- Infrastructure Management Group, Institute for Construction and Infrastructure Management, ETH Zürich, Zurich, Switzerland
In order to be effective, road safety officers must have a complete overview of the accidents in their area of responsibility, including information pertaining to the location and severity of the accidents, and how the number of accidents are developing over time. Ideally, this information is stored in a Geographic Information System (GIS) enabled database, which helps to facilitate data processing and analysis, which enables improved understanding of the reasons for the accidents and the proposals of how to improve road safety. This paper presents a case study based on accident reports from the Zurich City Police. Using a joint GIS and time series analysis based on negative binomial regression, the data is analyzed to identify trends in accident development for several accident subgroups (e.g., bicycle accidents, senior citizen accidents, e-bike accidents) and specific locations. The subgroup of bicycle accidents will be discussed in more detail. The time series analysis is corrected for exposure (e.g., the increasing number of e-bikes) and forecasts the number of accidents which are likely to occur in the future. Significantly higher numbers of accidents than those expected serve as an early warning that further investigation, leading to possible interventions, is required. The case study shows that with this information, it is possible to identify both geographical areas and accident subgroups that have deviating patterns in accident numbers, and should be further investigated. For bicycle accidents, 4 out of 12 districts exceed the average accident trend by over 95% and 3 districts have an accident number that is over 10% higher than that district's forecast, with the highest being 33% above the already increasing accident trend. Other accident subgroups are presented in summary form. The results of the analysis allow consistent and automated analysis across all potential areas for improving road safety, helping to focus the efforts of road safety managers on those areas where their efforts are most effective.
1. Introduction
Accident analysis is a fundamental part of road safety management. The likelihood of occurrence of road accidents and their severity are influenced by various factors, whose effects are sometimes difficult to determine due to interactions between them. The effects of these factors also change through space and time. The determination of the need for road safety improvement interventions requires both a reliable data basis and suitable and effective methods for evaluating the data.
This paper shows how a joint GIS and time series analysis can be used to analyze the accident situation in a city. The first step is to correct the data for exposure (changes in number of vehicles and their specific mileage) to reflect changes in mobility behavior. The second step is to conduct a trend analysis to determine the accident situation and its significance. The results of these two steps are then used to detect geographically distributed irregularities in the accident situation and irregularities within the subgroups (e.g., bike accidents, senior citizen accidents, etc.) and thus can be used by road safety managers, to help them focus their accident prevention interventions on those areas where their efforts are most effective.
GIS based approaches provide valuable additional information by being able to detect areas with increased accident occurrences by statistical means and thus being able to extract spatial accident distribution models that provide further understanding of development of accident rates. This paper contributes to this approach by presenting a case study that uses a standard statistical model and standardized data, in order to have an overview on spatially distributed accident rate development, but also enables road safety officers with less in-depth statistical knowledge to get an overview on the accident development in their areas of responsibility and thus enables them to act in a focused way.
The data used in this work (all traffic accidents in the city of Zurich, Switzerland; from January 2004 to December 2018) was provided by the Department of Transport (DAV) of the city of Zurich. Zurich is the largest Swiss city with about 425,000 inhabitants and another 470,000 commuters (Stadt Zürich, 2018). The methodological insights gained are easily transferable to other cities.
The rest of this paper is structured as follows: First, a literature analysis is provided in section 2. The methodology is then explained (section 3), as well as how the methodology was used in the case study (section 4). Finally, the results and an outlook on possible future work are given in section 5.
2. Literature
The literature section is divided into three parts: (1) an overview of previous work in this field, (2) legal foundations, which provide a framework for the investigation in Zurich, for example by requiring certain statistical methods for accident analysis, and (3) references to the statistical methods used in this paper.
GISs have proven to be invaluable tools for traffic safety studies. For example, Petch and Henson (2000) used GIS information to investigate child traffic casualties, taking into consideration the spatial differences in traffic, socio-economic factors, etc. on a district-based level; Steenberghen et al. (2004) used point pattern techniques to identify accident-prone areas and show the impact of traffic-calming measures. To further improve GIS-based traffic safety studies, specialized spatial-statistical methods were developed to also present the spatially distributed aspects of information. Li et al. (2007) presented a Bayesian approach to identify and rank road segments based on temporal patterns of crash risks, Aguero-Valverde (2013) presented multivariate spatial models that have their roots in disease mapping, Zeng and Huang (2014) presented a Bayesian spatial joint model for crash prediction for an urban road network, Deublein et al. (2015) used a Bayesian network model to predict accidents on Swiss highways, and Garcia de Soto et al. (2018) presented a model using artificial neural networks to predict accident frequency for the same task. However, all the above methods require in-depth statistical knowledge to perform the analyzes presented in the literature.
