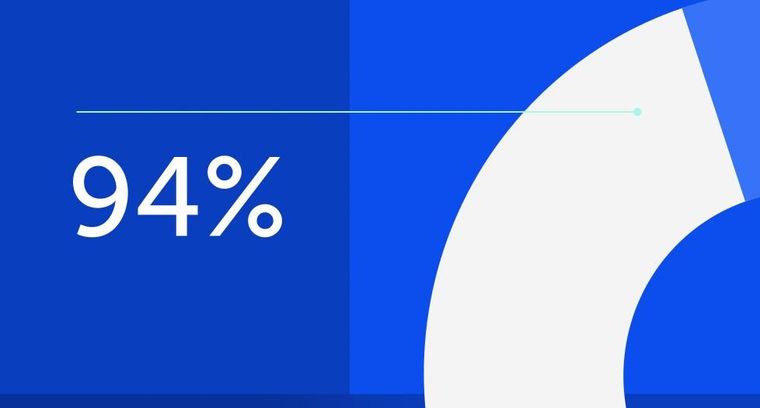
94% of researchers rate our articles as excellent or good
Learn more about the work of our research integrity team to safeguard the quality of each article we publish.
Find out more
PERSPECTIVE article
Front. Sustain. Cities, 15 December 2021
Sec. Innovation and Governance
Volume 3 - 2021 | https://doi.org/10.3389/frsc.2021.786563
This article is part of the Research TopicEnvironmental Data, Governance and the Sustainable CityView all 9 articles
The increasing amount of data collected about the environment brings tremendous potential to create digital systems that can predict the impact of intended and unintended changes. With growing interest in the construction of Digital Twins across multiple sectors, combined with rapid changes to where we spend our time and the nature of pollutants we are exposed to, we find ourselves at a crossroads of opportunity with regards to air quality mitigation in cities. With this in mind, we briefly discuss the interplay between available data and state of the science on air quality, infrastructure needs and areas of opportunities that should drive subsequent planning of the digital twin ecosystem and associated components. Data driven modeling and digital twins are promoted as the most efficient route to decision making in an evolving atmosphere. However, following the diverse data streams on which these frameworks are built, they must be supported by a diverse community. This is an opportunity to build a collaborative space to facilitate closer working between instrument manufacturers, data scientists, atmospheric scientists, and user groups including but not limited to regional and national policy makers.
Air pollution is a major cause of death and disease and has been identified as the significant public health concern across Europe (Manisalidis et al., 2020; Khomenko et al., 2021). In many UK cities, exceedances of NO2 above EU thresholds are observed and PM2.5 levels frequently exceed the new 5 μg/m3 annual level set by the World Health Organisation (WHO, 2021). As interventions such as clean air zones are rolled out, the nature of pollution will inevitably change. An important class of pollutants, Volatile Organic Compounds (VOCs) have traditionally been thought of as arising from sources such as industry, fuel evaporation and road traffic. Whilst these sources have diminished considerably, a vast range of different VOCs are now emitted from a range of new sources (Lewis, 2018; McDonald et al., 2018). These are challenging to identify and characterize and we have yet to fully understand their contribution to toxicity, ozone and particle formation. There is considerable evidence that bio-aerosol such as pollen, bacteria, other allergens and air pollution combine to exacerbate human responses (Plaas and Paerl, 2021). The challenges facing local authorities charged with delivering air quality solutions are substantial; the sources and distribution of air pollution vary from city to city and the solutions will often need to be designed to fit specific local contexts.
The increasing amount of data collected about the environment brings tremendous potential for the creation of replicate digital systems that can be used to predict the intended or unintended impacts and consequences of specific policies and the way we conduct our lives. A Digital Twin is a virtual counterpart of a given system. In an operational setting, a Digital Twin would use near to real-time data to provide a snapshot of how the system is responding to multiple stressors. This can be used to assess how the system may respond to changes in future conditions (Fuller et al., 2020; Jones et al., 2020; Rasheed et al., 2020; Bauer et al., 2021).
As cities move to become more sustainable, the integration and use of this corpus of data in predictive computational models is important. The desirable target would be for these digital tools to inform the decision-making process of the relevant administrative bodies, leading to policies that enhance the physical and mental health of the population and improve environmental outcomes. However, this relies on a set of “digital tools” that can facilitate the delivery of relevant information from specialist scientific systems to policy makers, who must consider multiple trade-offs and have less specialist knowledge.
