- 1Department of Biomedical Sciences, School of Dental Medicine, University of Nevada Las Vegas, Las Vegas, NV, United States
- 2Center for Oral Health Research, College of Dentistry, University of Kentucky, Lexington, KY, United States
- 3Division of Periodontology, College of Dentistry, University of Kentucky, Lexington, KY, United States
The epithelial barrier at mucosal sites comprises an important mechanical protective feature of innate immunity, and is intimately involved in communicating signals of infection/tissue damage to inflammatory and immune cells in these local environments. A wide array of antimicrobial factors (AMF) exist at mucosal sites and in secretions that contribute to this innate immunity. A non-human primate model of ligature-induced periodontitis was used to explore characteristics of the antimicrobial factor transcriptome (n = 114 genes) of gingival biopsies in health, initiation and progression of periodontal lesions, and in samples with clinical resolution. Age effects and relationship of AMF to the dominant members of the oral microbiome were also evaluated. AMF could be stratified into 4 groups with high (n = 22), intermediate (n = 29), low (n = 18) and very low (n = 45) expression in healthy adult tissues. A subset of AMF were altered in healthy young, adolescent and aged samples compared with adults (e.g., APP, CCL28, DEFB113, DEFB126, FLG2, PRH1) and were affected across multiple age groups. With disease, a greater number of the AMF genes were affected in the adult and aged samples with skewing toward decreased expression, for example WDC12, PGLYRP3, FLG2, DEFB128, and DEF4A/B, with multiple age groups. Few of the AMF genes showed a >2-fold increase with disease in any age group. Selected AMF exhibited significant positive correlations across the array of AMF that varied in health and disease. In contrast, a rather limited number of the AMF significantly correlated with members of the microbiome; most prominent in healthy samples. These correlated microbes were different in younger and older samples and differed in health, disease and resolution samples. The findings supported effects of age on the expression of AMF genes in healthy gingival tissues showing a relationship to members of the oral microbiome. Furthermore, a dynamic expression of AMF genes was related to the disease process and showed similarities across the age groups, except for low/very low expressed genes that were unaffected in young samples. Targeted assessment of AMF members from this large array may provide insight into differences in disease risk and biomolecules that provide some discernment of early transition to disease.
Introduction
The oral mucosa is a critical protective interface between external and internal environments and serves as a barrier to the myriad of microbial species in the oral microbiome. Due to the inherent structure where hard tissues (i.e., teeth) break through an intact epithelial barrier, this anatomical region is one where there is a significant risk of bacterially induced infection and inflammation.
To help manage this continual septic environment, a diverse family of antimicrobial factors is produced, which defend the oral cavity and other mucosal surfaces of the body. The oral cavity is home to the second-most diverse microbiome in humans, and the health of the mouth is influenced by the presence of autochthonous bacteria, as well as by intrinsic and extrinsic host factors. Prior to the evolution of adaptive immunity, innate immunity played a role as the principal defense system. The innate immune system augmented the physical and chemical barriers by producing antimicrobial biomolecules (antimicrobial factors; AMF) with functionality against Gram-positive and negative bacteria, parasites, fungi, and viruses [1–3]. Thus, these factors, as a first line of defense, have multiple functions and properties that influence aspects of innate defenses and contribute to regulation of colonization by microorganisms.
The host expresses these protective molecules from immune and non-immune cells that are released into the local environment to act rapidly to combat invasion and infection with bacteria and other microorganisms. Oral epithelial cells, salivary glands and neutrophils secrete a wide profile of antimicrobial gene products detected in gingival crevicular fluid and saliva [2, 4–9]. Many of these AMFs are also highly concentrated in GCF providing a local microenvironment enriched in factors to help control the supra- and subgingival microbiomes [1, 3, 10]. Nevertheless, mechanistic considerations of this system support that the antimicrobial capacity of oral fluids permits and even enhances colonization of the oral cavity by various microorganisms, but prevents extensive microbial overgrowth of the oral and oropharyngeal tissues.
The mechanism of action of these AMFs against microbes and pathogens is attributed to the disruption of the microbial cell membrane [11–13], competition for nutrients [14–16], and microbial aggregation and mechanical or biological removal [17–19]. Also of importance is evidence regarding substantial variation between different species of oral bacteria and even strains of the same species in susceptibility to individual AMFs, such as LL-37 (CAMP) and hBD3 [1, 20–22]. In particular, major pathobionts including P. gingivalis, A. actinomycetemcomitans and F. nucleatum have shown relative resistance to some of these AMFs [23–26]. Thus, documenting the portfolio of the AMFs that are produced/released under different stimuli, as well as modulating influences (e.g., smoking, diabetes, nutrition etc.) will help in understanding the variation in disease expression, progression, and risk for periodontal diseases [13, 20].
The aim of this study was to map the gingival tissue AMF profile to determine alterations in disease and lesion resolution, as well as the impacts of age on this profile. The hypothesis of this study was that the gingival tissues would present an AMF expression profile with patterns affected by age and with specific features of AMF responses that would be correlated with certain bacteria or clusters/complexes of bacteria.
Materials and Methods
Animals and Diet
Rhesus monkeys (Macaca mulatta) (n = 36; 17 male, 19 female) housed at the Caribbean Primate Research Center at Sabana Seca, Puerto Rico were examined for periodontal health [27–29] and were used to determine the results from a ligature-induced periodontitis model. Young (≤ 3 years); adolescent (3–7 years); adult (12–16 years); and aged (18–23 years) were used in the study with 9 animals/group. The non-human primates were fed a 20% protein, 5% fat, and 10% fiber commercial monkey diet (diet 8773, Teklad NIB primate diet modified: Harlan Teklad, Madison, WI). The diet was supplemented with fruits and vegetables, and water was provided ad libitum in an enclosed corral setting.
As we have reported previously the protocol was approved by the Institutional Animal Care and Use Committees (IACUC) of the University of Puerto Rico and University of Kentucky and a ligature disease model was utilized [30]. The clinical examination included probing pocket depth (PPD) and bleeding on probing (BOP; 0–5 scale) [31]. Periodontal health was defined by mean Pocket Depth (PD) ≤ 3.0 mm and mean Bleeding on Probing (BOP) ≤ 1 (0–5 scale) in a full mouth examination excluding 3rd molars and canines [30]. Ligature-induced periodontal disease was initiated as we have previously reported [30] and gingival and subgingival plaque samples taken at 0.5, 1, and 3 months (Initiation/Progression), and 2 months after removal of ligatures and local factors (Resolution). Determination of periodontal disease at the sampled site was documented by assessment of the presence of BOP and probing pocket depth of >4 mm, as we have described previously [28]. Changes in these clinical measures of BOP and PPD in health, during disease initiation and progression, and with resolution in these age groups have been described previously [32]. Briefly, all animals demonstrated significant increases in BOP within 0.5 months, with somewhat elevated levels in the younger age groups. PPD increases were noted in all animals across all age groups with peak disease at 1 or 3 months. However, in both young and adolescent animals, the PPD measures for rate of change and peak levels of disease were less than in the adult and aged group. At resolution, both BOP and PPD measures decreased across all age groups, albeit generally remaining above measures for the baseline, healthy tissues.
Microbiome Analysis
Subgingival bacterial samples were obtained from the 36 animals by a curette and analyzed using a MiSeq instrument [33, 34] for the total composition of the microbiome from each sample [35, 36]. Sequences were clustered into phylotypes based on their sequence similarity and these binned phylotypes were assigned to their respective taxonomic classification using the Human Oral Microbiome Database (HOMD V13) (http://www.homd.org/index.php?name=seqDownload &file&type=R) as we have described previously [33]. Raw data were deposited at the NIH NCBI (BioProject ID PRJNA516659). Statistical differences of bacterial OTUs were determined with a t-test (p < 0.05). Correlations of OTUs within the oral microbiome were determined using a Pearson correlation coefficient analysis (p < 0.05). Correlations between the microbiome components and the gingival gene expression were determined only for matching samples derived from the same tooth in each of the animals. Matching samples with sufficient microbiome signals were compared for 46 samples in adults and 25 samples from the young group obtained at health and throughout the ligature model. As we have reported previously [33], of 396 OTUs identified in the non-human primate oral samples the targeted OTU selection for this study was 49 in the young/adolescent samples and 58 in the adult/aged samples with a relative abundance coverage of ~88% and ~91% of reads in all samples.
Gingival Tissue Sample Collection and mRNA Analysis
Gingival tissue samples of healthy and diseased sites were surgically collected and total RNA extracted for microarray analysis. The analytic hybridization was to the GeneChip® Rhesus Gene 1.0 ST Array (Affymetrix, Santa Clara, CA, USA) for the ligature-induced periodontitis model similar to methods we have described previously [27, 28, 37–39].
Data Analysis
Genes for the array of the innate antimicrobial factors (n = 114) targeted in the analysis as identified in Table 1. Beyond the specific Affymetrix probe annotation provided by the company, we annotated within the GeneChip® Rhesus Gene 1.0 ST Array additional probes for the host genes in this microarray. These included unannotated probes, whereby the nucleotide base sequence (https://www.affymetrix.com/analysis/index.affx#1_2) for each probe ID was subjected to a Blast (https://blast.ncbi.nlm.nih.gov/Blast.cgi) query that identified the M. mulatta gene ID with the greatest percent identify for the specific sequence. We selected the most targeted gene ID that always showed >90% identity and routinely >95% identity for annotating the gene list for the analysis.
The expression intensities across the samples were estimated using the Robust Multi-array Average (RMA) algorithm with probe-level quintile normalization, as implemented in the Partek Genomics Suite software version 6.6 (Partek, St. Louis, MO). The differential expression was initially compared using one way ANOVA across time points within an age group. For genes that had significant mean differences, two sample t-tests were used to investigate differences comparing baseline healthy to disease and resolution samples. Statistical significance was considered by a p-value < 0.05 adjusted for the number of correlations tested using a Benjamini-Hochberg procedure. The data have been uploaded into GEO accession GSE180588 (https://www.ncbi.nlm.nih.gov/gds). Correlation analyses were determined using a Pearson Correlation Coefficient with a p-value < 0.05 and adjusted for the number of correlations tested. Frequently a qPCR technique is used as a validation step for microarray outcomes. While we did not incorporate this approach into the analysis of this large array of AMF genes, we have previously published the use of qPCR to validate 28 genes from the larger microarray dataset including inflammatory cytokines and chemokines, apoptosis genes, and neuropeptides and receptors, and various transcription factors that demonstrated consistent directional changes, albeit, the magnitude of change was frequently somewhat greater with the qPCR [40–43].
Clustering analysis was conducted that included analysis of 18 AMF genes. A parametric t-test (α = 0.05) was used to identify the differentially expressed genes and bacteria at 0.5, 1, 3, and 5 months using baseline expression as control. Those that were differentially expressed, in at least at one of the time points in one or more age groups, were identified and entered into this analysis to examine clustering of both age groups and disease time points. The Principal Components Analysis (PCA) plot was created from results generated with BioVinci software (BioTuring Inc.). Transcript data of the 18 AMF genes that had high variance scores from PCA analysis were averaged across all animals in the same age category. Gene expression data was used to create the unrooted dendrogram by calculating the distance matrix with the Euclidean method followed by ward D2 linkage clustering method using the Ape package in R version 4.1.1. The data was normalized on a scale of 0 to 1, with the lowest value set at 0 and the highest value at 1, to generate the heatmap and rooted dendrogram for the cluster analyses. The agglomerative hierarchal cluster analyses were performed by calculating the distance matrix using the Squared Euclidean method and by average linkage (between-group) clustering method using SPSS v27 (IBM).
Results
Age Effects on Innate Antimicrobial Factor Gene Expression in Healthy Gingival Tissues
The normalized expression of transcripts for the 114 innate antimicrobial factors is demonstrated in Figure 1 with the genes stratified into high (n = 22), intermediate (n =29), low (n = 18), and very low (n = 45) expression based upon the healthy/baseline adult gingival specimens. In healthy/baseline gingival tissues, generally there were some observed differences in expression based upon age, including variations in both younger and aged animal samples. For example, DEFB4A/B, LTF, and TF were all decreased in the younger age groups, while CST6 (cystatin E/M), DEFB126, DEFB105/A/B, S100A7, and DEFB106A/B were lower in all age groups compared to adult levels. Figure 2 displays volcano plots of the AMF genes that were altered in young, adolescent, and aged samples compared to the adult levels in health. Seventeen genes were 2-fold and/or significantly different (p < 0.01) in the young animals. Fewer gene differences were seen in adolescent samples (n = 8), while 8 of the genes differed in the aged animals. Of note, APP, CCL28, DEFB113, DEFB126, FLG2, and PRH1 were differentially expressed in healthy gingival tissues across multiple age groups.
