using a photoplethysmography (PPG) approach. Detecting variations in blood volume in the skin's microvascular tissues at a cheap cost and without causing any harm is possible with the help of an integrated circuit made by MAX30102. Multiple medical IoT applications, including oxygen saturation measurement, blood pressure monitoring, and heart rate tracking, have contributed to the development of PPG technology. The IR LEDs and photo sensors in this gadget allow for non-invasive monitoring of heart rate and blood oxygen levels. Since blood has a higher absorption of light than other tissues, PPG sensors may detect variations in blood flow by measuring variations in light intensity. The voltage signal from a PPG is linked to the blood flow rate through the arteries. This method is superior because it can detect even minute fluctuations in blood volume. MAX30102 and MLX90614 sensor is interfaced to the TTGOT ESP32 IoT module, and the real-time information's shared with the cloud service without delay.
In Figure 4, this gadget to track vitals, including temperature, heart rate, and blood oxygen level, indicates its potential utility in preventing the spread of pneumonia through airborne and droplet-borne pathogens. For ICU and in-patients, a WBAN can be used to remotely monitor the patient's vitals in real-time, eliminating the requirement for a medical expert to be physically present at all times. Wireless body area networks are a new paradigm that may report a patient's health status and send other health-related data to a server in real-time. In this research, IoT-integrated WBAN is also used to reduce the power needs of the biosensor networks, hence boosting the WBAN's ability to diagnose illness and keep tabs on patients' health records.
Figure 4. Biosensor deployment in WBAN network for patients monitoring and tracking of physiological parameters using non-invasive sensors.
Maintaining the optimal indoor air quality is crucial to cutting down on hospital-acquired illnesses. The safety and well-being of patients are the major concerns of researchers and hospital administrators; as a result, a significant focus is placed on the regulation of environmental parameters such as temperature, humidity, and moisture levels. Temperature and relative humidity (RH) have a significant impact on the ability of viruses, bacteria, and fungus to survive in indoor air. The transmission of infectious diseases via airborne infection necessitates strict environmental control in hospitals. Virus survival weakens when the temperature rises from 20° to 24°, then 30°C, and RH to 23–80%. For example, influenza survival is at its lowest at 21°, and bacterial survival in the air reduces above roughly 24–40°C to 60% RH and <25% RH, respectively. Nevertheless, the air quality in operating rooms, ICUs, and inpatient rooms has to be carefully maintained to prevent the spread of illnesses brought on by the numerous chemicals present in indoor air; CO2, NO2, and VOC are some examples of these gases. There has been less emphasis on air quality in outpatient clinics, otorhinolaryngology, orthopedics, and pharmacies.
The Indian Society of Critical Care Medicine (ISCCM), Centers for Disease Control and Prevention (CDC), and other organizations have issued standards, guidelines, and recommendations for ICU functionality. The essential functions like temperature, humidity, and air distribution will provide a hospitable living conditions and a secure atmosphere for the patients, ICU staff, and visitors. Intensive care units around the world have a broad range of recommended temperatures, from 16°C to 25°C. While the highest allowable Index of Microbial Air Contamination (IMA) is five for isolation rooms and operating rooms, the maximum allowable IMA is 25 in ICU and dialysis units, but it varies by region. Monitoring the quality of ICU air is more important than we realize; ICU patients and healthcare workers might suffer from poor air quality, which could have a negative impact on their health and well-being. Poor air quality can also lead to many health issues and even increased heart rate.
This section describes a WSN-assisted IoT enabled ICU air quality monitoring system developed on a Raspberry Pi 4B SoM computer. The analog VOC and air quality sensors are interfaced using MCP 3008 analog to digital converter. It has an inbuilt inter-integrated circuit (I2C) protocol to establish a single wire serial communication with the Raspberry Pi computer. This developed handheld unit can measure indoor air quality, ambient parameters, and the presence of various VOC compounds to reduce the risk of airborne and droplet-borne infectious diseases. The PMS5003 sensor is most suited for recognizing particles, which are the most dangerous kind of air pollution and have a diameter of around 2.5 μm. PM2.5 particles are so small that they may travel through the respiratory system, into the brain, and even into the bloodstream, where they can cause a wide range of adverse health effects. Carbon dioxide is not toxic even in high amounts, but it may produce sleepiness, exhaustion, and other symptoms if it is present in an enclosed space where people are breathing it. Nitrogen dioxide can be exhaled by individuals who have lung disorders such as asthma and tuberculosis and those who are being treated with mechanical ventilators. The MQ131 gas sensor possesses high sensitivity to nitrogen oxides, particularly NO2. Even in low quantities, nitrogen oxides may irritate the upper respiratory tract and lungs, which can cause harm to the human respiratory system. As shown in Figure 5, using an IoT network, data on the concentrations of specific air pollutants has been continuously uploaded to the cloud and made accessible through the mobile application.
