- 1Department of Civil Engineering, Indian Institute of Technology Kanpur, Kanpur, India
- 2Design Programme, Indian Institute of Technology Kanpur, Kanpur, India
- 3Atmospheric Particle Technology Laboratory at Centre for Environmental Science and Engineering (CESE), Indian Institute of Technology Kanpur, Kanpur, India
This study reports on the high-resolution data set of ground-level O3, surface-bound polycyclic aromatic hydrocarbons (SB-PAHs), and particle's number concentrations (range: 10 to 1,000 nm, referred to as condensation nucleus concentration: CNC) during a Diwali festival campaign (conducted from 08th to 16th Nov.2015) at Kanpur location. In this study, we have made an attempt to assess the change in atmospheric composition and chemistry (based on SB-PAHs, O3, and CNC) during Diwali festival (11th Nov.) and compared the results with pre-Diwali (08th−10th Nov.) and post-Diwali (12th−16th Nov.) scenarios. The wind pattern and cluster analysis have revealed a quite similar feature that from 10th to 16th of November the prevailed winds were north-westerly (NW). It is noteworthy that NW-winds during post-monsoon season (Oct–Nov) favors the long-range transport of biomass burning emissions (LRT-BB) from its source region in upwind Indo-Gangetic Plain (IGP). The influence of LRT-BB emissions at the receptor site during Diwali and post-Diwali period was reflected by the substantial increase in average concentrations of PM2.5, O3 and CNC (difference has been ascertained from a two-tailed t-test). The Lenshchow-type analysis revealed that the firecrackers (FC) burst and LRT-BB emissions have lead to increase the concentrations of CNC by 54% and 86%, respectively over the urban background level. On the other hand, the FC burst and LRT-BB increased the concentrations of O3 by 12% and 31% (over the urban background), respectively. Lenschow-type analysis revealed that FC burst and LRT-BB increased the daily PM2.5 concentration by 11% and 36%, respectively over its urban background level (286 μg m−3). However, the SB-PAHs concentrations were found to be decreased by 6% and 2%, respectively, during the FC burst activity and LRT-BB emissions. Based on the observations pertaining to the decrease in SB-PAHs concentrations from the Lenschow-type analysis and anti-correlation between SB-PAHs and O3 the heterogeneous-phase chemical reactivity and loss of SB-PAHs has been inferred in this study.
Introduction
Diwali festival is one the major festivals celebrated across India. It is also known as the Festival of Lights and people celebrate it with lots of enthusiasm and through cultural activities. This festival is celebrated on a particular date either in the month of October or November based on the Indian Vedic Calendar. However, worsening of air quality on Diwali festival due to bursting of firecrackers (FC) is one of the major concerns raised by many researchers (Singh et al., 2010; Joshi et al., 2016; Ambade, 2018; Izhar et al., 2018; Rastogi et al., 2019). It is worthwhile mentioning that unlike in the western and southern parts of India, the air quality in northern part of India worsens during October–November due to additional input of pollutants from the long-range transport of biomass burning emissions (LRT-BB) (Rajput et al., 2011, 2016; Kaskaoutis et al., 2014; Jethva et al., 2019; Sharma et al., 2019). Thus, LRT-BB poses a challenge in estimating the emissions due to FC burst over the urban background emission (manifested primarily by vehicular exhaust, industrial emission, and soil dust resuspension) in northern India. The overall pollution load at any given time over a city site is due to, the emissions occuring within the urban agglomeration (hereafter referred to as urban background emissions) and those getting transported from long distances (e.g., LRT-BB), atmospheric chemistry resulting into the formation of new compounds, and episodic emissions (e.g., FC burst on Diwali). To the best of our knowledge, the quantitative estimate of pollutant's concentration only due to FC, after correcting for the contribution of LRT-BB, has not been examined previously for the northern India.
Besides the characterization and impact of primary species (Menon et al., 2002; Ramana et al., 2010; Andreae and Ramanathan, 2013; Sahu et al., 2017; Satish and Rastogi, 2019), there has been a deep interest globally in looking at the features pertaining to chemical transformations occuring under ambient atmospheric conditions (Perraudin et al., 2007; Rudich et al., 2007; Kaiser et al., 2011; George et al., 2015; Nguyen et al., 2016; Pöhlker et al., 2018; Rajput et al., 2018; Rajput and Gupta, 2020). For example, a recent study assesses the secondary organic aerosols (SOA) formation due to ozone utlizing the instrumental variable analysis (IVA) (Rajput and Gupta, 2020). In fact, many studies have focussed on the atmospheric reactivity and health impacts of several types of organic compounds including the polycyclic aromatic hydrocarbons (PAHs) (Ackerman et al., 1994; Maria et al., 2004; Che et al., 2016; Ruehl et al., 2016; Singh and Gupta, 2016; Agarwal et al., 2018). The surface layer reactivity of PAHs with the atmospheric oxidants (e.g., O3 or OH radical) has been found to be asociated with an enhanced cloud condensation nuclei (CCN) activation efficiency and toxicity (of a particle) (Perraudin et al., 2007; Kaiser et al., 2011). The surface-layer oxidation reaction of PAHs plausibly leading to an enhanced CCN activation efficiency is shown through a schematic diagram (Figure 1). This study was conducted with two major objectives: (i) to quantify the contribution of FC burst vis-à-vis LRT-BB emissions to the total burden of pollutants (SB-PAHs, PM2.5, O3 and CNC) above the urban background level at central IGP (Kanpur location) and, (ii) to investigate the association between the SB-PAHs and O3 concentrations during day and nighttime of pre-Diwali, Diwali, and post-Diwali periods.
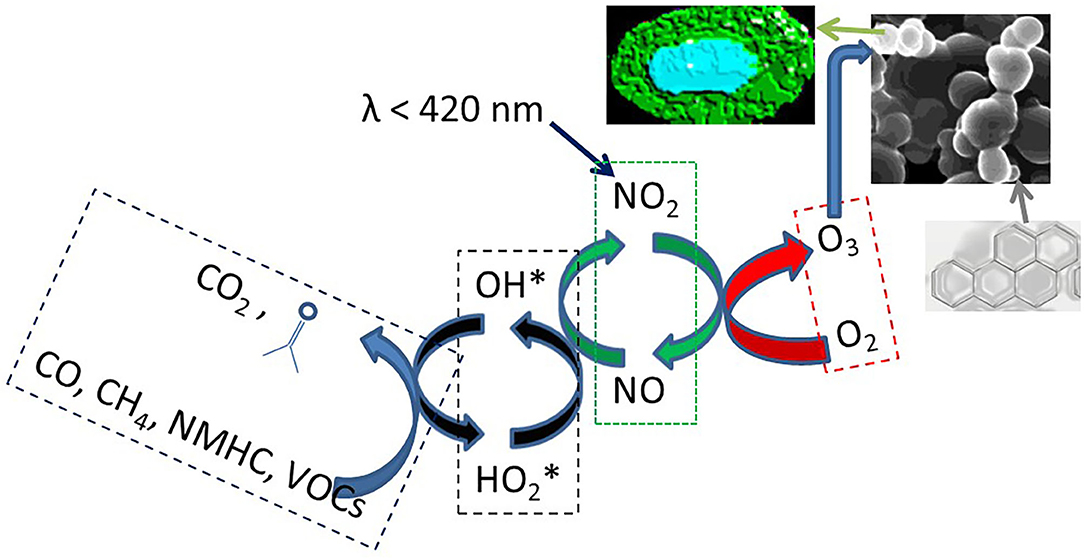
Figure 1. A cartoon mimicking tropospheric coupled system of HOx and NOx cycle involving the oxidation of CO/NMHCs/VOCs/CH4 and forming O3 that further oxidizes SB-PAHs (and other organics) plausibly leading to the formation of CCN (cloud condensation nuclei).
