- 1Department of Industrial Digitalization, School of Technology and Innovations, University of Vaasa, Vaasa, Finland
- 2Research Program in Systems Oncology, Faculty of Medicine, University of Helsinki, Helsinki, Finland
- 3Department of Oral Medicine and Diagnostic Science, College of Dentistry, King Saud University, Riyadh, Saudi Arabia
- 4Department of Pathology, University of Helsinki, Helsinki, Finland
- 5Department of Otorhinolaryngology – Head and Neck Surgery, University of Helsinki and Helsinki University Hospital, Helsinki, Finland
- 6Division of Ear, Nose and Throat Diseases, Department of Clinical Sciences, Intervention and Technology, Karolinska Institutet and Karolinska University Hospital, Stockholm, Sweden
- 7Institute of Biomedicine, Pathology, University of Turku, Turku, Finland
- 8Faculty of Dentistry, University of Misurata, Misurata, Libya
The application of deep machine learning, a subfield of artificial intelligence, has become a growing area of interest in predictive medicine in recent years. The deep machine learning approach has been used to analyze imaging and radiomics and to develop models that have the potential to assist the clinicians to make an informed and guided decision that can assist to improve patient outcomes. Improved prognostication of oral squamous cell carcinoma (OSCC) will greatly benefit the clinical management of oral cancer patients. This review examines the recent development in the field of deep learning for OSCC prognostication. The search was carried out using five different databases—PubMed, Scopus, OvidMedline, Web of Science, and Institute of Electrical and Electronic Engineers (IEEE). The search was carried time from inception until 15 May 2021. There were 34 studies that have used deep machine learning for the prognostication of OSCC. The majority of these studies used a convolutional neural network (CNN). This review showed that a range of novel imaging modalities such as computed tomography (or enhanced computed tomography) images and spectra data have shown significant applicability to improve OSCC outcomes. The average specificity, sensitivity, area under receiving operating characteristics curve [AUC]), and accuracy for studies that used spectra data were 0.97, 0.99, 0.96, and 96.6%, respectively. Conversely, the corresponding average values for these parameters for computed tomography images were 0.84, 0.81, 0.967, and 81.8%, respectively. Ethical concerns such as privacy and confidentiality, data and model bias, peer disagreement, responsibility gap, patient-clinician relationship, and patient autonomy have limited the widespread adoption of these models in daily clinical practices. The accumulated evidence indicates that deep machine learning models have great potential in the prognostication of OSCC. This approach offers a more generic model that requires less data engineering with improved accuracy.
Introduction
A total of 377, 713 new cases of oral cavity and lip cancer and 177, 757 deaths related to oral cancer were reported in the year 2020 [1]. Considering the location of oral squamous cell carcinoma (OSCC) and the corresponding aggressive behavior of this disease, it has been reported to have significant effects on the patients' post-treatment quality of life [2]. Recently, clear advances in diagnostic techniques and treatment modalities have been achieved [3]. However, OSCC is still characterized by a low average survival rate [4]. Accurate prognostication remains of utmost importance to improve survival rates [5].
Traditionally, the treatment of cancer depends mainly on tumor staging. However, staging discrepancies have contributed to inaccurate prognostication in OSCC patients [2]. Despite the increasing number of prognostic markers, the overall prognosis of the disease has not changed significantly [6]. This may be due to the challenges in the integration of these markers in the current staging system [7, 8]. Additionally, individualized treatment of patient on a case-by-case basis is lacking. Therefore, improved diagnostic and prognostic accuracy could significantly assist the clinicians in making informed decisions regarding appropriate treatment for better survival [9].
To this end, machine learning techniques (shallow learning) have been reported to offer improved prognostication of OSCC [9, 10]. Of note, the use of machine learning has been reported to provide a more accurate prognostication than the traditional statistical analyses [9, 11–14]. Machine learning techniques have been able to show promising results because they are able to discern the complex relationships between the variables contained in the dataset [9]. Considering the touted feasibility and benefits of the machine learning techniques in cancer prognostication, its application in this field has attracted significant attention in recent years. This is because it is poised to assist the clinicians in making informed decisions thereby improving and promoting better management of patient health. Interestingly, the advancements in technology have led to the modification of shallow machine learning to deep machine learning. This deep learning approach has also been touted to improve cancer management.
In this study, we aim to systematically review the published studies that have utilized deep machine learning techniques for OSCC prognostication. This is necessary to show the state-of-the-art performance of deep learning analytic methods for prognostication of the disease. Thus, the focused question was: “Does deep machine learning technique play a role in improving prognostication accuracy and guiding clinicians in making an informed decision.”
Methods
Search Protocol
Detailed literature searches were performed using databases such as OvidMedline, PubMed, Scopus, Web of Science, and Institute of Electrical and Electronics Engineers (IEEE) from their inception until 15 May 2021. RefWorks software was used to properly manage the potentially relevant articles and remove any duplicate articles. Additionally, the reference lists of the included articles were manually searched to ensure that all the relevant articles have been included.
Search Strategy
The search approach was developed by combining search keywords: [((“oral cancer” OR “oral squamous cell carcinoma” OR “pre-cancerous” OR “oral potentially malignant”) AND (“deep learning”))].