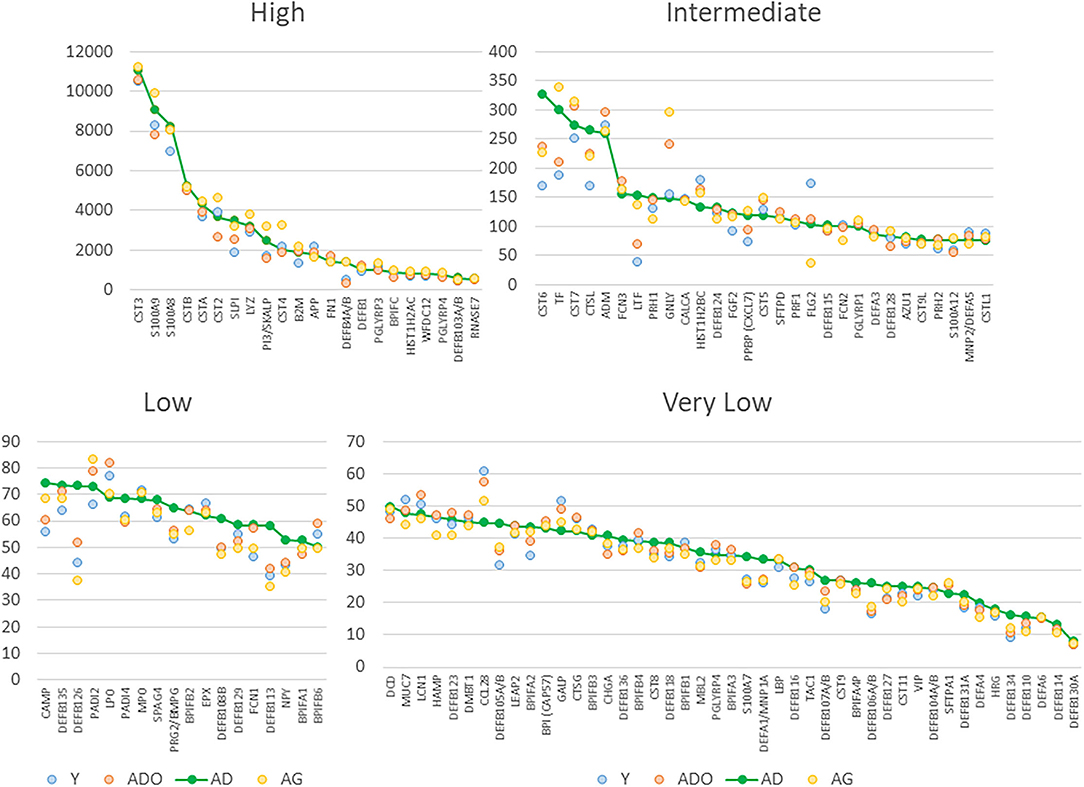
Figure 1. Normalized expression values for antimicrobial factors categorized as high, intermediate, low and very low expression levels based upon the adult healthy tissues. Each point denotes mean value from 9 animals/group. The genes are sequenced based upon the expression level in adult samples.
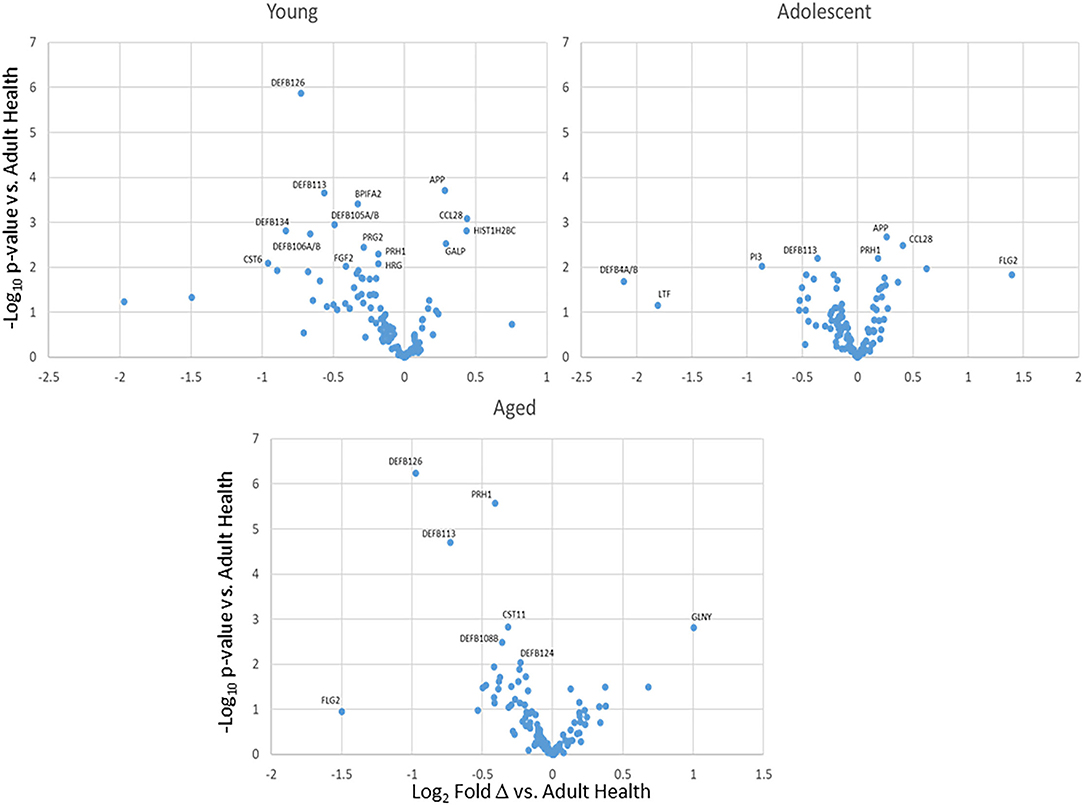
Figure 2. Volcano plots of differential AMF gene expression levels in health tissues from various age groups compared to healthy adults. Each point represent the mean value from 9 animals at baseline.
Age Effects on Innate Antimicrobial Factor Gene Expression in Progressing Periodontitis
Supplementary Figures 1A–D depict the alterations in transcript levels for the array of antimicrobial factor genes comparing baseline healthy levels to initiation (0.5 months), progression (1 and 3 months) and resolution (5 months) of periodontal lesions. Examination of fold differences showed a limited number of changes in the highly expressed genes in the young and adolescent animals. A greater number of these genes were affected in the adult and aged samples with skewing toward decreased expression of these genes across all age groups. WDC12, PGLYRP3, and DEF4A/B were decreased in disease across multiple age groups. Supplementary Figure 1B shows the differential expression of the intermediate expressed transcripts for these factors. Consistent findings were decreased FLG2, DEFB128, and increased levels of CTSL and LTF with disease. Low expression genes are shown in Supplementary Figure 1C with no differences in any of the genes in young animals. PADI4 and FCN1 were increased in the other groups, and DEFB126, DEFB113, DEFB106A/B, DEFB110, DEFB127, and DEFA6, were all decreased in the adult samples. Supplementary Figure 1D summarizes the differences in very low expression AMF genes compared to baseline healthy levels in the age groups. Minimal differences were noted in adolescent, adult and aged samples. A number of the AMF appeared decreased in the young samples with disease, albeit, these did not attain a 2-fold difference from baseline expression levels.
Figure 3 summarizes the antimicrobial factor genes that were significantly altered in the different age groups with disease and resolution. The data demonstrate 30 (young) and 12 (adolescent) AMF genes that were increased with FN1, PGLYRP3, ADM, CTSL, LTF, FLG2, and DEFB115 being represented in both these age groups. Of these AMF genes, BPIFC, FLG2, and multiple DEFB genes were decreased in expression with disease in one or both young and adolescent animals. A similar evaluation of these differences in the adult and aged animals is also displayed. First, the number of the affected transcripts was only about 40% of those in the youngest animals. Secondly, about 60% of these genes were significantly decreased in both age groups. Increased FN1, CTSL, ADM, PADI4, and FCN1 was noted in the adult and the aged groups, as well as most being elevated in the younger animal groups. Decreased levels of expression of multiple genes including DEFB4A/B, PGLYRP3, BPIFC, WFDC12, PI3/SKALP, DEFB128 were observed in adults and aged samples, with many of these also decreased in the young and adolescent samples during disease. As the repertoire of genes selected for this study were primarily related to epithelial cell biology, the distribution of the decreased AMF in the older groups versus young samples inferred that the more destructive disease process in the adult and aged animals was reflected in the apparent disruption of the breadth of AMF responses designed to maintain homeostasis.
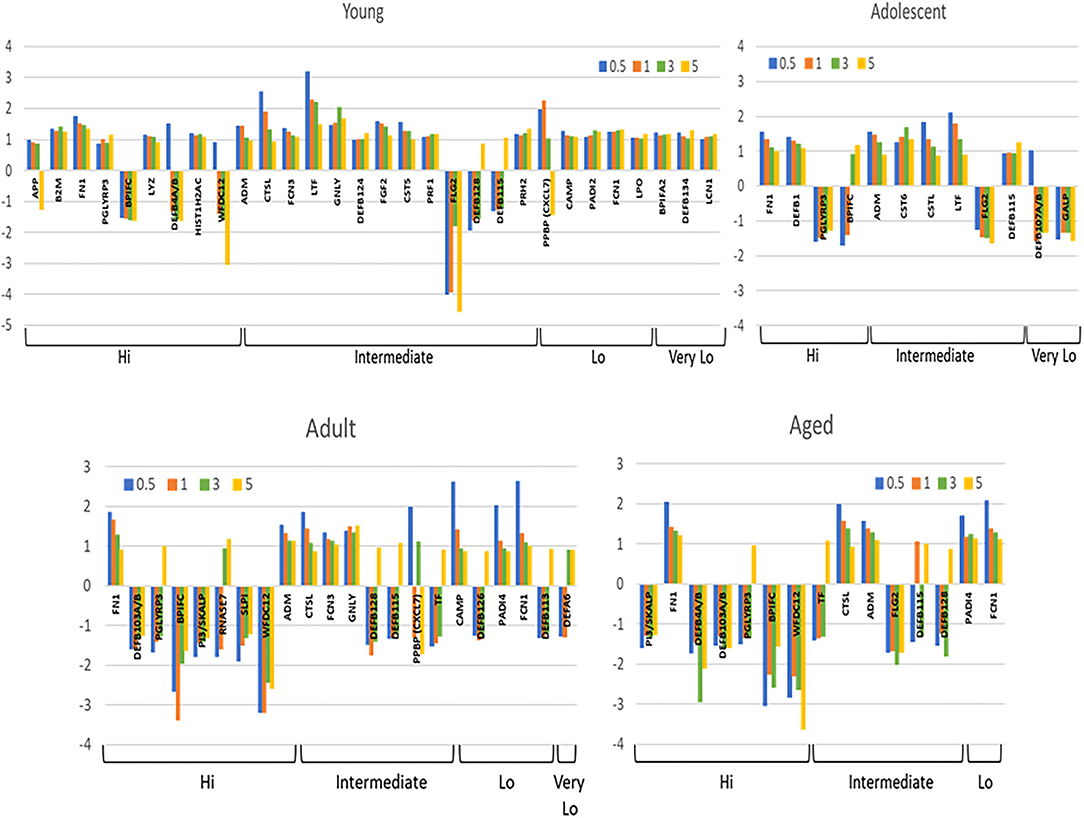
Figure 3. Array of AMF genes that were significantly (p < 0.05) differentially expressed at least at one time point during disease and resolution in tissues from the 4 age groups. Bars denote mean fold-difference from baseline at each time point. Genes are organized into expression level categories based upon Figure 1.
Figures 4A,B examined the concept that there could exist coincident programming and responses across these AMF genes in health and disease. In each case, a rather limited number of correlations across this array of AMF were noted in healthy tissue in any age group, suggesting a rather independent control of these responses to the local microbiome during health. In contrast, there were many more extensive correlations in gene expression across the AMF factors during disease. Interestingly, the frequency of these correlations increased from young through aged animals in the disease samples. Additionally, the pattern of these relationships was striking in the aged animals with a large frequency of significant positive correlations across a wide range of the AMF.
