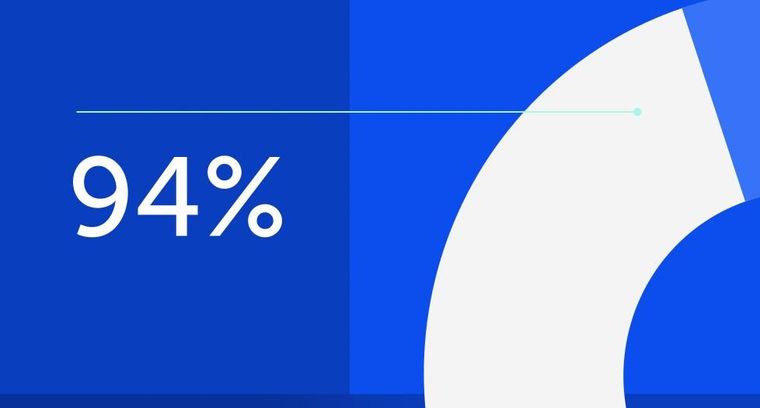
94% of researchers rate our articles as excellent or good
Learn more about the work of our research integrity team to safeguard the quality of each article we publish.
Find out more
ORIGINAL RESEARCH article
Front. Sustain. Cities, 13 January 2022
Sec. Smart Technologies and Cities
Volume 3 - 2021 | https://doi.org/10.3389/frsc.2021.787076
This article is part of the Research TopicData-Driven Approaches to Enable Urban TransformationView all 5 articles
The methodology presented in this paper produces a circular economy jobs (CE jobs) measure. Using jobs as a proxy indicator, these measure gives cities a robust number to indicate progress toward the circular economy and is designed to serve as a first step in developing a circular economy strategy. The CE jobs measure tracks the inputs and outputs of goods in a city's “boundaries” through the material import dependency of the city's economic sectors. At the same time, tracking and assessing the circularity of the local jobs in these economic sectors will also provide city leaders with an indication of which sectors circularity is happening and could potentially happen. This paper also concludes that the process of coming to the CE jobs has two parts, the first more relevant to the local government and the second better influenced by the national government. Both need to come together for a truly circular local economy to happen.
Increasing awareness of the socio-economic and environmental shortcomings of our existing economic model sparked renewed interest in the circular economy model. The International Resource Panel (Fischer-Kowalski et al., 2011) has been actively promoting, for almost a decade now, the urgency of closing materials loops. Their work puts an emphasis on the concept of “decoupling,” describing that economic growth should occur without an increase in environmental harm, both in terms of resource depletion and pollution (Swilling et al., 2013).
Circular economy is a way to transform the current economic model toward a sustainable future, decoupling natural resource use and environmental impacts from economic activity UNEP (2020). The transition toward a circular economy can create business and economic opportunities by introducing new design strategies, production processes, and business models (Webster, 2017). Simultaneously, workers' rights need to be safeguarded and the workforce needs to be skilled and reskilled to get the most out of this transition (Circle Economy, 2020).
Measuring the transition to a circular economy is, however, still a work in progress. Countries and regions around the globe have started by monitoring their raw-material import dependency. For example, the EU is tracking self-sufficiency in raw materials (among other indicators) as part of their monitoring framework toward a circular economy (EC, 2020a,b).
Since an overwhelming majority of this economic growth and overall material consumption is happening in cities, it is no surprise that cities have also started to work on their own circular economy strategies (see, for example, LWARB, 2017; Ma.Paris, 2017). Their actions, as centers of political and economic activity, are expected to have a significant impact on the global Circular Economy transition. It is, however, particularly challenging for cities to quantify their circular economy transition. Their boundaries are not as defined, and, thus, the movement of goods and people are not closely monitored.
This paper builds on the work of the United Nations Environment Programme (UNEP) and Circle Economy (CE), two organizations that have been working to advance circularity in cities around the world by making it more measurable and actionable. It presents a methodology that uses employment as an indicator toward a circular economy, merging two approaches—a method produced by UNEP that is founded on urban metabolism and a second method developed by Circle Economy method is founded on IO Analysis and a framework for circular economy activities based on a literature review.
By implementing an environmentally extended Input-Output analysis at a city level, CE and UNEP aim to:
1. Consider the strategic and practical application of circular economy at city level using what CE defines as “key elements of a circular economy.”
2. Illustrate how cities contribute to overall global raw material consumption reduction by quantifying the material import dependencies (MID) through the use of raw material equivalents.
3. Combine these two approaches to come up with a CE jobs measure that will serve as a globally replicable first step to developing a circular economy plan at city level.
This methodology is illustrated in two different cities. The first is a city from the Global North, center of the European Union, and capital of Belgium: Brussels. The second is a coastal city from the Global South, capital of the province of Pernambuco, and the fourth-largest agglomeration in Brazil: Recife. The results are then presented, followed by the opportunities on future areas of work, particularly on data and the informal sector.
Yalçn and Foxon (2021) propose the following policy recommendations for the transition to a Circular Economy: “(a) being pragmatic in establishing plans, (b) quantifying and qualifying contribution of CE at the local level, (c) focusing on understanding and managing resource flows, (d) prioritizing sectors with a higher potential of minimizing environmental impacts and with the bigger opportunity to trigger economic efficiency, (e) facilitating city level collaborations with major urban stakeholders, (f) creating support schemes for businesses, (g) creating an approach that is more inclusive to citizen and community, and (h) support for changing consumer behaviors.” The presented research on this paper aims to contribute to the realization of these recommendations that accurately describe the goals set by both contributing organizations.
The method described below certainly entails a number of limitations. It does not address the challenge of defining administrative boundaries, nor does it put a framework on the meaning of “local jobs.” Both remain political decisions rather than a part of this methodology. The method also does not touch upon the informal economy, among other topics seen as areas for future work.
Limitations in data use also need to be acknowledged, as we had to balance replicability and accuracy.
Bottom-up data would be a more accurate and reliable source, but this is not possible for cities all over the world. To facilitate replicability, national Input-Output tables were used, as these are present in most national accounting systems. Data from the national level was then downscaled to get a snapshot of the local economy. The downscaling process introduces some uncertainty into the computations. By downscaling national-level data, we assume: (1) sectors interact in direct proportion to the volume of employment and (2) the same import shares which may not always be the case.
The downscaling process if performed by aligning the national IO tables to local employment data. A full reconstruction of city-level IO tables is outside the scope of this paper. Nonetheless, for a deeper analysis of intercity economic interactions, such tables would be necessary. Such an analysis could foster interesting results in the domain of city level circularity, as one could understand circularity at a regional level.
We used national level material import dependency (MID) to compute the circularity of each economic sector, and this also sacrificed accuracy. Downscaled tables also reflect national-level import dependencies rather than city-level import dependencies. This introduces a bias toward large countries, which inevitably have smaller import dependencies. MID describes the share of imported materials rather than absolute material consumption, not taking into account other external environmental impacts. For example, countries with a large production of non-renewable energy would have a low import dependency vis a vis carbon-intense industries. By using environmentally extended multi-regional input-output tables (EE-MRIO), as is the case of EORA, we can extend this analysis to take into account other environmental impacts of economic sectors. Future research in this direction should consider the implementation of other environmental indicators for the definition of a circular economy1.
It is also important to stress that the resulting “circular economy jobs measure” in this exercise is not recommended as a benchmarking tool, and should be looked at with the context of the city being analyzed in mind. It is undoubtedly possible to use it to benchmark against cities of the same type and size, but it has little meaning to use as a comparison for cities as different as Brussels and Recife, which are used, in this paper, to illustrate the methodology.