With the boom in smart city projects and distributed networks of sensors, online dashboards have emerged as a method of providing information in a way that can be consumed by non-specialists. Whilst this gives a near to real time picture of the environment, the onus is still on users to understand and integrate this data into their decision-making systems in order to make it useful. Such solutions have been widely criticized for failing to adequately reflect local conditions, being imposed in a one-size-fits-all fashion, and concealing important questions about its provenance and presentation (Marvin et al., 2015). Digital Twinning ecosystems need to avoid these pitfalls by being scientifically robust and co-created with users, as espoused by the Gemini Principles published by the Centre for Digital Built Britain in December 2018 (Bolton et al., 2008).
The scientific community has developed several modeling platforms that aim to simulate the emission, advection and evolution of pollutants in the atmosphere. Based on the known chemical and physical processes that dictate the abundance of specific compounds in the atmosphere and their effect on the environment and human health, these tools have proven to be essential in quantifying the impact of policy adoption (Kukkonen et al., 2012; Thunis et al., 2016; Viaene et al., 2016). However, with an increasing demand for higher spatial resolution and the inclusion/integration of more processes known (or deemed) to impact atmospheric composition, the traditional approach to air quality modeling is likely to have a limited lifespan. Data driven techniques have the potential to project near to medium term forecasts based on streams of data generated by urban monitoring networks. Given the complex nature of pollutant emission and dispersion, a better understanding of how different factors may affect air quality may be provided by implementing a Digital Twin of urban air. What happens if a planned traffic intervention leads to increase traffic through another neighborhood? Can we integrate transport data and personal mobility data into a near real-time model of personal exposure for population exposure estimates? These are the sorts of questions that could be answered by utilizing different “flavors” of Digital Twins but which might otherwise have been difficult to answer using traditional models based on first principles. Indeed, it is important to note that the ecosystem of Digital Twins of urban air will likely cover multiple scales and solutions driven by diverse user needs, from hyper localized frameworks of an indoor office to twins of regional centers. Figure 1 attempts to provide a visual schematic of how data generated from physical assets are connected through a range of digital infrastructures to build a Digital Twin. In this case, an integrated network of sensors (e.g., environment and mobility) provide the data on which air quality and personal exposure forecasting techniques can predict and adapt to change. These predictions could include identifying periods of health risk to sensitive cohorts of the population. Combined with various analytics, the Digital Twin would allow a playback of multiple scenarios whilst also providing a platform for simulating the potential impacts of various interventions before implementation in the real-world. For example, a playback scenario in the urban air quality domain could be revisiting historical data streams and implementing a hypothetical set of changes in planning to ascertain whether increases in personal exposure could have been avoided. This may include assessing whether specific road closures forced traffic to move into busy residential areas and increase levels of pollution for residents there. Conversely, supporting regional and local data streams may reveal through historical playbacks that conditions beyond the control of local interventions dictated levels over specific periods. This rather simple schematic is distinctly different from running a pollutant dispersion model on a high performance computing cluster based on prescribed gridded emissions. The Digital Twin ingests near to real-time data from a range of disparate sources from across a city, providing an end-to-end decision system that captures local dependencies.
Figure 1. A schematic showing how data generated from physical assets that are used to monitor an urban environment are used to build a virtual counterpart; the Digital Twin. Using a range of forecasting and analytics allows scenario playback and the potential impacts of interventions to be assessed.
With the increased accessibility of powerful edge computing devices (Shi et al., 2016; Sittón-Candanedo et al., 2019), the landscape of computing provision is changing. The traditional notion that only a small subset of researchers can develop and apply computational models of our atmosphere is also fading. The environment we wish to simulate is also changing. Urban regeneration, increased home-working, traffic interventions and decarbonization of the transport sector all alter the mixture of pollutants we are exposed to and where we spend our time. All these factors point toward a paradigm change in the way air quality modeling should be carried out.
We do not formulate a proposed technological architecture of a Digital Twin here. Rather, we briefly discuss the interplay between available data and state of the science, infrastructure needs and areas of opportunities that should inform—and arguably drive—subsequent planning of the Digital Twin ecosystem and associated components.
The Digital Twin ecosystem will rely on data that captures the evolving composition of the atmosphere. The usefulness of this data, and thus of any digital twin, is informed by the evolving state of the underlying science.