Eligibility Criteria
Inclusion Criteria
The Population, Exposure, Comparator, Outcomes, and Study design (PECOS) framework was used to define the research question(s) of this review. Thus, the P in the PECOS framework represents population (patients) with OSCC; E depicts that deep machine learning has been applied for prognostication, C ensures that the parameter of interest have OSCC patients with or without this parameter, O indicates that there is a clear outcome to be determined by the deep learning techniques, and S indicates that observational studies and/or clinical trials were also considered. Thus, original, observational, and clinical trials that utilized deep learning techniques for prognostication in OSCC were included. Additionally, only studies published in the English language were considered.
Exclusion Criteria
Studies in languages other than English and those that did not utilize deep learning for prognostication in oral cancer were excluded. Case reports, editorials, surveys, book chapter, comparative papers, symposium articles, conference articles, short communications, abstracts, opinions, perspectives, invited reviews, and letters to the editor were also excluded.
Study Selection
The study selection process was carried out in two distinct phases. Firstly, the titles and abstracts of potentially relevant articles were examined after the removal of duplicates. This phase was conducted by two independent reviewers (R.A., & O.Y.). A data extraction sheet was used for this process to ensure proper documentation with a Cohen's Kappa coefficient (κ = 0.91) for inter-observer reliability. This stage was followed by consensus meeting and discussion to resolve possible discrepancies before the study could be included in this review. For the second phase, these two independent reviewers extracted relevant information relating to the study characteristics of each of these potentially relevant articles.
Parameters Extracted
The independent reviewers (R.A., & O.Y.) extracted the following information from each of the included studies; author (s) name, year of publication, country, oral cancer description, study objectives, sample population, type of data used, performance of the deep learning model, and conclusions. This information is presented in Table 1.
Quality Assessment of the Included Studies
The Preferred Reporting Items for Systematic Review and Meta-Analysis (PRISMA) methodology was used to document the searching and screening processes in this study (Figure 1) [46, 47]. The quality of the included studies were accessed using the Prediction model Risk of Bias Assessment Tool (PROBAST) as shown in Table 2. Additionally, PROBAST was used to evaluate and assess the risk of bias (ROB) of the potential studies to be included in this review. As the study examines deep machine learning method, the predictors' parameter from the PROBAST tool was modified to include the robustness of the methodology used in the included studies.
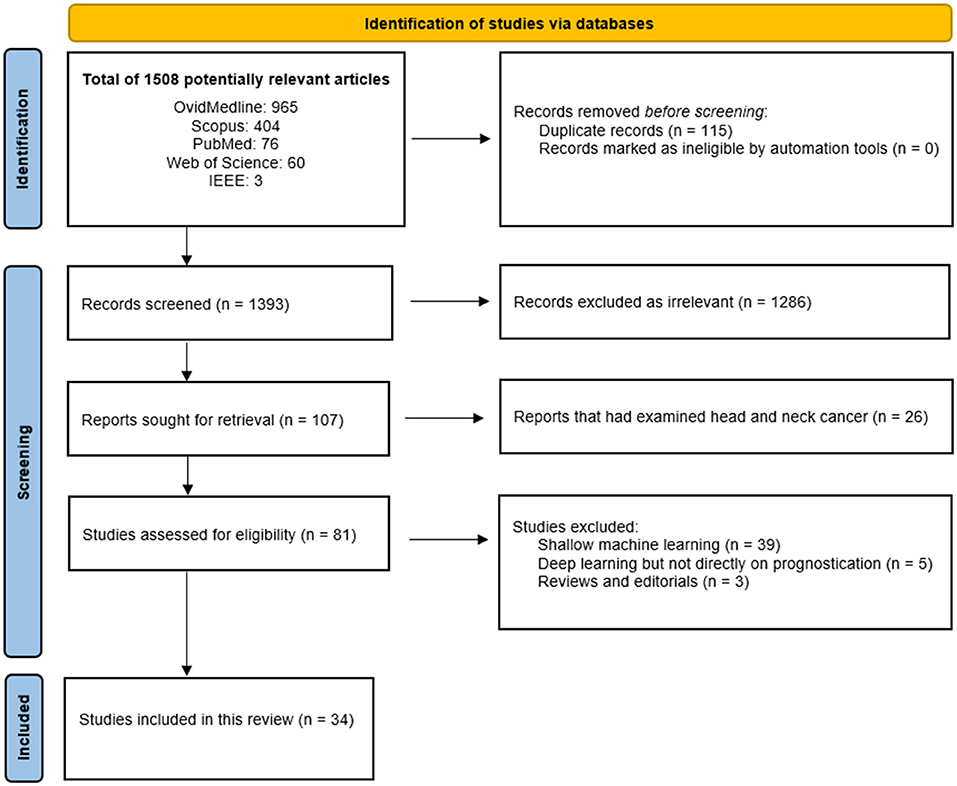
Figure 1. The PRISMA flow chart for the included studies [46].
Results
Results of the Database Search
A total of 34 studies met the eligibility criteria and were included in this review [2, 15–45, 48]. The details of the study selection process have been described using the PRISMA flowchart (Figure 1) [46]. The included studies that utilized deep learning for prognostication of OSCC are summarized in Table 1. These studies concluded that the deep learning techniques could offer assistance to the clinicians in making informed decisions regarding choosing treatment options to avoid under-treatment or unnecessary treatments and thus achieving better management of the disease.