The selection of the cities aims to ensure that the presented method is applicable to a diverse context. For the selection of cities, it was important for the research team to include a global south city in order to address the existing data-bias toward large global north cities (Creutzig et al., 2019). Both cities present also a very different economic profile and exist on a very different national context. The country in which both cities exist has a direct impact on the results, because of the computes material import dependency (MID). The presented examples are from: (a) a net-importer country (Belgium) and (b) a net-exporter one (Brazil). This is extremely relevant because of the given local-national relationship. Although it is impossible to cover the full spectrum of cities with only two examples, this selection points toward the need to address the individuality of urban-systems and the need to develop specific policies and tools able to address the individuality of cities. The team is currently working on expanding the analysis to a larger city sample in order to compute a larger spectrum of cities and their economic profiles.
The circular economy is a system that is restorative and regenerative by design, by introducing new design strategies, production processes and business models (Ellen MacArthur Foundation, 2015). It provides an alternative to the current linear take-make-waste economy. It finds its origins in urban metabolism (UM), which is committed to mapping resource consumption, asset accumulation and waste emissions in cities (Pincetl et al., 2012); industrial ecology, which embraces a systemic approach to cycling materials and energy (Saavedra et al., 2018); cradle to cradle, where product design is the central challenge toward high value cycling of materials (McDonough, 2003); and finally the performance economy, which decouples the relationship between product use and ownership (Stahel, 2010). While the concept is not new, its application in the past has been primarily at the global and national scale. Local level application of Circular Economy occurred primarily in the informal sector and waste management (Vanhuyse et al., 2021). Possible applications at the city level are gaining ground only recently.
A shift from linear to circular economy is necessary if we aim to achieve the environmental goals set by the international community, currently available literature on the topic systematically points out to the same conclusion. The linear economic model not only continues to bring about irreparable damage to our environment, but also aggravates socio-economic inequalities. Whereas the literature on the circular economy mostly emphasizes the minimization of waste and environmental impacts on the one hand, and the related potential business opportunities (Ghisellini et al., 2016) on the other, an increasing amount of studies argues that the circular economy can have multiple benefits on society and the labor market (for example, (Wijkman and Skaynberg, 2015; Webster, 2017). The previous collaboration between Brussels, UNEP, the World Council on City Data, and Ecocity Builders (UNEP, 2019) highlighted the need to ensure that these socio-economic inequalities are not mirrored in the circular economy transition. Notwithstanding its potential benefits, workers rights need to be safeguarded and the workforce needs to be skilled and reskilled to get the most out of this transition (Circle Economy, 2020).
Thus, the impact of the transition toward the circular economy on the labor market needs to be measured and linked to the associated resource impacts, to develop an understanding of both the environmental and societal impacts of the circular economy.
Several institutions worldwide have started developing methods to measure the transition toward a circular economy.
The European Union has developed a monitoring framework for the circular economy which includes a list of 10 indicator sets to “capture the main elements of a circular economy” (EC, 2020a) These indicator groups are production and consumption indicators (such as EU self-sufficiency for raw materials), waste management indicators, and competitiveness and innovation indicators (which include “private investment, jobs and gross value added related to circular economy sectors,” and specifically persons employed in these sectors).
The indicator framework being developed by the OECD is meant to evaluate the circular economy strategies in cities and regions. Their proposed indicators include both environmental and economic measures. The Stockholm Environment Institute is currently drafting its urban circularity assessment framework (SEI, 2020), while the Platform for Accelerating the Circular Economy (PACE) has convened key stakeholders, and works to harmonize approaches to measuring the progress and impacts of a circular economy transition (PACE, 2021). The United Nations Environment Programme (UNEP), Ecocity Builders, and the World Council on City Data (WCCD) worked with Brussels Environment to develop a framework to improve monitoring of the city-region's circular economy plan (UNEP, 2019).
There is consensus among experts that harmonization of tools and measures are critical to an effective and just circular economy transition. The OECD's indicator framework is targeted, for example, at a more coherent application of circularity among its member countries. There is also a shared understanding that alignment, through platforms like PACE, may bring about better results. Yet, in spite of ongoing efforts, there remains a clear gap in measuring overall circularity at city level.
There is global demand for the adoption of appropriate tools to measure and monitor the transitions toward Circular Economy (Jacobi et al., 2018; Kalmykova et al., 2018; Arruda et al., 2021; Martinho, 2021; Papageorgiou et al., 2021). This paper aims to be a contribution toward the definition and adoption of monitoring measures at a local level. Vanhuyse et al. (2021) argue that the current discussion of Circular Cities has a limited focus on informal sector in low and middle income countries and on governance issues related to waste management. With this paper, we aim to contra balance this bias on the existing body of literature by adding the quantification of Circular Economy Jobs at a local level. Any holistic framework for Circular Economy needs to integrate sustainability, resilient, as well as social issues (Suárez-Eiroa et al., 2021). A focus on consumption patters, as well as citizen inclusion in the current CE discourse is still a gap in current research (Schggl et al., 2020). By including a component describing local jobs attached to Circular Economy activities, we are able to integrate an important social issue.
The second component addressed, in this paper, is on material consumption of specific sectors, moving the end-of-pipe discussion toward overall material consumption, and specifically material import. End-of-pipe solutions for local actions are only “short sight options” and not likely to translate into “a sustainable trend” (Santagata et al., 2020). Jacobi et al. (2018) also support this idea on their research, favoring a shift of focus from end of life recycling strategies toward a more integrated approach. The presented analysis showcase an integration of material consumption and a social component, described via available local circular-jobs.
This paper presents a methodology that describes the circularity of all economic sectors in a city and tracks the number of workers engaged in the circular economy. The proposed method measures employment related to the circular economy by applying the concept of the “key elements of the circular economy” (described below), as well as raw material consumption. As such, the method considers both the circularity of activities and material use by sectors.
The end result, a “circular economy job measure,” is an entry point to articulating circular economy pathways, which can help city leaders make the case for a circular economy.
As mentioned in the introduction, there are two complementary frameworks that shape the method presented in this paper: (1) employment related to the circular economy (we use the shorthand “circular jobs” in this paper) are defined by means of the “key elements of the circular economy,” which we shall elaborate on below; (2) circularity of the economic sectors is measured through material use. The first provides insight in the intent of implementing circular economy strategies, while the second provides insight into the impact of these activities on overall material consumption and consequently on the environment. This section elaborates on these conceptual foundations of the methodology.
The city of Recife, with an estimated population of 1.6 million and an employment rate of 43.9% is2 the capital city of the Brazilian state of Pernambuco. It is also the heart of the fourth-largest urban agglomeration in Brazil and serves a metropolitan population of about 4 million, many of whom work in the city. A network of rivers largely defines Recife's urban form.
Brussels in this report refers to the Brussels Capital Region (BCR), a metropolitan area that includes 19 municipalities3, including the City of Brussels, which is the capital of Belgium. The BCR, when compared to Recife, is smaller in terms of land area and population. But beyond the over 1 million population of the BCR, the metropolitan area also serves the wider urban area extending toward Ghent, Antwerp, Leuven, and Walloon Brabant, which is home to over 5 million people. BCR has an unemployment rate of 17.8%4.
The choice of Recife and Brussels to demonstrate the combined methodology emerged from various discussions and a list of criteria co-developed between UNEP and Circle Economy. We wanted to work with:
1. one city in the global north and another in the global south.
2. cities in our existing pool of cities—those with whom we had a familiarity in terms of the data availability and data management framework. Both Recife and Brussels had the appropriate data “ecosystem” favorable for this type of analysis. UNEP worked with both cities in previous occasions on resource flows and urban metabolism. Circle Economy also worked with Belgium (including Brussels) on circular economy jobs.