In Switzerland, road safety is an important political issue that has led to the development of a stringent road safety program (Schweizerischer Bundesrat, 2010), which comprises a set of regulations and standards concerning statistical methods that are used in road safety assessments. A benefit of these regulations is that they create nationwide comparability of accident situations, which is an important basis for systematic evaluation. An overview of the regulations is provided in Table 1.
The norm SN 641 711 (Schweizerischer Verband der Strassen- und Verkehrsfachleute (VSS), 2015) requires 6 key questions to be answered about accidents: (1) How many, (2) where, (3) how, (4) when, (5) who, and (6) why. These questions are then divided further in total 26 “standard statistics” answering the questions. All of those should be answered using the statistical methods given in SN 640 008 (Schweizerischer Verband der Strassen- und Verkehrsfachleute (VSS), 2000). This norm pair has, however, been criticized as being too complicated because the given statistical methods (e.g., multivariate statistics for accident development) are tailored to experienced statisticians rather than road safety officers.
Based on the legal requirements, in particular the norm SN 641 711, and to find a compromise between statistical complexity and useability, the family of negative binomial (NB) regression models is chosen for this work as the basis for the statistical procedure. This family of regression models is based on the negative binomial distribution, but the models themselves slightly vary in their parametrizations. Hilbe (2011) summarizes the different models. The NB2 model was selected as suggested in the VSS Research Report 1634 (Schweizerischer Verband der Strassen- und Verkehrsfachleute (VSS), 2018).
In summary, GIS combined with the use of advanced statistical methods provides valuable insight into accident situation development. However, this is mainly tailored to experienced statisticians with substantial in-depth knowledge. The normative prescribed statistical methods in Switzerland try to find a balance between complexity and usability, building on an excellent data basis framework for making assessing the accident situations in Swiss cities more accessible to less statistically experienced persons. Similar methods can also be transferred to cities in other countries, and might help increase the overall awareness about traffic accident situations in areas without dedicated traffic accident statisticians.
3. Methodology
This section describes the general methodology of this paper before applying it to the case study in section 4.
3.1. Data Correction
3.1.1. Exogeneity Correction
Accident records from Zurich show a sharp increase in the number of accidents between 2014 and 2016 (Figure 1). This increase, however, is principally because of the changed reporting of property damage accidents. Before 2015, minor property damage accidents1 were not included in the accident records. From July 2015, however, all accidents (also including minor accidents) were recorded. This change lead to an increase of approximately 2,000 accidents per year. The data was adjusted to correct for the differences attributed to the different data collection methods. In the years following the change (i.e., 2016–2018), property damage accidents with the 2,000 smallest values were excluded. As the change took place in July 2015, i.e., mid year, only the 1,000 smallest property damage accidents were removed for 2015. This affected from 2015 to 2018 in total 366 or 6.4% of the bicycle accidents.
3.1.2. Exposure Correction
As mobility behavior in the city of Zurich has changed over time (Federal Statistical Office Switzerland, 2018), so has the exposure to accidents. For example, there has been an increase of 50% in bicycle trips between 2012 and 2018, whereas the frequency of bicycle accidents has increased by over 70%.
Exposure correction must be integrated into the model calculation to adjust for the increase in exposure. For this purpose, an index of the traffic volume for each vehicle type is created from the data of the automated traffic counting stations within the city of Zurich, which is based on a weighted average of the average frequencies of each vehicle type per counting station. Additional index values were calculated based on the overall traffic development, allowing an index value to be available for the years before the commissioning of the automated traffic counting stations (Stadt Zürich Tiefbau- und Entsorgungsdepartement (TED), 2018).
The indexing is performed using an indexing Equation (Equation 1). The accident counts in year 2012 stay the same, but the counts of vehicles in the other years are adapted using this indexing equation to adjust for exposure.
with
rA,i… Accident rate in year i,
nA,i… Number of accidents in year i, and
ηi… Index for year i with base year 2012.