Instrumentation to measure the composition and abundance of air pollution and infer its health effects has developed considerably. National ground-based networks, research super-sites, satellite data, model outputs, health data, emissions databases and a rich source of urban data and population activity data are now available with varying velocity and veracity. However, there is a disconnect between information provided by the established distributed sensor networks and lower cost instrumentation used for research. The range of available low-cost air quality sensor technologies is constantly evolving, with price entry points in some cases less than £100. Using this technology appropriately comes with a number of challenges, however, including: calibration and bias correction; maintaining long term operational reliability; and optimizing network structure whilst quantifying the relevant uncertainties and biases. In many cases, biases and uncertainties are too large and, as such, their utility as data streams for networks and thus Digital Twins is significantly reduced at present. Importantly, quantifying their scientific value is ongoing.
The last decade has seen significant advances in our understanding of how poor air quality affects human health. We need to link emissions and pollution loadings to health impacts on the population via a consideration of the way people and pollution interact across an urban environment. To date, inventories have been coupled to models that carry the detailed chemistry and physics capturing the distribution of air pollution at the time and space scales of interest. These vary from the street canyon to the urban and regional scales. Current models that predict concentrations of PM2.5, NOx and O3 are often computationally expensive and cannot readily be directly coupled to models of activity across a city. Hence exposure studies have, to date, largely coupled behaviour models to air quality scenarios in an offline way. However, observations of individuals' pathways through the urban landscape (and hence their personal exposure) can be used to test predictive capability. This provides a clear opportunity for Digital Twins.
Whilst the natural focus of a Digital Twin of urban air is on the development and application of machine learning (ML) and statistical tools, the underlying digital infrastructure also plays a crucial role in enabling data harvesting, calibration, standardization, security and information governance. All these elements, which sit primarily within the domain of data engineering, ensure that this digital ecosystem is built in compliance with requirements of trust, data privacy and data sustainability.
• Meta-data standards: These are of paramount importance. The development of pipelines for the collection and provision of data should put particular emphasis on adopting data structures able to expose and contextualize data coming from different sources in a unified fashion. There are de facto standards to represent sensor-generated data and the underlying assets/devices, such as the “Semantic Sensor Network Ontology (SSN)” (Compton et al., 2012). Adhering to established web ontologies can help navigation of available data catalogs and generate consensus around data representation in the urban environment. As the data is represented and contextualized using the same standard, it can be exposed through a unified API.
• Labeled datasets: We now see the emergence of publicly available labeled datasets across multiple environmental domains for the purpose of developing AI based systems [e.g., ClimateNet (Prabhat et al., 2021)]. The term label is used here to relate a measured quantity to a specific type of event, for example the identification of compounds with varying toxicity, particulate types, or regional haze events. To the best of our knowledge, there are no such commonly held databases for generic air-quality purposes. For the purpose of instrument calibrations, whilst there are databases provided/held by instrument manufacturers, the status as pertains the relevance for key signatures to key pollutants/compounds or generic tracers remains unclear. For large scale air quality “events”, we would require a database of identified atmospheric conditions that lead to formation of significant levels of pollution to inform forecasting methodologies.
• Communications: Here we consider the need for fast and reliable communications between measurement nodes and decision systems and/or air quality visualizations. This also includes potential use in network calibration and assimilating large quantities of data for learning/refitting ML focused tools. 5G communications in particular have potential for underpinning distributed networks and assimilation of disparate data sources. There is evidence of development around “smart” network calibration of low-costs sensors using 5G technologies. The increased bandwidth also facilitates the integration of other data sources that can be useful in interpreting air-quality events and developing services around that, for example traffic data, energy use, and footfall.
Given the thematic challenges and infrastructural needs, there are several potential developments that would support a digital twinning ecosystem of urban air quality.
• Source and process identification at the edge: Measurement methods are now many orders of magnitude more sensitive, can often resolve thousands of individual molecules, and can retrieve information on pollution systems in multiple ways simultaneously (e.g., Vasquez, 2021). As a result, an increasing amount of data is now recovered. Much of the work in identifying source and process contributions to detected pollutant signatures (e.g., Organic Particulate matter source apportionment) relies on methodologies that have been used for 10 years or more. There is limited evidence of work beyond traditional unsupervised methods that require expert manual interpretation. There is even less evidence of the use of supervised learning methods for classification purposes, where existing classifications are taken from previous ambient or laboratory studies and used to interpret emerging data. With significant investment in high-grade instruments, edge computing could be used to exploit the wealth of information captured in an instrument response function and provide near real-time information to users on source types, potentially combining ancillary data from traffic flows or meteorological data.