3. cities where we have a good understanding of the local context5 and where there is a local partner willing to help if necessary.
Data for this exercise came from the following sources:
• National monetary IO Tables were sourced from EORA, as were the environmental extensions that were used to create the Material Import Dependencies.
• Employment data was sourced from local partners. In the case of Brussels, we sourced the data from Graydon; in the case of Recife we sourced the data from the national statistical office.
In an effort to develop a common understanding of the circular economy, the Circle Economy research team continuously consolidates expert knowledge and literature about the characteristics activities of a circular economy, resulting in the Key Elements Framework (see Table 1).
The Key Elements framework is a conceptual framework of eight elements of circularity that can be applied at different intervention levels (for example, national, regional, city sector, business, product, process, or material) toward defining a strategy to transition to a circular economy.
The KE framework consists of three core elements and five enabling elements. Core elements deal with physical flows directly, whilst enabling elements deal with creating the conditions or removing barriers, for a circular transition6.
The core elements serve to narrow (more efficient use of resources), slow (multiple use of resources), close (use of wasted resources), or regenerate (use of reusable resources) material loops (Bocken and Short, 2016). The enabling elements, then, serve to overcome barriers to mainstream, scale up and accelerate core elements.
The Key Elements identify the majority of terms linked to the circular economy, covering the activities, strategies, and interventions used to promote greater levels of circularity. They do not include impact areas, such as clean air or resource use.
The KE are summarized on Table 2 below.
The KE Framework serves as the basis to classify sectors according to core or enabling circular strategies. Sectors that are neither core nor enabling are classified as indirectly circular sectors. For a full export of sector classifications (see, Annex I A.1). We also take the material use of sectors into account in this analysis, as described in the next section. With this new classification, and lens on material use of sectors, we reorganize economic input output tables and study the interaction between core, enabling and indirect sectors.
The second framework in this paper looks at quantifying circularity of material use. Given the challenge of doing this quantification, most cities that have attempted to measure circularity have turned to urban metabolism approaches, mainly through methodologies that have been developed and applied in academia.
One of the most used approaches is to undertake an Economy-Wide Material Flow Analysis (EW-MFA). This type of analysis looks at the entire economy of a city-system and attempts to quantify all material flows within the system. One of the earliest urban EW-MFA studies was done in Brussels and published in 1977 by Duvigneaud (1977). It quantified energy, various physical material inputs, and local extraction, as well as emissions, exports, and other outflows to account for all material flows within this system. These studies were often visualized in the form of Sankey diagrams7. The use of Physical Input-Output tables for the description of material flows within urban systems has also been applied by the academic body (see Liang and Zhang, 2011; Zhang et al., 2014). However, due to its large scope, this is also often the most time-consuming methodology, and it is directly bound to the amount and quality of the data available.
Another approach would entail focusing on a specific group of materials or a single flow and to undertake a Substance Flow Analysis (SFA). Examples of this work could be a city-wide water metabolism study or an analysis of the phosphorus cycle through an urban-system. A counterpart to the SFA method is the more classical Life Cycle Analysis (LCA) approach, which focuses on one specific process in detail, for example, the flow description of nickel-cadmium batteries. This approach is often most useful for industry and product LCA.
Data collection is by far the most challenging aspect of UM. On an urban level, resources flow much more freely than on a national scale. The actors involved in monitoring this information can also be difficult to identify– if a monitoring scheme exists at all– and information is often scattered. In many cases, data has to be collected at the local government level, retrieved from private repositories of academic institutions, or expensive “gray-box-data” from the private sector.
The work of UNEP acknowledges that many medium-sized cities in the global south face a systematic problem with data collection and consequently in the adoption of urban metabolism approaches. This is, however, where circular economy needs to happen. We know that urban agglomerations with the highest population growth rate are medium and small cities often with a population lower than 1 million (UN, 2015).
UNEP has addressed this lack of data by developing a model that, in the absence of measured-data, would give city leaders a robust estimate as a starting point in understanding their material flows. This model, called the Spatial Microsimulation Urban Metabolism (SMUM8) tool, combines two powerful approaches for the simulation of resource flows within cities: spatial microsimulation (SM) and urban metabolism (UM).
The SMUM tool creates a representative “synthetic” population that provides insights on individual groups in the population, broken down for instance by income, education, age, or household size (Munõz, 2018). UNEP then constructs local level decarbonization and decoupling scenarios using the synthetic population. Through this modeling tool, the city can have a snapshot of how geographic/social clusters of the population may be affected in any economic transition, thus, helping in city-planning.
The methodology used in this paper is essentially a simplified version of the EW-MFA. It still tries to capture resource use but instead of using sankey diagrams, detailing the flow of resources in and out of the city, it uses Input-Output tables fed by proxy local data.
The decision to combine the two approaches resulted from various discussions between UNEP and Circle Economy. These conversations and analytical reviews revealed that the circular economy approach was helpful for city practitioners in planning their circular economy strategy, but did not really make a strong, quantifiable connection to global material use and its potential environmental impacts. Conversely, the method proposed by UNEP was most relevant to national governments seeking to capture the gains of a circular economy at the local level to their overall environmental strategy, but it left the local implementation open, and did not provide immediate local level transition pathways. The proposed combined method does both. It quantifies circular economy jobs at a single point in time and at an aggregated level (by economic sector). This gives insight into the current state and allows for the identification of relevant economic sectors to further accelerate the transition toward a circular economy.
The entire process can be summarized in Figure 1 below, and then discussed in detail further below.
One of the premisses for the construction of this model was that the required input data to feed the model should be easily accessible for as many cities as possible in order to: (a) leave no city behind (Acuto and Parnell, 2016) and (b) break the cycle of systematic-bias of urban-science toward large agglomeration of the global north (Creutzig et al., 2019). The presented method requires only 3 datasets, two of them should be available only at a national level. The third input data-set is the hardest of all three to get. In order to downscale the national-level IO table (input one) to the city level, we require: (a) employment data at a national-level (input two) and (b) similar constructed employment data at a city level. By far, the most difficult input-data-set to get is the city-level employment data. Working with many cities, we have identified the national level micro dataset behind the national labor force survey to be the most suitable dataset to extract this type of information.
The model modifies the final demand vector, as estimates for circular final demand. The output required by industry to meet this circular final demand is calculated using the Leontief Inverse, and is our proxy for circular output of the economy. To determine circular activity per sector we take the proportion of this circular output over the original economic output, and we apply this proportion to the number of jobs in the sector to determine the circular employment. Therefore: a circularity of 10% defines that 10 percent of all local jobs are circular.
This method is different to simply counting the number of circular jobs according to whether they directly work in circular sectors (for instance the number of jobs in maintenance and repair) because it also counts the interdependency of that sector in the context of the economy. For instance, the more sectors the repair industry interacts with (supplies to and purchases from) then the higher the proportion of output will be required to meet a given level of demand. Furthermore, the more the manufacturing industry, for example, interacts with the repair industry then the model will result in a higher proportion of circular jobs in that industry too.
The estimates for circular final demand per sector are dependent on combining the two frameworks described above. The sectors organizing the employment data and the IO table are categorized into core, enabling, and indirectly according to the Key Elements framework, and assumptions are applied per each category. The MID coefficient per sector is determined from the national level Physical IO tables, and used in the estimation of circular final demand of indirectly circular sectors—which are typically the materially intensive sectors.
More detail about the assumptions to estimate circular final demand can be found below.