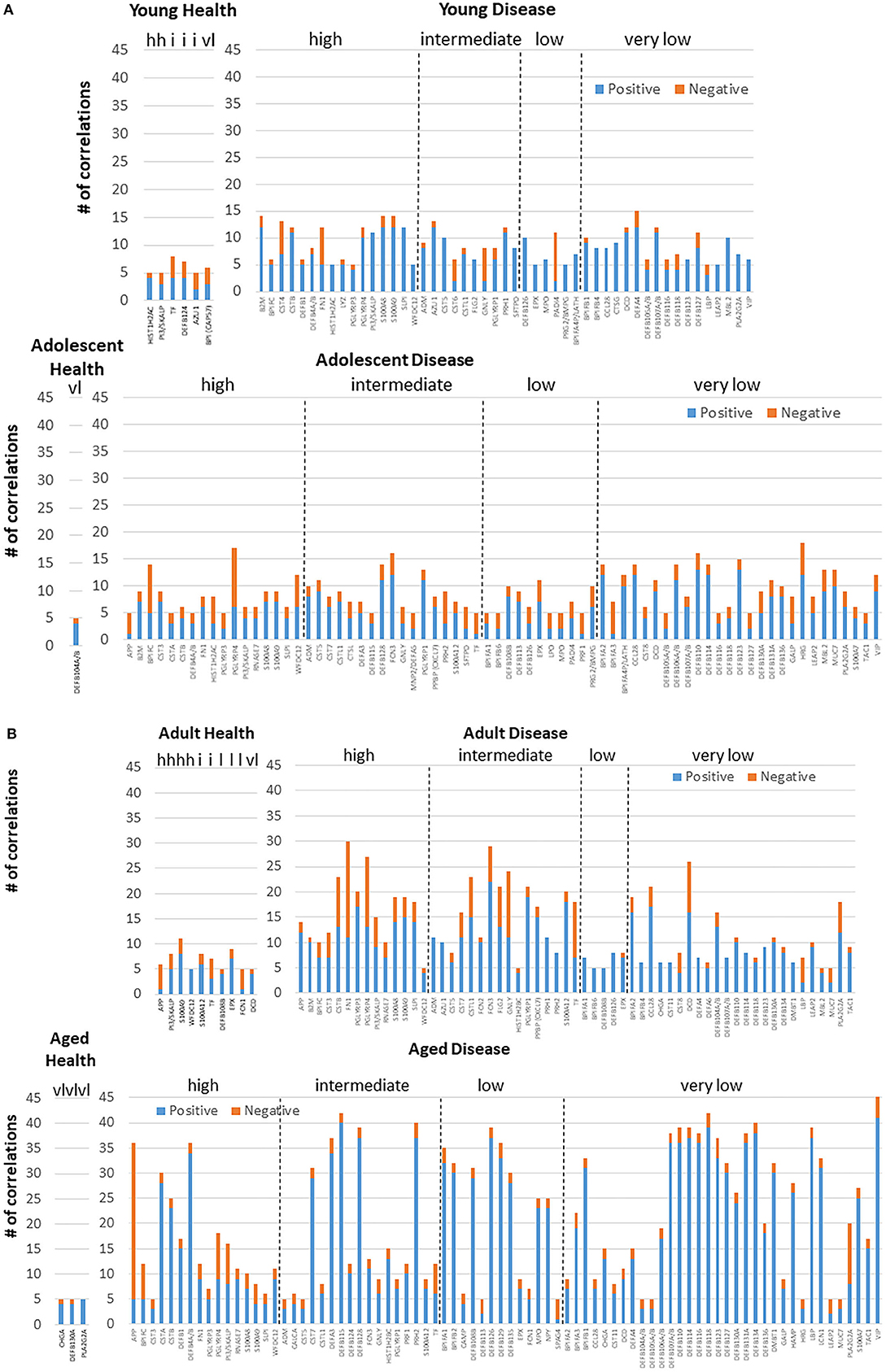
Figure 4. Correlations among the AMF gene in (A) young and adolescent health and disease and (B) adult and aged health and disease. The bars denote the number of either positive or negative significant correlations for each of the genes showing 5 or more significant correlations across the entirety of the 114 AMF group examined. Genes are organized into expression level categories based upon Figure 1 (h, high; i, intermediate; l, low; vl, very low).
Figure 5 provides a visualization of the relationship among the various AMFs related to both age and health/disease of the gingival tissues. The emphasis of the cluster analysis and heatmap is for 18 of the AMF genes that showed distinct expression differences across the age groups and related to the stage of health, disease, and resolution of the gingival tissues. This analysis identified 5 clusters with the primary grouping based upon stage of disease, with age as a secondary feature. Additionally, similarities in expression were noted between baseline and resolution specimens. The figure also provides a Principal Components Analysis using the same set of genes. This analytic approach also identified 5 groups of expression and also demonstrated disease as the primary feature controlling the AMF expression patterns. Finally, Figure 5 also summarizes these same linkages in an unrooted tree structure. Features show the profiles of AMF expression are quite similar in healthy and resolution samples, albeit the health samples from adult and aged animals were somewhat unique. Secondly, the disease samples in the young and adolescent animals were all in the same general root structure, while progressing disease (i.e., 1 and 3 month) for the adults and aged animals grouped together. Finally, the disease initiation AMF profiles (i.e., 2 weeks) in the adult and aged samples showed some unique features compared to all other disease specimens.
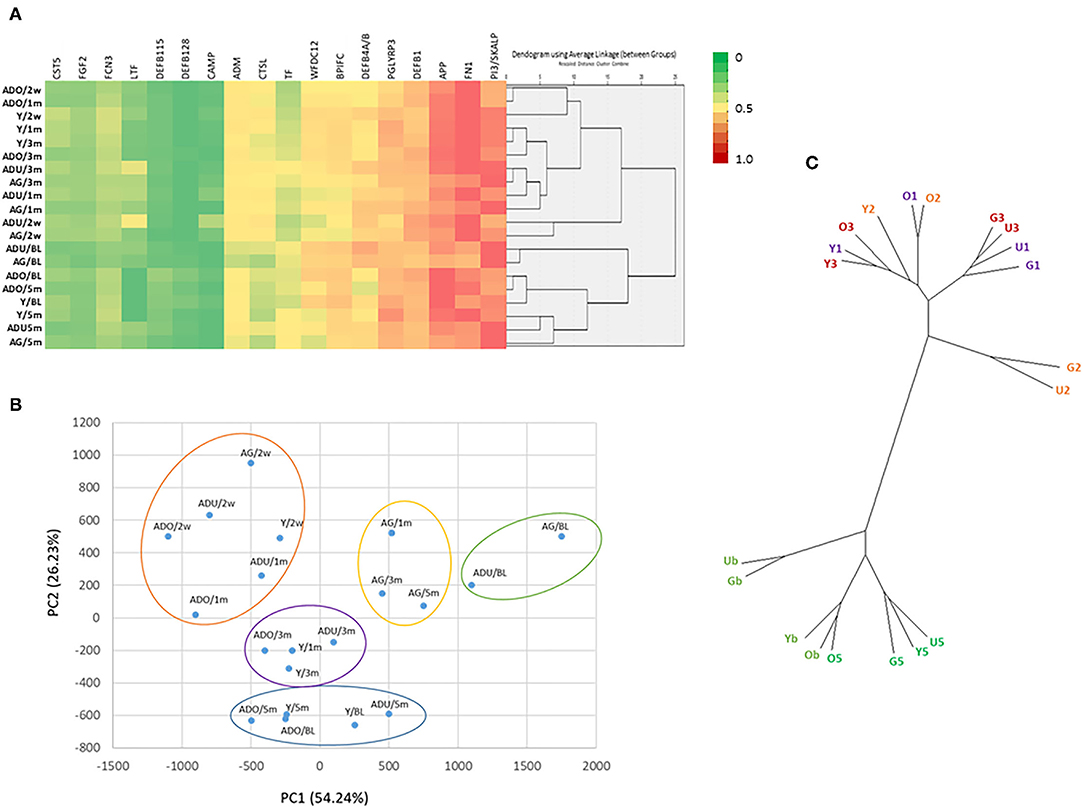
Figure 5. (A) Heatmap and clustering using 18 AMF genes that discriminated age and the disease process. (B) Principal Components Analysis of 18 AMF genes. Y, young; O, Adolescent; U, Adult; G, Aged samples; b, baseline; 2, initiation; 1 and 3, progression; 5, resolution of disease. Each point denotes the mean of the age group and time point. (C) Unrooted tree representation of grouping of the AMF gene expression. Labels are as in (B).
While oral bacteria clearly trigger the destructive inflammatory responses of periodontitis, substantial literature has identified an array of host factors that could directly account for the features of inflammation, soft tissue destruction, and alveolar bone resorption in this disease. As such, we asked the question regarding the relationship between the expression of AMF genes and targeted host genes associated with the local environmental tissue changes in the periodontium. Figure 6 presents the data identifying the frequency of significant correlations in AMF and the genes that could impact the environment and tissues. The striking feature was the much higher frequency of these relationships in the younger animals compared to the older adult/aged group in both health and disease. Also apparent was the large number of these correlations in the younger groups during disease.
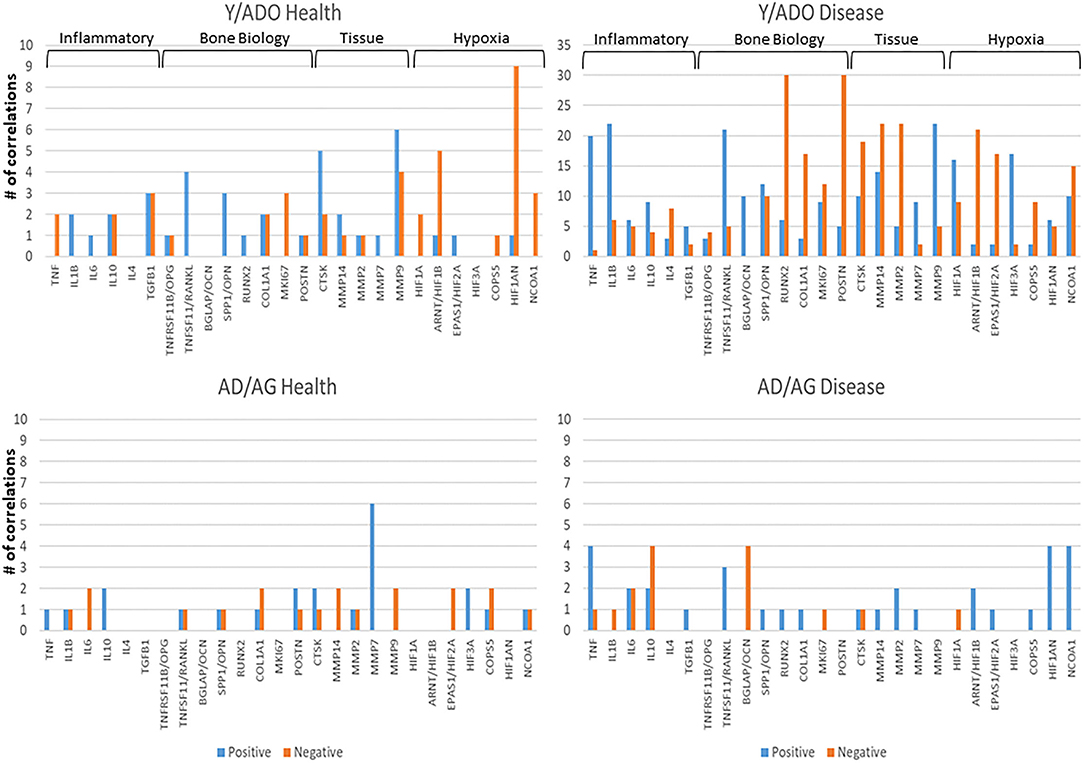
Figure 6. Correlations between AMF gene expression levels and various groups of genes related to the tissue microenvironment in younger (Y/ADO) and older (AD/AG) animal gingival tissues. Colors denote number of significant positive or negative correlations of each tissue gene with the array of AMF genes.
Oral Microbiome Components and Innate Antimicrobial Factor Gene Expression in Health and Disease
The oral microbiome in humans and non-human primates is quite complex, with many similarities in the distribution of phyla, genera, and species [44–46]. As it is clear that an array of host factors, including the AMFs interact with components of the microbiome, we examined details of these potential relationships. Figure 7A displays the distribution of the various primary genera of bacteria within the oral microbiome of samples from the younger animals. Substantial changes occur with disease initiation (0.5 months) that are generally retained during disease progression and remain quite distinct from the healthy microbiome even with clinical resolution of disease (5 months). A similar profile of genera is provided in Figure 7B for the older group of animals. While there were many similarities in the microbial distribution during different stages of disease, a striking change was the increase in Porphyromonas, Fusobacterium, and Fretibacterium with disease in the older animal samples.