Studies Characteristics of Relevant Studies
The majority of these studies used a convolutional neural network (CNN) [2, 15–22, 24–26, 28, 31–36, 38–41, 43–45, 48, 49]. Several data types such as gene expression data [15, 45], spectra data [20, 21, 29, 34, 37, 44, 48], and other image data types—anatomical [16], intraoral [17], histology [18, 27], auto-fluorescence [19, 22], cytology-image [23], neoplastic [40], clinical [28, 36, 38], oral lesions [42], computed tomography images [24–26, 33, 35, 41, 49], clinicopathologic [2], saliva metabolites [31], histopathological [30, 32, 43], and pathological [39] images have been used in the included studies.
Considering the reported performance metrics (specificity, sensitivity, and accuracy) and the accumulated evidence presented in the included studies, deep machine learning models have great potential in the prognostication of OSCC. This approach offers a more generic model that requires less data engineering with improved accuracy.
A single study reported the performance of deep learning with four different performance metrics (sensitivity, specificity, accuracy, and area under receiving operating characteristics curve [AUC]) [16]. Similarly, a total of 11 studies reported the combination of the trio of sensitivity, specificity, and accuracy as the performance metrics for the deep machine learning method [15, 19–21, 24, 25, 30, 35, 37, 38, 42]. Both specificity and sensitivity were used to depict the performance of the model [17, 20, 22, 27, 48]. Additionally, specificity and accuracy were also used to demonstrate the performance of the deep learning model for prognostication in OSCC [23]. Other studies used either accuracy, C-index (concordance index), F1-score, or Dice similarity coefficient (Dsc) mean value as the performance metrics for reporting the potential benefits of the deep learning model [2, 18, 18, 26, 28, 29, 31–33, 39–41, 44, 45, 49].
Most of these studies used either spectra data or computed tomography images [20, 21, 24–26, 29, 33–35, 37, 41, 44, 48, 49]. The average specificity, sensitivity, area under receiving operating characteristics curve [AUC]), and accuracy for studies that used spectra data were 0.97, 0.99, 0.96, and 96.6%, respectively. Conversely, the corresponding average values for these parameters for computed tomography images were 0.84, 0.81, 0.967, and 81.8%, respectively.
The deep machine learning method has been reported to show promising results in the prediction and detection of OSCC [2, 15, 16, 18, 19, 22–24, 27–30, 34, 36, 39, 44] and lymph node metastasis [25, 33, 41]. Furthermore, the deep machine learning model have been reported to show significant prognostication of grading of the disease [32, 43] and survival prediction of oral cancer patients [2, 35]. Additional, the ability of deep machine learning to differentiate between precancerous (potentially malignant) lesions and OSCC [15, 17, 23, 26, 38, 39, 42], as well as between the disease and, for example, periodontitis disease [31] has been highlighted in different studies. Also, deep machine learning models have shown touted benefits that differentiate between oral tongue squamous cell carcinoma (OTSCC) and non-tumorous tissue [20, 21, 26, 37, 48].
Quality Assessment of the Studies Included in the Review
According to the PROBAST assessment, most (91.2%) of the included studies showed an overall low risk of bias and also exhibited low concern regarding applicability (Table 2).
Discussion
In this systematic review, the utilization of deep machine learning for prognostication in oral squamous cell carcinoma was examined. The deep learning methodology had been used to analyze various types of medical data such as clinicopathologic, histopathologic, gene expression, image, Raman spectroscopy, saliva metabolites, and computed tomography for better prognostication in OSCC. This review showed that a range of novel imaging modalities such as computed tomography (or enhanced computed tomography) images and spectra data have shown significant applicability to improve OSCC outcomes. Hence, deep machine learning methodology combined with medical imaging data can offer better and improved prognostication of OSCC. This can significantly assist the clinical management of patients with the disease [50].
The performance of the deep learning technique was mostly reported with either the combination of sensitivity, specificity, and accuracy or using a single performance metric. Based on the reported accuracy of the deep machine learning techniques in the included studies, it is evident that the deep machine learning technique can play a significant role toward the improved prognostication of oral cancer and guide clinicians in making informed decisions. The approach of using deep learning for prognostication can provide low-cost screening [19, 36], smartphone-based solution [17, 23], deep learning-based automatic prognostication [18, 27, 32], and early detection and prediction of outcomes [15, 17, 23, 24, 37–39, 42].
The utilization of deep machine learning for prognostication include the distinction between potentially malignant disease and OSCC [15, 17, 26, 39], differentiation between the oral tongue squamous cell carcinoma from non-tumorous tissue [20, 21, 34], prediction and detection of oral squamous cell carcinoma [16, 18, 22–24, 51], diagnosis of lymph node metastasis [25, 33], differentiation between OSCC and diseases such as periodontitis [31], ability to offer multi-class grading of OSCC [32], and prediction of survival in OSCC patients [2].
The afore-mentioned diagnostic ability and prognostication can greatly benefit the clinical management of OSCC patients [50]. For instance, deep learning can assist the pathologists in the effective multi-class grading, thereby, assisting in the timely and effective treatment protocol for the patients [32]. This can reduce operational workload and the possibility of burnout for the pathologists and enhance the proper management of the disease through timely grading [32]. Similarly, the deep learning model is capable of stratifying the patients into high-risk patients where they could be assigned to a more aggressive regimen or low-risk where more conservative treatment may be enough. This informed decision could assist in the overall survival of these patients by reducing the possibility of side effects such as hormonal disorder, trismus, or dental disease [52, 53].