A. GATHER AND PREPARE DATA
Here are the steps we took in order to gather data and proceed with the calculation
We gathered the following data:
• Macro-economic data:
– National IO Tables: all national IO tables are retrieved from EXIOBASE Tukker et al. (2009, 2011, 2013); Stadler et al. (2014); Wood et al. (2014); Stadler (2018)9
– National Population
– Regional/City Population
• Employment data:
– National Employment data at granular sectors level (Labour force survey)
– Regional/City Employment data at granular sectors level10
• Material data
– National RME data11
To facilitate replicability, we used both national level employment data and demographic data from national statistics agencies. We then used EORA12, a global database estimating emissions and resource extractions by industry, to populate our national level input-output tables. In the case of Brazil and Belgium, the databases of the statistics agencies were free, but this may not always be the case in every country.
As a precursor to the calculation, all economic sectors are classified into either core circular sectors, or enabling circular sectors as per the previous section on the key elements of the circular economy (see Appendix III for a full list of sector codes with classifications).
We scale down our national datasets to regional scale using the socioeconomic data as scaling factors. We use employment data to scale down the IO table.
Employment coefficient of a sector
Population coefficient of a city
Standard IO Table
Interindustrial
For i=j=1 to n number of sectors represented in the national IO table
Final Demand
For i=1 to n number of sectors represented in the national IO table
Expanded IO Table
B. CALCULATE MATERIAL IMPORT DEPENDENCY / SECTOR
We proceed to estimate the circular final demand of all the sectors.
This is a three-part calculation, one for each typology: core, enabling, and indirect. Our model hypotheses that circularity takes on a different form depending on the sector in question13.
Array of CE coefficients
Mc (Core)
Me (Enabling)
Md (Indirect)
Core CE coefficients
if Zi is a core sector
Enabling CE coefficients
if Zj is an eanabling sector
Indirect CE coefficients
if Zi is an indirect sector
(a) Circular activity in core circular sectors: all activity in core sectors.
Core sectors are those that are agreed upon in the literature as being key to the circular economy, and includes sectors such as waste management, materials recovery, generation of renewable energy, repair services, etc. The proposed method assumes that all activities and output of these sectors are 100% circular.
(b) Circular activity in enabling circular sectors: the extent to which enabling sector services are utilized by core circular sectors.
Enabling sectors are those sectors that remove barriers for core circular activities, and include sectors such as digital services and design. The proposed method therefore calculates the proportion of circular activity within an enabling sector as the proportion of enabling sector services that are utilization by the core sectors over the total utilisation of these services by other sectors.
(d) Circular activity in indirectly circular sectors: the extent to which indirectly sectors utilise core sector services, and the materials are not imported (i.e., with the material multiplier described above applied to the sector in question.)
Indirectly circular sectors are all other sectors that do not play a direct role in furthering the transition to the circular economy, yet can adopt circular practices, such as the agriculture and manufacturing sectors. The proposed method, therefore, calculates the proportion of circular activity within an indirectly circular sector by deriving the total core circular services.
(f) Linear activity in all sectors: all activity in sectors that promote a linear economy.
Linear activities refer to activities like mining, oil extraction, and other High CO2 Emitting sectors.
C. REBALANCE ECONOMY
Material Import Dependency
RMEimp Imported Raw Material Equivalent
RMEcba Domestic Consumption RME
RMEexp Exported Raw Material Equivalent
In this step, we use our Circular Final Demand vector and the original interindusrial matrix, and apply the Leontief Inverse to obtain the “Circular” output required by industry to meet Circular Final Demand. This circular output vector is divided by the original output vector to obtain a proportion of circular output in the sector.
In this step, we integrate the material multiplier with the indirect activity one and converted to a single multiplier to indicate circularity per sector (see Equation 12).
With help of the Leontif inverse matrix (see Equation 14), we estimate the share of circular economy jobs per economic sector (see Equation 16).
Indirect CE coefficient by MID
A matrix (coefficients)
Calculate the Leontief L
Get dx final demand vector
Final Circular Coefficient
The resultant vector described above is in the range of 0 to 1 and is applied to raw data on employment per sector in the city. The resultant number of jobs is considered the circular jobs in the city, and can be analyzed by sector, by key element, or in aggregate for the region. The multiplier is applied as a simple multiplication to the original employment numbers (see Equation 17).
Final CE Jobs Vector
Computation steps represented through IO tables. The first row of Figure 2 show the original IO input tables for Belgium (used for Brussels) and Brazil (used for Recife). By observing both IO tables, we see that Brazil has a more diverse economy that the one from Belgium. The second row, showing the MID values for both countries, show a much higher import dependency for the country of Belgium. Sectors: Maintenance and Repair, Wholesale Trade and Retail Trade have the highest MID values in Brazil, while in Belgium: Mining and Quarrying has the lowest MID.
The third row of the table of Figure 2 shows the downscale/expanded city level IO table. This IO table represents the city level economy. On these IO tables we see again that the economy of Recife is more diverse than the one of Brussels.
One of the strongest arguments made against this method among its developers what the downscaling process of data to city level. The mayor issue with this downscaling process was that one of the computation input data-set is not scalable to this level. This fact creates a computational bias toward large countries with low international material dependencies. This is the case of Brazil. In the following pages, we present a simple comparison of results by interchanging the “home” country-input-dataset (Brazil for Recife and Belgium for Brussels).
This comparison is interesting because we can see how national level context influences the city level performance. When using the Brazil input data, both cities achieve a higher circularity measure (see Figures 3, 4), proving so, the existing bias toward large economies. In theory, we could use the quantification of this bias to calibrate the model. Nonetheless, in this section we only present a simple comparison of the cities, taking advantage that we only discuss the computation of two individual cities (see conclusion for a word on future steps and on how we aim to use this comparison method for future research).
On the other hand, if we contextualize both cities on a small, high income, net-importer country, both cities have a lower circularity measure. This indicated, to an extent, that it is harder to become “national-level” circular if the city is located within the boundaries of such a country. As part of our future research, we aim to define circularity at the subregional level. Following this argument, it might be easier for cities to achieve circularity at a subnational level (outside the scope of this research).
In this paper, we opt to analyse each city on its own context, acknowledging this bias, we do not recommend using this method for a purely comparative analysis between cities. We nonetheless see this section as a calibration step because it allows us to interpret the numbers correctly. If we would to compare only the home context of cities (9.96% for Recife and 8.44% for Brussels) we might conclude that Recife is a more circular city. This might in fact be an error when comparing the both cities under the same national context. The net difference between both cities might (more research is needed to make this claim yet) in fact be exactly the opposite, making Brussels the more circular one (see Table 3).
The results of the combined methodology reveal that the percentage of circular economy jobs in Brussels and Recife are 8.51% and 9.96% respectively (Figure 5). A further analysis of these results will show that these numbers serve not as a basis of comparison between two very different contexts such as that of Recife and Brussels but rather as a starting point for the city to improve. Through policy development and action, cities can track their circular economy transition through this method.
Interpreting the numbers
Table 4 shows the aggregated number of circular jobs by EORA activities for the city of Recife. Education, Health, and Other Services is the economic activity with the highest number of circular jobs in the city, with a total share of 27% of all circular jobs. Together with the second-largest economic activity, Financial Intermediation and Business Activities, the first two EORA economic activities hold a share of 50% of circular jobs. Both sectors hold a 47% share in the city of Brussels, but at an inverse ranking, with Financial Intermediation and Business Activities at the top position (see Table 5) This indicates that should these sectors adopt circular strategies there will be a prominent trickle through effect into other sectors that are interdependent with them. The economy is, in a sense, centred around these sectors, and they are well positioned to operate as the driving force.
Table 4. (top-20) Economic sectors for Recife (9.96%) sorted by total number of circular economy jobs aggregated to EORA classification.
Table 5. (top-20) Economic sectors for Brussels (8.51%) sorted by total number of circular economy jobs aggregated to EORA classification.