Methodology
Field Campaign
A 9 day campaign from 08th Nov 2015 (local time: 10:00 h) to 16th Nov 2015 (local time: 06: 00 h) was conducted at Kanpur site (26.30 °N, 80.14 °E, 142 m amsl.) in central part of the Indo-Gangetic Plain (IGP). In order to assess the atmospheric chemistry and episodic emission strength from FC burst on Diwali festival day, the entire campaign has been sub-divided into three periods: pre-Diwali (08th−10th Nov.), Diwali (11th Nov.) and post-Diwali (12th−16th Nov.). In this campaign, we have measured high-resolution (Δt = 1-min, n ≈ 10 k data points) near ground-level O3, surface-bound polycyclic aromatic hydrocarbons (SB-PAHs), and particle's number concentrations from 10 to 1,000 nm by condensation particle counter (referred to as CNC, condensation nucleus concentration). For these measurements, three online analyzers have been utilized which were housed in the Atmospheric Particle Technology Laboratory (APTL, first floor, ~25 ft. from the ground) at the Center for Environmental Science & Engineering (CESE) building in the premises of Indian Institute of Technology Kanpur (IITK). The APTL lab remains usually maintained at temperature ~22°C. These instruments were kept nearby on a platform in the lab, and their inlets (separated by <1 m) were allowed to sample air from one of the windows. The window from which the air was sampled for the real-time analysis is situated on the rear side of the CESE building and it does not faces any direct road emission. Relevant details on each instrumentation are explained below:
Real-Time Measurements of Particle's Surface-Bound PAHs
The desktop model of the photoelectric aerosol sensor (PAS 2000, Table 1, Δt = 1-min, n ≈ 10 k data points) uses a Krypton Chlorine excimer lamp that produces photons of energy 5.6 eV peaking at 222 nm wavelength (Niessner and Walendzik, 1989). These photons of 5.6 eV are utilized to photo-ionize PAHs molecules that are adsorbed onto the surface of a sampled particle (Marr et al., 2006). SB-PAHs (unsubstituted) have lower ionization energies and so get ionized upon exposure to photons from KrCl lamp whereas the gas-phase PAHs have higher ionization energies and so neither they get ionized nor be measured by PAS (photoelectric aerosol sensor) (Seki, 1989). Followed by ionization, an electric field removes the ejected electrons whereas the positively charged particles are collected on a filter element and the electric current thus generated is measured by an inbuilt electrometer. The output signal measured by the electrometer is theoretically proportional to the PAHs mass collected by the filter element. It is worthwhile mentioning here that the PAS instrument can measure only “PM surface-bound total PAHs (SB-PAHs)” and cannot provide PAH speciation which will require techniques such as gas chromatography coupled with mass spectrometery (GC-MS) or liquid chromatography coupled with mass spectrometery (LC-MS), among others. The sample preparation followed by chemical analysis of PAHs by GC-MS or LC-MS is time-consuming and results into low-resolution data. Therefore, a sensor technique viz. PAS has been utilized for continuous monitoring of SB-PAHs in this study. The main advantages of PAS (works on aerosol photoionization technique) are its high sensitivity and ability to perform continuous (real-time) measurements with a response time of <10 s. The PAS sensor for the detection of SB-PAHs has been developed by EcoChem Analytics, USA. The instrument was factory-calibrated for measuring PAHs concentrations up to 1,000 ng m−3 (limit of detection: 10 ng m−3) with an uncertainty of <20%. The instrument performance and background signal check were routinely assessed as per technical specifications provided by the manufacturer.
Real-Time Monitoring of O3
Ozone Analyzer (Thermo Scientific; Model # 49i, Table 1, Δt = 1-min, n ≈ 10 k data points), designated by the United States Environmental Protection Agency (USEPA # EQOA-0880-047), is equipped with dual cell (sample and reference) and measures the concentration of O3 in ambient air by UV-photometric technique. The O3 measurement technique is a well-established technique, the details of which can be found in several papers, e.g., Lal et al. (2000). Briefly, O3 molecule absorbs UV photon at a wavelength of 254 nm and the quantum of absorbed UV photons is directly proportional to the O3 concentration. The instrument has a response time of 20 s with a detection limit of 5 ppb and can measure up to 200 ppm. The averaging time for data retrieval was set to 1 min (= 60 s). At the inlet, a Teflon particulate filter was mounted to not allow the entry of any particle in the gas analyzer. The measuring principle of O3 analyzer is based on the Beer-Lambert law. The calibration and zero checks were performed as per the manufacturer's specifications for data quality control and assurance. The uncertainty on O3 measurement is <5%.
Real-Time Monitoring of CNC
Condensation particle counter (CPC model 3007, TSI Inc., Table 1, Δt = 1-min, n ≈ 10 k data points) is a portable condensation nucleus counter (CNC) which measures the condensation nucleus concentration (CNC) in the size range of 0.01–1 μm. The field performance and single particle detection efficiency of this instrument are provided elsewhere (Hämeri et al., 2002; Devi et al., 2013). This instrument was factory calibrated and is capable of measuring particles concentrations as high as 105 cm−3. The zero counts were routinely checked by connecting the CPC monitoring unit inlet with a High-Efficiency Particulate Air (HEPA) filter and we were always satisfied to conduct the measurements. The TSI CPC-3007 is operated with isopropyl alcohol as a condensing fluid. The uncertainty of cumulative CNC measurements by CPC was <1%.
Lenschow-Type Analysis
The Lenschow-type analysis was carried out for each parameter viz. O3, CNC, PM2.5, and SB-PAHs. The details of PM2.5 data set can be found elsewhere (Rajput and Gupta, 2020). Briefly, the hourly averaged data set was segregated into 3-different bins corresponding to UB (urban background), UB+FC+BB (urban background+firecrackers burst+biomass burning contribution), and UB+BB (urban background+biomass burning contribution) depending on their association with wind profile and information on FC burst (on Diwali). The north-westerly wind system favoring LRT-BB emissions was marked for the BB contribution. The UB was represented by the data set which has neither any contributions from FC burst nor from the LRT-BB. For details on finger-printing of sources, based on the analysis of air pollutants data coupled to prevailing winds, the reference is made to the original work by Lenschow et al. (2001).
Results and Discussion
Wind Pattern Analyses
Real-time diurnal measurements have encouraged us to look into more detailed features of atmospheric composition variability during the campaign. The wind direction and wind-speed (refer to scale at the bottom, Figure 2) along with the statistics (given on each panel) were studied through the wind-rose plot. Statistical and data analysis have been carried out utilizing openair package in R software (Carslaw and Ropkins, 2012). The frequency pattern of wind along with its direction and speed are shown here for entire sampling dates during daylights (aka daytime: from 07:00 h to 17:00 h local time, top panel) and nighttime (from 00:00 h to 06:59 h and from 17:01 h to 23:59 h; bottom panel). The winds for each one of the sampling dates (i.e., from 08th to 16th Nov) are shown under the dedicated panel both for the daylight and nighttime periods (Figure 2).