The availability of medical data in different formats (multi-omics data—genomic, expression, proteomic, transcriptomic, and clinicopathologic data) through various databases such as the cancer genome atlas (TCGA), gene expression omnibus (GEO) has emerged as a great challenge to the traditional statistical methods of cancer prognostication [50]. Additionally, with the increase in computational power, advancement in technology (neural network model architecture), availability of medical dataset, the widely used shallow machine learning techniques have been modified to produce a deep machine learning technique, also known as the deep neural network (DNN) [54]. Interestingly, shallow machine learning has been reported to show promising results in various prognostication tasks such as prediction of locoregional recurrences [9, 10], survival [55], occult nodal metastasis [56], and performed better than other methods such as nomograms [57].
Despite these promising results by the shallow machine learning techniques, the deep machine learning techniques have been reported to perform equally or outperform the shallow machine learning method [50, 58, 59] as it is more flexible, requires less feature engineering, and consist of complex layers and multiple neurons in each layer [50, 60, 61] (Figure 2). This gives deep machine learning a better predictive power [50]. An example of the deep neural network commonly used in cancer prognostication is the convolutional neural network (CNN) which is usually used for medical image data [50] (Figure 2). In CNN, the convolution and max-pooling layer are responsible for feature extraction of the input data [62] (Figure 2). While the convolution layers facilitate feature extraction from the image data, the pooling layers ensure that overfitting is minimized. The results from the convolution and pooling layers are passed to the fully connected layer for classification into labels (output) [62]. Apart from the CNN, the recurrent neural network (RNN) is another type of deep neural network which is suitable for text and sequence data [50].
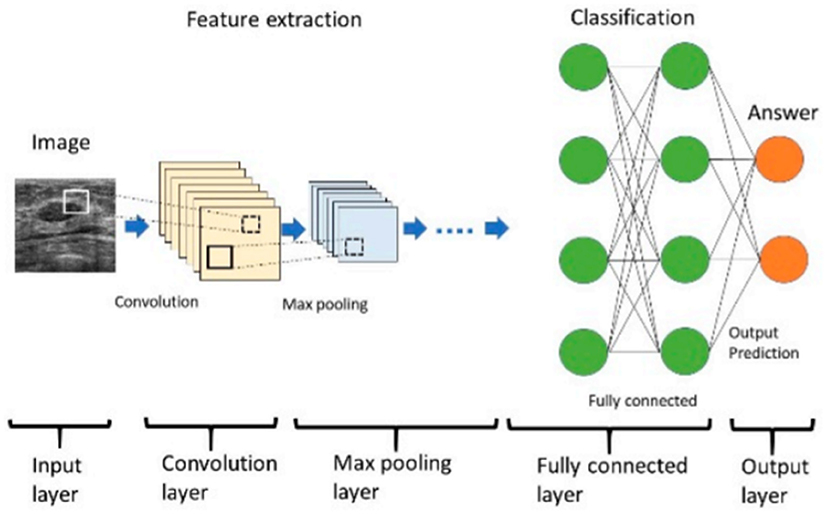
Figure 2. The architecture of a convolutional neural network [62].
In spite of the prospects of the deep learning models to improve OSCC outcomes through improved detection and diagnosis, most of these models have found widespread adoption in daily clinical practices. Several reasons have been attributed to the limited use of these models in clinical practice. A recent study showed that ethical concerns limited the potential use of these models in actual practices [63]. These ethical concerns include privacy and confidentiality, data and model bias, peer disagreement, responsibility gap, patient-clinician relationship, and patient autonomy [63]. Similarly, a recent study by Alabi et al., highlighted the concerns that are either inherent to the science of machine learning (technical) or the actual clinical implementation [64]. These include black box concern, amount of data, interpretability, explainability, and generalizability [64].
The strength of this systematic literature review is that it specifically examined the published studies that had examined deep learning in OSCC. This approach ensured that the contribution of the state-of-the-art deep learning techniques in OSCC was specifically examined. In addition, it offers the opportunity to understand the future research avenue of the application of deep learning in OSCC. An example of an exciting research area would be the development of new data fusion algorithms for improved prognostication in disease.
The main limitation is that most of the included studies used different performance metrics for the evaluation of the deep learning techniques. Similarly, the deep learning techniques used different data types in the analyses. Thus, it was challenging to make an insightful conclusion on the performance of these deep learning technique. Additionally, the dataset used to train the model was relatively small in most of the studies. Most of the developed deep learning models in the published studies were not externally validated. The study by Alhadi et al., provided an update on staging and World Health Organization grading as reliable OSCC prognostic indicators [65]. To the best of our knowledge, there is a dearth of published studies that have examined the application of machine learning for staging. Therefore, this serves as a potential area of further research in the future.
In conclusion, there is an increase in the application of deep learning for prognostication in OSCC. The deep learning models are poised to predict cancer prognosis more accurately. Thereby, offering precision and personalized management of the disease. It has shown to be better or equivalent to the current approaches in daily clinical practices. It is expected that the deep learning techniques can assist in the proper management of OSCC through improved diagnostic performance, insightful clinical decision making, streamline clinicians' work, offer a potential to reduce cancer care costs in the screening, and an effective assessment and surveillance of the disease. Thus, the clinicians and patients can spend more time in communication and in making shared decisions to improve the quality of care. In the future, it is important to develop deep learning models that combine multiple datasets from multiple modalities.
Summary Points
What Was Already Known on the Topic
There are several published studies on the application of machine learning techniques to analyze oral squamous cell carcinoma (OSCC).
What Knowledge This Study Adds
This study systematically reviewed the published studies that examined the application of deep machine learning techniques for prognostication in OSCC.