Figure 5. Biosensor deployment in WSN network for the air quality monitoring and tracking of VOC components.
Poorly maintained or functioning Heating, Ventilation, and Air-Conditioning (HVAC) system increases the possibility of airborne (coronavirus, influenza) and droplet-borne (tuberculosis, asthma, CAP) diseases in ICUs (Saran et al., 2020). Non-ventilator hospital-acquired pneumonia may affect persons of all ages, but children, the elderly, and pediatric patients are at the greatest risk and have severe consequences. According to the World Health Organization (WHO), CAP was responsible for 15% of all fatalities throughout the world in children under the age of 5 years old (WHO, 2022). The fast development of pneumonia in the elderly increases their risk for serious sickness and mortality by 20% (Bonnassot et al., 2020). The diagnosis of pneumonia is very difficult in ventilated and ICU patients due to the low sensitivity and specificity of chest radiography techniques (Luo et al., 2021). Exhaled breath contains VOC, some of which can be produced by bacteria and inflammatory processes. The main VOC parameters used in the VAP and HAP research are acetone, butanone, isopropanol, methanol, methyl, ketone, pentane, etc. Volatile organic compounds in exhaled breath of ventilated ICU patients can be used to discriminate between patients with pneumonia, patients with colonized airways, and controls.
Carbon dioxide, a byproduct of cellular metabolism, can significantly affect the lungs, which may be of therapeutic importance in very unwell patients, especially those with acute respiratory distress syndrome (ARDS) (Mehrabadi et al., 2021; Awad et al., 2022). Numerous reports have shown that CO2 may harm the lungs regardless of pH (Kacmarek et al., 2018; Pirapuraj et al., 2021). To prevent CO2 rebreathing, an average adult must provide adequate fresh gas flows. Critical care ventilators are not equipped with CO2 absorbers and should be set to deliver a new oxygen supply between two and three times per minute. The Table 1 summarizes the non-dispersive infrared (NDIR) CO2 sensors utilized in various researches, along with some technical details about each. Its unique sensing range, response time, operating voltage range, and VOC compound sensitivity led to its selection.
The MG-811 is a metal oxide sensor with a higher sensitivity for CO2 and low selectivity for alcohol and CO. This sensor measures the concentration of CO2 in the air by tracking how much IR light is absorbed by CO2 molecules. The MG 811 sensor's range of CO2 detection ability makes it ideal for checking the air quality in healthcare facilities; it can detect concentrations as low as 350 ppm and as high as 10,000 ppm. The Figaro TGS 822 is a tin dioxide (SnO2) semiconductor sensor, and its conductivity increases as the gas concentration in the air increases. TGS 822 has a high sensitivity to organic solvent vapors and volatile vapors. For the detection of VOC like formaldehyde, toluene, and benzene, researchers could rely on the MS-1100 semiconductor VOC gas sensor. It has numerous applications, including ventilators, air filters, and monitoring air quality in confined locations. The MS1100's large dynamic range and accuracy over a wide range of target gas concentrations make it suitable in many contexts. As a result of their low current consumption and great sensitivity, these sensors are well-suited for use in portable devices, ICUs, and ventilators.
Ground ozone is formed as a result of a photochemical interaction between nitrogen and volatile organic molecules. Reactive oxygen species (ROS) are formed when ozone enters the lungs and cause respiratory problems such as asthma. ULPSM-O3 968-046 is a low-power linear voltage output healthcare sensor from SPEC that can measure ozone, CO, NO2, and methane with excellent resolution. The following equation is used to determine the target concentration of the gas.