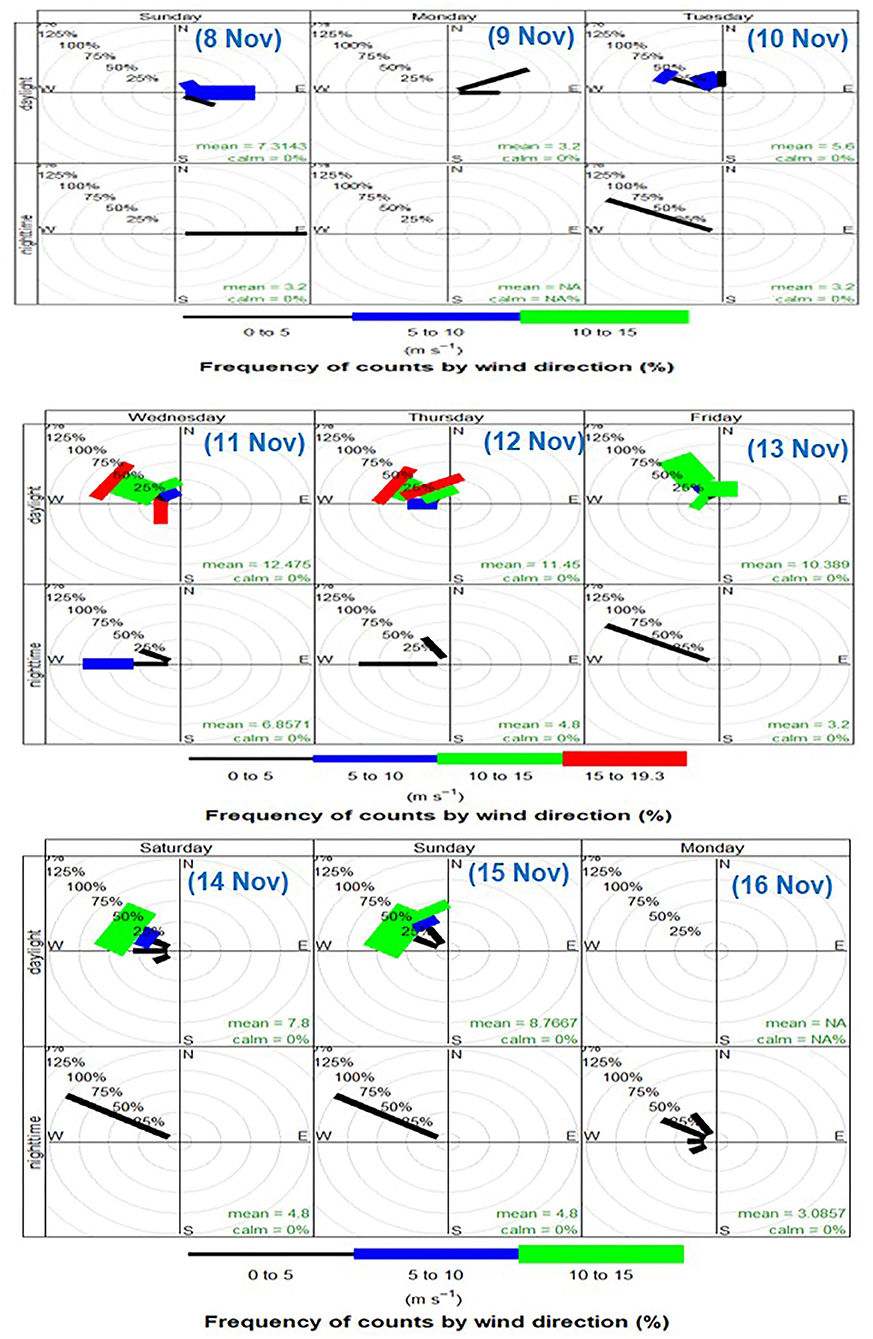
Figure 2. Wind-rose diagram showing day-night variability pattern of wind speed (shown by different colors) and direction frequencies during the entire campaign.
During the entire campaign, the winds were calm for most of the nighttime hours (80–93%) with an average speed <1 m s−1, exception being on Diwali festival night (average speed = 3.2 m s−1). The wind patterns during daylight hours suggested that on 08th and 09th Nov (pre-Diwali) winds were easterly to north-easterly whereas on the 10th Nov (also a pre-Diwali period) the wind-direction changed to north–to–north-westerly. During the entire pre-Diwali period (08th−10th Nov) the daytime wind speed was <10 m s−1. More interestingly, the winds on Diwali festival day (11th Nov) continued blowing from the north-west direction with some contributions from south direction too. As far as the wind speed is concerned, it was > 15 m s−1 with an average value of 11 m s−1 on the 11th Nov (Diwali festival daylight hours). During rest of the dates (post-Diwali from 12th to 16th Nov) the prevailed winds were mainly north-westerly both during daylight and nighttime hours (Figure 2). It is worthwhile mentioning that during October–November period the north-westerly winds favor transport of emissions from source-region of open biomass (paddy-residue) burning, active in upwind IGP, to the downind locations and marine atmospheric boundary layer over the Bay of Bengal (Kaskaoutis et al., 2014; Rajput et al., 2014). Furthermore, for the entire campaign, during daylight hours the winds were calm for <22% of the time, exception being on 10th Nov (calm wind: 33%). A day-night summary of meteorological conditions during pre-Diwali, Diwali, and post-Diwali periods are given in Table 2.
Fire-Count Imageries (Over the Source-Region of Biomass Burning Emissions in IGP) and Air-Mass Cluster Analysis
MODIS (Moderate Resolution Imaging Spectroradiometer sensor, onboard NASA Terra and Aqua satellite) fire-count imageries (spatial resolution: 10 km) showing an intense open biomass (agricultural-waste paddy-residue) burning activity in north-western part of during the study period are shown in Figure 3. This observation is quite consistent with earlier observations reporting massive emissions of air pollutants from agricultural-waste burning in upwind IGP (Rajput et al., 2014, 2018). The details on land-use activity pattern and mapping of source-region of biomass burning emissions in upwind IGP are adopted from previous studies and shown in the Supplementary Material. Furthermore, a GIS-based open-source software viz. TrajStat was utilized for the air-mass cluster analysis (Figure 3). The cluster analysis is a widely used tool to understand the influence of cluster of trajectories on ambient level of air pollutants at the receptor site (Bansal et al., 2019; Rajput and Gupta, 2020). Briefly, in the cluster analysis method, the measured air pollutant concentrations are assigned to the corresponding trajectories and the nearest trajectories are clustered according to an angle distance function (Sirois and Bottenheim, 1995). Relevant details on cluster analysis are given elsewhere (Wang et al., 2009). To carry out the cluster analysis, a 5-day air-mass back trajectories (AMBTs, GDAS 0.5°m, @ 1,000 m above ground level) data have been utilized. These AMBTs were retrieved from NOAA HYSPLIT (Hybrid Single-Particle Lagrangian Integrated Trajectory) model (Draxler and Rolph, 2003; Stein et al., 2015).
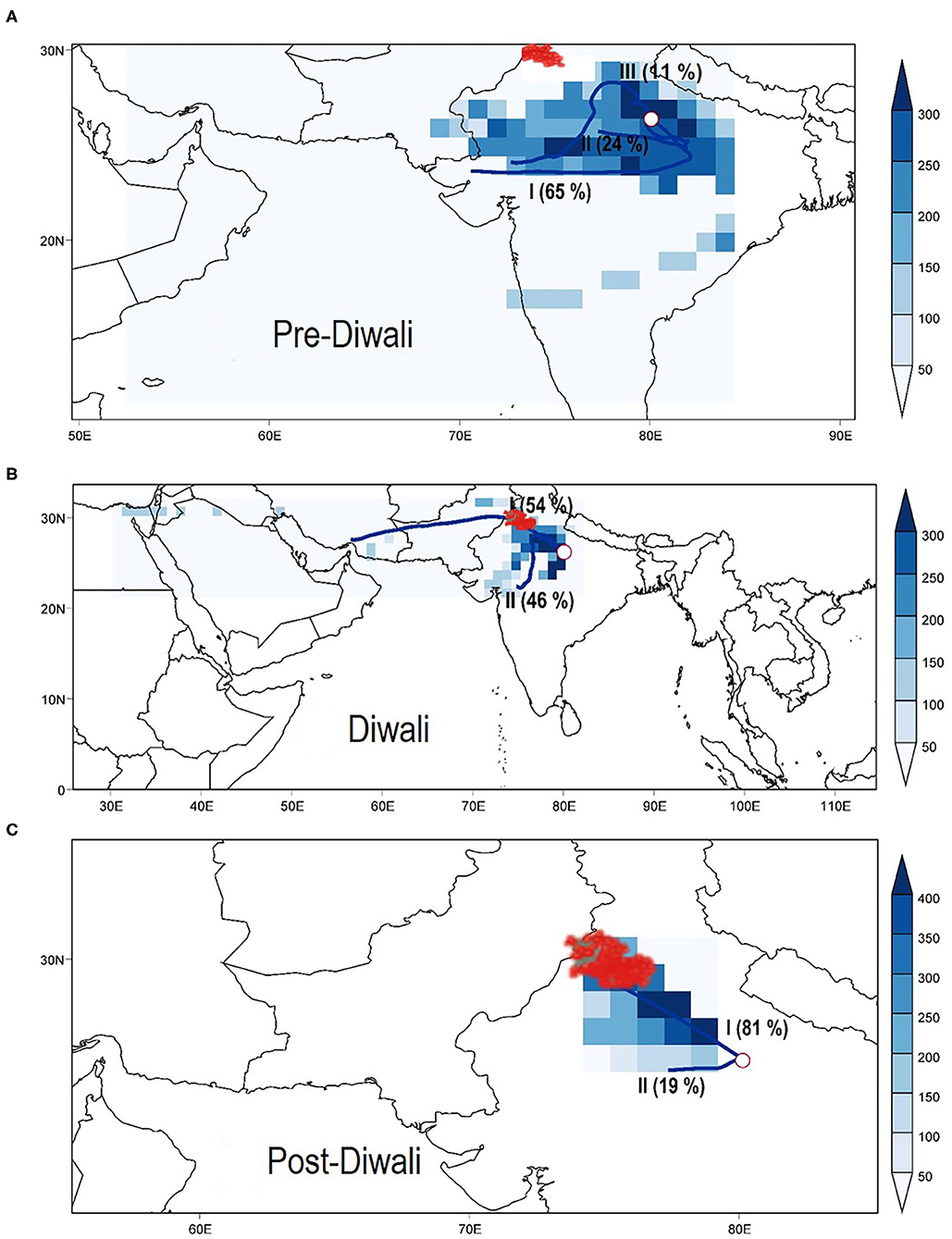
Figure 3. Air-mass cluster analysis and fire-count imageries (shown by red-circles, over source region of biomass burning) during (A) pre-Diwali, (B) Diwali, and (C) post-Diwali periods.