• Hyper-Local-forecasting: Traditional chemical-transport models are expensive and can be slow to generate results. Hyper-local forecasting methods provide day-month forecasts of concentrations using historical and near to real time data combined with ancillary data (e.g., traffic). Once trained these methods provide a rapid and cost-effective deployment option for a range of stakeholders and could be integrated with the previous developments on source and process identification. There is a lack of evidence of scalability and/or applicability to varying urban topologies/background contributions and a gap in formulating methods that rely not only on atmospheric data, but also draw in other data products. This would be of particular use in the evaluation of urban designs and predicting the impacts of potential interventions.
• Replacement of traditional numerical methods and/or hybrid process level-ML air-quality models. Retaining the numerical basis of existing models of air quality, whilst increasing the physical/chemical complexity they represent, requires improvement in computational efficiency of the solution process. In some cases, there may be no existing theoretical, and thus numerical, framework for an end-end process (e.g., emission to health outcome). In other cases, the model complexity may be too great for initialization or compute constraints. Combining ML and process-based models would enable use of disparate data-like images and time series to build such frameworks whilst also including provenance of known physics/chemistry of the atmosphere. The next generation of earth system models are likely going to merge machine learning and traditional process driven models and exploit growing datasets of global observations (Reichstein et al., 2019). New programming languages now enable the development of combined ML and process models, where the ML component learns from the model environment. Moreover, a new generation of ML algorithms is emerging which can leverage a priori physical knowledge (e.g., Neural Ordinary Differential Equations). These hybrid “physics informed” approaches could improve trust in new predictive systems.
• Natural Language Processing [NLP] and social media analysis: A tool for detecting behaviour change and response to environmental stressors. Social media analysis tools have been used to assess citizen and knowledge responses to natural hazards. Air quality NLP studies have demonstrated the potential for extracting links between citizen observed conditions and changes in air-quality (e.g., Gurajala et al., 2019). Understanding the sentiment of social media posts could offer significant insights into better understanding citizen responses to interventions and, more broadly, response to changing conditions in a future climate/net-zero world.
• Visualization: Augmented (AR) and Virtual reality (VR) now sit within services used for engaging and informing citizens of the choices they make in the environment. Whilst delivery of air quality information exists through dashboards and web portals, AR services could also benefit from integration with rapid communications where delivery of real-time information on air-quality conditions could be streamed to edge devices, such as mobile phones, from a 5G network.
Air quality is complex, and is affected by a variety of interdependent factors. A significant evidence base is required to build accurate and trustworthy digital twins. However, with appropriate consideration of the required digital infrastructure, there are a number of opportunities that will ensure developments are driven by the evolving need for air quality amelioration, exploiting existing expertise in monitoring and modeling technologies.
Data driven modeling and Digital Twins may well be the most efficient route to decision making in an evolving atmosphere. As we have discussed in this manuscript, a Digital Twin might ingest near to real-time data from a range of disparate sources from across a city, providing an end-to-end decision system that captures local dependencies that would otherwise be difficult to achieve using traditional numerical models. However, following the diverse data streams on which these frameworks are built, we must also ensure they are available to and supported by a diverse community. This is an opportunity to build a collaborative space to facilitate closer working between instrument manufacturers, data scientists, environmental scientists, and user groups including but not limited to regional and national policy makers. Whether this takes the form of regional to national forums, centers of excellence, or national twinning programmes, diverse representation is key to ensuring digital twins have a sustainable future built on scientific evidence, efficient use of data, and trust.
We need a skilled workforce across national and local governments that is capable of co-designing, implementing and interrogating outputs and components of a digital twin to inform policy and local decision-making. It has been noted that the key barrier to UK implementation of data driven techniques for air quality policy is a digital skills shortage across the public sector (Department DfE, 2021). Developing Digital Twin ecosystems with data holders and users will drive the creation of data innovation roles, and provide a mechanism for upskilling current employees with improved digital skills. Doing so will ensure that Digital Twins reflect specific local contexts and needs, providing usable insights and securing legitimacy among key users.