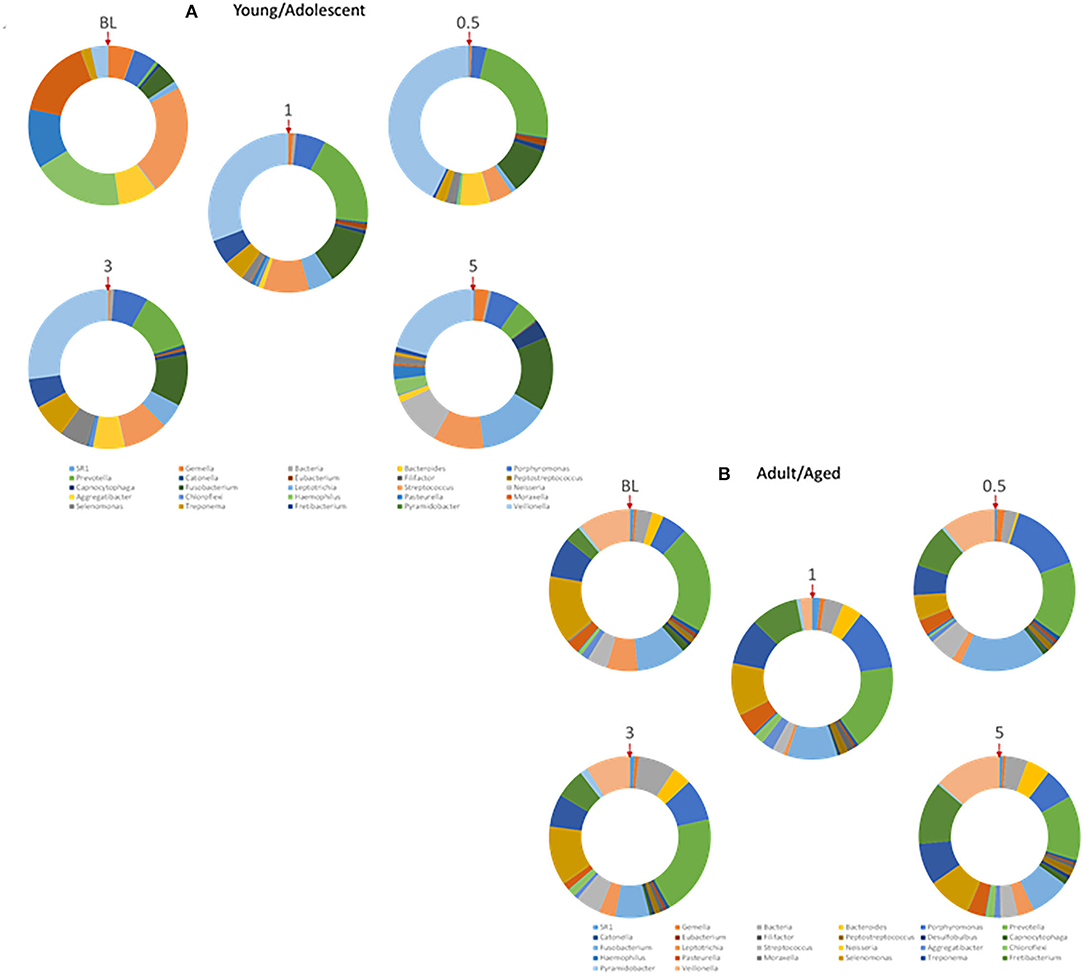
Figure 7. Relative abundance of various genera of bacteria within the microbiomes of (A) younger (Young/Adolescent) or (B) older (Adult/Aged) gingival tissues. Each ring represents one sampling time point. Red arrow denotes starting point for identification of individual genera.
Figure 8 demonstrates the overall relationship between the microbial burden (total bacterial signal readout) and the normalized signal for the microarray values for the entire set of AMFs. The results showed the microbial burden increased with disease in both age groups; however, the levels returned to near baseline in the older group of animals (AD/AG), while remaining increased by nearly 60% in the Y/ADO group. However, the pattern of general AMF gene expression was quite different in the 2 age groups. The levels at baseline were significantly greater in the AD/AG group, but decreased with disease initiation and progression. In the Y/ADO group, the AMF levels increased at the early stages of disease. Both age groups showed AMF gene levels approximating baseline values in the resolution samples.
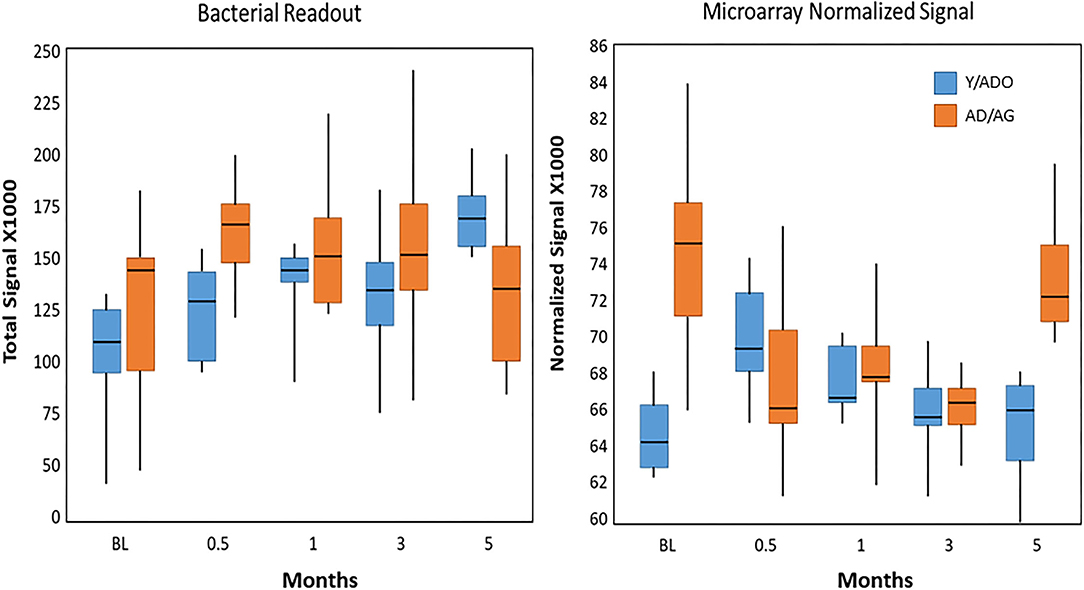
Figure 8. Box and whisker plot of total abundance of the bacterial signals in samples from younger and older animal groups at baseline, with disease, and in resolution samples. Similar plot tabulated the sum of the microarray normalized signals for all AMFs in each sample.
Figure 9 shows the relationship between the AMF gene expression levels and correlations with the array of OTUs in the microbiome samples. We included 49 OTUs that comprised readouts accounting for 77–86% in the younger group and 58 OTUs representing 74–82% in the older group. In healthy samples from the younger group (Figure 9A), we identified that CST5, DEFA3, DEFB128, DEFB131A, GALP, MBL2, TF, WFDC12, BPIFA4P, and PI3/SKALP showed elevated frequencies of significant correlations. The frequency of AMF-microbial relationships decreased substantially during disease with only FN1 represented. In the resolution samples, while fewer transcripts were significantly correlated, DCD, DEFB103A/B, DEFB129, S100A12, WFDC12, BPIFA3, and BPIFA4P all showed extensive correlations across the various OTUs. Figure 9B provides a comparison with the adult/aged group samples with 21 genes demonstrating frequent correlations (>10) in the healthy tissues samples. As noted in the younger samples, this was decreased to only PRG2 and LEAP2 in the disease samples. A contrast with healthy tissues was observed in the resolution samples from the adult/aged group, with a limited number of AMF genes showing any correlations with the microbiome components, and only CST9, DEFB126, DEFB127, and S100A7 demonstrating an elevated number of correlations.
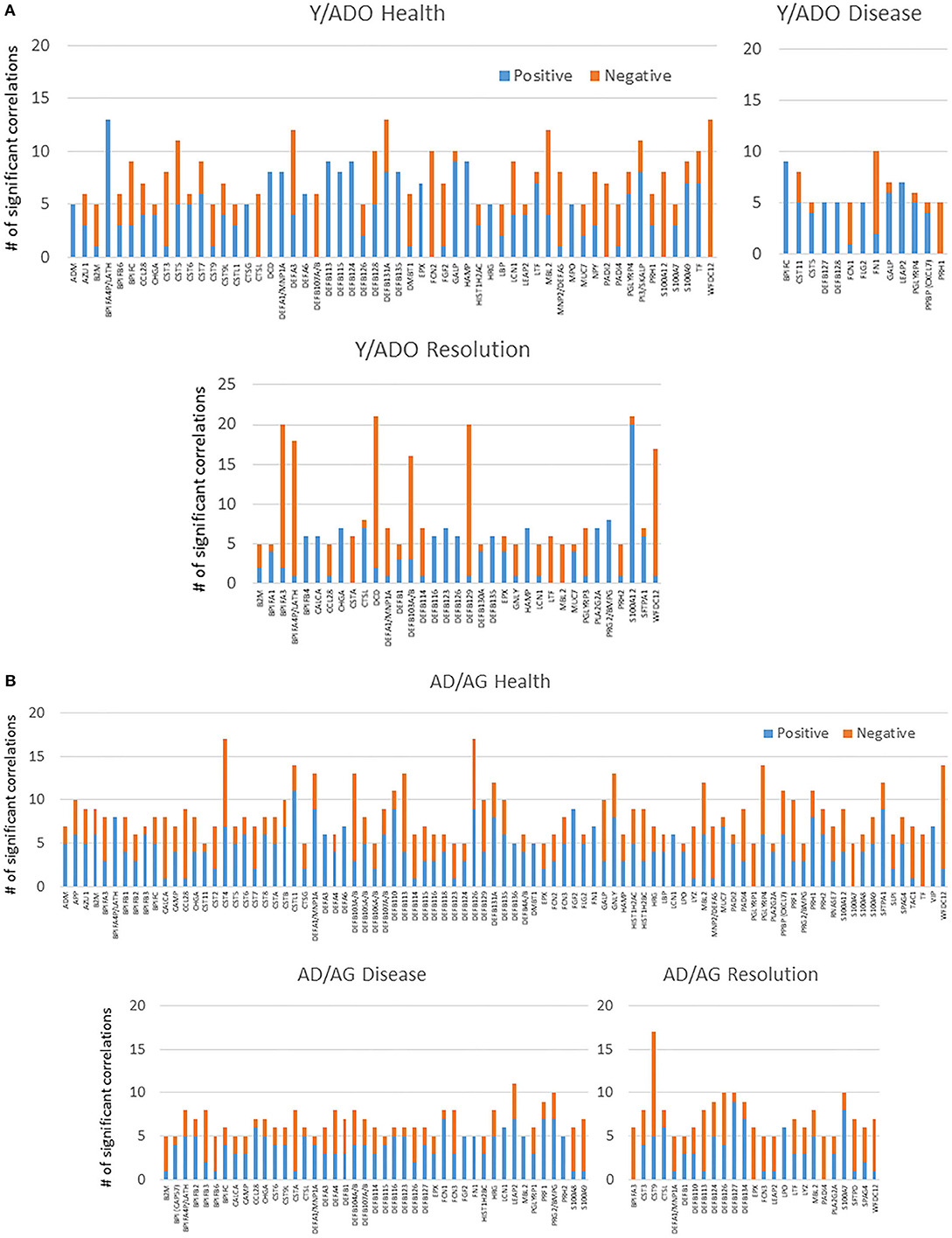
Figure 9. Significant positive or negative correlations between relative abundance of the 49 OTUs (A) in the younger group or 58 OTUs (B) in the older group included in the microbiome analysis and individual AMF genes. Bars denote number of OTUs correlated with the specific AMF gene. Only AMF genes with significant correlations with 5 or more of the microbial OTUs are included.
The data was also evaluated to identify the members of the oral microbiome that dominated in correlations with the array of AMF gene expression patterns in the juxtaposed gingival tissues. Figure 10A shows multiple OTUs that were highly correlated with the AMF in healthy young/adolescent samples that included Leptotrichia_unclassified, Prevotella_unclassified, Capnocytophaga_unclassified, Bacteroidetes_unclassified, P. gingivalis HMT619, SR1_[G-1] sp. HMT345, E. infirmum HMT105, Prevotella sp. HMT313, T. socranskii HMT769, P. intermedia HMT643, and Leptotrichia sp. HMT223. In disease, these correlations were much more limited with none of the bacteria demonstrating >15 correlations. In contrast, in the resolution samples, 8 OTUs showed an elevated frequency of correlations with the AMF gene expression levels and included Treponema_unclassified, Capnocytophaga_unclassified, T. denticola HMT584, SR1_[G-1] sp. HMT345, Fusobacterium sp. HMT203, Prevotella sp. HMT304, and T. socranskii HMT769.