One of the observations from Figure 3 relates that if a trajectory cluster is passing more frequently through NW-direction to the receptor site then it is expected to have elevated levels of air pollutants from LRT-BB as compared to the case when trajectories were arriving from other directions. Let us now analyze the clusters shown in Figure 3. During Pre-Diwali period (Figure 3A), there was very little influence of NW-air masses (11%) but more importantly not passing through the source-region of biomass burning emission. It is important to mention here that these 11% NW-air masses were prevailed on 10th Nov. Furthermore, during the Post-Diwali period (Figure 3C), majority of air masses (81%) were originating from source-region of biomass burning emission and arriving from NW-direction to the receptor site. Summing up, clubbing the data set from our campaign, satellite observations on fire-counts along with the cluster analysis, it can be summarized that the receptor site (at Kanpur) was influenced with the LRT-BB emissions through NW-direction (from upwind IGP) mainly during post-Diwali and Diwali periods.
Co-variability Analysis of SB-PAHs, O3, and CNC
The co-variability features of SB-PAHs (n = 10,829), O3 (n = 10,951), and the sub-micron particles number concentrations (CNC, n = 9,444) are shown in Figure 4. It is evident from this figure that the high abundance of CNC (i.e., submicron particles, orange dots) was associated with higher concentration of O3 (blue dots) and with the lower concentration of SB-PAHs (black dots, Figure 4). Thus, field based observations revealed that peaks in O3 concentration were associated with dips in SB-PAHs concentration. This observation is much more pronounced during Diwali and post-Diwali period (i.e., from 11th to 16th Nov). Further features in this regard have been discussed in the following section. The summary of the data set is shown in Figure 5 and also given in Table 3. We have carried out statistical two-tailed t-test analysis to infer about difference in measured species concentrations during Diwali as compared to those in Pre-Diwali and Post-Diwali periods. Accordingly, comparing the concentrations during Diwali vs. Pre-Diwali it revealed that O3 (t = 11.1; p <0.0001), SB-PAHs (t = 2.4; p < 0.05), and CNC (t = 53.9; p < 0.0001) were significantly different during these periods. Furthermore, comparing the concentrations during Diwali vs. Post-Diwali periods it revealed that O3 (t = 4.5; p < 0.0001), SB-PAHs (t = 6.3; p < 0.05), and CNC (t = 28.7; p < 0.0001) were also significantly different during these periods.
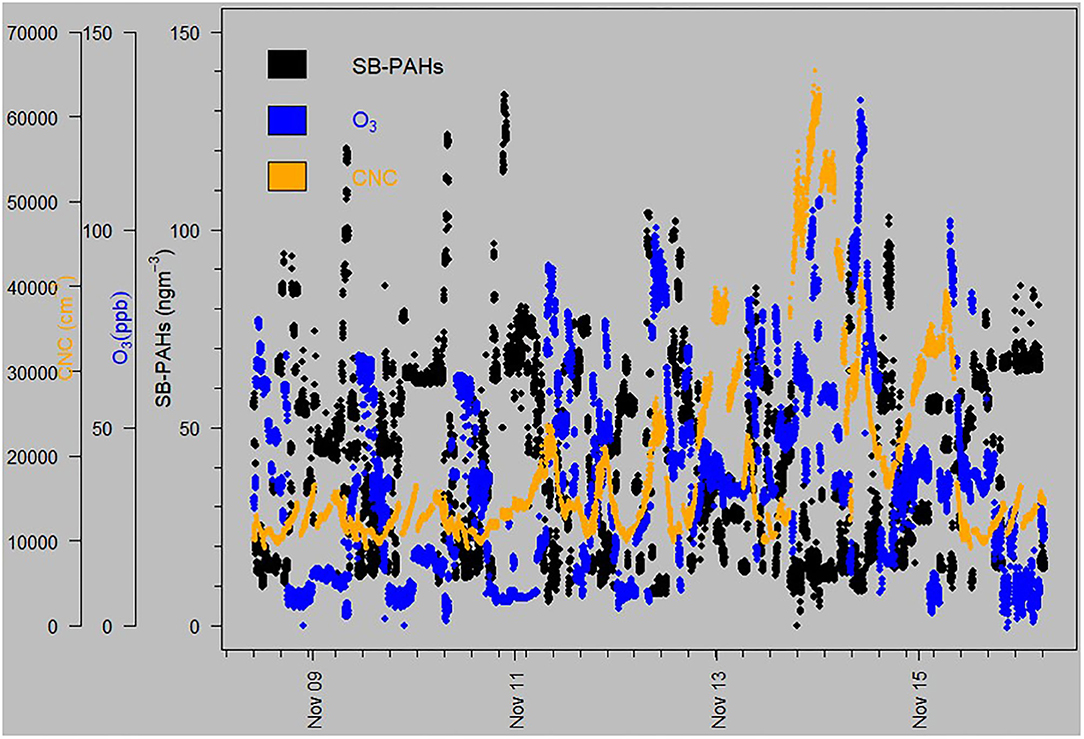
Figure 4. Diurnal profile of simultaneously measured surface-bound PAHs, ozone, and CPC-based particle's number concentration (CNC) during entire campaign.