The original contributions presented in the study are included in the article/supplementary material, further inquiries can be directed to the corresponding author.
DT conceived, wrote, and compiled the article. TB contributed to the discussion of needs around sensor technologies. HC framed the discussion around air quality challenges and emerging pollutants. JE and CJ contributed to the role of stakeholder governance. EM compiled the data standards and ontology requirements and NR framed the narrative around access and opportunities of emerging technologies. CJ reviewed and edited the article. All authors contributed to the article and approved the submitted version.
This work was supported by the EPSRC UKCRIC Manchester Urban Observatory (University of Manchester) (grant no: EP/P016782/1).
The authors declare that the research was conducted in the absence of any commercial or financial relationships that could be construed as a potential conflict of interest.
All claims expressed in this article are solely those of the authors and do not necessarily represent those of their affiliated organizations, or those of the publisher, the editors and the reviewers. Any product that may be evaluated in this article, or claim that may be made by its manufacturer, is not guaranteed or endorsed by the publisher.
Bauer, P., Stevens, B., and Hazeleger, W. (2021). A digital twin of earth for the green transition. Nat. Clim. Chang 11, 80–83. doi: 10.1038/s41558-021-00986-y
Bolton, A., Enzer, M., Schooling, J., et al (2008). The Gemini Principles: Guiding Values for the National Digital Twin and Information Management Framework. Centre for Digital Built Britain and Digital Framework Task Group. Available online at: https://www.cdbb.cam.ac.uk/system/files/documents/TheGeminiPrinciples.pdf
Compton, M., Barnaghi, P., Bermudez, L., García-Castro, R., Corcho, O., Cox, S., et al. (2012). The ssn ontology of the w3c semantic sensor network incubator group. J. Web Semant. 17, 25–32. doi: 10.1016/j.websem.2012.05.003
Department for Education (2021). Skills for Jobs: Lifelong Learning for Opportunity and Growth. Available online at: https://assets.publishing.service.gov.uk/government/uploads/system/uploads/attachment_data/file/957810/Skills_for_jobs_lifelong_learning_for_opportunity_and_growth__print_version_.pdf
Fuller, A., Fan, Z., Day, C., and Barlow, C. (2020). Digital twin: enabling technologies, challenges and open research. IEEE Access 8, 108952–108971. doi: 10.1109/ACCESS.2020.2998358
Gurajala, S., Dhaniyala, S., and Matthews, J. N. (2019). Understanding public response to air quality using tweet analysis. Soc. Media Soc. 5:205630511986765. doi: 10.1177/2056305119867656
Jones, D., Snider, C., Nassehi, A., Yon, J., and Hicks, B. (2020). Characterising the digital twin: a systematic literature review. CIRP J. Manufact. Sci. Technol. 29, 36–52. doi: 10.1016/j.cirpj.2020.02.002
Khomenko, S., Cirach, M., Pereira-Barboza, E., Mueller, N., Barrera-Gómez, J., Rojas-Rueda, D., et al. (2021). Premature mortality due to air pollution in european cities: a health impact assessment. Lancet Planetary Health 5, e121–e134. doi: 10.1016/S2542-5196(20)30272-2
Kukkonen, J., Olsson, T., Schultz, D. M., Baklanov, A., Klein, T., Miranda, A. I., et al. (2012). A review of operational, regional-scale, chemical weather forecasting models in europe. Atmos. Chem. Phys. 12, 1–87. doi: 10.5194/acp-12-1-2012
Lewis, A. C.. (2018). The changing face of urban air pollution volatile organic compounds in u.s. urban air increasingly derive from consumer products. Science 359, 744–745. doi: 10.1126/science.aar4925
Manisalidis, I., Stavropoulou, E., Stavropoulos, A., and Bezirtzoglou, E. (2020). Environmental and health impacts of air pollution: a review. Front. Public Health 8:14. doi: 10.3389/fpubh.2020.00014
Marvin, S., Luque-Ayala, A., and McFarlane, C. (2015). Smart Urbanism: Utopian Vision or False Dawn? 1st Edn. London: Taylor and Francis Inc.