In Equation (1), C is the gas concentration in parts per million (ppm), Cf is the sensor calibration factor (Volt/ppm), Vs is the sensor output voltage, Va is the voltage output in a clean-air. Va is defined by the following equation:
Vref and Voff are the reference signal and offset factor of the sensor, respectively. The sensor calibration factor Cf is the product of sensitivity code Scode and the gain of trans impedance amplifier TIAgain as given in Equation (3).
Virus survival is greatly affected by temperature since it may influence the condition of viral proteins and the DNA. The temperature ranges from 21 to 24°C and below 30°C, which is optimal for virus life. The lifespan of airborne viruses in aerosols is also influenced by the interaction between temperature and RH. Bacterial survival seems to be reduced across the board at temperatures higher than 24°C. Carbon monoxide has a detrimental impact on bacteria when the RH is <25%, but a protective effect when the RH is more than 90%. The interior temperature and the RH of the air can be regulated to reduce the danger of viral transmission via the air. High temperatures and RH have been linked to reducing the spread of Covid'19, the new coronavirus (Bhalla et al., 2020; Bilandi et al., 2021). Although it has been proven that high moisture prevents the transmission of different airborne and droplet diseases by as much as 60%, growth accelerates dramatically after it surpasses the limit. Reducing influenza virus transmission by keeping hospital facilities at a certain temperature and RH (Boonsong et al., 2022).
Airborne infectious pathogens may be effectively controlled in various settings; it must be determined which airborne pathogen provides the most danger to patients and healthcare workers in hospitals. The ICU, operating room, neonatal ICU, emergency department, treatment rooms, and inpatient and outpatient sections all have various temperature and RH settings. There will be a wide range of airborne and droplet-borne infectious agents, each with its circumstances. It will be necessary to determine which pathogen offers the most danger to hospital patients and caregivers. Thus, specialized temperature and humidity management are needed to reduce the spread of infectious diseases in ICUs, hospitals, and healthcare facilities. DHT22 is deployed in indoor areas to measure the temperature and RH. It is a highly précised and accurate sensor suitable for medical and industrial applications with I2C protocol interfacing. It has a high sampling rate (0.5 Hz), wide humidity (0–100%), and temperature range (−40°C to 125°C) with an accuracy of 2% and ±0.5, respectively. Medical professionals, patients, and hospital visitors might benefit from a new sensor mechanism that reduces the possibility of viral aerosol transmission. Temperature and humidity must be precisely controlled in healthcare facilities to limit the transmission of infectious diseases.
The WSN networking devices included a high-resolution 7-inch touch Display Serial Interface (DSI) screen. The DSI is a mobile device display controller cost-reduction standard developed by the Mobile Industry Processor Interface (MIPI) Alliance. The host serves as the data source for the images, and the device serves as the final destination. Hence this standard establishes the serial bus protocol for exchanging information between the two.
Non-ventilator hospital-acquired pneumonia monitoring is challenging since the clinical criteria for NV-HAP are not only subjective but often erroneous, inconsistently recorded, and time-consuming to implement. Alternative surveillance strategies are currently being developed; many of these have resulted in creating a screening algorithm for NV-HAP that considers radiographic procedures performed within 48 h of admission, the lack of mechanical ventilation, and the presence of fever, among other symptoms. It is necessary to conduct NV-HAP surveillance that is accurate, performed promptly, and as effectively as possible to inform preventative and monitoring activities. Surveillance gathers information that is crucial for assessing the scale of the issue, highlighting high-risk patients, and assessing the efficacy of preventive efforts. Antibodies, lifestyle, environmental factors, hospitalization, ICU, and ventilator admission all increase the risk of pneumonia (Almaslukh, 2021). Many instances of pneumonia may be prevented by pneumococcal vaccinations, but they cannot prevent all of them. People who are at risk for pneumonia complications may, however, be less likely to have these consequences. Antibiotics work well for bacterial pneumonia and antifungal medications work well for fungal pneumonia, but antibiotics have little impact on viral pneumonia.