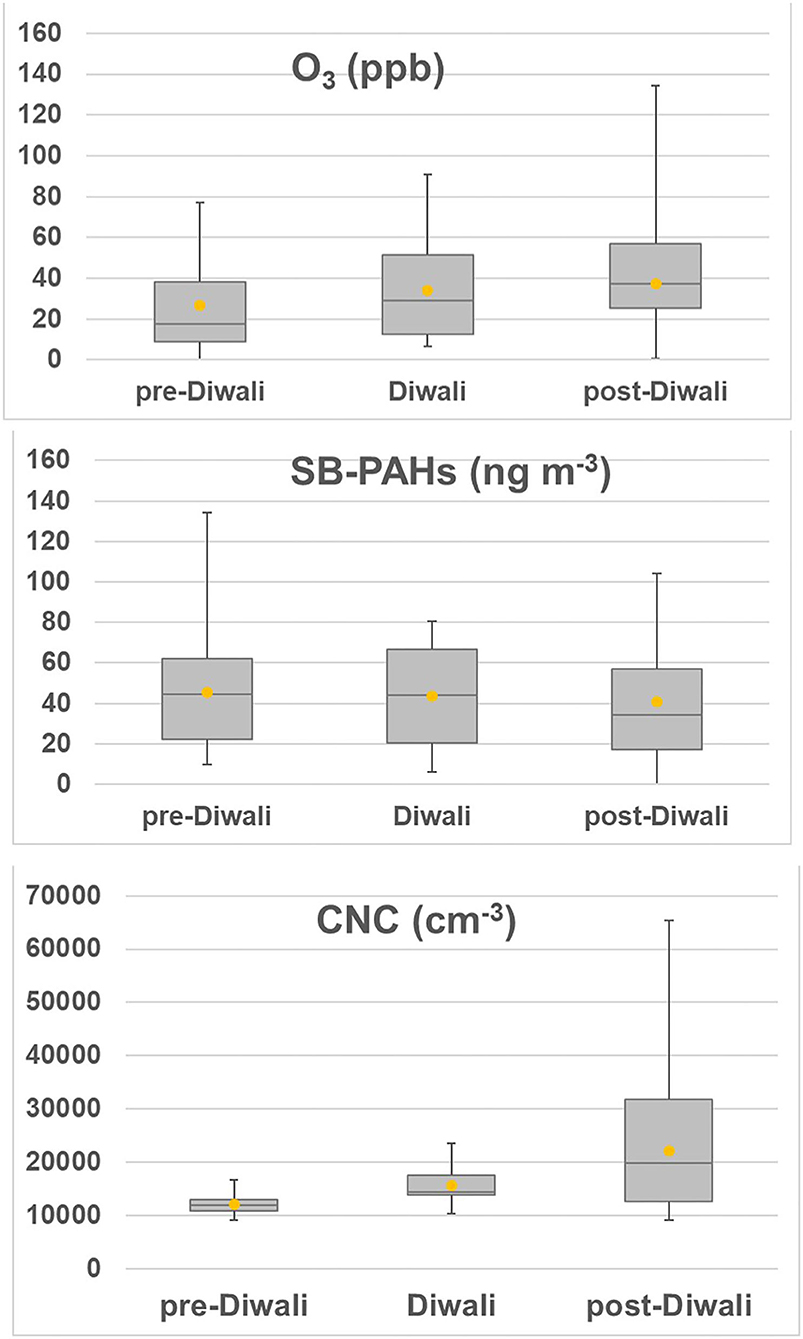
Figure 5. Boxplot showing a summary of overall variability [minimum, maximum, median (horizontal line), and mean (filled circle)] of O3, SB-PAHs, and CNC during pre-Diwali, Diwali, and post-Diwali periods.
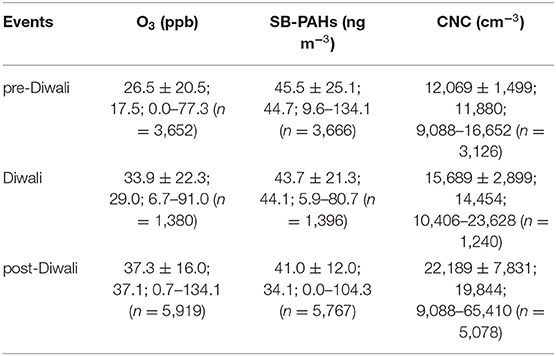
Table 3. Concentration [Avg.±SD; median; range (no. of samples)] of O3, SB-PAHs and CNC during the three events (pre-Diwali, Diwali, and post-Diwali).
Correlation Analyses of O3 vs. SB-PAHs as a Function of CNC
A 3-D linear correlation plot is shown in Figure 6 for three events (pre-Diwali, Diwali, and post-Diwali) and two periods (daylight and nighttime). The first assessment on maximum concentrations revealed that O3 was <150 ppb, SB-PAHs is <150 ng m−3 and CNC is <66 k cm−3 during the entire campaign (Figure 6). The CNC was <20 k cm−3 during pre-Diwali, <30 k cm−3 during Diwali and <66 k cm−3 during post-Diwali (CNC concentration pattern: post-Diwali >Diwali >pre-Diwali). Furthermore, the maximum concentrations of CNC were observed in nighttime hours during the post-Diwali period (12th−16th Nov). The correlation analyses revealed that during daylight hours, O3 and SB-PAHs were significantly anti-correlated on pre-Diwali (R2 = 0.57, p < 0.05), Diwali (R2 = 0.71, p < 0.05) and post-Diwali period (R2 = 0.71, p < 0.05). During nighttime hours, the anti-correlation was found to be weak during pre-Diwali (R2 = 0.09, p > 0.05) and post-Diwali period (R2 = 0.37, p > 0.05). However, the anti-correlation was found to be strong during Diwali (R2 = 0.71, p < 0.05). It is worthwhile mentioning here that a previous study has suggested the production of ozone from firecrackers burst (Attri et al., 2001). Summing up, the linear correlation analysis revealed that SB-PAHs and O3 have moderate-to-high association (p < 0.05) during all the period exception being that on pre-Diwali and post-Diwali nighttime while the anti-correlation was weak (p > 0.05). Furthermore, the CNC concentrations showed a large gradient in the correlation plot during Diwali and post-Diwali (varying from ~10 k to 65 k particles cm−3) as O3 increases and SB-PAHs decreases. However, the overall variability in CNC during the pre-Diwali period, varying from ~10 k to 16 k cm−3, is quite less as compared to those in Diwali and post-Diwali periods (also refer to Figure 5). It is important to note here that maximum CNC concentrations were observed during post-Diwali followed by Diwali and then pre-Diwali. Toward this, the explanation for higher abundance of CNC during Diwali period is attributable to additional input (i.e., besides urban background emissions) from FC burst and LRT-BB emissions. However, the higher CNC during post-Diwali period was by-and-large due to additional input from LRT-BB. It is worthwhile mentioning that the poor ventilation and shallower boundary layer height could also be responsible for a small fractional rise in the air pollutant's levels during the post-Diwali period (Table 2). The anti-correlation between SB-PAHs and O3 could be attributable to chemical oxidation of SB-PAHs by O3. It has been suggested previously that such chemical reactions have implications to enhanced CCN activity and toxicity of ambient aerosols (Perraudin et al., 2007; Kaiser et al., 2011).
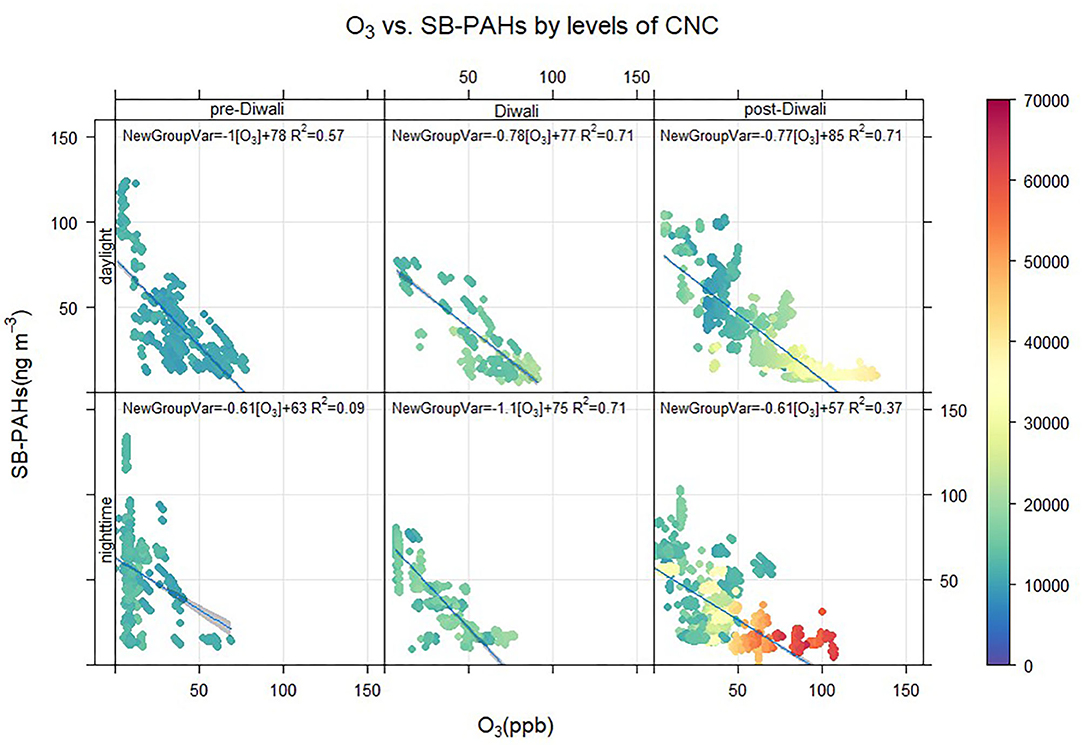
Figure 6. Linear regression analyses (p < 0.05, exception being on pre-Diwali and post-Diwali nighttime when p > 0.05) of O3 (X-axis, in ppb) vs. SB-PAHs (Y-axis, in ng m−3) by levels of CPC-based particle's number concentration (CNC, Z-axis in cm−3 shown on a scale at the right side of the plot) during pre-Diwali, Diwali, and post-Diwali periods for both daylight and nighttime hours.