The majority of these studies used a convolutional neural network (CNN).
This review showed that a range of novel imaging modalities such as computed tomography (or enhanced computed tomography) images and spectra data have shown significant applicability to improve oral cancer outcomes.
The average specificity, sensitivity, area under receiving operating characteristics curve [AUC]), and accuracy for studies that used spectra data were 0.97, 0.99, 0.96, and 96.6%, respectively. Conversely, the average specificity, sensitivity, AUC, and accuracy for computed tomography images were 0.84, 0.81, 0.967, and 81.8%.
The study concluded that the deep learning techniques could offer assistance to the clinicians in making informed decisions regarding choosing treatment options to avoid under-treatment or unnecessary treatments for the better management of OSCC.
Data Availability Statement
The original contributions generated for the study are included in the article/supplementary material, further inquiries can be directed to the corresponding author/s.
Protocol and Registration
The databases of the International Prospective Register of Systematic Reviews (PROSPERO) were searched for any registered protocols on a similar topic to this systematic review. The protocol on the methodology of this review was therefore submitted to PROSPERO for a registration protocol number.
Author Contributions
RA, ME, AA, and AM: study concepts and study design and data analysis and interpretation. RA and OY: studies extraction. IB, OY, and AA: acquisition and quality control of included studies. RA, OY, AA, and AM: manuscript preparation. AM and IB: manuscript review. AA, RA, and IB: manuscript editing. All authors approved the final manuscript for submission.
Funding
The School of Technology and Innovations, University of Vaasa Scholarship Fund. Turku University Hospital Fund, Helsinki University Hospital Research Fund. Sigrid Jusélius Foundation. A profound appreciation to Ida Montinin Säätio for the doctoral support.
Conflict of Interest
The authors declare that the research was conducted in the absence of any commercial or financial relationships that could be construed as a potential conflict of interest.
Publisher's Note
All claims expressed in this article are solely those of the authors and do not necessarily represent those of their affiliated organizations, or those of the publisher, the editors and the reviewers. Any product that may be evaluated in this article, or claim that may be made by its manufacturer, is not guaranteed or endorsed by the publisher.
References
1. Sung H, Ferlay J, Siegel RL, Laversanne M, Soerjomataram I, Jemal A, et al. Global cancer statistics 2020: GLOBOCAN estimates of incidence and mortality worldwide for 36 cancers in 185 countries. CA Cancer J Clin. (2021) 71:209–49. doi: 10.3322/caac.21660
2. Kim DW, Lee S, Kwon S, Nam W, Cha I-H, Kim HJ. Deep learning-based survival prediction of oral cancer patients. Sci Rep. (2019) 9:6994. doi: 10.1038/s41598-019-43372-7
3. Warnakulasuriya S. Global epidemiology of oral and oropharyngeal cancer. Oral Oncol. (2009) 45:309–16. doi: 10.1016/j.oraloncology.2008.06.002
4. Siegel RL, Miller KD, Jemal A. Cancer statistics, 2020. CA Cancer J Clin. (2020) 70:7–30. doi: 10.3322/caac.21590
5. Huang S, Yang J, Fong S, Zhao Q. Artificial intelligence in cancer diagnosis and prognosis: opportunities and challenges. Cancer Lett. (2020) 471:61–71. doi: 10.1016/j.canlet.2019.12.007
6. Kim K-Y, Li S-J, Cha I-H. Nomogram for predicting survival for oral squamous cell carcinoma. Genomics Inform. (2010) 8:212–8. doi: 10.5808/GI.2010.8.4.212
7. da Silva SD, Alaoui-Jamali MA, Soares FA, Carraro DM, Brentani HP, Hier M, et al. TWIST1 is a molecular marker for a poor prognosis in oral cancer and represents a potential therapeutic target: prognostic-therapeutic impact of TWIST1. Cancer. (2014) 120:352–62. doi: 10.1002/cncr.28404
8. Lee S, Kim HJ, Cha I-H, Nam W. Prognostic value of lymph node count from selective neck dissection in oral squamous cell carcinoma. Int J Oral Maxillofac Surg. (2018) 47:953–8. doi: 10.1016/j.ijom.2018.03.007
9. Alabi RO, Elmusrati M, Sawazaki-Calone I, Kowalski LP, Haglund C, Coletta RD, et al. Machine learning application for prediction of locoregional recurrences in early oral tongue cancer: a Web-based prognostic tool. Virchows Arch. (2019) 475:489–97. doi: 10.1007/s00428-019-02642-5
10. Alabi RO, Elmusrati M, Sawazaki-Calone I, Kowalski LP, Haglund C, Coletta RD, et al. Comparison of supervised machine learning classification techniques in prediction of locoregional recurrences in early oral tongue cancer. Int J Med Inf. (2019) 136:104068. doi: 10.1016/j.ijmedinf.2019.104068
11. Zhu L, Luo W, Su M, Wei H, Wei J, Zhang X, et al. Comparison between artificial neural network and Cox regression model in predicting the survival rate of gastric cancer patients. Biomed Rep. (2013) 1:757–60. doi: 10.3892/br.2013.140
12. Faradmal J, Soltanian AR, Roshanaei G, Khodabakhshi R, Kasaeian A. Comparison of the performance of log-logistic regression and artificial neural networks for predicting breast cancer relapse. Asian Pac J Cancer Prev. (2014) 15:5883–8. doi: 10.7314/APJCP.2014.15.14.5883
13. Chien C-W, Lee Y-C, Ma T, Lee T-S, Lin Y-C, Wang W, et al. The application of artificial neural networks and decision tree model in predicting post-operative complication for gastric cancer patients. Hepatogastroenterology. (2008) 55:1140–5.