3.2. Trend Analysis
The statistical methods used are described in a Swiss norm (see Table 1), and are therefore only presented here in summary form. The basis of the calculation is the negative binomial distribution shown in Equation (2). The equation is presented in alternative parametrization with r = 1/α, p = 1/(1+αμ), and (1 − p) = αμ/(1+αμ); as shown in Hilbe (2011).
with
y… response,
μ… mean, and
α… dispersion parameter.
The link function (a function that connects the predictor to the distribution function, and is also prescribed by the chosen statistical model) used in the calculation is shown in Equation (3).
with
βn… parameters, and
Xn… observations.
The negative binomial regression is performed using a generalized linear model in conjunction with an IRLS algorithm (Hilbe, 2011). The Pearson residuals are then calculated according to the procedure described in the Swiss norm. Values above 2 are defined as outliers and the corresponding observation points are marked for a more detailed analysis.
3.3. Unusual Accident Development
The results of the analysis can show areas with unusual accident development. For this, the accidents are divided into 12 different zones (corresponding to the districts of the city of Zurich). For these zones, a generalized linear model (GLM) is estimated using all but the last data point. Then, a 95% prediction range is estimated for this last data point using the bootstrap technique, to create several GLMs from the data.
Each GLM is created using a random sample from the empirical distribution, assuming the sample from the empirical distribution is correct and representative for the true distribution. This is done for n = 1, 000 samples, in order to have 1,000 GLMs, that can then be used to infer the statistical parameters for the accident rate.
The prediction range is then an interval, where the last data point should lie within the range with 95% confidence (i.e., an interval provided by 95% of the several GLMs, given the previous data points. If the last data point is outside of this interval for a particular zone, the zone and data point are flagged, indicating an accident rate in the specific zone outside of 95% of the GLMs' forecasts, signifying unusual accident development.
4. Case Study
This section presents the city of Zurich case study.
4.1. Overview
4.1.1. Data
This case study was conducted using accident data from the city of Zurich. It covers all road traffic accidents registered by the police in the area of the city of Zurich in the period from 01 January 2004 to 31 December 2018. Each observation corresponds to one accident. Several persons or objects may be involved in an accident. The accident data set contains 87 variables and 64,394 observations. In summary, the variables cover the following topics: accident location, date and time, weather, road type, and condition, means of transport involved, severity of the accident (fatalities, serious injuries, minor injuries, property damage), amount of property damage, main cause, type of accident, and whether children or elderly people were involved in the accident. For the model, the parameters for accident location and the accident timestamp are used directly, the other parameters are used to form subgroups.
4.1.2. Focus Area: Bike Accidents
For the sake of brevity, the results are presented here only for bicycle accidents. More results for other types of accidents can be found in Hermann (2019). From 2004 to 2018, 5,723 bicycle accidents were reported. These include 5,374 accidents with a classic bicycle, 109 accidents with at least one fast e-bike (up to 45 km/h) and 299 accidents with at least one slow e-bike (up to 25 km/h). 59 accidents involved both at least one e-bike and at least one classic bicycle. Of the 5,374 accidents, 11.2% resulted in property damage, 68.4% in minor injuries, 20.0% in severe injuries, and 0.4% in fatal injuries. For the following analysis, classic bicycles and e-bikes (fast and slow) are examined together, as they were only reported separately from 2012 on. Additionally, the vulnerability of the riders is comparable because of the low level of protection, and they are also treated similarly in legal terms. For this analysis, all accident outcomes are grouped together. As minor and major injuries make up over 88% of all accident outcomes, the analysis inherently focuses on those. Because of the fortunately low number of fatalities (approx. 1.6 per year), a sufficient sample size for district-wise evaluation is not available.
4.2. Trend Analysis
The trend analysis as well as the values used to assess unusual accident development are estimated for all types of bicycle accidents. The results are shown in Figure 2, where Figure 2A shows the raw data of the accidents and Figure 2B the results of the trend analysis with all corrections.
The two subfigures of Figure 2 show the years 2007–2018 on the x-axis and either the accidents or the indexed accidents on the y-axis. The gray shaded area shows the 95% prediction interval for the GLMs (i.e., the area where 95% of the GLMs lie within) and the blue line shows 95% prediction range. Although both figures show an increasing trend in bicycle accidents (Figure 2A) respective indexed accident rate (Figure 2B), the differences are quite significant and underline the importance of correct exposure and exogeneity correction. The raw accident numbers (Figure 2A) show a significant trend of +10% per year, whereas the trend is only +2.3% per year when all of the corrections are applied (Figure 2B).