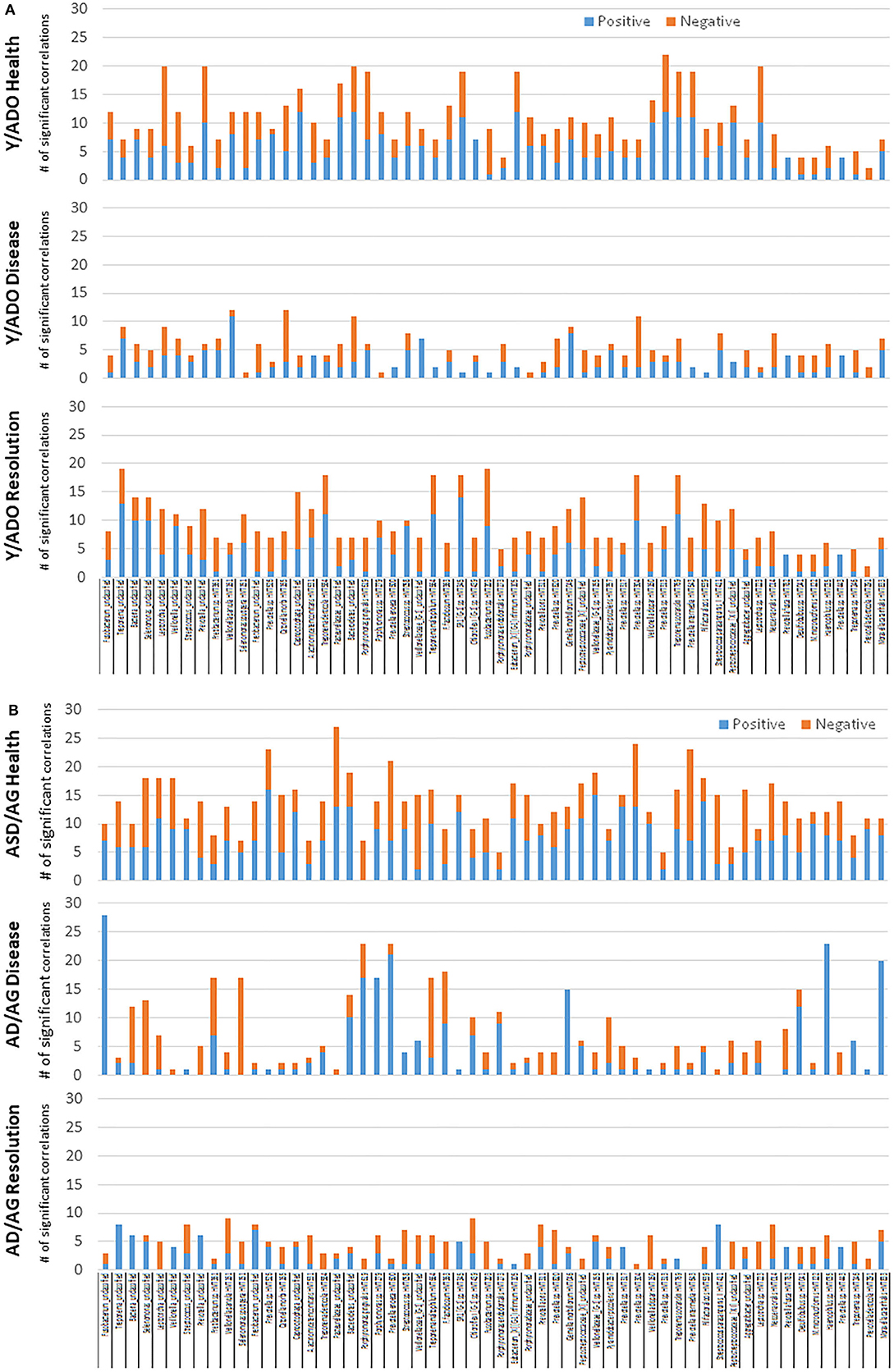
Figure 10. Significant positive or negative correlations between individual AMF genes and relative abundance of specific OTUs (A) in the younger group or (B) in the older group. Bars denote number of AMF correlated with the specific OTU.
Figure 10B summarizes the microbial components with numerous AMF correlations in the adult/aged group samples. In the healthy samples, 24 OTUs showed a large number of significant correlations to the AMF transcripts. A different subset of these were seen in disease, highlighted by Fusobacterium_unclassified, Fretibacterium sp. HMT361, S. sputigena HMT151, P. gingivalis HMT619, Porphyromonas sp. HMT279, P. enoeca HMT600, T. maltophilum HMT664, F. fastidiosum HMT363, G. morbillorum HMT046, Desulfobulbus sp. HMT041, Haemophilus sp. HMT035, and M. catarrhalis HMT833. Finally, in the resolution samples, this magnitude of correlations was generally absent in the adult/aged samples.
Supplementary Figure 2 presents a summary of the AMF genes that are most greatly affected by components of the microbiome. In this analysis, the bacterial families that predominated in the microbiome were stratified into high and low levels based upon median family level in the adult/aged samples. We then determined the AMF gene levels based upon the ratio of high/low levels of the microbial families. Most notable was that across the bacteria, the majority of the AMF were increased with lower relative abundance of the various bacterial families. In particular, expression of WFDC12, PBPP, CST5, BPIFC, DEFB103A, PI3/SKALP, SLPI, LTF, and DEF4A/B were most broadly impacted by showing decreased levels with higher levels of multiple families of bacteria in the microbiome. Additionally, Lactobacillales, Pasteurellales, Selenomonadales, and Veillonellales demonstrated the greatest frequency of lower expression with the higher relative abundance in the samples.
Microbial Complexes and Inflammatory Gene Expression in Aging
A pattern of microbiome members and specific groups of the AMF were noted within these individual correlation analyses. This pointed to a feature, in which there appeared complexes of the bacteria that related to a particular profile of the AMF, potentially related to their general or specific antimicrobial features. Figure 11A provides a profile of the characteristics of these complexes related to patterns of AMF expression. First of note was that two bacterial complexes in healthy younger samples primarily showed positive correlations with the AMF, while YH/AMF2 was skewed toward negative correlations with the AMF. The complexes were represented by unique individual microbiome features, with the members comprised of a mix of species that are classically considered more pathogenic and others identified as commensal. With disease, the members of the complexes changed with YD/AMF1 and YD/AMF2 oriented more toward pathogenic species and YD/AMF3 generally considered commensals. This difference was noted with the relative correlation distribution of the complexes to the AMF.
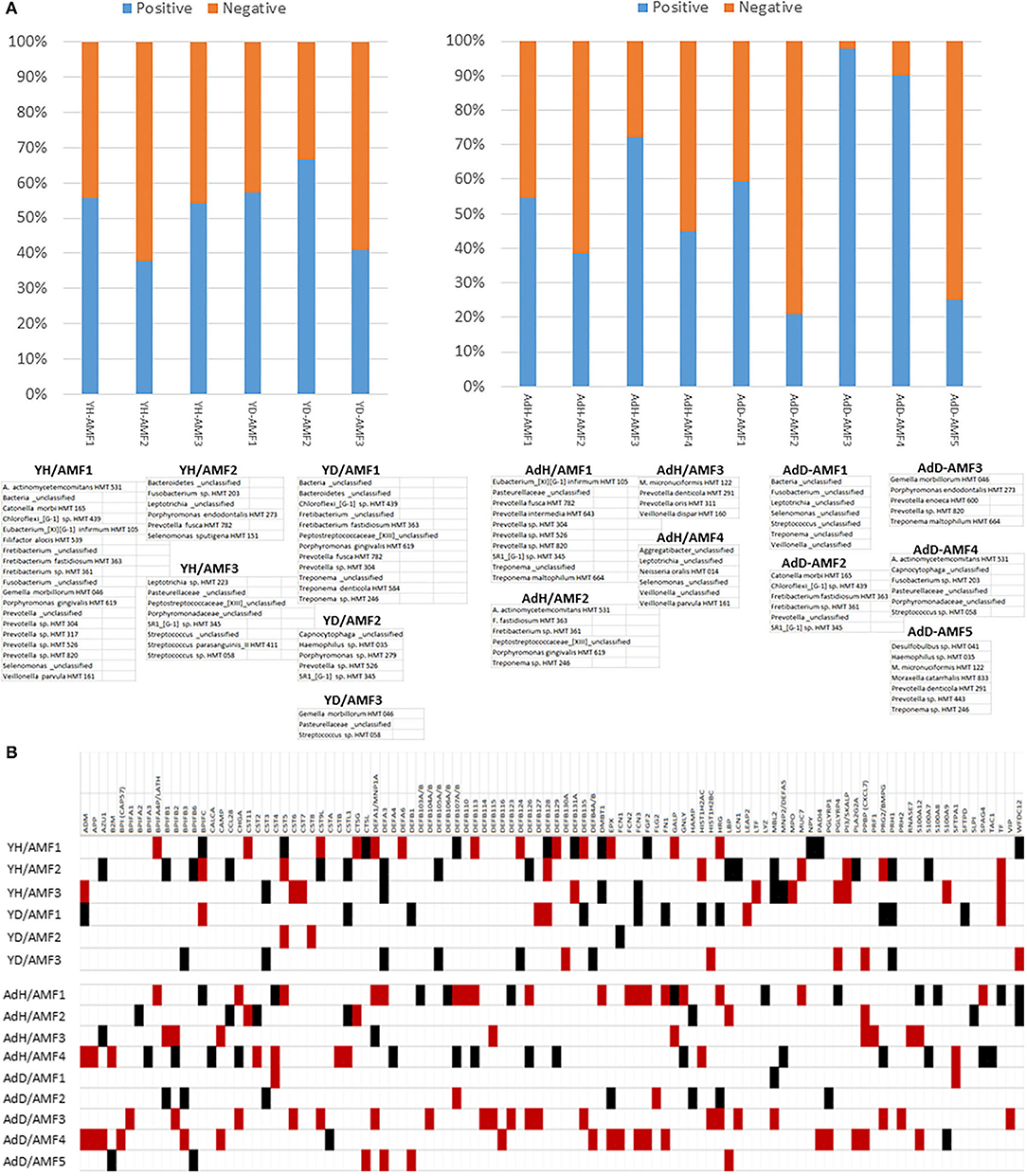
Figure 11. (A) Relative distribution of positive and negative correlations across the AMF genes related to specific bacterial complexes in young (Y) or older (Ad) animals samples. Complexes are identified in healthy samples (e.g., YH, AdH) or diseased samples (e.g., YD, AdD). (B) Correlations between microbiome complexes and individual AMF gene levels. The red box denotes significant positive correlations and the black denotes significant negative correlations with the array of bacteria in the identified complex.
In the adult/aged samples, AdH/AMF1 and AdH/AMF3 showed more dominant positive correlations with the AMF. Of interest was that AdH/AMF1 demonstrated a prevalence of what would be considered pathogenic species, while AdH/AMF3 was generally commensals. In contrast AdH/AMF2 and AdH/AMF4 showed dominant negative correlations with AdH/AMF2 showing mostly more pathogenic species. Substantial changes were observed in the complexes in the adult/aged disease samples. AdD/AMF2 and AdD/AMF5 showed a high prevalence of negative correlations with the AMF and AdD/AMF2 was highly represented by more pathogenic species. In contrast, AdD/AMF3 showed almost entirely significant positive correlations with the AMF, and was composed of species generally considered as commensals.
Figure 11B provides a tabulation of the individual AMF that displayed the predominance of correlations to the various bacterial complexes. First, 103 of the AMF showed significant correlations to one or more of the bacterial complexes. Secondly, specific complexes demonstrated enhanced numbers of these correlations including YH/AMF1, YH/AMF2, AdH/AMF1, AdH/AMF4, AdD/AMF3, and AdD/AMF4. Within the young healthy samples only 10–15% of the individual AMF actually overlapped between the two complexes and all were in an opposite correlation direction. Fewer specific correlations were noted in the young disease samples with unique AMF patterns for each complex and with only BPIFC, CST5, DEFA3, DEFB128, PRH1, and TF showing an elevated frequency of correlations.
The four complexes in the adult/aged samples also showed low overlap of AMF (1–8 AMF similar), while the disease samples exhibited even less overlap (1–3 AMF similar). The AMF genes that demonstrated the greatest prevalence of correlations in these older animal samples included BPIFB2, CHGA, CST4, DEFA1, DEFB107A, DEFB126, HRG, PPBP, and S100A12.