14. Gohari MR, Biglarian A, Bakhshi E, Pourhoseingholi MA. Use of an artificial neural network to determine prognostic factors in colorectal cancer patients. Asian Pac J Cancer Prev. (2011) 12:1469–72.
15. Shams W, Htike Z. Oral cancer prediction using gene expression profilling and machine learning. Int J Appl Eng Res. (2017) 12:4893–8. Available online at: http://irep.iium.edu.my/60438/
16. Aubreville M, Knipfer C, Oetter N, Jaremenko C, Rodner E, Denzler J, et al. Automatic classification of cancerous tissue in laserendomicroscopy images of the oral cavity using deep learning. Sci Rep. (2017) 7:11979. doi: 10.1038/s41598-017-12320-8
17. Uthoff RD, Song B, Sunny S, Patrick S, Suresh A, Kolur T, et al. Point-of-care, smartphone-based, dual-modality, dual-view, oral cancer screening device with neural network classification for low-resource communities. PLoS ONE. (2018) 13:e0207493. doi: 10.1371/journal.pone.0207493
18. Das DK, Bose S, Maiti AK, Mitra B, Mukherjee G, Dutta PK. Automatic identification of clinically relevant regions from oral tissue histological images for oral squamous cell carcinoma diagnosis. Tissue Cell. (2018) 53:111–9. doi: 10.1016/j.tice.2018.06.004
19. Song B, Sunny S, Uthoff RD, Patrick S, Suresh A, Kolur T, et al. Automatic classification of dual-modalilty, smartphone-based oral dysplasia and malignancy images using deep learning. Biomed Opt Express. (2018) 9:5318. doi: 10.1364/BOE.9.005318
20. Yan H, Yu M, Xia J, Zhu L, Zhang T, Zhu Z. Tongue squamous cell carcinoma discrimination with Raman spectroscopy and convolutional neural networks. Vib Spectrosc. (2019) 103:102938. doi: 10.1016/j.vibspec.2019.102938
21. Yu M, Yan H, Xia J, Zhu L, Zhang T, Zhu Z, et al. Deep convolutional neural networks for tongue squamous cell carcinoma classification using Raman spectroscopy. Photodiagnosis Photodyn Ther. (2019) 26:430–5. doi: 10.1016/j.pdpdt.2019.05.008
22. Chan C-H, Huang T-T, Chen C-Y, Lee C-C, Chan M-Y, Chung P-C. Texture-map-based branch-collaborative network for oral cancer detection. IEEE Trans Biomed Circuits Syst. (2019) 13:766–80. doi: 10.1109/TBCAS.2019.2918244
23. Sunny S, Baby A, James BL, Balaji D, N V A, Rana MH, Gurpur P, et al. A smart tele-cytology point-of-care platform for oral cancer screening. PLOS ONE. (2019) 14:e0224885. doi: 10.1371/journal.pone.0224885
24. Jeyaraj PR, Samuel Nadar ER. Computer-assisted medical image classification for early diagnosis of oral cancer employing deep learning algorithm. J Cancer Res Clin Oncol. (2019) 145:829–37. doi: 10.1007/s00432-018-02834-7
25. Ariji Y, Fukuda M, Kise Y, Nozawa M, Yanashita Y, Fujita H, et al. Contrast-enhanced computed tomography image assessment of cervical lymph node metastasis in patients with oral cancer by using a deep learning system of artificial intelligence. Oral Surg Oral Med Oral Pathol Oral Radiol. (2019) 127:458–63. doi: 10.1016/j.oooo.2018.10.002
26. Xu S, Liu Y, Hu W, Zhang C, Liu C, Zong Y, et al. An early diagnosis of oral cancer based on three-dimensional convolutional neural networks. IEEE Access. (2019) 7:158603–611. doi: 10.1109/ACCESS.2019.2950286
27. Das DK, Koley S, Bose S, Maiti AK, Mitra B, Mukherjee G, et al. Computer aided tool for automatic detection and delineation of nucleus from oral histopathology images for OSCC screening. Appl Soft Comput. (2019) 83:105642. doi: 10.1016/j.asoc.2019.105642
28. Shaban M, Khurram SA, Fraz MM, Alsubaie N, Masood I, Mushtaq S, et al. A novel digital score for abundance of tumour infiltrating lymphocytes predicts disease free survival in oral squamous cell carcinoma. Sci Rep. (2019) 9:13341. doi: 10.1038/s41598-019-49710-z
29. Jeyaraj PR, Panigrahi BK, Samuel Nadar ER. Classifier feature fusion using deep learning model for non-invasive detection of oral cancer from hyperspectral image. IETE J Res. (2020) 1–12. doi: 10.1080/03772063.2020.1786471
30. Panigrahi S, Das J, Swarnkar T. Capsule network based analysis of histopathological images of oral squamous cell carcinoma. J King Saud Univ Comput Inf Sci. (2020). doi: 10.1016/j.jksuci.2020.11.003
31. Kouznetsova VL, Li J, Romm E, Tsigelny IF. Finding distinctions between oral cancer and periodontitis using saliva metabolites and machine learning. Oral Dis. (2020) 27:484–93. doi: 10.1111/odi.13591
32. Das N, Hussain E, Mahanta LB. Automated classification of cells into multiple classes in epithelial tissue of oral squamous cell carcinoma using transfer learning and convolutional neural network. Neural Netw. (2020) 128:47–60. doi: 10.1016/j.neunet.2020.05.003