4.3. Unusual Accident Development
The discrepancies between the expected and actual values show that there is no abnormal increase in the number of expected accidents in 2018 (Figure 3). This is indicated by the fact that the last data point lies within the blue bar, which represents the 95% prediction range for the next observation.
However, when looking at the spatially distributed data, more information emerges, as shown in the next section.
4.4. GIS-Analysis
The result are shown in compact form for the 12 city districts of the city of Zürich in Figure 4. Additionally, the graphs for each district are provided in the Supplementary Material to this paper.
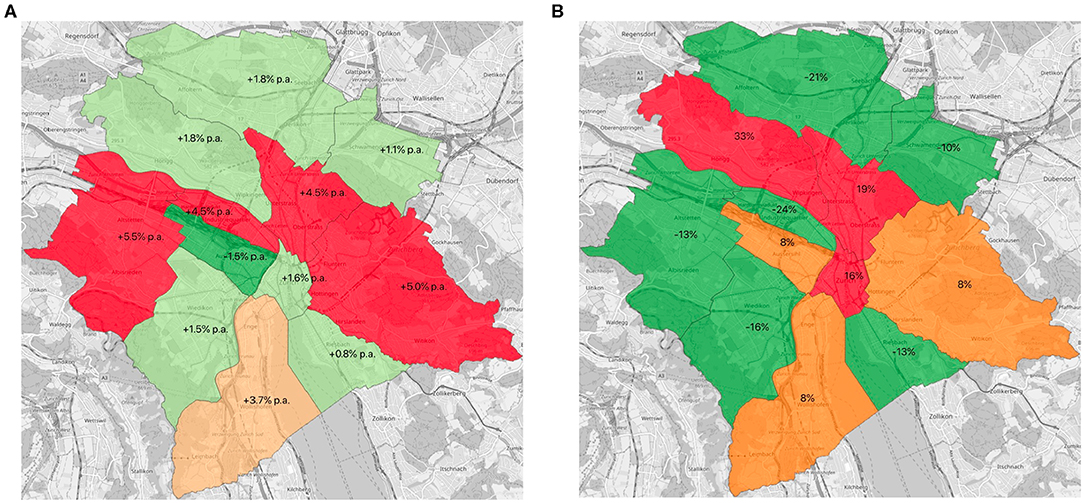
Figure 4. GIS-based display of results; (A): Accident trend, (B): Difference of predicted and actual accidents.
All 12 districts are displayed with their outline and the trend in percent increase/decrease, and colored according to the trend analysis in Figure 4A. Dark green indicates a decreasing trend in exposure and exogeneity-corrected bicycle accident rate, light green indicates an increasing trend that is below the average trend of +2.3% for Zurich overall, orange indicates a trend above the average trend of +2.3%, but below +4%, and red indicates a trend of +4% or more.
Figure 4B shows the difference between the predicted accidents and the observed accidents, both color-coded and in numbers. Dark green indicates areas where the actual accident numbers are more than 10% below the predicted numbers, orange indicates areas where the actual accident numbers are between 0 and 10% higher than the predicted numbers, and red indicates areas where the actual accident numbers exceed the prediction by more than 10%.
It can be seen that the corrected accident rate trend in the city center is below average (0.8, 1.6, or −1.5% per year), while in the outer districts there is a tendency for increasing trends, which also extends across the city, just north of the city center.
In the difference of the prediction and actual accidents map, it can be seen that in the district with 4.5% increase (District 10, plot in the Supplementary Material), there is an additional 19% excess of accident numbers beyond the predicted numbers, signifying that the actual accident development is even higher than the already high increasing trend, although still within the 95% prediction interval from the early warning system.
In the district with the 5.5% increasing trend (District 9, plot in the Supplementary Material), there is, however, an unusual drop in accidents noticeable, as can be seen in the supplementary material, where all prediction plots for the districts are provided.
This shows an unusual accident development as well, although a desired one.
4.5. Conclusions
Significant deviations from predicted accident rates found in specific areas of a city demonstrate that processes or events are taking place that have not been considered in the initial model. This should give the road safety manager cause for concern, and should help him/her focus his/her efforts in understanding the accident situation. For example, presented with the results above, the road safety manager might want to reassess the exposure and check whether or not all relevant exogenous aspects are integrated into the initial model. Additionally, he/her might want to look at unexpected changes in traffic patterns that may cause the geographical distribution of the anomalies.