Retail Trade (16% rec, 9% bru) and Transport (5% rec, 7% bru) are two sectors that are present in both cities, with still a representative share. This indicates a potentially and relative easy growth increase in circularity through these sectors. These economic sectors are both important providers of local jobs (176k jobs in Recife and 109k jobs in Brussels) and still with a low circular share. This circular share can be increased, in the Transport sector for example, by increasing the interaction between the core circular sectors like waste management and materials recovery and the transport sector—i.e., increasing the redistribution of waste in the local economy.
Hotels and Restaurants (4% rec, 4% bru); Construction (4% rec, 3% bru); and Wholesale Trade (5% rec, 5% bru) are on, or below, the 5% mark of circular jobs. We interpret this as sectors that are relevant for the local economy, and with a high potential to become more circular. These sectors, for the most part, are indirectly circular sectors that are also considered through the lens of material import dependency. Low results here preliminarily indicate two transition pathways—one to increase the proportion of inputs from core circular industries (i.e., to favor repair services, regenerative energy, or materials recovery over the alternatives), and two to increase the proportion of inputs from regional economies (i.e., to shorten supply chains).
Maintenance and Repair (3% rec, 3% bru) is an economic sector that provides more local jobs in Recife (21k) than in Brussels (11k), but in both cases have a marginal effect on the overall circularity of the city. This is a core sector, and so can increase circularity in the city not only through the increase in the volume of employment but also through establishing greater interdependency between this sector and other (prominent) sectors in the economy.
For the city of Brussels, important sectors are: Public Administration (1% rec, 6% bru); and Post and Telecommunications (1% rec, 6% bru). These results reflect the well known fact that Brussels is a centre of public administration, and indicate that these sectors could have a more influential role to play in the context of Brussels than in Recife.
In Recife, Agriculture (<1% rec, <1% bru) is an interesting sector to look at, even if the sector currently provides a low number of local jobs (3k in Recife) it has a high share of circular jobs, 11% of all local jobs in Agriculture are considered circular. This share is the highest share in the city of Recife. Considering Agriculture is an indirectly circular sector, and considered through the lens of material import dependency, this high result indicates that much of Agriculture is taking place nationally. For the city of Recife, there is the opportunity to grow the volume of jobs, namely circular jobs, which can interact with the large Agricultural national economy—for example through establishing strong rural urban links, building local supply chains and stimulating local businesses which utilise local produce and/or process local organic waste to return to agricultural land for nutrients. These activities could potentially even bring about increased food security for the city of Recife and which An increase of local jobs in this sector would be a low-hanging fruit to achieve a higher circularity measure.
The analysis at this level of detail gives us an idea of the current status of the city and the possible macro-level interventions that can be implemented at a city level to foment the growth of specific economic sectors. This analysis can be well aligned to national level policies and be integrated with other macro-level analysis. Another advantage of presenting the results at this level of aggregation is the homogeneity of the results, making them comparable.
Nonetheless, this level of detail is not enough to think about more meso-level policy implementations. Tables 6, 7 show the top-10 number of circular jobs at the lowest available aggregation level. This level varies from city to city, depending on which level the input data is available. In this case, the data resolution of Brussels provides a much lower aggregation level. A direct comparison between cities at this level of detail is therefore much more complex.
Table 6. (top-10) Economic sectors for Recife (9.96%) sorted by total number of circular economy jobs.
Table 7. (top-10) Economic sectors for Brussels (8.51%) sorted by total number of circular economy jobs.
Table 6 shows the top-10 number of circular jobs for the city of Recife. The table present at the top-10 almost only service oriented activities. Nonetheless, on this table, we also see the construction of buildings making the top-10 ranking.
Table 7 shows the top-10 number of circular jobs for the city of Brussels. Similar to Recife, we see here almost only service-oriented sectors. We see many administration jobs, management, and restaurants.
The Tables 4–7 illustrate two important findings of this study.
1. This is not a benchmarking tool, or at least not for cities of completely different profiles.
The results show that the number of local jobs of particular sectors clearly define the top of the list. The ranking therefore indicates the type of city. The results also demonstrate that because the number of local jobs in a specific sector carries a significant weight in the calculation, it does not make sense to compare two cities with as different employment profiles as Recife and Brussels. It may, over time, be possible to develop city typologies and compare circular economy trajectories within a group of cities with the same economic sector profiles, and population demographic.
2. Circular economy at city level cannot be achieved by the city alone.
Local activities do not happen in a vacuum, but are rather affected by national level policy and decision-making.
We imagine the CE jobs measure to be a tool for an informed discussion. We hope that stakeholders, including national government and private sector, will use this to better support a city in its aspiration to become more circular.
A third important finding in this study that was inferred from but not shown in the tables above is the correlation between the material multipliers (MID coefficients) and absolute number of local jobs. Except for coefficients close to zero or no values and outliers, a correlation between MID coefficients and the number of jobs exists.
Correlation, of course, does not mean causality. This relationship is a reflection of our current economic system, where larger economic sectors demand equally large amounts of resources, and at the same time provide the most jobs. This makes big multinational companies attractive to cities. A circular scenario, however, will contradict this relationship and would presumably promote a “decoupling” of both variables. A shift in this correlation can therefore be an additional indicator of circularity.
Petit-Boix and Leipold (2018) suggest on their work to increase the attention given to Circular Economy toward social consumption and urban planning. The work presented in this paper deliver a small contribution toward both points. By including desegregated information coming from a labor force survey, we are de facto including all attached demographic data of individuals to the model. By including demographic data into the model, we are also able to estimate consumption patterns at a micro level (outside the scope of this research). With some more detail data we could, theoretically, develop spatial models that help the development of urban planning, by monitoring the transition at a municipal level. A clear advantage of increasing the spatial detail of this analysis is that one could develop individual transition strategies for the specific needs of the municipality, as proposed by Bassi et al. (2021) and Heshmati and Rashidghalam (2021).
As repeatedly mentioned in this paper, we see the CE jobs measure as a first step. UNEP and Circle Economy, under a broader consortium that includes ICLEI-Local Governments for Sustainability (ICLEI) have started to expand the methodology using UNEP's Spatial Microsimulation Urban Metabolism (SMUM) modeling tool (Munõz, 2018). Using a combination of SMUM and proposed policy actions recommended by the government, an expanded version of the methodology will be used to create future scenarios and gain insight to the city's circular economy trajectory. The consortium aims to expose these results through existing digital products servicing city consultants and policy makers—the Circle City Scan Tool and the Circular Jobs Monitor14.
The current methodology takes the population and employment data at an aggregate level and is not able to capture the effect that policy can have on marginalized groups. With SMUM, the researcher can identify segments of the population and policy decisions can be assessed at a more granular level, particularly on how they would impact specific sectors of the population. Identifying key sectors and understanding the levers of a circular economy would then support a local government in deciding where to concentrate limited resources for strategic, bottom-up data gathering.
Along the same lines of trying to capture a just transition to a more circular economy, UNEP and Circle Economy faced challenges in including informality in this methodology. With little to no data available on this topic, measuring the impact of the circular economy transition on this vulnerable section of the population becomes difficult. Not having data on the informal sector thus skews the available data on employment. The databases used in this analysis do not include any information related to the informal sector. Thus, jobs in sectors that would otherwise be contributing to the circular economy (particularly those referenced as “core sectors”) are not captured in the analysis.
Employment data used for Recife, for example, is arguably incomplete because informality in Recife's labor market was not taken into account. This includes not only those who are full-time informal workers but also those who are working formally but are engaging in informal work on the side (e.g., small entrepreneurs selling food). The results of the computations are, therefore, heavily skewed toward sectors that have a low degree of informality. Especially when analysing employment in the circular economy, it is imperative to take informal workers into account, as informality is mostly present in agriculture, waste management and the lower end of manufacturing (ILO, 2018).