Lenschow-Type Analysis: Assessment of Predominant Source Impact Over an Urban Background
Before we discuss on the Lenschow-type analysis and its application for quantifying emissions impact above the urban background level, let us first understand the need for applying this analysis. One of the simple ways to do that is to look into similar type of studies (e.g., from previous Diwali campaigns) and their conclusions drawn. There are two things which we need to reiterate here that (i) Diwali is celebrated during a day in October–November period and, (ii) almost the entire IGP experiences massive emisssions throughout these 2 months period from LRT-BB. Now, let us discuss briefly how the previous studies around Diwali period have assessed the change in pollutants concentration due to the FC burst. Previous researchers have documented very systematically the ambient concentrations of air pollutants [both particulate matter (PM) as well as trace gases] around Diwali period (Singh et al., 2010; Chatterjee et al., 2013; Ambade, 2018). For example, a study from an upwind IGP location at Delhi has documented pollutants concentrations for 6 years from 2002 to 2007 (Singh et al., 2010). Another study from a downwind location at Jamshedpur has documented PM and trace gases concentrations around Diwali period in 2014 (Ambade, 2018). From a further downwind location at Kolkata in IGP, a study has reported lots of species including metals, ionic composition in PM10 and SO2 around Diwali period in 2010 (Chatterjee et al., 2013). It is important to mention here that all these aforementioned studies have compared on a 1-to-1 basis the pre-/post-Diwali emissions with Diwali emissions and attributed the change in a particular polutant's concentration on Diwali due to the FC burst. These studies from various locations in IGP concluded that due to FC burst the pollutants concentrations do rise by a factor of 1.5–3 or 50–300%. However, if these previous studies (from the IGP) would have accounted for massive emissions from LRT-BB then the actual rise in pollutants concentrations due to the FC burst could have been quantified. With this regional background information, here we present an approach called the Lenschow-type analysis which would serve the purpose of quantifying emissions due to FC burst and LRT-BB over the regional background.
Lenschow et al. (2001) proposed an approach in 2001 to quantitatively estimate the contribution of PM sources spiking pollutants concentration above the regional background level. Their idea was basically to conduct a set of ambient PM measurements (spatially resolved) representative of regional background and those capturing records for episodic or long-range transported source components. Subsequently, grouping the data set into bins of sources corresponding to regional background emission and then estimating the contribution of episodic source and long-range transport was suggested in their study. The Lenschow-type analysis (Table 4) has been carried out to estimate quantitatively the impact of additional sources leading to increase (above the UB) the levels of air pollutants (Lenschow et al., 2001). It is worthwhile mentioning here that in this study we have applied the similar approach but on a temporally resolved ambient data set. Based on the Lenshchow-type analysis, we have estimated that only due to FC burst the CNC concentrations were increased by 54% whereas due to the impact from LRT-BB the CNC concentrations were increased by 86% over the urban background concentration level (at the receptor site). Thus, LRT-BB emissions appeared to be the major source (as evident from the increase in CNC concentrations by 86% than the urban background) of air pollution during the study period and results in enhancing the CNC burden by a facor of 1.6 when compared to that with the FC burst. Likewise, Lenschow-type analysis further revealed that O3 concentrations increased by 31% and 12% due to the LRT-BB and FC burst, respectively, over the urban background emission. However, in sharp contrast the SB-PAHs concentrations were found to be decreased by 2 and 6% (over the urban backgound emission), respectively, during the LRT-BB emission and FC burst (Table 4). Lenschow-type analysis also revealed that the urban background concentration of daily PM2.5 was 286 μg m−3 (Table 4). The FC burst and LRT-BB increased the daily PM2.5 concentration by 11% and 36%, respectively over its urban background level (286 μg m−3) (Figure 7). The application of Lenschow-type analysis in this study from IGP has led to the new insights of estimating the impact of additional sources spiking the pollutants concentrations over typical emissions within an urban agglomeration.
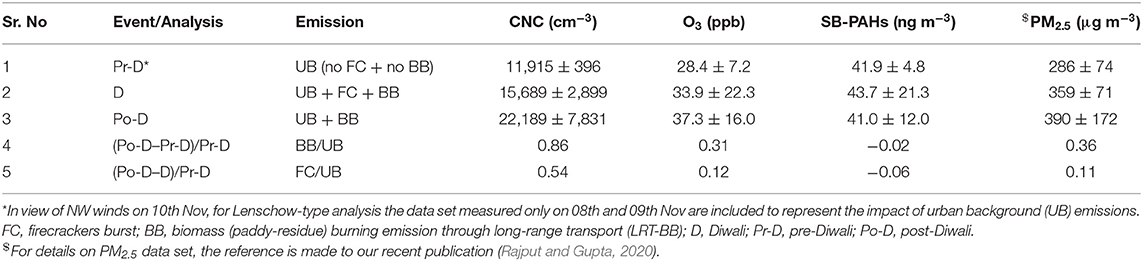
Table 4. Lenschow-type analysis to estimate episodic source impact over the local background emission in central IGP at Kanpur.
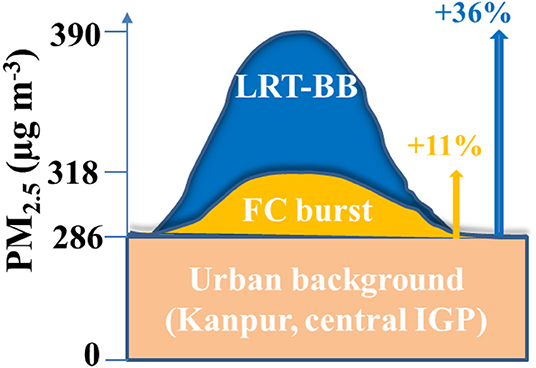
Figure 7. Sources contributions schematic (deduced from Lenschow-type analysis) over an urban agglomeration (Kanpur) in central Indo-Gangetic Plain.
Conclusions
In this study, we have conducted a 9 day (from 8th to 16th Nov 2015) long real-time (high-resolution: 1 min, n ≈ 10 k data points) measurement campaign assessing the diurnal profiles of SB-PAHs, O3 and CNC during pre-Diwali (8th−10th Nov), Diwali (11th Nov) and post-Diwali periods (12th−16th Nov). Wind analysis indicated that initially on 08th and 09th Nov the winds were easterly–to–north-easterly with intensity (mostly <5 m s−1) whereas from 10th onwards the winds were predominantly north-westerly (varying from 10 m s−1 to > 15 m s−1). A quite similar inference has been made based on the air-mass cluster analysis. The source-region of large-scale post-harvest agricultural-waste burning emissions lies in the north-west direction of the study region. Under prevailing NW winds, the LRT-BB emissions appeared to change the atmospheric composition and chemistry over the central IGP. Higher concentrations of CNC, PM2.5, and O3 during Diwali are attributable to the additional input from FC burst, and substantial contribution from LRT-BB emissions. However, higher concentrations of CNC, PM2.5, and O3 on post-Diwali period are mainly due to the LRT-BB emissions.