The case study shows that GIS and standardized time series accident information helps road safety managers understand their accident situation, and therefore, will help increase road safety.
5. Summary and Outlook
Accidents result in property damage, injuries and deaths, and have indirect economic and societal impacts. It is advantageous to reduce them as much as possible. In this paper it is shown that through the use of GIS and time series information it is possible to identify both geographical areas and accident subgroups that show trends in accident numbers that deviate from those expected; something useful in focusing efforts to improve road safety. The results of the analysis allow consistent and automated identification of potential areas for improving road safety, helping to focus the efforts of road safety managers on those areas where their efforts are most effective.
Additionally, due to the use of standard models from statistics, road safety managers can easily add additional aspects (e.g., more detailed exposure correction from additional vehicle counting stations, traffic flow patterns,…) into the calculations and thus can improve the accuracy of the predictions and get more accurate results and a good basis for informed decisions (e.g., a split of E-bikes and normal bikes).
The case study presented showed an increase in bicycle accidents in the city of Zurich, and that a part of this increase was because of an increase in the number of bike trips, i.e., exposure. The time series analysis thus facilitates communication by being able to distinguish between a rise in accident numbers purely because of the higher number of bike trips and a rise in accident numbers because of other influences. This provides well-founded arguments for planning road safety interventions, if required. While this case study paper focuses on bicycle accidents, more in-depth analyzes of other accident groups can be found in Hermann (2019).
The insights gained through the analysis are not only useful in understanding the accident situation in the city of Zurich, but because of the standardized accident data collection and the standardization of the procedure on how to calculate the accident rate trend (i.e., the NB2 model) given in the norm, the same method can be used for all cities across Switzerland. By employing standard models from statistics, this method can also be used on an international scale with only minor adaptations if an appropriate data basis is available or can be built.
The results of the analysis apply specifically to the city of Zurich. Other cities facing similar challenges, however, can use the same method to improve their understanding of their accident situation.
Data Availability Statement
The data analyzed in this study were provided by the Traffic Department of the City of Zurich (Dienstabteilung Verkehr, Stadt Zürich) and cannot be made publicly available. Requests to access these data should be directed to Dr. Wernher Brucks, wernher.brucks@zuerich.ch.
Author Contributions
CK and JH contributed conception and design of the study. JH organized the database. CK and JH performed the statistical analysis and produced the result graphs and maps. CK wrote the manuscript. All authors contributed to manuscript revision, read and approved the submitted version.
Conflict of Interest
The authors declare that the research was conducted in the absence of any commercial or financial relationships that could be construed as a potential conflict of interest.
Acknowledgments
The authors would like to thank Markus Deublein of the Swiss Council for Accident Prevention and Wernher Brucks of the Department of Transport of the City of Zurich for providing the accident data and for their detailed and valuable feedback. Map data copyrighted OpenStreetMap contributors and available from https://www.openstreetmap.org.
Supplementary Material
The Supplementary Material for this article can be found online at: https://www.frontiersin.org/articles/10.3389/frsc.2020.00021/full#supplementary-material
Supplementary Figure 1. Bike Accidents, District 1.
Supplementary Figure 2. Bike Accidents, District 2.
Supplementary Figure 3. Bike Accidents, District 3.
Supplementary Figure 4. Bike Accidents, District 4.
Supplementary Figure 5. Bike Accidents, District 5.
Supplementary Figure 6. Bike Accidents, District 6.
Supplementary Figure 7. Bike Accidents, District 7.
Supplementary Figure 8. Bike Accidents, District 8.
Supplementary Figure 9. Bike Accidents, District 9.
Supplementary Figure 10. Bike Accidents, District 10.
Supplementary Figure 11. Bike Accidents, District 11.
Supplementary Figure 12. Bike Accidents, District 12.
Supplementary Figure 13. Bike Accidents, Zurich (all districts).
Footnote
1. ^Despite lacking a formal definition, Dr. Wernher Brucks (Head of Traffic Safety, City of Zurich Traffic Department) stated that the widely used definition of a minor property damage accident corresponds to an accident resulting in property damage of less than CHF 500.