Discussion
Antimicrobial factors (AMFs) in the oral cavity range from the relatively large agglutinating mucins to ion scavenging molecules, to smaller membrane-perforating cationic peptides [2, 13, 47, 48]. These AMFs are important contributors to maintaining the balance between health and disease in this complex environment. These AMFs are part of the host innate immune response in this environment and are produced by epithelial cells, polymorphonuclear leukocytes, and salivary gland cells to contribute to protection of the oral cavity with overlapping and unique functions. Beyond the direct bacterial effects including affecting microbial viability, and functions of bacterial LPSs and peptidoglycans, many AMFs possess host cell regulatory functions including apoptosis, proinflammatory, and chemotactic activities. Various studies have identified alterations in AMFs in the oral cavity of humans with periodontitis [49–55], as well as demonstrating the capacity of various oral bacteria to trigger production of these biomolecules from various cells in culture. However, generally these studies have focused on one or a few of the innate immune components related to health and disease [9]. This study mapped the relative abundance of 114 AMF genes in non-human primate gingival tissues in health, disease and resolution, as well as the effect of age on this expression. Moreover, this expression was examined related to the presence of the major 50–60 bacterial OTUs in the oral microbiome, and the relationship to genes expressed in the tissues that indicate local environmental features in the gingiva.
Of the AMF characterized in the study, expression levels in healthy tissues demonstrated various AMFs expressed at high to very low levels. Comparing the expression across age showed a limited number of these genes differed, albeit, some unique features were displayed in both young and aged animals, e.g., CST6, CTSL, CAMP, DEFB126, CCL28. Additionally, the number of the AMF genes that differed significantly from adult levels were routinely greatest in samples from young animals, with an overlap of about 50% of the differentially expressed genes across the age groups. Extension of the determination of the AMF expression focused on changes related to disease initiation and progression, as well as in tissues with clinically resolved lesions. Again, nearly twice as many of the AMFs were altered in the young samples vs. the other age groups. While most of these differences were identified in disease samples, a subset of the AMF remained altered even in the resolution samples. Also, in the young and adolescent groups 60–70% of the genes were upregulated during disease, while nearly? were down-regulated in the adult and aged animals. This observation could be related to a more resilient oral microbiome that we described before in aging vs. young animals. As such, the young animals appeared to show more significant dysbiotic changes with the disease process. Nevertheless, there was extensive overlap in the targeted AMF genes that were affected in all age groups.
The detection of overlapping AMF expression features within the samples across age groups suggested the potential for distinctive correlation patterns of AMF. Exploring these correlations in healthy tissues showed a somewhat limited frequency of significant correlation across the array of AMFs. Young and adolescent animals displayed ~20% of the AMF genes with numerous correlations, while 30% of the genes showed high correlations in the adults and nearly 50% of the genes were highly correlated in the aged samples. This was somewhat unexpected, as both innate and adaptive immune responses are generally negatively associated with the aging process. However, it appears that many of the AMFs may be conserved during aging, and show an increased similarity in regulation of expression. Also, of note in this dataset was that previous reports indicated that the antimicrobial factor changes in saliva did not appear to cluster around similar functions for the biomolecules, but showed diverse properties including LPS-binding protein (LBP) altered coupled with cystatins, lipocalin 1, and perforin 1 [56, 57]. Here the array of altered AMFs could be assigned to multiple functional targets and may more broadly reflect changes in the microbiome, to which various host cells are responding. A striking feature of the AMF gene expression patterns was exposed when examining the clustering of the AMF expression in gingival tissues from the various age groups, and in health and disease. Our previous results have supported that the transition from health to disease was a major driving feature of altered gingival gene expression patterns for multiple pathways [27, 30, 37, 38, 40, 41, 58–62]. While some age differences were observed, the major changes appeared to be disease related. In a similar fashion with the AMF, cluster analysis clearly emphasized that the characteristics of the gingival tissues (i.e., health, disease, resolution) appeared to be the major controlling factor in the relationship of AMF expression. This suggested that evolution of the AMF producing pathways was similar with aging processes. These biomolecules are a downstream expression of numerous molecular pathways of innate immunity. Thus, some common features of cell receptors, cellular signaling, or transcription and translation processes may be under similar control in aging, more than previously considered. Identification of these regulatory components should provide improved insight into alterations in host resistance to infection, neoplasia, and even autoimmunity that could occur with aging. As noted since the AMFs represent an array of biomolecules with various cellular origins within the gingival tissues, an understanding of the temporal cellular differences during disease initiation and resolution would add additional knowledge to the features of the specific cellular responses that occur and result in alter AMF levels. Current technology using single cell RNASeq studies provides a method to explore the cellular composition in complex tissues and uses specific mRNA patterns to describe the tissue composition [63]. Related to this, various approaches have been used to explore existing large gene expression datasets to also document the character of the cellular makeup of tissues. The approach uses cell-specific convolution and a computation method of population-specific expression analysis (PSEA) to describe the cell composition [64–66]. Specifically, Momen-Heravi et al. [67] has recently reported on the use of this technique to characterize the cellular composition of human gingival tissues in health and disease. The AMF data was extracted from a larger microarray study that is amenable to this type of bioinformatics exploration and should add insights into the changes in cellular composition that underpin these AMF gene alterations in disease. An additional focus can also be generated from the breadth of findings in this study such that hypothesis generation can be developed based upon the more detailed findings of individual AMF and their potential molecular role in homeostasis and/or disease. An example of this concept is in the findings with ficolin (FCN1) that was generally significantly positively related to the disease process. The ficolins are soluble pattern recognition molecules that contribute to complement activation via the lectin pathway [68]. Ficolins in serum are complexed with MBL-associated serine proteases and bind to carbohydrates present on the surface of microbes as components of innate immune responses. Related to periodontitis, studies have shown that LPS-induced inflammation can induce a significant ficolin response [69]. While the ficolin response/function has very limited findings in periodontitis studies have shown that the mirolysin of T. forsythia degrade these AMF and alter complement aspects of innate immunity [70]. Additionally, through its interaction with complement, one could propose an important role due to the clear data implicating complement activation and disease associated with P. gingivals [71–73].
Clear data demonstrate that exposure of gingival epithelial cells to oral bacteria that have been associated with periodontitis results in the production of an array of innate immune molecules, including β-defensins and CAMP (LL-37) [2]. Furthermore, the importance of the array of AMFs have been supported by a number of genetic disorders associated with decreased AMF production in the oral cavity, and increased susceptibility to bacterial infections [2, 74, 75]. Additional examples include mucin-7 and lactoferrin being significantly altered in patients with periodontitis [76]. Thus, we evaluated the relationship of this array of AMFs with expression of genes that would reflect a changing periodontal environment that would contribute to tissue alterations consistent with a disease process. The results showed few correlations between the AMF levels and the expression of the various tissue and environmental genes in the older group of animals. However, numerous significant correlations were observed in the younger group that were most noted in disease samples, and displayed generally positive correlations with inflammatory genes and significant negative correlations with the bone biology, tissue destructive/remodeling, and hypoxia genes. The numerous correlations were unexpected, but may reflect a more regulated response capacity of the gingival tissues in the younger group, which is consistent with less destructive disease [42].
It is also clear that the characteristics of the oral microbiome in the subgingival sulcus that is juxtaposed to the gingival tissues shows interactions that are critical for homeostasis. However, alterations toward a dysbiotic microbiome occur with disease and are reflected by substantial gene expression changes in the tissues. We have previously described the microbiome at sites of periodontal lesions in the non-human primates and how these differ in healthy and resolved lesion samples [33, 77–79]. The microbiome data presented distinct differences in samples from younger and older animals, as well as changes in the microbial distribution with disease in the younger group of animals. Interestingly, at the level of the 26 dominant microbial genera, the changes were more subtle in samples from the older animals with Fretibacterium the most distinctive of the changes. This relatively recently identified genera within the phylum Synergistetes has been identified in multiple studies as a potential microbial diagnostic marker of disease [80–82]. Moreover, it was identified as a member of a group of microbes that remained elevated in persistent aggressive disease patients post-therapy [83, 84]. Thus, the findings in this non-human primate model are reflective of the disease microbiome in humans.
The data was then interrogated to estimate relationships among specific microbial components of the microbiome and the patterns of AMF expression in the tissues. In both groups of animals, frequent significant correlations across a wide range of the AMF were observed in the healthy samples, with 20–25% greater frequency of positive correlations. In contrast, during disease ~40–50% fewer correlations were noted, although in both age groups the positive relationships dominated. Finally at resolution, while the positive and negative correlation frequencies were similar in both the age groups, the striking feature was the lower number of AMF genes that contributed to these correlations. There is a general lack of comparable literature with which to compare these findings; however, one interpretation is that with tissue homeostasis these AMF represent a family of genes that are responsive to the oral microbiome and may help regulate homeostasis. During disease this relationship is substantially decreased potentially reflecting the dysbiosis and host dysregulation, or being driven by members comprising the new dysbiotic microbiome. An additional question was also addressed regarding the biologic features of healthy tissues and gingival tissues that represent resolved lesions. The data showed a clear difference in AMF expression in healthy compared to disease resolved tissues in both age groups, with the greatest loss of relationships in the resolution samples from the older animals.
A previous study focused on an array of antimicrobial peptides (AMPs) that showed a wide variation between individuals and were modulated by disease [50]. The results suggested salivary AMPs present in periodontal health and disease showed some correlations of specific antimicrobial peptides and clusters of these AMPs with bacterial species and clusters of species in the oral microbiome. Our results drilled down to individual OTU/species level and explored these relationships with the broader AMF gene levels in health, disease and resolution. In health, the portfolio of individual bacteria showed 54 and 73% with elevated correlations in the younger and older groups, respectively. The microbial representation of the correlations with AMF was decreased greatly during disease, with only 4 OTUs in the younger group and only 30% of the bacteria in the older group samples, although these were comprised of Fusobacterium, Bacteroidetes, Porphyromonas, Prevotella, Treponema, Fretibacterium, and Chloroflexi genera. Similarly, a substantial difference in the correlation patterns between the bacteria and AMF was seen in the younger vs. the older animal resolution samples. The samples from the younger animals appeared to re-establish the AMF-bacterial correlations in resolution samples, while these were totally lost in the older animal specimens.
Finally, as periodontitis reflects changes in the geospatial and functional aspects of microbial complexes in the subgingival microbiome [85–88] we explored the presence of microbial consortia and estimated their combined role in regulating the AMF patterns with age and in health and disease. We had previously reported on these types of microbial consortia that were significantly associated with gingival tissue responses of apoptosis, autophagy and hypoxia genes [77, 78]. Additionally, we identified other microbial complexes in the microbiome of young and older animals that related to the expression of an array of immunoglobulin genes in the gingival tissues (in press). Thus, we identified multiple microbial complexes in the young and older animal samples that significantly correlated with a group of the AMF in health and disease. The features of these complexes identified clearly different complexes in the young and older animal samples in both health and disease. Individual complexes in the young animals showed a skewing toward either positive or negative correlations with the AMF in health or disease. In contrast, there was a distinct predilection for individual complexes to exhibit positive or negative correlation with the array of AMF. The complexes also demonstrated a prevalence of more pathogenic species, mostly commensals, or a mixture of these species within the same complex. Finally, there was generally limited overlap in the specific AMF that correlated with these complexes within health or disease samples in either age grouping. These results supported, as was observed with the relationship to apoptosis, autophagy, hypoxia and immunoglobulin genes, somewhat distinct patterns of microbial complex relationships to these host AMF responses, which underpins certain host-microbe interactions that may be critical for the maintenance of gingival homeostasis or can reflect a basis for the transition to a disease process. Further work remains to better understand the detailed temporal nature of these interactions and potential utilization for these parameters for estimating risk of disease progression or more limited response to standard therapy.
Data Availability Statement
The datasets presented in this study can be found in online repositories. The names of the repository/repositories and accession number(s) can be found below: https://www.ncbi.nlm.nih.gov/, GSE180588; https://www.ncbi.nlm.nih.gov/, PRJNA516659.
Ethics Statement
The protocol was approved by the Institutional Animal Care and Use Committees (IACUC) of the University of Puerto Rico and University of Kentucky and a ligature disease model was utilized.
Author Contributions
JE and OG developed and implemented the experimental design, obtained samples, interpreted the results, and constructed the report. SK provided technical assistance in preparation and analysis of the samples, provided content, and reviewed the manuscript. LN provided the advanced statistical analyses on the data, provided content, and reviewed the manuscript All authors contributed to the article and approved the submitted version.
Funding
This work was supported by National Institute of Health grant P20GM103538.
Conflict of Interest
The authors declare that the research was conducted in the absence of any commercial or financial relationships that could be construed as a potential conflict of interest.
Publisher's Note
All claims expressed in this article are solely those of the authors and do not necessarily represent those of their affiliated organizations, or those of the publisher, the editors and the reviewers. Any product that may be evaluated in this article, or claim that may be made by its manufacturer, is not guaranteed or endorsed by the publisher.