The major goal of this research is to keep to track the air quality and other VOC. Carbon monoxide, CO2, VOC, temperature, humidity, and PM2.5 were the pollutants targeted in the ICU, in-patient rooms, and other hospital treatment rooms to avoid hospital-acquired illnesses, notably NV-HAP. Atmospheric PM with a diameter of fewer than 2.5 μm, or PM2.5, can only be seen using a microscope. Particles smaller than 2.5 μm may bypass the nose and reach deep into the lungs. Exposure to fine particles is strongly associated with increased mortality risk from cardiovascular and respiratory diseases. On a clear day, PM2.5 concentrations may be as low as 5 and are harmful above 35.4 g/m3, 0–12 is normal, 12–35 is moderate and sensitive for persons with repository symptoms, and 35–55 is unhealthy and increases the risk of respiratory, heart, or lung illness in sensitive people. Carbon dioxide is produced during normal cellular metabolism and excreted. Acute lung damage, hypercapnia, etc., are only a few of the many impacts exerted by this potent biological agent. The air quality inside a building has been shown to significantly affect people's health, productivity, health worries, sleep, comfort, and well-being. Concentrations of 600, 800, 1,000, and 1,500 ppm of CO2 were used in this study. All experiment sessions are conducted on the same day, separated by 2 h, and maintained at a constant temperature, ventilation rate, and humidity level. Up to 600 ppm of CO2 is excellent, 800 ppm is very good, and 1,000 ppm is acceptable. Since the pollutant count is between 1,000 and 1,500, proper ventilation is necessary. Up to 800 ppm is ideal for ICUs and hospital wards, while <1,000 ppm is anticipated in outdoor settings.
Volatile organic compounds will increase the likelihood of airborne and droplet-borne diseases, notably the NV-HAP. Poor air quality has been highlighted as a potential source of health concerns for healthcare workers and in-patients. Both the WSN and WBAN networks are installed in hospitals, albeit in distinct locations. Indoor air quality and VOC components in various locations of hospital is plotted in Figure 6. Low risk of air pollution is found in the ICU, in-patient rooms, the pharmacy, and the treatment areas. In contrast, there is a high risk of air pollution in the reception and visitor lounge. Because of the prevalence of VOC chemicals in ICUs, regular patient rooms, and consultation rooms, the transmission of NV-HAP is more likely in all of these environments. Those parameters are broadcast to an IoT cloud platform, which is also accessible in the mobile application by means of a Wi-Fi or Bluetooth protocol. The developed indigenous system has been installed in various locations of government and private hospitals.
Furthermore, it is essential that hospitals have realistic and generalizable tactics that they may use to properly implement this new approach of prevention. Despite the fact that NO2 is often regarded as a major pollutant, there are no notable disparities in the NO2 concentrations among the various hospitals. Climate and seasonal changes affect the total NO2 concentration. The amounts of CO, CO2, VOC, or NO2 were not significantly affected by an air-cleaner HVAC system placed in ICU units. People of all ages are susceptible to NV-HAP, although youngsters and the elderly are at the greatest risk of developing the disease. In spite of this, NV-HAP may cause substantial harm to new born babies. The NV-HAP incidence rate is calculated as follows:
In Equation (5), IRhd is the rate of NV-HAP per patient's days Nhap is the number of NV-HAP cases and Nhd is the total number of adult patient days. Researchers prospectively monitored the air quality and VOC levels in various parts of a hospital for a whole year through the monsoon, winter, summer, and spring.