References
Aguero-Valverde, J. (2013). Multivariate spatial models of excess crash frequency at area level: case of Costa rica. Accident Anal. Prevent. 59, 365–373. doi: 10.1016/j.aap.2013.06.014
Bundesamt für Strassen (ASTRA) (2017). Fachapplikation Verkehrsunfälle (VU) – Instruktionen zum Ausfüllen des Unfallaufnahmeprotokolls (UAP) 2018.
Deublein, M., Schubert, M., Adey, B. T., and Garcia de Soto, B. (2015). A Bayesian network model to predict accidents on swiss highways. Infrastruct. Asset Manage. 2, 145–158. doi: 10.1680/jinam.15.00008
Federal Statistical Office Switzerland (2018). Mobility and Transport. Available online at: https://www.bfs.admin.ch/bfs/de/home/statistiken/mobilitaet-verkehr/personenverkehr/leistungen.assetdetail.11207451.html
Garcia de Soto, B., Bumbacher, A., Deublein, M., and Adey, B. T. (2018). Predicting road traffic accidents using artificial neural network models. Infrastruct. Asset Manage. 5, 132–144. doi: 10.1680/jinam.17.00028
Hermann, J. S. (2019). Zeitreihenanalyse von unfalldaten zur identifikation von auffälligkeiten im unfallgeschehen der stadt Zürich (Master's thesis). ETH Zürich, Zurich, Switzerland.
Li, L., Zhu, L., and Sui, D. Z. (2007). A GIS-based Bayesian approach for analyzing spatial-temporal patterns of intra-city motor vehicle crashes. J. Transport Geogr. 15, 274–285. doi: 10.1016/j.jtrangeo.2006.08.005
Petch, R., and andHenson, R. (2000). Child road safety in the urban environment. J. Transport Geogr. 8, 197–211. doi: 10.1016/S0966-6923(00)00006-5
Schweizerischer Bundesrat (2010). Botschaft zu Via sicura, Handlungsprogramm des Bundes f ür mehr Sicherheit im Strassenverkehr (BBI 20108447). Available online at: https://www.admin.ch/opc/de/federal-gazette/2010/8447.pdf
Schweizerischer Verband der Strassen- und Verkehrsfachleute (VSS) (2000). SN 640 008 Strassenverkehrsunfälle. VSS.
Schweizerischer Verband der Strassen- und Verkehrsfachleute (VSS) (2015). SN 641 711 Strassenverkehrssicherheit. VSS.
Schweizerischer Verband der Strassen- und Verkehrsfachleute (VSS) (2018). VSS-Forschungsprojekt Nr. 1634.
Stadt Zürich (2018). Zürich in Zahlen. Available online at: https://www.stadt-zuerich.ch/portal/de/index/portraet_der_stadt_zuerich/zuerich_in_zahlen.html
Steenberghen, T., Dufays, T., Thomas, I., and Flahaut, B. (2004). Intra-urban location and clustering of road accidents using GIS: a belgian example. Int. J. Geograph. Inform. Sci. 18, 169–181. doi: 10.1080/13658810310001629619
Keywords: GIS, time series, bicycle accidents, road safety, accident development
Citation: Kielhauser C, Herrmann JS and Adey BT (2020) Identifying Changes in Bicycle Accident Trends Using GIS and Time Series Information in the City of Zürich. Front. Sustain. Cities 2:21. doi: 10.3389/frsc.2020.00021
Received: 18 March 2020; Accepted: 01 May 2020;
Published: 28 May 2020.
Edited by:
Krzysztof Goniewicz, Military University of Aviation, PolandReviewed by:
Meleckidzedeck Khayesi, World Health Organization, SwitzerlandVolkan Emre UZ, Izmir Institute of Technology, Turkey
Copyright © 2020 Kielhauser, Herrmann and Adey. This is an open-access article distributed under the terms of the Creative Commons Attribution License (CC BY). The use, distribution or reproduction in other forums is permitted, provided the original author(s) and the copyright owner(s) are credited and that the original publication in this journal is cited, in accordance with accepted academic practice. No use, distribution or reproduction is permitted which does not comply with these terms.
*Correspondence: Clemens Kielhauser, kielhauser@ibi.baug.ethz.ch
†Present address: Julia S. Herrmann, Center of Competence Crowd Management, Mobility Hub and Facility Management, Infrastructure, Swiss Federal Railways, Bern, Switzerland