McDonald, B. C., Gouw, J. A. D., Gilman, J. B., Jathar, S. H., Akherati, A., Cappa, C. D., et al. (2018). Volatile chemical products emerging as largest petrochemical source of urban organic emissions. Science 359, 760–764. doi: 10.1126/science.aaq0524
Plaas, H. E., and Paerl, H. W. (2021). Toxic cyanobacteria: A growing threat to water and air quality. Environ. Sci. Technol. 55, 44–64. doi: 10.1021/acs.est.0c06653
Prabhat K.ashinath, K., Mudigonda, M., Kim, S., Kapp-Schwoerer, L., Graubner, A., Karaismailoglu, E., et al. (2021). Climatenet: an expert-labeled open dataset and deep learning architecture for enabling high-precision analyses of extreme weather. Geoscientific Model Dev. 14, 107–124. doi: 10.5194/gmd-14-107-2021
Rasheed, A., San, O., and Kvamsdal, T. (2020). Digital twin: Values, challenges and enablers from a modeling perspective. IEEE Access 8, 21980–22012. doi: 10.1109/ACCESS.2020.2970143
Reichstein, M., Camps-Valls, G., Stevens, B., Jung, M., Denzler, J., Carvalhais, N., and Prabhat (2019). Deep learning and process understanding for data-driven earth system science. Nature 566, 195–204. doi: 10.1038/s41586-019-0912-1
Shi, W., Cao, J., Zhang, Q., Li, Y., and Xu, L. (2016). Edge computing: vision and challenges. IEEE Internet Things J. 3, 637–646. doi: 10.1109/JIOT.2016.2579198
Sittón-Candanedo, I., Alonso, R. S., Corchado, J. M., Rodríguez-González, S., and Casado-Vara, R. (2019). A review of edge computing reference architectures and a new global edge proposal. Future Generation Comput. Syst. 99, 278–294. doi: 10.1016/j.future.2019.04.016
Thunis, P., Miranda, A., Baldasano, J. M., Blond, N., Douros, J., Graff, A., et al. (2016). Overview of current regional and local scale air quality modelling practices: assessment and planning tools in the eu. Environ. Sci. Policy 65, 13–21. doi: 10.1016/j.envsci.2016.03.013
Vasquez, K.. (2021). Measuring atmospheric trace gases using mass spectrometry. Nat. Rev. Earth Environ. 2:305. doi: 10.1038/s43017-021-00163-x
Viaene, P., Belis, C. A., Blond, N., Bouland, C., Juda-Rezler, K., Karvosenoja, N., et al. (2016). Air quality integrated assessment modelling in the context of eu policy: a way forward. Environ. Sci. Policy 65, 22–28. doi: 10.1016/j.envsci.2016.05.024
World Health Organization (2021). WHO Global Air Quality Guidelines: Particulate Matter (PM2.5 and PM10), Ozone, Nitrogen Dioxide, Sulfur Dioxide and Carbon Monoxide. World Health Organization. Available online at: https://apps.who.int/iris/handle/10665/345329
Keywords: air quality, digital twins, cities, environment, data
Citation: Topping D, Bannan TJ, Coe H, Evans J, Jay C, Murabito E and Robinson N (2021) Digital Twins of Urban Air Quality: Opportunities and Challenges. Front. Sustain. Cities 3:786563. doi: 10.3389/frsc.2021.786563
Received: 30 September 2021; Accepted: 23 November 2021;
Published: 15 December 2021.
Edited by:
Joop De Kraker, Maastricht University, NetherlandsReviewed by:
Guy Coulson, National Institute of Water and Atmospheric Research (NIWA), New ZealandCopyright © 2021 Topping, Bannan, Coe, Evans, Jay, Murabito and Robinson. This is an open-access article distributed under the terms of the Creative Commons Attribution License (CC BY). The use, distribution or reproduction in other forums is permitted, provided the original author(s) and the copyright owner(s) are credited and that the original publication in this journal is cited, in accordance with accepted academic practice. No use, distribution or reproduction is permitted which does not comply with these terms.
*Correspondence: David Topping, ZGF2aWQudG9wcGluZ0BtYW5jaGVzdGVyLmFjLnVr
Disclaimer: All claims expressed in this article are solely those of the authors and do not necessarily represent those of their affiliated organizations, or those of the publisher, the editors and the reviewers. Any product that may be evaluated in this article or claim that may be made by its manufacturer is not guaranteed or endorsed by the publisher.
Research integrity at Frontiers
Learn more about the work of our research integrity team to safeguard the quality of each article we publish.