The United Nations Environment Programme (UNEP) is actively working to assist the world in achieving the 17 sustainable development goals (SDGs). In this research, we propose environmental sustainability to realize the SDGs and guarantee its core tenets-good health, industrial innovation, and prosperity. Keeping the environment clean is crucial to people's health and happiness. Conversely, inadequate management of dangerous chemicals, air pollution, and other environmental issues such as chemical exposure, climate change, and UV radiation negatively affect human health. Due to a lack of regulations requiring hospitals to monitor pneumonia rates, a significant obstacle in preventing pneumonia and, particularly, NV-HAP remains a hidden burden in hospitals. Non-ventilator hospital-acquired pneumonia was discovered in general and intensive care wards for patients of all ages. This suggests that all patients bear some risk for NV-HAP, even if some individuals are at greater risk than others. Patients seldom get preventative therapeutic measures for NV-HAP in acute care settings. To address this issue, healthcare facilities should consider the mounting data pointing to NV-HAP as a widespread HAI and support the implementation of NV-HAP preventive methods. However, even the healthiest people are in danger. Previous HAP prevention research addressed particular patient categories; most intervention trials focused on ventilated patients, rather than ICUs and inpatients. To ascertain the true scope and effect of NV-HAP, it is necessary to conduct more monitoring and evaluation of incidence. Prevention of NV-HAP should receive the same degree of attention, focus, and resources as other HAIs. Wireless body area networks have emerged as a source of hope in the fight against an aging population, chronic illnesses, airborne, and droplet borne diseases. Wireless body area networks employ several biosensor nodes embedded in wearable devices to monitor vital signs, including oxygen saturation (SpO2), body temperature, and pulse rate. Importantly, these vitals are sent to a doctor through a secured network, and mobile application allowing for quick and easy remote diagnosis. Intensive care units, inpatient rooms, and other hospital areas is benefited from the WSN's ability to monitor indoor air quality and VOC components via wireless sensors. Built with a Raspberry Pi 4B SoM computer, this Wi-Fi-enabled healthcare WSN network monitors and maintains sensor networks in a given area. The goal of SDG would have benefited from a reduction in child mortality from avoidable causes, including pneumonia and other respiratory disorders. It is also essential to develop indoor air quality monitoring and management mechanisms to make green infrastructure, improve energy efficiency, and lessen their negative environmental impacts. The proposed method outperforms prior state-of-the-art approaches in computing cost, communication overhead, storage cost, and energy use, as determined by the performance analysis.
The original contributions presented in the study are included in the article/supplementary material, further inquiries can be directed to the corresponding author/s.
Both authors listed have made a substantial, direct, and intellectual contribution to the work and approved it for publication.
The authors declare that the research was conducted in the absence of any commercial or financial relationships that could be construed as a potential conflict of interest.
All claims expressed in this article are solely those of the authors and do not necessarily represent those of their affiliated organizations, or those of the publisher, the editors and the reviewers. Any product that may be evaluated in this article, or claim that may be made by its manufacturer, is not guaranteed or endorsed by the publisher.
Almaslukh, B. (2021). A lightweight deep learning-based pneumonia detection approach for energy-efficient medical systems. Wirel. Commun. Mob. Comput. 5556635, 1–14. doi: 10.1155/2021/5556635
Awad, M. E., El-Garhy, A. M., Eldosoky, M. A., and Soliman, A. M. (2022). “Modeling and simulation of respiratory system for Acute Respiratory Distress Syndrome (ARDS) associated with COVID-19,” in 2021 38th National Radio Science Conference (NRSC) (Mansoura), 232–242.