The researchers are working on several tools to try and include informality in the equations. We expect that this work can only provide an indicative figure based on literature, but ultimately the number of the informal sector in urban areas has to be calibrated to local definitions of informality and be built around a political discussion with city officials. We are currently piloting this expanded work, looking at the inclusion of informality in Bogor, Indonesia, where the informal sector is estimated to account for 34 percent of the working population.
One of the main considerations in this work is the availability of data, and the issue of data-scarcity that most impacts global south cities. To “leave no city behind” (see (Acuto and Parnell, 2016) we need to develop methods that can be applied across the large range of cities around the globe.
With the aim of implementing this method in both data-rich and data-scarce cities, this paper used downscaled data from available national-level datasets. This process has largely been aided by existing global data management solutions like EXIOBASE and EORA. There is an intention to continue to encourage national governments to use and populate these existing tools.
But even this simplified process requires a minimum set of city-level data. What would be helpful is that as this exercise is done in more cities, we would be able to develop city typologies and thus when even the minimum data needed is not available, they can be “replaced” by proxy data from similar cities (see (Creutzig et al., 2015; Rosado et al., 2016).
Local governments have the ability to accelerate the adoption of Circular Economy policies at a national level, in the case of global south cities the intervention of governments for the adoption of CE concept plays a very important role (Patwa et al., 2021). A transition toward a Circular Economy cannot depend on government actions alone, pressure from civil society and behavioral change from consumers plays also a significant role in this transition (Marchesi and Tweed, 2021; Patwa et al., 2021). Future work by this research team aims to integrate skill levels and occupational characteristics to this analysis in order to identify gaps in existing local skill structures, acknowledging the importance of human capital for the transition to a Circular Economy (Dagilienė et al., 2021).
This paper successfully demonstrated the effective application of an environmentally extended Input-Output analysis at a city level, which combined the two approaches developed by UNEP and Circle Economy into one CE jobs measure. The process of getting to this measure provides useful information in the development of a local circular economy strategy, including:
• The progress a city has made toward the circular economy using jobs as a proxy indicator.
• The relationship of the city's economic sectors and the national material import dependencies.
• Granular employment data on each economic sector, allowing the decision-makers to identify low-hanging fruit, and where they can most impact circular economy at city and national level.
• A circular economy job measure, which connects local and national circularity and serves as a baseline to track improvement in the city's economic transition.
The presented methodology on this paper proposed the use of a national level multi-regional environmentally extended input output table (MRIO) and an extract of the labor force survey, describing local employment, for the construction of a city-level circularity measure. This measure is computed by combining both datasets and quantifies as the number of local circular jobs on the city. This is achieved by classifying all economic sector by its circular activity and using material import dependencies to identify the resource dependency of individual economic sectors. The main advantage of this approach is that both required datasets are available in most cities around the globe, this is important for us to include cities with a low data infrastructure.
The obtained results from this approach represent the circularity of a city by quantifying the total number of existing local circular jobs. In the case of Brussels, 8.51% of all existing local jobs are considered circular, while in Recife 9.96% of all local jobs are considered circular. This measure indicates, on the one hand, how circular a city economy is, and on the other hand, shows the potential that a city has for the creation of new local circular jobs. While the circular measure, as stated before, should not be used to compare cities, the ranking of economic sectors can serve cities to identify key economic sectors to accelerate the transition to a circular economy.
Possible transition paths to a circular economy are city-specific, and therefor the presented method does not aim to find generic solutions, but aims to empower cities to develop their own strategies by understanding their underlying economic system. The decision to place local jobs at the center of the discussion allows it to focus this discussion on the residents from those cities, making it easier for cities to understand possible transition pathways and ensure a just transition for everyone.
Like many other existing pieces of work on indicators and circular economy, the circular economy jobs methodology contributes to the development of a shared understanding of circularity. It however takes an extra step by focusing on jobs and making the human aspect of the circular economy more visible. Focusing on employment allows cities to plan for reskilling and upskilling. The focus on the number of jobs and those “unemployed” also forces decision-makers to acknowledge who is most affected by a circular economy transition.
Because of its potential as an indicator for a just circular economy transition, the methodology presented in this paper also contributes to international agreements such as the Sustainable Development Goals (SDGs) and the New Urban Agenda. The methodology provides a way to quantify country gains on SDG 12 Sustainable Consumption and Production, thus helping overall reporting on the SDGs. It can also add to the narrative of SDG 8 on decent work and economic growth, and SDG 11 building sustainable cities and communities. At the same time, it would also help city leaders in fulfilling the goals of the three transformative commitments of the New Urban Agenda.
While the focus of this paper, the circular economy jobs measure, is narrow, we believe there are opportunities to expand and develop further. There are opportunities to better understand the role of informality, there are ways to create typologies to ease data scarcity issues, and we can work with cities to understand how policy interventions can be used to improve their CE jobs measure.
A lot can be quantified, but we also recognize that much of what will happen next would be a conversation. One that will hopefully lead to political decisions that are as good for the planet as they are for the economy.
The data analyzed in this study is subject to the following licenses/restrictions: Local employment data for the city of Brussels need to be requested, all other data is public available. All data sources are described on the submitted annex. Requests to access these datasets should be directed to https://www.worldmrio.com/eora26/.
All authors listed have made a substantial, direct, and intellectual contribution to the work and approved it for publication.
The authors declare that the research was conducted in the absence of any commercial or financial relationships that could be construed as a potential conflict of interest.
All claims expressed in this article are solely those of the authors and do not necessarily represent those of their affiliated organizations, or those of the publisher, the editors and the reviewers. Any product that may be evaluated in this article, or claim that may be made by its manufacturer, is not guaranteed or endorsed by the publisher.
The Supplementary Material for this article can be found online at: https://www.frontiersin.org/articles/10.3389/frsc.2021.787076/full#supplementary-material
1. ^When computing the national MID, we obtained a 0 result for multiple sectors in both analyzed countries. Whether this is a consequence of missing data, incorrect data, or if the MID actually equals 0 is unclear.
2. ^https://cidades.ibge.gov.br/brasil/pe/recife/panorama
3. ^https://en.wikipedia.org/wiki/Brussels#Municipalities
4. ^All data retrieved from http://bisa.brussels/bestanden/publicaties/bru19/bisa_kc_Brussel.pdf.
5. ^The case studies done by UNEP on these two cities are available at: https://resourceefficientcities.org/cities/.
6. ^https://www.circle-economy.com/resources/the-key-elements-of-the-circular-economy-framework
7. ^A Sankey diagram is a flow diagram showing the different resource flows using arrows, whose widths are proportional to the size/rate of the flows.
9. ^Other available data sources include: WIOD Timmer (2012), EORA Lenzen et al. (2012, 2013); Moran and Wood (2014); Kanemoto et al. (2016); Moran et al. (2018); and GTAP Aguiar et al. (2019).
10. ^Data sets required for the projection of populations (population census or registers) are available for 83 per-cent of the global population UN-DESA (2016) including labor force participation.
11. ^Regionally-benchmarked EU available data on RME, see (Liang and Zhang, 2011) and (EUROSTAT, 2014) can be used, in this paper we use satellite material data from EORA.
13. ^Note: Sectors must be at a level where they fit into one category. Otherwise, estimates must be applied to generate n proxy sectors from 1 sector, each within core or enabling or indirectly. This is problematic because the difference between core and indirect might be at a low aggregation level, as is the case of renewable energies. Assuming a uniform distribution among sectors for the allocation of proxy sectors might indeed be a computational error. A possible solution for the mitigation of this problem might be the use of existing distributions from a subnational distribution from a similar city, or conducting surveys and bottom up data gathering to make an informed estimate.