33. Ariji Y, Sugita Y, Nagao T, Nakayama A, Fukuda M, Kise Y, et al. CT evaluation of extranodal extension of cervical lymph node metastases in patients with oral squamous cell carcinoma using deep learning classification. Oral Radiol. (2020) 36:148–55. doi: 10.1007/s11282-019-00391-4
34. Xia J, Zhu L, Yu M, Zhang T, Zhu Z, Lou X, et al. Analysis and classification of oral tongue squamous cell carcinoma based on Raman spectroscopy and convolutional neural networks. J Mod Opt. (2020) 67:481–9. doi: 10.1080/09500340.2020.1742395
35. Fujima N, Andreu-Arasa VC, Meibom SK, Mercier GA, Salama AR, Truong MT, et al. Deep learning analysis using FDG-PET to predict treatment outcome in patients with oral cavity squamous cell carcinoma. Eur Radiol. (2020) 30:6322–30. doi: 10.1007/s00330-020-06982-8
36. Fu Q, Chen Y, Li Z, Jing Q, Hu C, Liu H, et al. A deep learning algorithm for detection of oral cavity squamous cell carcinoma from photographic images: a retrospective study. EClinicalMedicine. (2020) 27:100558. doi: 10.1016/j.eclinm.2020.100558
37. Ding J, Yu M, Zhu L, Zhang T, Xia J, Sun G. Diverse spectral band-based deep residual network for tongue squamous cell carcinoma classification using fiber optic Raman spectroscopy. Photodiagnosis Photodyn Ther. (2020) 32:102048. doi: 10.1016/j.pdpdt.2020.102048
38. Jubair F, Al-karadsheh O, Malamos D, Al Mahdi S, Saad Y, Hassona Y. A novel lightweight deep convolutional neural network for early detection of oral cancer. Oral Dis. (2021). doi: 10.1111/odi.13825. [Epub ahead of print].
39. Welikala RA, Remagnino P, Lim JH, Chan CS, Rajendran S, Kallarakkal TG, et al. Automated detection and classification of oral lesions using deep learning for early detection of oral cancer. IEEE Access. (2020) 8:132677–93. doi: 10.1109/ACCESS.2020.3010180
40. Paderno A, Piazza C, Del Bon F, Lancini D, Tanagli S, Deganello A, et al. Deep learning for automatic segmentation of oral and oropharyngeal cancer using narrow band imaging: preliminary experience in a clinical perspective. Front Oncol. (2021) 11:626602. doi: 10.3389/fonc.2021.626602
41. Tomita H, Yamashiro T, Heianna J, Nakasone T, Kobayashi T, Mishiro S, et al. Deep learning for the preoperative diagnosis of metastatic cervical lymph nodes on contrast-enhanced computed ToMography in patients with oral squamous cell carcinoma. Cancers. (2021) 13:600. doi: 10.3390/cancers13040600
42. Nanditha BR, Geetha Kiran A, Chandrashekar HS, Dinesh MS, Murali S. An ensemble deep neural network approach for oral cancer screening. Int J Online Biomed Eng IJOE. (2021) 17:121. doi: 10.3991/ijoe.v17i02.19207
43. Musulin J, Štifanić D, Zulijani A, Cabov T, Dekanić A, Car Z. An enhanced histopathology analysis: an ai-based system for multiclass grading of oral squamous cell carcinoma and segmenting of epithelial and stromal tissue. Cancers. (2021) 13:1784. doi: 10.3390/cancers13081784
44. Trajanovski S, Shan C, Weijtmans PJC, de Koning SGB, Ruers TJM. Tongue tumor detection in hyperspectral images using deep learning semantic segmentation. IEEE Trans Biomed Eng. (2021) 68:1330–40. doi: 10.1109/TBME.2020.3026683
45. Kim Y, Kang JW, Kang J, Kwon EJ, Ha M, Kim YK, et al. Novel deep learning-based survival prediction for oral cancer by analyzing tumor-infiltrating lymphocyte profiles through CIBERSORT. OncoImmunology. (2021) 10:1904573. doi: 10.1080/2162402X.2021.1904573
46. Page MJ, McKenzie JE, Bossuyt PM, Boutron I, Hoffmann TC, Mulrow CD, et al. The PRISMA 2020 statement: an updated guideline for reporting systematic reviews. BMJ. (2021) 372:n71. doi: 10.1136/bmj.n71
47. Moher D, Liberati A, Tetzlaff J, Altman DG, PRISMA Group. Preferred reporting items for systematic reviews and meta-analyses: the PRISMA statement. PLoS Med. (2009) 6:e1000097. doi: 10.1371/journal.pmed.1000097
48. Yan H, Yu M, Xia J, Zhu L, Zhang T, Zhu Z, et al. Diverse region-based CNN for tongue squamous cell carcinoma classification with raman spectroscopy. IEEE Access. (2020) 8:127313–28. doi: 10.1109/ACCESS.2020.3006567
49. Ariji Y, Fukuda M, Nozawa M, Kuwada C, Goto M, Ishibashi K, et al. Automatic detection of cervical lymph nodes in patients with oral squamous cell carcinoma using a deep learning technique: a preliminary study. Oral Radiol. (2021) 37:290–296. doi: 10.1007/s11282-020-00449-8
50. Zhu W, Xie L, Han J, Guo X. The application of deep learning in cancer prognosis prediction. Cancers. (2020) 12:603. doi: 10.3390/cancers12030603