Ayan, E., Karabulut, B., and Ünver, H. M. (2022). Diagnosis of pediatric pneumonia with ensemble of deep convolutional neural networks in chest X-ray images. Arab. J. Sci. Eng. 47, 2123–2139. doi: 10.1007/s13369-021-06127-z
Baker, D., and Quinn, B. (2018). Hospital acquired pneumonia prevention initiative-2: incidence of nonventilator hospital-acquired pneumonia in the United States. Am. J. Infect. Control. 46, 2–7. doi: 10.1016/j.ajic.2017.08.036
Bartley, D., Panchasarp, R., Bowen, S., Deane, J., and Ferguson, J. K. (2021). How accurately is hospital acquired pneumonia documented for the correct assignment of a hospital acquired complication (HAC)? Infect. Dis. Health. 26, 67–71. doi: 10.1016/j.idh.2020.09.004
Bhalla, N., Pan, Y., Yang, Z., and Payam, A. F. (2020). Opportunities and challenges for biosensors and nanoscale analytical tools for pandemics: COVID-19. ACS Nano. 14, 7783–7807. doi: 10.1021/acsnano.0c04421
Bilandi, N., Verma, H. K., and Dhir, R. (2021). An intelligent and energy-efficient wireless body area network to control coronavirus outbreak. Arab. J. Sci. Eng. 46, 8203–8222. doi: 10.1007/s13369-021-05411-2
Bonnassot, P., Barben, J., Tetu, J., Bador, J., Bonniaud, P., Manckoundia, P., et al. (2020). Clostridioides difficile infection after pneumonia in elderly patients: which antibiotic is at lower risk? J. Hosp. Infect. 2020:S0195-6701(20)30240-1. doi: 10.1016/j.jhin.2020.05.009
Boonsong, W., Senajit, N., and Prasongchan, P. (2022). Contactless body temperature monitoring of In-Patient Department (IPD) using 2.4 GHz microwave frequency via the Internet of Things (IoT) network. Wirel. Pers. Commun. 124, 1961–1976. doi: 10.1007/s11277-021-09438-4
Curtis, S. J., Rathnayaka, A., Wu, F., Al Mamun, A., Spiers, C., Bingham, G., et al. (2022). Feasibility of bluetooth low energy wearable tags to quantify healthcare worker proximity networks and patient close contact: a pilot study. Infect. Dis. Health. 27, 66–70. doi: 10.1016/j.idh.2021.10.004
Davila, S. (2020). Non-ventilator health care-associated pneumonia (NV-HAP): taking action to improve NV-HAP outcomes. Am. J. Infect. Control. 48, A28–A35. doi: 10.1016/j.ajic.2020.03.004
Garvey, M. I., Wilkinson, M. A. C., Woodall, H., Smith, C., Holden, K., Cartin, J., et al. (2021). Mouth care matters - a HAP prevention strategy. J. Infect. 83, 381–412. doi: 10.1016/j.jinf.2021.05.032
Güner, C. K., and Kutlutürkan, S. (2022). Role of head-of-bed elevation in preventing ventilator-associated pneumonia bed elevation and pneumonia. Nurs. Crit. Care 27, 635–645. doi: 10.1111/nicc.12633
Hayashitani, M., Yumoto, E., Hosoi, T., Kubo, M., Hiramitsu, H., Kato, K., et al. (2019). “Field trial of aspiration pneumonia predicion based on electronic medical records,” in 2019 IEEE International Conference on Healthcare Informatics (ICHI) (Xi'an), 1–2. doi: 10.1109/ICHI.2019.8904811
Iqbal, J., Adnan, M., Khan, Y., AlSalman, H., Hussain, S., Ullah, S. S., et al. (2022). Designing a healthcare-enabled software-defined wireless body area network architecture for secure medical data and efficient diagnosis. J. Healthc. Eng. 2022, 9210761. doi: 10.1155/2022/9210761
Kacmarek, R. M., Villar, J., and Berra, L. (2018). Exhaled CO2, a guide to ARDS management during lung-protective ventilation? J. Crit. Care. 45, 229–230. doi: 10.1016/j.jcrc.2018.03.013
Liao, Y. H., Wang, Z. C., Zhang, F. G., Abbod, M. F., Shih, C. H., Shieh, J. S., et al. (2019). Machine learning methods applied to predict ventilator-associated pneumonia with Pseudomonas aeruginosa infection via sensor array of electronic nose in intensive care unit. Sensors. 19, 1866. doi: 10.3390/s19081866
Luo, Y., Zhang, Y., Sun, X., Dai, H., and Chen, X. (2021). Intelligent solutions in chest abnormality detection based on YOLOv5 and ResNet50. J. Healthc. Eng. 2021, 2267635. doi: 10.1155/2021/2267635
Malik, N. N., Alosaimi, W., Irfan Uddin, M., Alouffi, B., and Alyami, H. (2020). Wireless sensor network applications in healthcare and precision agriculture. J. Healthc. Eng. 2020, 8836613. doi: 10.1155/2020/8836613