Aguiar, A., Chepeliev, M., Corong, E. L., McDougall, R., and van der Mensbrugghe, D. (2019). The GTAP data base: version 10. J. Glob. Econ. Anal. 4, 1–27. doi: 10.21642/jgea.040101af
Arruda, E. H., Melatto, R. A. P. B., Levy, W., and de Melo Conti, D. (2021). Circular economy: a brief literature review (2015–2020). Sustain. Oper. Comput. 2, 79–86. doi: 10.1016/j.susoc.2021.05.001
Bassi, A. M., Bianchi, M., Guzzetti, M., Pallaske, G., and Tapia, C. (2021). Improving the understanding of circular economy potential at territorial level using systems thinking. Sustain. Prod. Consumpt. 27, 128–140. doi: 10.1016/j.spc.2020.10.028
Bocken, N., and Short, S. (2016). Towards a sufficiency-driven business model: experiences and opportunities. Environ. Innov. Soc. Trans. 18, 41–61. doi: 10.1016/j.eist.2015.07.010
Circle Economy (2020). Circularity Gap Report The Netherlands: Closing the Circularity Gap in The Netherlands. Technical Report, Circle Economy, Amsterdam, The Netherlands.
Creutzig, F., Baiocchi, G., Bierkandt, R., Pichler, P.-P., and Seto, K. C. (2015). Global typology of urban energy use and potentials for an urbanization mitigation wedge. Proc. Natl. Acad. Sci. U.S.A. 112, 6283–6288. doi: 10.1073/pnas.1315545112
Creutzig, F., Lohrey, S., Bai, X., Baklanov, A., Dawson, R., Dhakal, S., et al. (2019). Upscaling urban data science for global climate solutions. Glob. Sustain. 2:e2. doi: 10.1017/sus.2018.16
Dagilien, L., Varani, V., and Bruneckien, J. (2021). Local governments perspective on implementing the circular economy: a framework for future solutions. J. Clean. Prod. 310, 127340. doi: 10.1016/j.jclepro.2021.127340
Duvigneaud, P., and Denayeyer-De, S. S. (1977). “Lecosysteme urbs, in lecosystemeurbain bruxellois, in productivite en belgique,” in Traveaux de la Section Belge du Programme Biologique International, eds P. Duvigneaud, and P. Kestemont (Brussels), 581–597.
EC (2020b). Monitoring Framework: Circular Economy Indicators. Technical Report, European Commission, Brussels.
Eurostat. (2014). Estimates for Raw Material Consumption (RMC) and Raw Material Equivalents (RME) conversion factors. European Statistical Office, Luxembourg. Available Online at: http://ec.europa.eu/eurostat/documents/1798247/6191533/RMEproject-Introduction-Oct2015.pdf/041dd5c9-e691-4123-badb-d1ed3686c08c
Fischer-Kowalski, M., Swilling, M., Weizsacker, E. V., Ren, Y., Moriguchi, Y., Crane, W., et al. (2011). Decoupling Natural Resource Use and Environmental Impacts From Economic Growth. Technical Report, IRP, United Nations Environment Programme, Nairobi, Kenya.
Ghisellini, P., Cialani, C., and Ulgiati, S. (2016). A review on circular economy: the expected transition to a balanced interplay of environmental and economic systems. J. Clean. Prod. 114, 11–32. doi: 10.1016/j.jclepro.2015.09.007
Heshmati, A., and Rashidghalam, M. (2021). Assessment of the urban circular economy in sweden. J. Clean. Prod. 310, 127475. doi: 10.1016/j.jclepro.2021.127475
ILO (2018). Decent Work and the Sustainable Development Goals: A Guidebook on SDG Labour Market Indicators. Technical Report, International Labour Organization, Geneva.
Jacobi, N., Haas, W., Wiedenhofer, D., and Mayer, A. (2018). Providing an economy-wide monitoring framework for the circular economy in austria: status quo and challenges. Resour. Conserv. Recycling 137, 156–166. doi: 10.1016/j.resconrec.2018.05.022
Kalmykova, Y., Sadagopan, M., and Rosado, L. (2018). Circular economy from review of theories and practices to development of implementation tools. Resour. Conserv. Recycling 135, 190–201. doi: 10.1016/j.resconrec.2017.10.034
Kanemoto, K., Moran, D., and Hertwich, E. G. (2016). Mapping the carbon footprint of nations. Environ. Sci. Technol. 50, 10512–10517. doi: 10.1021/acs.est.6b03227
Lenzen, M., Kanemoto, K., Moran, D., and Geschke, A. (2012). Mapping the structure of the world economy. Environ. Sci. Technol. 46, 8374–8381. doi: 10.1021/es300171x
Lenzen, M., Moran, D., Kanemoto, K., and Geschke, A. (2013). Building EORA: a global multi683 region inputdatabase at high country and sector resolution. Econ. Syst. Res. 25, 20–49. doi: 10.1080/09535314.2013.769938
Liang, S., and Zhang, T. (2011). Data acquisition for applying physical input-output tables in chinese cities. J. Ind. Ecol. 15, 825–835. doi: 10.1111/j.1530-9290.2011.00372.x
LWARB (2017). London's Circular Economy Route Map. Technical Report, London Waste and Recycling Board, London.
Marchesi, M., and Tweed, C. (2021). Social innovation for a circular economy in social housing. Sustain. Cities Soc. 71, 102925. doi: 10.1016/j.scs.2021.102925
Martinho, V. J. P. D. (2021). Insights into circular economy indicators: Emphasizing dimensions of sustainability. Environ. Sustain. Indicators 10, 100119. doi: 10.1016/j.indic.2021.100119
Moran, D., Kanemoto, K., Jiborn, M., Wood, R., Tobben, J., and Seto, K. C. (2018). Carbon footprints of 13 000 cities. Environ. Res. Lett. 13, 064041. doi: 10.1088/1748-9326/aac72a
Moran, D., and Wood, R. (2014). Convergence between the EORA, WIOD, EXIOBASE, and OPENEU's consumption-based carbon accounts. Econ. Syst. Res. 26, 245–261. doi: 10.1080/09535314.2014.935298
Munoz, H. M. E. (2018). Spatial Microsimulation Urban Metabolism (SMUM). Paris: United Nations Environment Programme. Available Online at: https://smum.readthedocs.io/en/latest/ (accessed June 12, 2018).