The daily average concentrations of O3 and CNC exhibited a quite similar pattern: post-Diwali >Diwali >pre-Diwali, whereas the SB-PAHs showed a different pattern with the highest concentration during pre-Diwali >Diwali >post-Diwali (trends are confirmed based on the two tailed t-test). Their diurnal profile also relates to the similar findings that when CNC and O3 concentrations peaked up then the SB-PAHs showed a dip and vice-versa. The daylight and nighttime linear correlation analyses of SB-PAHs and O3 as a function of CNC were carried out, and the results showed a moderate to strong anti-correlation during the entire study period, with exceptions being observed for pre-Diwali and post-Diwali nighttime hours when the correlation was weak. Based on the Lenshchow-type analysis, it has been estimated from this study that the CNC and O3 concentrations were increased (over UB) by 86 and 31%, respectively due to the LRT-BB emissions. Furthermore, only due to the FC burst the CNC and O3 concentrations were increased by 54 and 12%, respectively. Lenschow-type analysis further revealed that FC burst and LRT-BB increased the daily PM2.5 concentration over the urban agglomeration by 11% and 36%, respectively. However, the SB-PAHs concentrations were found to be decreased by 2 and 6% during LRT-BB emissions and FC burst, respectively. This study, highlighting the plausibility of surface-layer (heterogeneous-phase) reactivity of SB-PAHs with O3 has potential implications to enhanced particle's toxicity and CCN activity of aerosols over the IGP. Future studies would be required to examine the causal inference of the chemical reactivity of individual PAH.
Data Availability Statement
The raw data supporting the conclusions of this article will be made available by the authors, without undue reservation.
Author Contributions
PR and TG conceptualized this study. The data analysis presented in this paper was performed by PR. The manuscript has been written by PR. All authors were involved in collection of data during entire campaign used in this analysis. All authors discussed the results and commented on the manuscript.
Funding
TG was thankful to the Indian National Science Academy for partial funding support [INSA Grant # SP/YSP/113/2015(1091)] to conduct this study.
Conflict of Interest
The authors declare that the research was conducted in the absence of any commercial or financial relationships that could be construed as a potential conflict of interest.
Acknowledgments
Authors thank Prof. Shivam Tripathi for providing access to meteorological data from met-station situated nearby the sampling site inside the IITK campus. We are also thankful to Mr. Sanjay Gupta and Rajendra Maurya for technical support during successful conduct of this campaign. PR thanks to Onam Bansal (PhD, Punjabi University, Patiala) for sharing her expertise in cluster analysis. We express our sincere thanks to the reviewers for providing constructive comments and suggestions.
Supplementary Material
The Supplementary Material for this article can be found online at: https://www.frontiersin.org/articles/10.3389/frsc.2020.622050/full#supplementary-material
References
Ackerman, A. S., Toon, O. B., and Hobbs, P. V. (1994). Reassessing the dependence of cloud condensation nucleus concentration on formation rate. Nature 367, 445–447. doi: 10.1038/367445a0
Agarwal, A. K., Singh, A. P., Gupta, T., Agarwal, R. A., Sharma, N., Rajput, P., et al. (2018). Mutagenicity and cytotoxicity of particulate matter emitted from biodiesel-fueled engines. Environ. Sci. Technol. 52, 14496–14507. doi: 10.1021/acs.est.8b03345
Ambade, B. (2018). The air pollution during Diwali festival by the burning of fireworks in Jamshedpur city, India. Urban Clim. 26, 149–160. doi: 10.1016/j.uclim.2018.08.009
Andreae, M. O., and Ramanathan, V. (2013). Climate's dark forcings. Science 340, 280–281. doi: 10.1126/science.1235731
Attri, A. K., Kumar, U., and Jain, V. K. (2001). Formation of ozone by fireworks. Nature 411:1015. doi: 10.1038/35082634
Bansal, O., Singh, A., and Singh, D. (2019). Characteristics of Black Carbon aerosols over Patiala Northwestern part of the IGP: source apportionment using cluster and CWT analysis. Atmos. Poll. Res. 10, 244–256. doi: 10.1016/j.apr.2018.08.001
Carslaw, D. C., and Ropkins, K. (2012). Openair — an R package for air quality data analysis. Environ. Modell. Softw. 27–28, 52–61. doi: 10.1016/j.envsoft.2011.09.008
Chatterjee, A., Sarkar, C., Adak, A., Mukherjee, U., Ghosh, S. K., and Raha, S. (2013). Ambient air quality during Diwali festival over Kolkata – a mega-city in India. Aerosol Air Qual. Res. 13, 1133–1144. doi: 10.4209/aaqr.2012.03.0062
Che, H. C., Zhang, X. Y., Wang, Y. Q., Zhang, L., Shen, X. J., Zhang, Y. M., et al. (2016). Characterization and parameterization of aerosol cloud condensation nuclei activation under different pollution conditions. Sci. Rep. 6:24497. doi: 10.1038/srep24497
Devi, J. J., Gupta, T., Jat, R., and Tripathi, S. N. (2013). Measurement of personal and integrated exposure to particulate matter and co-pollutant gases: a panel study. Environ. Sci. Poll. Res. Int. 20, 1632–1648. doi: 10.1007/s11356-012-1179-3
Draxler, R. R., and Rolph, G. D. (2003). HYSPLIT (Hybrid Single-Particle Lagrangian Integrated Trajectory) Model Access via NOAA ARL READY Website. NOAA Air Resources Laboratory. Silver Spring, MD. Available online at: http://www.arl.noaa.gov/ready/hysplit4.html (accessed February 3, 2019).
George, C., Ammann, M., D'Anna, B., Donaldson, D. J., and Nizkorodov, S. A. (2015). Heterogeneous photochemistry in the atmosphere. Chem. Rev. 115, 4218–4258. doi: 10.1021/cr500648z
Hämeri, K., Koponen, I. K., Aalto, P. P., and Kulmala, M. (2002). The particle detection efficiency of the TSI-3007 condensation particle counter. J. Aerosol Sci. 33, 1463–1469. doi: 10.1016/S0021-8502(02)00090-3
Izhar, S., Rajput, P., and Gupta, T. (2018). Variation of particle number and mass concentration and associated mass deposition during Diwali festival. Urban Clim. 24, 1027–1036. doi: 10.1016/j.uclim.2017.12.005
Jethva, H., Torres, O., Field, R. D., Lyapustin, A., Gautam, R., and Kayetha, V. (2019). Connecting crop productivity, residue fires, and air quality over Northern India. Sci. Rep. 9, 16594. doi: 10.1038/s41598-019-52799-x
Joshi, M., Khan, A., Anand, S., and Sapra, B. K. (2016). Size evolution of ultrafine particles: differential signatures of normal and episodic events. Environ. Poll. 208, 354–360. doi: 10.1016/j.envpol.2015.10.001
Kaiser, J. C., Riemer, N., and Knopf, D. A. (2011). Detailed heterogeneous oxidation of soot surfaces in a particle-resolved aerosol model. Atmos. Chem. Phys. 11, 4505–4520. doi: 10.5194/acp-11-4505-2011
Kaskaoutis, D. G., Kumar, S., Sharma, D., Singh, R. P., Kharol, S. K., Sharma, M., et al. (2014). Effects of crop residue burning on aerosol properties, plume characteristics, and long-range transport over northern India. J. Geophys. Res. Atmos. 119, 5424–5444. doi: 10.1002/2013JD021357
Lal, S., Naja, M., and Subbaraya, B. H. (2000). Seasonal variations in surface ozone and its precursors over an urban site in India, Atmos. Environ. 34, 2713–2724. doi: 10.1016/S1352-2310(99)00510-5