Mehrabadi, M. A., Aqajari, S. A. H., Azimi, I., Downs, C. A., Dutt, N., Rahmani, A. M., et al. (2021). “Detection of COVID-19 using heart rate and blood pressure: lessons learned from patients with ARDS” in 2021 43rd Annual International Conference of the IEEE Engineering in Medicine and Biology Society (EMBC) (Guadalajara), 2140–2143. doi: 10.1109/EMBC46164.2021.9629794
Munro, S. C., Baker, D., Giuliano, K. K., Sullivan, S. C., Haber, J., Jones, B. E., et al. (2021). Nonventilator hospital-acquired pneumonia: a call to action: recommendations from the National Organization to Prevent Hospital-Acquired Pneumonia (NOHAP) among nonventilated patients. Infect. Control Hosp. Epidemiol. 42, 991–996. doi: 10.1017/ice.2021.239
Pantoja, L. D. M., do Nascimento, R. F., and de Araújo Nunes, A. B. (2016). Investigation of fungal volatile organic compounds in hospital air. Atmos. Pollut. Res. 7, 659–663. doi: 10.1016/j.apr.2016.02.011
Pirapuraj, P., Minnoli, N. V., Saranrai, M., and Thevaruban, K. (2021). “Automated ventilation system for maintaining indoor CO2 concentration to reduce allergic and respiratory diseases: for rural area in Batticaloa, Sri Lanka,” in 2021 Asian Conference on Innovation in Technology (ASIANCON) (Ravet), 1–8. doi: 10.1109/ASIANCON51346.2021.9544870
Quinn, B., Giuliano, K. K., and Baker, D. (2020). Non-ventilator health care-associated pneumonia (NV-HAP): best practices for prevention of NV-HAP. Am. J. Infect. Control. 48, A23–A27. doi: 10.1016/j.ajic.2020.03.006
Saran, S., Gurjar, M., Baronia, A., Sivapurapu, V., Ghosh, P. S., Raju, G. M., et al. (2020). Heating, ventilation and air conditioning (HVAC) in intensive care unit. Crit. Care. 24, 194. doi: 10.1186/s13054-020-02907-5
Skrvan, A., Hudec, R., and Matuska, S. (2022). “Design of a cheap pulse oximeter for home care systems,” in 2022 ELEKTRO (ELEKTRO) (Krakow), 1–6. doi: 10.1109/ELEKTRO53996.2022.9803610
Vignari, M. (2020). Non-ventilator health care-associated pneumonia (NV-HAP): NV-HAP risk factors. Am. J. Infect. Control. 48, A10–A13. doi: 10.1016/j.ajic.2020.03.010
WHO (2022). Pneumonia. Available online at: https://www.who.int/news-room/fact-sheets/detail/pneumonia (accessed August 28, 2022).
Youssef, T. A., Aissam, B., Khalid, D., Imane, B., and Miloud, J. E. (2020). “Classification of chest pneumonia from X-ray images using new architecture based on ResNet,” in 2020 IEEE 2nd International Conference on Electronics, Control, Optimization and Computer Science (ICECOCS) (Kenitra), 1–5. doi: 10.1109/ICECOCS50124.2020.9314567
Keywords: bio-sensors, droplet and airborne diseases, Internet of Things, pneumonia, sustainable life, volatile organic compounds, wireless body area network, wireless sensor network
Citation: Abubeker KM and Baskar S (2022) Wireless sensor and wireless body area network assisted biosensor network for effective monitoring and prevention of non-ventilator hospital-acquired pneumonia. Front. Sustain. Cities 4:1063067. doi: 10.3389/frsc.2022.1063067
Received: 06 October 2022; Accepted: 21 October 2022;
Published: 16 November 2022.
Edited by:
V. R. Sarma Dhulipala, Anna University, IndiaReviewed by:
Rinesh Sahadevan, Jigjiga University, EthiopiaCopyright © 2022 Abubeker and Baskar. This is an open-access article distributed under the terms of the Creative Commons Attribution License (CC BY). The use, distribution or reproduction in other forums is permitted, provided the original author(s) and the copyright owner(s) are credited and that the original publication in this journal is cited, in accordance with accepted academic practice. No use, distribution or reproduction is permitted which does not comply with these terms.
*Correspondence: K. M. Abubeker, a21hYnViZWtlcjgyQGdtYWlsLmNvbQ==
Disclaimer: All claims expressed in this article are solely those of the authors and do not necessarily represent those of their affiliated organizations, or those of the publisher, the editors and the reviewers. Any product that may be evaluated in this article or claim that may be made by its manufacturer is not guaranteed or endorsed by the publisher.
Research integrity at Frontiers
Learn more about the work of our research integrity team to safeguard the quality of each article we publish.