Pace. (2021). Circular Indicators for Governments: Accelerating action in the circular economy. Platform for Accelerating the Circular Economy, The Hague, Netherlands. Available Online at: https://pacecircular.org/sites/default/files/2021-04/CircularIndicatorsForGovernments_FINAL.pdf
Papageorgiou, A., Henrysson, M., Nuur, C., Sinha, R., Sundberg, C., and Vanhuyse, F. (2021). Mapping and assessing indicator-based frameworks for monitoring circular economy development at the city-level. Sustain. Cities Soc. 75, 103378. doi: 10.1016/j.scs.2021.103378
Patwa, N., Sivarajah, U., Seetharaman, A., Sarkar, S., Maiti, K., and Hingorani, K. (2021). Towards a circular economy: an emerging economies context. J. Bus. Res. 122, 725–735. doi: 10.1016/j.jbusres.2020.05.015
Petit-Boix, A., and Leipold, S. (2018). Circular economy in cities: reviewing how environmental research aligns with local practices. J. Clean. Prod. 195, 1270–1281. doi: 10.1016/j.jclepro.2018.05.281
Pincetl, S., Bunje, P., and Holmes, T. (2012). An expanded urban metabolism method: toward a systems approach for assessing urban energy processes and causes. Landscape Urban Plann. 107, 193–202. doi: 10.1016/j.landurbplan.2012.06.006Rosado
Rosado, L., Kalmykova, Y., and Patrcio, J. (2016). Urban metabolism profiles. an empirical analysisof the material flow characteristics of three metropolitan areas in sweden. J. Clean. Prod. 126, 206–217. doi: 10.1016/j.jclepro.2016.02.139
Saavedra, Y. M., Iritani, D. R., Pavan, A. L., and Ometto, A. R. (2018). Theoretical contribution of industrial ecology to circular economy. J. Clean. Prod. 170, 1514–1522. doi: 10.1016/j.jclepro.2017.09.260
Santagata, R., Zucaro, A., Viglia, S., Ripa, M., Tian, X., and Ulgiati, S. (2020). Assessing the sustainability of urban eco-systems through emergy-based circular economy indicators. Ecol. Indicat. 109, 105859. doi: 10.1016/j.ecolind.2019.105859
Schggl, J.-P., Stumpf, L., and Baumgartner, R. J. (2020). The narrative of sustainability and circular economy - a longitudinal review of two decades of research. Resour. Conserv. Recycling 163, 105073. doi: 10.1016/j.resconrec.2020.105073
SEI (2020). Urban Circularity Assessment Framework. Technical Report, Stockholm Environment Institute, Stockholm.
Stadler, K., Steen-Olsen, K., and Wood, R. (2014). The rest of the world estimating the economic structure of missing regions in global multi-regional input output tables. Econ. Syst. Res. 26, 303–326. doi: 10.1080/09535314.2014.936831
Stadler, K., Wood, R., Bulavskaya, T., Sodersten, C.-J., Simas, M., Schmidt, S., et al. (2018). EXIOBASE 3: developing a time series of detailed environmentally extended multi-regional input-output tables. J. Ind. Ecol. 22, 502–515. doi: 10.1111/jiec.12715
Stahel, W. R. (2010). The Performance Economy, 2nd Edn. (Hampshire: Palgrave Macmillan), 349. Available Online at: https://www.ebook.de/de/product/9647298/w_stahel_the_performance_economy.html
Surez-Eiroa, B., Fernndez, E., and Mndez, G. (2021). Integration of the circular economy paradigm under the just and safe operating space narrative: twelve operational principles based on circularity, sustainability and resilience. J. Clean. Prod. 322, 129071. doi: 10.1016/j.jclepro.2021.129071
Swilling, M., M. S., Robinson, B., and M, H. (2013). City-Level Decoupling: Urban Resource Flows and the Governance of Infrastructure Transitions. (Nairobi: United Nations Environment Programme).
Timmer, M. (2012). The World Input-Output Database (WIOD): Contents, Sources and Methods. Technical Report, Working Paper Number 10, Version 0.9. Working Paper Number 10.
Tukker, A., de Koning, A., Wood, R., Hawkins, T., Lutter, S., Acosta, J., et al. (2013). EXIOPOL development and illustrative analyses of a detailed global mr ee sut/iot. Econ. Syst. Res. 25, 50–70. doi: 10.1080/09535314.2012.761952
Tukker, A., Goldbohm, R. A., de Koning, A., Verheijden, M., Kleijn, R., Wolf, O., et al. (2011). Environmental impacts of changes to healthier diets in europe. Ecol. Econ. 70, 1776–1788. doi: 10.1016/j.ecolecon.2011.05.001
Tukker, A., Poliakov, E., Heijungs, R., Hawkins, T., Neuwahl, F., Rueda-Cantuche, J. M., et al. (2009). Towards a global multi-regional environmentally extended input output database. Ecol. Econ. 68, 1928–1937. doi: 10.1016/j.ecolecon.2008.11.010
UN (2015). World Urbanization Prospects: The 2014 Revision: (ST/ESA/SER.A/366). Technical Report, United Nations, New York, NY.
UN-DESA (2016). The World's Cities in 2016 - Data Booklet. Technical Report, United Nations Department of Economic and Social Affairs, New York, NY.
UNEP (2019). Brussels Capital Region: Circular Economy. Technical Report, United Nations Environment Programme, Nairobi.
UNEP (2020). UNEP Circularity Platform. Technical Report, United Nations Environment Programme, Nairobi.
Vanhuyse, F., Fejzi, E., Ddiba, D., and Henrysson, M. (2021). The lack of social impact considerations in transitioning towards urban circular economies: a scoping review. Sustain. Cities Soc. 75, 103394. doi: 10.1016/j.scs.2021.103394
Webster, K. (2017). The Circular Economy: A Wealth of Flows, ed E. MacArthur (Cowes: Ellen MacArthur Foundation Publishing).
Wilkman, A., and Skaynberg, K. (2015). The circular economy and benefits for society -jobs and climate clear winners in an economy based on renewable energy and resource efficiency. Club of Rome, Mava, Zurich, Switzerland. Available Online at: http://wijkman.se/wp-content/uploads/2015/05/The-Circular-Economy-and-Benefits-for-Society.pdf
Wood, R., Hawkins, T. R., Hertwich, E. G., and Tukker, A. (2014). Harmonising national input output tables for consumption-based accounting experiences from EXIOPOL. Econ. Syst. Res. 26, 387–409. doi: 10.1080/09535314.2014.960913
Yal, N. G., and Foxon, T. J. (2021). A systemic approach to transitions towards circular economy: The case of brighton and hove. Clean. Environ. Syst. 3, 100038. doi: 10.1016/j.cesys.2021.100038
Zhang, Y., Linlin, X., and Weining, X. (2014). Analyzing spatial patterns of urban carbon metabolism: a case study in beijing, china. Landscape Urban Plann. 130, 184–200. doi: 10.1016/j.landurbplan.2014.05.006
https://docs.google.com/document/d/1Xfbt_0e8OamCPAicocmiLmw0VW4Nux8DoEy7SKAVLCw
Keywords: circular economy, IO tables, MID, RME, cities
Citation: Muñoz H. ME, Novak M, Gil S, Dufourmont J, Goodwin Brown E, Confiado A and Nelemans M (2022) Tracking a Circular Economy Transition Through Jobs: Method Development and Application in Two Cities. Front. Sustain. Cities 3:787076. doi: 10.3389/frsc.2021.787076
Received: 30 September 2021; Accepted: 16 December 2021;
Published: 13 January 2022.
Edited by:
Sujit Sikder, Leibniz Institute for Ecological Urban and Regional Development (IOER), GermanyReviewed by:
Md. Moynul Ahsan, Ankara University, TurkeyCopyright © 2022 Muñoz H., Novak, Gil, Dufourmont, Goodwin Brown, Confiado and Nelemans. This is an open-access article distributed under the terms of the Creative Commons Attribution License (CC BY). The use, distribution or reproduction in other forums is permitted, provided the original author(s) and the copyright owner(s) are credited and that the original publication in this journal is cited, in accordance with accepted academic practice. No use, distribution or reproduction is permitted which does not comply with these terms.
*Correspondence: M. Esteban Muñoz H., ZXN0ZWJhbi5tdW5vekB1bi5vcmc=; Marijana Novak, bWFyaWphbmFAY2lyY2xlLWVjb25vbXkuY29t
Disclaimer: All claims expressed in this article are solely those of the authors and do not necessarily represent those of their affiliated organizations, or those of the publisher, the editors and the reviewers. Any product that may be evaluated in this article or claim that may be made by its manufacturer is not guaranteed or endorsed by the publisher.
Research integrity at Frontiers
Learn more about the work of our research integrity team to safeguard the quality of each article we publish.