Acknowledgments
We express our gratitude to the Caribbean Primate Research Center (CPRC), supported by grant P40RR03640, specifically Drs. Janis Gonzalez Martinez, Luis Orraca, and Armando Burgos, and the Center for Oral Health Research in the College of Dentistry at the University of Kentucky. We also thank the Microarray Core of University Kentucky for their invaluable technical assistance, and Dr. A. Stromberg for initial normalization of the microarray data. We also thank the Genomic Core Laboratory of University Kentucky for their invaluable technical and data management assistance.
Supplementary Material
The Supplementary Material for this article can be found online at: https://www.frontiersin.org/articles/10.3389/froh.2022.817249/full#supplementary-material
Supplementary Figure 1. (A–D) Altered transcript levels expressed as fold-difference from baseline healthy levels to initiation (0.5 months), progression (1 and 3 months) and resolution (5 months) of periodontal lesions in each age group.
Supplementary Figure 2. Differences in AMF expression levels based upon categorization of bacterial families in the microbiome into high and low levels. Each point denotes the value of an AMF gene level based upon expression in high vs. low family microbiome samples.
References
1. Bechinger B, Gorr SU. Antimicrobial peptides: mechanisms of action and resistance. J Dent Res. (2017) 96:254–60. doi: 10.1177/0022034516679973
2. Gorr SU. Antimicrobial peptides of the oral cavity. Periodontol 2000. (2009) 51:152–80. doi: 10.1111/j.1600-0757.2009.00310.x
3. Wertz PW, de Szalay S. Innate antimicrobial defense of skin and oral mucosa. Antibiotics. (2020) 9:159. doi: 10.3390/antibiotics9040159
4. Levy O, Canny G, Serhan CN, Colgan SP. Expression of BPI (bactericidal/permeability-increasing protein) in human mucosal epithelia. Biochem Soc Transact. (2003) 31:795–800. doi: 10.1042/bst0310795
5. Bosshardt DD, Lang NP. The junctional epithelium: from health to disease. J Dent Res. (2005) 84:9–20. doi: 10.1177/154405910508400102
6. Joly S, Organ CC, Johnson GK, McCray PB Jr, Guthmiller JM. Correlation between beta-defensin expression and induction profiles in gingival keratinocytes. Mol Immunol. (2005) 42:1073–84. doi: 10.1016/j.molimm.2004.11.001
7. Hosokawa I, Hosokawa Y, Komatsuzawa H, Goncalves RB, Karimbux N, Napimoga MH, et al. Innate immune peptide LL-37 displays distinct expression pattern from beta-defensins in inflamed gingival tissue. Clin Exp Immunol. (2006) 146:218–25. doi: 10.1111/j.1365-2249.2006.03200.x
8. Gursoy M, Gursoy UK, Liukkonen A, Kauko T, Penkkala S, Kononen E. Salivary antimicrobial defensins in pregnancy. J Clin Periodontol. (2016) 43:807–15. doi: 10.1111/jcpe.12581
9. Lynge Pedersen AM, Belstrom D. The role of natural salivary defences in maintaining a healthy oral microbiota. J Dent. (2019) 80(Suppl. 1):S3–12. doi: 10.1016/j.jdent.2018.08.010
10. Ghosh SK, Feng Z, Fujioka H, Lux R, McCormick TS, Weinberg A. Conceptual perspectives: bacterial antimicrobial peptide induction as a novel strategy for symbiosis with the human host. Front Microbiol. (2018) 9:302. doi: 10.3389/fmicb.2018.00302
11. Canny G, Cario E, Lennartsson A, Gullberg U, Brennan C, Levy O, et al. Functional and biochemical characterization of epithelial bactericidal/permeability-increasing protein. Am J Physiol Gastrointest Liver Physiol. (2006) 290:G557–67. doi: 10.1152/ajpgi.00347.2005
12. Canny G, Levy O. Bactericidal/permeability-increasing protein (BPI) and BPI homologs at mucosal sites. Trends Immunol. (2008) 29:541–7. doi: 10.1016/j.it.2008.07.012
13. Gorr SU. Antimicrobial peptides in periodontal innate defense. Front Oral Biol. (2012) 15:84–98. doi: 10.1159/000329673
14. Rosa L, Lepanto MS, Cutone A, Ianiro G, Pernarella S, Sangermano R, et al. Lactoferrin and oral pathologies: a therapeutic treatment. Biochem Cell Biol. (2021) 99:81–90. doi: 10.1139/bcb-2020-0052
15. Lu J, Francis J, Doster RS, Haley KP, Craft KM, Moore RE, et al. Lactoferrin: a critical mediator of both host immune response and antimicrobial activity in response to streptococcal infections. ACS Infect Dis. (2020) 6:1615–23. doi: 10.1021/acsinfecdis.0c00050
16. Fine DH. Lactoferrin: a roadmap to the borderland between caries and periodontal disease. J Dent Res. (2015) 94:768–76. doi: 10.1177/0022034515577413
17. Krzysciak W, Jurczak A, Piatkowski J, Koscielniak D, Gregorczyk-Maga I, Kolodziej I, et al. Effect of histatin-5 and lysozyme on the ability of Streptococcus mutans to form biofilms in in vitro conditions. Postepy Hig Med Dosw. (2015) 69:1056–66.
18. Sukhithasri V, Nisha N, Biswas L, Anil Kumar V, Biswas R. Innate immune recognition of microbial cell wall components and microbial strategies to evade such recognitions. Microbiol Res. (2013) 168:396–406. doi: 10.1016/j.micres.2013.02.005
19. Peyyala R, Ebersole JL. Multispecies biofilms and host responses: “discriminating the trees from the forest”. Cytokine. (2013) 61:15–25. doi: 10.1016/j.cyto.2012.10.006
20. Gorr SU, Abdolhosseini M. Antimicrobial peptides and periodontal disease. J Clin Periodontol. (2011) 38(Suppl. 11):126–41. doi: 10.1111/j.1600-051X.2010.01664.x
21. Altman H, Steinberg D, Porat Y, Mor A, Fridman D, Friedman M, et al. In vitro assessment of antimicrobial peptides as potential agents against several oral bacteria. J Antimicrob Chemother. (2006) 58:198–201. doi: 10.1093/jac/dkl181
22. Bedran TB, Mayer MP, Spolidorio DP, Grenier D. Synergistic anti-inflammatory activity of the antimicrobial peptides human beta-defensin-3 (hBD-3) and cathelicidin (LL-37) in a three-dimensional co-culture model of gingival epithelial cells and fibroblasts. PLoS ONE. (2014) 9:e106766. doi: 10.1371/journal.pone.0106766
23. Joly S, Maze C, McCray PB Jr, Guthmiller JM. Human beta-defensins 2 and 3 demonstrate strain-selective activity against oral microorganisms. J Clin Microbiol. (2004) 42:1024–9. doi: 10.1128/JCM.42.3.1024-1029.2004
24. Ouhara K, Komatsuzawa H, Yamada S, Shiba H, Fujiwara T, Ohara M, et al. Susceptibilities of periodontopathogenic and cariogenic bacteria to antibacterial peptides, {beta}-defensins and LL37, produced by human epithelial cells. J Antimicrob Chemother. (2005) 55:888–96. doi: 10.1093/jac/dki103
25. Ji S, Hyun J, Park E, Lee BL, Kim KK, Choi Y. Susceptibility of various oral bacteria to antimicrobial peptides and to phagocytosis by neutrophils. J Periodontal Res. (2007) 42:410–9. doi: 10.1111/j.1600-0765.2006.00962.x
26. Genco CA, Maloy WL, Kari UP, Motley M. Antimicrobial activity of magainin analogues against anaerobic oral pathogens. Int J Antimicrob Agents. (2003) 21:75–8. doi: 10.1016/S0924-8579(02)00275-3
27. Gonzalez OA, Novak MJ, Kirakodu S, Stromberg AJ, Shen S, Orraca L, et al. Effects of aging on apoptosis gene expression in oral mucosal tissues. Apoptosis. (2013) 18:249–59. doi: 10.1007/s10495-013-0806-x
28. Gonzalez OA, Stromberg AJ, Huggins PM, Gonzalez-Martinez J, Novak MJ, Ebersole JL. Apoptotic genes are differentially expressed in aged gingival tissue. J Dent Res. (2011) 90:880–6. doi: 10.1177/0022034511403744
29. Ebersole JL, Steffen MJ, Reynolds MA, Branch-Mays GL, Dawson DR, Novak KF, et al. Differential gender effects of a reduced-calorie diet on systemic inflammatory and immune parameters in nonhuman primates. J Periodontal Res. (2008) 43:500–7. doi: 10.1111/j.1600-0765.2008.01051.x
30. Ebersole JL, Kirakodu S, Novak MJ, Stromberg AJ, Shen S, Orraca L, et al. Cytokine gene expression profiles during initiation, progression and resolution of periodontitis. J Clin Periodontol. (2014) 41:853–61. doi: 10.1111/jcpe.12286
31. Ebersole JL, Steffen MJ, Gonzalez-Martinez J, Novak MJ. Effects of age and oral disease on systemic inflammatory and immune parameters in nonhuman primates. Clin Vaccine Immunol. (2008) 15:1067–75. doi: 10.1128/CVI.00258-07
32. Ebersole JL, Nguyen L, Gonzalez OA. Gingival tissue antibody gene utilization in aging and periodontitis. J Periodontal Res. (in press).
33. Kirakodu S, Chen J, Gonzalez Martinez J, Gonzalez OA, Ebersole J. Microbiome profiles of ligature-induced periodontitis in nonhuman primates across the lifespan. Infect Immun. (2019) 87:e00067–19. doi: 10.1128/IAI.00067-19
34. Kozich JJ, Westcott SL, Baxter NT, Highlander SK, Schloss PD. Development of a dual-index sequencing strategy and curation pipeline for analyzing amplicon sequence data on the MiSeq Illumina sequencing platform. Appl Environ Microbiol. (2013) 79:5112–20. doi: 10.1128/AEM.01043-13
35. Schloss PD, Westcott SL, Ryabin T, Hall JR, Hartmann M, Hollister EB, et al. Introducing mothur: open-source, platform-independent, community-supported software for describing and comparing microbial communities. Appl Environ Microbiol. (2009) 75:7537–41. doi: 10.1128/AEM.01541-09
36. Edgar RC, Haas BJ, Clemente JC, Quince C, Knight R. UCHIME improves sensitivity and speed of chimera detection. Bioinformatics. (2011) 27:2194–200. doi: 10.1093/bioinformatics/btr381
37. Gonzalez OA, Novak MJ, Kirakodu S, Stromberg A, Nagarajan R, Huang CB, et al. Differential gene expression profiles reflecting macrophage polarization in aging and periodontitis gingival tissues. Immunol Investig. (2015) 44:643–64. doi: 10.3109/08820139.2015.1070269
38. Gonzalez OA, Novak MJ, Kirakodu S, Orraca L, Chen KC, Stromberg A, et al. Comparative analysis of gingival tissue antigen presentation pathways in ageing and periodontitis. J Clin Periodontol. (2014) 41:327–39. doi: 10.1111/jcpe.12212
39. Meka A, Bakthavatchalu V, Sathishkumar S, Lopez MC, Verma RK, Wallet SM, et al. Porphyromonas gingivalis infection-induced tissue and bone transcriptional profiles. Mol Oral Microbiol. (2010) 25:61–74. doi: 10.1111/j.2041-1014.2009.00555.x
40. Ebersole JL, Kirakodu S, Novak MJ, Orraca L, Stormberg AJ, Gonzalez-Martinez J, et al. Comparative analysis of expression of microbial sensing molecules in mucosal tissues with periodontal disease. Immunobiology. (2019) 224:196–206. doi: 10.1016/j.imbio.2018.11.007
41. Ebersole JL, Novak MJ, Orraca L, Martinez-Gonzalez J, Kirakodu S, Chen KC, et al. Hypoxia-inducible transcription factors, HIF1A and HIF2A, increase in aging mucosal tissues. Immunology. (2018) 154:452–64. doi: 10.1111/imm.12894
42. Gonzalez OA, Nagarajan R, Novak MJ, Orraca L, Gonzalez-Martinez JA, Kirakodu SS, et al. Immune system transcriptome in gingival tissues of young nonhuman primates. J Periodontal Res. (2016) 51:152–63. doi: 10.1111/jre.12293
43. Ferrin J, Kirakodu S, Jensen D, Al-Attar A, Peyyala R, Novak MJ, et al. Gene expression analysis of neuropeptides in oral mucosa during periodontal disease in non-human primates. J Periodontol. (2018) 89:858–66. doi: 10.1002/JPER.17-0521
44. Colombo APV, Paster BJ, Grimaldi G, Lourenco TGB, Teva A, Campos-Neto A, et al. Clinical and microbiological parameters of naturally occurring periodontitis in the non-human primate Macaca mulatta. J Oral Microbiol. (2017) 9:1403843. doi: 10.1080/20002297.2017.1403843
45. Ocon S, Murphy C, Dang AT, Sankaran-Walters S, Li CS, Tarara R, et al. Transcription profiling reveals potential mechanisms of dysbiosis in the oral microbiome of rhesus macaques with chronic untreated SIV infection. PLoS ONE. (2013) 8:e80863. doi: 10.1371/journal.pone.0080863
46. Velusamy SK, Sampathkumar V, Ramasubbu N, Paster BJ, Fine DH. Aggregatibacter actinomycetemcomitans colonization and persistence in a primate model. Proc Natl Acad Sci USA. (2019) 116:22307–13. doi: 10.1073/pnas.1905238116
47. Dommisch H, Jepsen S. Diverse functions of defensins and other antimicrobial peptides in periodontal tissues. Periodontol 2000. (2015) 69:96–110. doi: 10.1111/prd.12093
48. Niu JY, Yin IX, Mei ML, Wu WKK, Li QL, Chu CH. The multifaceted roles of antimicrobial peptides in oral diseases. Mol Oral Microbiol. (2021) 36:159–71. doi: 10.1111/omi.12333
49. Jourdain ML, Pierrard L, Kanagaratnam L, Velard F, Sergheraert J, Lefevre B, et al. Antimicrobial peptide gene expression in periodontitis patients: a pilot study. J Clin Periodontol. (2018) 45:524–37. doi: 10.1111/jcpe.12879
50. Grant M, Kilsgard O, Akerman S, Klinge B, Demmer RT, Malmstrom J, et al. The human salivary antimicrobial peptide profile according to the oral microbiota in health, periodontitis and smoking. J Innate Immunity. (2019) 11:432–44. doi: 10.1159/000494146
51. Khodaii Z, Mehrabani M, Rafieian N, Najafi-Parizi GA, Mirzaei A, Akbarzadeh R. Altered levels of salivary biochemical markers in periodontitis. Am J Dentistry. (2019) 32:183–6.