51. Sharma N, Om H. Usage of probabilistic and general regression neural network for early detection and prevention of oral cancer. Sci World J. (2015) 2015:1–11. doi: 10.1155/2015/234191
52. de Tolentino ES, Centurion BS, Ferreira LHC, de Souza AP, Damante JH, Rubira-Bullen IRF. Oral adverse effects of head and neck radiotherapy: literature review and suggestion of a clinical oral care guideline for irradiated patients. J Appl Oral Sci Rev FOB. (2011) 19:448–54. doi: 10.1590/S1678-77572011000500003
53. Diamant A, Chatterjee A, Vallières M, Shenouda G, Seuntjens J. Deep learning in head & neck cancer outcome prediction. Sci Rep. (2019) 9:2764. doi: 10.1038/s41598-019-39206-1
54. Esteva A, Robicquet A, Ramsundar B, Kuleshov V, DePristo M, Chou K, et al. A guide to deep learning in healthcare. Nat Med. (2019) 25:24–9. doi: 10.1038/s41591-018-0316-z
55. Karadaghy OA, Shew M, New J, Bur AM. Development and assessment of a machine learning model to help predict survival among patients with oral squamous cell carcinoma. JAMA Otolaryngol Neck Surg. (2019) 145:1115. doi: 10.1001/jamaoto.2019.0981
56. Bur AM, Holcomb A, Goodwin S, Woodroof J, Karadaghy O, Shnayder Y, et al. Machine learning to predict occult nodal metastasis in early oral squamous cell carcinoma. Oral Oncol. (2019) 92:20–5. doi: 10.1016/j.oraloncology.2019.03.011
57. Alabi RO, Mäkitie AA, Pirinen M, Elmusrati M, Leivo I, Almangush A. Comparison of nomogram with machine learning techniques for prediction of overall survival in patients with tongue cancer. Int J Med Inf. (2021) 145:104313. doi: 10.1016/j.ijmedinf.2020.104313
58. Poplin R, Varadarajan AV, Blumer K, Liu Y, McConnell MV, Corrado GS, et al. Prediction of cardiovascular risk factors from retinal fundus photographs via deep learning. Nat Biomed Eng. (2018) 2:158–64. doi: 10.1038/s41551-018-0195-0
59. Ching T, Zhu X, Garmire LX. Cox-nnet: an artificial neural network method for prognosis prediction of high-throughput omics data. PLOS Comput Biol. (2018) 14:e1006076. doi: 10.1371/journal.pcbi.1006076
60. Chollet F. Xception: deep learning with depthwise separable convolutions. In: 2017 IEEE Conference on Computer Vision and Pattern Recognition (CVPR). Honolulu, HI: IEEE (2017). p. 1800–7. doi: 10.1109/CVPR.2017.195
61. Szegedy C, Liu W, Jia Y, Sermanet P, Reed S, Anguelov D, et al. Going deeper with convolutions. In: 2015 IEEE Conference on Computer Vision and Pattern Recognition (CVPR). Boston, MA: IEEE (2015). p. 1–9. doi: 10.1109/CVPR.2015.7298594
62. Fujioka T, Mori M, Kubota K, Oyama J, Yamaga E, Yashima Y, et al. The utility of deep learning in breast ultrasonic imaging: a review. Diagnostics. (2020) 10:1055. doi: 10.3390/diagnostics10121055
63. Alabi RO, Tero V, Mohammed E. Machine learning for prognosis of oral cancer: what are the ethical challenges? In: CEUR-Workshop Proceedings (2020) 2373:1–22. Available online at: http://ceur-ws.org/Vol-2737/
64. Alabi RO, Youssef O, Pirinen M, Elmusrati M, Mäkitie AA, Leivo I, et al. Machine learning in oral squamous cell carcinoma: current status, clinical concerns and prospects for future—A systematic review. Artif Intell Med. (2021) 115:102060. doi: 10.1016/j.artmed.2021.102060
Keywords: machine learning, deep learning, oral cancer, prognostication, systematic reveiw
Citation: Alabi RO, Bello IO, Youssef O, Elmusrati M, Mäkitie AA and Almangush A (2021) Utilizing Deep Machine Learning for Prognostication of Oral Squamous Cell Carcinoma—A Systematic Review. Front. Oral. Health 2:686863. doi: 10.3389/froh.2021.686863
Received: 28 March 2021; Accepted: 15 June 2021;
Published: 26 July 2021.
Edited by:
Carolina Cavalieri Gomes, Federal University of Minas Gerais, BrazilReviewed by:
Alan Roger Santos-Silva, State University of Campinas, BrazilShankargouda Patil, Jazan University, Saudi Arabia
Copyright © 2021 Alabi, Bello, Youssef, Elmusrati, Mäkitie and Almangush. This is an open-access article distributed under the terms of the Creative Commons Attribution License (CC BY). The use, distribution or reproduction in other forums is permitted, provided the original author(s) and the copyright owner(s) are credited and that the original publication in this journal is cited, in accordance with accepted academic practice. No use, distribution or reproduction is permitted which does not comply with these terms.
*Correspondence: Rasheed Omobolaji Alabi, rasheed.alabi@helsinki.fi