Lenschow, P., Abraham, H. J., Kutzner, K., Lutz, M., Preuß, J. D., and Reichenbächer, W. (2001). Some ideas about the sources of PM10. Atmos. Environ. 35, S23–S33. doi: 10.1016/S1352-2310(01)00122-4
Maria, S. F., Russell, L. M., Gilles, M. K., and Myneni, S. C. (2004). Organic aerosol growth mechanisms and their climate-forcing implications. Science 306:1921. doi: 10.1126/science.1103491
Marr, L. C., Dzepina, K., Jimenez, J. L., Reisen, F., Bethel, H. L., Arey, J., et al. (2006). Sources and transformations of particle-bound polycyclic aromatic hydrocarbons in Mexico City. Atmos. Chem. Phys. 6, 1733–1745. doi: 10.5194/acp-6-1733-2006
Menon, S., Hansen, J., Nazarenko, L., and Luo, Y. (2002). Climate effects of black carbon aerosols in China and India. Science 297, 2250–2253. doi: 10.1126/science.1075159
Nguyen, T. K. V., Zhang, Q., Jimenez, J. L., Pike, M., and Carlton, A. G. (2016). Liquid water: ubiquitous contributor to aerosol mass. Environ. Sci. Technol. Lett. 3, 257–263. doi: 10.1021/acs.estlett.6b00167
Niessner, R., and Walendzik, G. (1989). The photoelectric aerosol sensor as a fast-responding and sensitive detection system for cigarette smoke analysis. Z. Anal. Chem. 333, 129–133. doi: 10.1007/BF00474022
Perraudin, E., Budzinski, H., and Villenave, E. (2007). Kinetic study of the reactions of ozone with polycyclic aromatic hydrocarbons adsorbed on atmospheric model particles. J. Atmos. Chem. 56, 57–82. doi: 10.1007/s10874-006-9042-x
Pöhlker, M. L., Ditas, F., Saturno, J., Klimach, T., de Angelis, I. H., Araùjo, A. C., et al. (2018). Long-term observations of cloud condensation nuclei over the Amazon rain forest – Part 2: variability and characteristics of biomass burning, long-range transport, and pristine rain forest aerosols. Atmos. Chem. Phys. 18, 10289–10331. doi: 10.5194/acp-18-10289-2018
Rajput, P., and Gupta, T. (2020). Instrumental variable analysis and atmospheric and aerosol chemistry. Front. Environ. Sci. 8, 1–13. doi: 10.3389/fenvs.2020.609846
Rajput, P., Mandaria, A., Kachawa, L., Singh, D. K., Singh, A. K., and Gupta, T. (2016). Chemical characterisation and source apportionment of PM1 during massive loading at an urban location in Indo-gangetic plain: impact of local sources and long-range transport. Tellus B: Chem. Phys. Meteorol. 68:30659. doi: 10.3402/tellusb.v68.30659
Rajput, P., Sarin, M., Sharma, D., and Singh, D. (2014). Characteristics and emission budget of carbonaceous species from post-harvest agricultural-waste burning in source region of the Indo-Gangetic Plain. Tellus B Chem. Phys. Meteorol. 66:21026. doi: 10.3402/tellusb.v66.21026
Rajput, P., Sarin, M. M., Rengarajan, R., and Singh, D. (2011). Atmospheric polycyclic aromatic hydrocarbons (PAHs) from post-harvest biomass burning emissions in the Indo-Gangetic Plain: isomer ratios and temporal trends. Atmos. Environ. 45, 6732–6740. doi: 10.1016/j.atmosenv.2011.08.018
Rajput, P., Singh, D. K., Singh, A. K., and Gupta, T. (2018). Chemical composition and source-apportionment of sub-micron particles during wintertime over Northern India: New insights on influence of fog-processing. Environ. Poll. 233, 81–91. doi: 10.1016/j.envpol.2017.10.036
Ramana, M. V., Ramanathan, V., Feng, Y., Yoon, S. C., Kim, S. W., Carmichael, G. R., et al. (2010). Warming influenced by the ratio of black carbon to sulphate and the black-carbon source. Nat. Geosci. 3, 542–545. doi: 10.1038/ngeo918
Rastogi, N., Singh, A., and Satish, R. (2019). Characteristics of submicron particles coming from a big firecrackers burning event: implications to atmospheric pollution. Atmos. Poll. Res. 10, 629–634. doi: 10.1016/j.apr.2018.11.002
Rudich, Y., Donahue, N. M., and Mentel, T. F. (2007). Aging of organic aerosol: bridging the gap between laboratory and field studies. Ann. Rev. Phys. Chem. 58, 321–352. doi: 10.1146/annurev.physchem.58.032806.104432
Ruehl, C. R., Davies, J. F., and Wilson, K. R. (2016). An interfacial mechanism for cloud droplet formation on organic aerosols. Science 351:1447–1450. doi: 10.1126/science.aad4889
Sahu, L. K., Tripathi, N., and Yadav, R. (2017). Contribution of biogenic and photochemical sources to ambient VOCs during winter to summer transition at a semi-arid urban site in India. Environ. Poll. 229, 595–606. doi: 10.1016/j.envpol.2017.06.091
Satish, R., and Rastogi, N. (2019). On the use of brown carbon spectra as a tool to understand their broader composition and characteristics: a case study from crop-residue burning samples. ACS Omega 4, 1847–1853. doi: 10.1021/acsomega.8b02637
Seki, K. (1989). Ionization energies of free molecules and molecular solids. Mol. Cryst. Liq. Cryst. Inc. Nonlinear Opt. 171, 255–270. doi: 10.1080/00268948908065800
Sharma, G., Sinha, B., Pallavi, N., Hakkim, H., Chandra, B. P., Kumar, A., et al. (2019). Gridded emissions of CO, NOx, SO2, CO2, NH3, HCl, CH4, PM2.5, PM10, BC and NMVOC from open municipal waste burning in India. Environ. Sci. Technol. 53, 4765–4774. doi: 10.1021/acs.est.8b07076
Singh, D. K., and Gupta, T. (2016). Effect through inhalation on human health of PM1 bound polycyclic aromatic hydrocarbons collected from foggy days in northern part of India. J. Hazard. Mater. 306, 257–268. doi: 10.1016/j.jhazmat.2015.11.049
Singh, D. P., Gadi, R., Mandal, T. K., Dixit, C. K., Singh, K., Saud, T., et al. (2010). Study of temporal variation in ambient air quality during Diwali festival in India. Environ. Monit. Assess. 169, 1–13. doi: 10.1007/s10661-009-1145-9
Sirois, A., and Bottenheim, J. W. (1995). Use of backward trajectories to interpret the 5-year record of PAN and O3 ambient air concentrations at Kejimkujik National Park, Nova Scotia. J. Geophys. Res. 100, 2867–2881. doi: 10.1029/94JD02951
Stein, A. F., Draxler, R. R., Rolph, G. D., Stunder, B. J. B., Cohen, M. D., and Ngan, F. (2015). NOAA's HYSPLIT atmospheric transport and dispersion modeling system. Bull. Am. Meteorol. Soc. 96, 2059–2077. doi: 10.1175/BAMS-D-14-00110.1
Keywords: PAHs, O3, Diwali, Indo-Gangetic Plain, Air pollution, Heterogeneous-phase chemical reactivity
Citation: Rajput P, Singh AK, Biswas K, Qadri AM and Gupta T (2021) Source Contribution of Firecrackers Burst vs. Long-Range Transport of Biomass Burning Emissions Over an Urban Background. Front. Sustain. Cities 2:622050. doi: 10.3389/frsc.2020.622050
Received: 27 October 2020; Accepted: 16 December 2020;
Published: 21 January 2021.
Edited by:
Konstantinos E. Kakosimos, Texas A&M University at Qatar, QatarReviewed by:
Leila Droprinchinski Martins, Federal Technological University of Paraná, BrazilSreekanth Vakacherla, Center for Study of Science, Technology and Policy—CSTEP, India
Copyright © 2021 Rajput, Singh, Biswas, Qadri and Gupta. This is an open-access article distributed under the terms of the Creative Commons Attribution License (CC BY). The use, distribution or reproduction in other forums is permitted, provided the original author(s) and the copyright owner(s) are credited and that the original publication in this journal is cited, in accordance with accepted academic practice. No use, distribution or reproduction is permitted which does not comply with these terms.
*Correspondence: Prashant Rajput, prashant.rajput@phfi.org; Tarun Gupta, tarun@iitk.ac.in
†Present address: Prashant Rajput, Centre for Environmental Health (CEH), Public Health Foundation of Indian, Gurugram, India