52. Guncu GN, Yilmaz D, Kononen E, Gursoy UK. Salivary antimicrobial peptides in early detection of periodontitis. Front Cell Infect Microbiol. (2015) 5:99. doi: 10.3389/fcimb.2015.00099
53. Yilmaz D, Guncu GN, Kononen E, Baris E, Caglayan F, Gursoy UK. Overexpressions of hBD-2, hBD-3, and hCAP18/LL-37 in gingiva of diabetics with periodontitis. Immunobiology. (2015) 220:1219–26. doi: 10.1016/j.imbio.2015.06.013
54. Wang P, Duan D, Zhou X, Li X, Yang J, Deng M, et al. Relationship between expression of human gingival beta-defensins and levels of periodontopathogens in subgingival plaque. J Periodontal Res. (2015) 50:113–22. doi: 10.1111/jre.12187
55. Davidovich NV, Solovieva NV, Galieva AS, Lepeshkin SY, Bashilova EN, Pisareva SN, et al. Role of antimicrobial peptides system in inflammatory periodontal diseases non-specific oral cavity protection. Klin Lab Diagn. (2021) 66:422–7. doi: 10.51620/0869-2084-2021-66-7-422-427
56. Choi S, Baik JE, Jeon JH, Cho K, Seo DG, Kum KY, et al. Identification of Porphyromonas gingivalis lipopolysaccharide-binding proteins in human saliva. Mol Immunol. (2011) 48:2207–13. doi: 10.1016/j.molimm.2011.06.434
57. Quintana M, Palicki O, Lucchi G, Ducoroy P, Chambon C, Salles C, et al. Inter-individual variability of protein patterns in saliva of healthy adults. J Proteomics. (2009) 72:822–30. doi: 10.1016/j.jprot.2009.05.004
58. Ebersole JL, Kirakodu SS, Orraca L, Gonzalez Martinez J, Gonzalez OA. Gingival transcriptomics of follicular T cell footprints in progressing periodontitis. Clin Exp Immunol. (2021) 204:373–95. doi: 10.1111/cei.13584
59. Ebersole JL, Kirakodu S, Novak MJ, Dawson D, Stromberg AJ, Orraca L, et al. Gingival tissue autophagy pathway gene expression profiles in periodontitis and aging. J Periodontal Res. (2021) 56:34–45. doi: 10.1111/jre.12789
60. Ebersole JL, Orraca L, Novak MJ, Kirakodu S, Gonzalez-Martinez J, Gonzalez OA. Comparative analysis of gene expression patterns for oral epithelium-related functions with aging. Adv Exp Med Biol. (2019) 1197:143–63. doi: 10.1007/978-3-030-28524-1_11
61. Pandruvada SN, Gonzalez OA, Kirakodu S, Gudhimella S, Stromberg AJ, Ebersole JL, et al. Bone biology-related gingival transcriptome in ageing and periodontitis in non-human primates. J Clin Periodontol. (2016) 43:408–17. doi: 10.1111/jcpe.12528
62. Ebersole JL, Kirakodu SS, Novak MJ, Orraca L, Martinez JG, Cunningham LL, et al. Transcriptome analysis of B cell immune functions in periodontitis: mucosal tissue responses to the oral microbiome in aging. Front Immunol. (2016) 7:272. doi: 10.3389/fimmu.2016.00272
63. Williams DW, Greenwell-Wild T, Brenchley L, Dutzan N, Overmiller A, Sawaya AP, et al. Human oral mucosa cell atlas reveals a stromal-neutrophil axis regulating tissue immunity. Cell. (2021) 184:4090–104.e15. doi: 10.1016/j.cell.2021.05.013
64. Zhang C, Zheng JH, Lin ZH, Lv HY, Ye ZM, Chen YP, et al. Profiles of immune cell infiltration and immune-related genes in the tumor microenvironment of osteosarcoma. Aging. (2020) 12:3486–501. doi: 10.18632/aging.102824
65. Wang X, Park J, Susztak K, Zhang NR, Li M. Bulk tissue cell type deconvolution with multi-subject single-cell expression reference. Nat Commun. (2019) 10:380. doi: 10.1038/s41467-018-08023-x
66. Danziger SA, Gibbs DL, Shmulevich I, McConnell M, Trotter MWB, Schmitz F, et al. ADAPTS: automated deconvolution augmentation of profiles for tissue specific cells. PLoS ONE. (2019) 14:e0224693. doi: 10.1371/journal.pone.0224693
67. Momen-Heravi F, Friedman RA, Albeshri S, Sawle A, Kebschull M, Kuhn A, et al. Cell type-specific decomposition of gingival tissue transcriptomes. J Dent Res. (2021) 100:549–56. doi: 10.1177/0022034520979614
68. Matsushita M. Ficolins in complement activation. Mol Immunol. (2013) 55:22–6. doi: 10.1016/j.molimm.2012.08.017
69. Jarlhelt I, Genster N, Kirketerp-Moller N, Skjoedt MO, Garred P. The ficolin response to LPS challenge in mice. Mol Immunol. (2019) 108:121–7. doi: 10.1016/j.molimm.2019.02.013
70. Jusko M, Potempa J, Mizgalska D, Bielecka E, Ksiazek M, Riesbeck K, et al. A Metalloproteinase Mirolysin of Tannerella forsythia inhibits all pathways of the complement system. J Immunol. (2015) 195:2231–40. doi: 10.4049/jimmunol.1402892
71. Olsen I, Lambris JD, Hajishengallis G. Porphyromonas gingivalis disturbs host-commensal homeostasis by changing complement function. J Oral Microbiol. (2017) 9:1340085. doi: 10.1080/20002297.2017.1340085
72. Slaney JM, Curtis MA. Mechanisms of evasion of complement by Porphyromonas gingivalis. Front Biosci. (2008) 13:188–96. doi: 10.2741/2669
73. Hajishengallis G. Complement and periodontitis. Biochem Pharmacol. (2010) 80:1992–2001. doi: 10.1016/j.bcp.2010.06.017
74. Hart TC, Atkinson JC. Mendelian forms of periodontitis. Periodontol 2000. (2007) 45:95–112. doi: 10.1111/j.1600-0757.2007.00233.x
75. Putsep K, Carlsson G, Boman HG, Andersson M. Deficiency of antibacterial peptides in patients with morbus Kostmann: an observation study. Lancet. (2002) 360:1144–9. doi: 10.1016/S0140-6736(02)11201-3
76. Groenink J, Walgreen-Weterings E, Nazmi K, Bolscher JG, Veerman EC, van Winkelhoff AJ, et al. Salivary lactoferrin and low-Mr mucin MG2 in Actinobacillus actinomycetemcomitans-associated periodontitis. J Clin Periodontol. (1999) 26:269–75. doi: 10.1034/j.1600-051X.1999.260501.x
77. Ebersole JL, Kirakodu SS, Gonzalez OA. Oral microbiome interactions with gingival gene expression patterns for apoptosis, autophagy and hypoxia pathways in progressing periodontitis. Immunology. (2021) 162:405–17. doi: 10.1111/imm.13292
78. Ebersole JL, Kirakodu SS, Neumann E, Orraca L, Gonzalez Martinez J, Gonzalez OA. Oral microbiome and gingival tissue apoptosis and autophagy transcriptomics. Front Immunol. (2020) 11:585414. doi: 10.3389/fimmu.2020.585414
79. Ebersole J, Kirakodu S, Chen J, Nagarajan R, Gonzalez OA. Oral microbiome and gingival transcriptome profiles of ligature-induced periodontitis. J Dent Res. (2020) 99:746–57. doi: 10.1177/0022034520906138
80. Na HS, Kim SY, Han H, Kim HJ, Lee JY, Lee JH, et al. Identification of potential oral microbial biomarkers for the diagnosis of periodontitis. J Clin Med. (2020) 9:1549. doi: 10.3390/jcm9051549
81. Lundmark A, Hu YOO, Huss M, Johannsen G, Andersson AF, Yucel-Lindberg T. Identification of salivary microbiota and its association with host inflammatory mediators in periodontitis. Front Cell Infect Microbiol. (2019) 9:216. doi: 10.3389/fcimb.2019.00216
82. Deng ZL, Szafranski SP, Jarek M, Bhuju S, Wagner-Dobler I. Dysbiosis in chronic periodontitis: key microbial players and interactions with the human host. Sci Rep. (2017) 7:3703. doi: 10.1038/s41598-017-03804-8
83. Nibali L, Sousa V, Davrandi M, Liu LS, Spratt D, Donos N. Patterns of subgingival microbiota in different periodontal phenotypes. J Dent. (2022) 117:103912. doi: 10.1016/j.jdent.2021.103912
84. Nibali L, Sousa V, Davrandi M, Spratt D, Alyahya Q, Dopico J, et al. Differences in the periodontal microbiome of successfully treated and persistent aggressive periodontitis. J Clin Periodontol. (2020) 47:980–90. doi: 10.1111/jcpe.13330
85. Borisy GG, Valm AM. Spatial scale in analysis of the dental plaque microbiome. Periodontol 2000. (2021) 86:97–112. doi: 10.1111/prd.12364
86. Proctor DM, Shelef KM, Gonzalez A, Davis CL, Dethlefsen L, Burns AR, et al. Microbial biogeography and ecology of the mouth and implications for periodontal diseases. Periodontol 2000. (2020) 82:26–41. doi: 10.1111/prd.12268
87. Mark Welch JL, Ramirez-Puebla ST, Borisy GG. Oral microbiome geography: micron-scale habitat and niche. Cell Host Microbe. (2020) 28:160–8. doi: 10.1016/j.chom.2020.07.009
Keywords: non-human primate, aging, microbiome, periodontitis, antimicrobial factors
Citation: Ebersole JL, Kirakodu S, Nguyen L and Gonzalez OA (2022) Gingival Transcriptome of Innate Antimicrobial Factors and the Oral Microbiome With Aging and Periodontitis. Front. Oral. Health 3:817249. doi: 10.3389/froh.2022.817249
Received: 17 November 2021; Accepted: 04 February 2022;
Published: 07 March 2022.
Edited by:
Gill Diamond, University of Louisville, United StatesReviewed by:
Tomoki Maekawa, Niigata University, JapanRenata Oliveira Mattos-Graner, Universidade Estadual de Campinas, Brazil
Camille Zenobia, AbbVie, United States
Copyright © 2022 Ebersole, Kirakodu, Nguyen and Gonzalez. This is an open-access article distributed under the terms of the Creative Commons Attribution License (CC BY). The use, distribution or reproduction in other forums is permitted, provided the original author(s) and the copyright owner(s) are credited and that the original publication in this journal is cited, in accordance with accepted academic practice. No use, distribution or reproduction is permitted which does not comply with these terms.
*Correspondence: Jeffrey L. Ebersole, amVmZnJleS5lYmVyc29sZSYjeDAwMDQwO3VubHYuZWR1