- 1Department of Oral Surgery and Pathology, School of Dentistry, Universidade Federal de Minas Gerais, Belo Horizonte, Brazil
- 2Department of Pathology, Biological Sciences Institute, Universidade Federal de Minas Gerais, Belo Horizonte, Brazil
- 3Department of Sanitary and Environmental Engineering, School of Engineering, Universidade Federal de Minas Gerais, Belo Horizonte, Brazil
- 4Department of Chemistry, Exact Sciences Institute, Universidade Federal de Minas Gerais, Belo Horizonte, Brazil
- 5Department of Analytical Chemistry, Institute of Chemistry, Universidade Federal da Bahia, Salvador, Brazil
Aging is not a matter of choice; it is our fate. The “time-dependent functional decline that affects most living organisms” is coupled with several alterations in cellular processes, such as cell senescence, epigenetic alterations, genomic instability, stem cell exhaustion, among others. Age-related morphological changes in dental follicles have been investigated for decades, mainly motivated by the fact that cysts and tumors may arise in association with unerupted and/or impacted teeth. The more we understand the physiology of dental follicles, the more we are able to contextualize biological events that can be associated with the occurrence of odontogenic lesions, whose incidence increases with age. Thus, our objective was to assess age-related changes in metabolic pathways of dental follicles associated with unerupted/impacted mandibular third molars from young and adult individuals. For this purpose, a convenience sample of formalin-fixed paraffin-embedded (FFPE) dental follicles from young (<16 y.o., n = 13) and adult (>26 y.o., n = 7) individuals was selected. Samples were analyzed by high-performance liquid chromatography-mass spectrometry (HPLC-MS)-based untargeted metabolomics. Multivariate and univariate analyses were conducted, and the prediction of altered pathways was performed by mummichog and Gene Set Enrichment Analysis (GSEA) approaches. Dental follicles from young and older individuals showed differences in pathways related to C21-steroid hormone biosynthesis, bile acid biosynthesis, galactose metabolism, androgen and estrogen biosynthesis, starch and sucrose metabolism, and lipoate metabolism. We conclude that metabolic pathways differences related to aging were observed between dental follicles from young and adult individuals. Our findings support that similar to other human tissues, dental follicles associated with unerupted tooth show alterations at a metabolic level with aging, which can pave the way for further studies on oral pathology, oral biology, and physiology.
Introduction
Dental follicles, also referred to as dental sacs, comprise a defined structure with a remarkable role in periodontogenesis and tooth eruption [1–4]. Classically, the definition of dental follicle includes the ectomesenchymal tissue derived from the neural crest that involves the tooth during development (i.e., odontogenesis) [5]. By a surgical definition, however, the term “dental follicle” comprises both the ectomesenchymal tissue around the developing tooth as well as the reduced enamel epithelium [6]. In cases when tooth eruption does not occur, the dental follicle remains attached to the coronal portion of the unerupted or impacted tooth [7]. Some pathological changes are described in this context, and are often age-dependent, as in the case of squamous and mucous cell metaplasia/prosoplasia. They involve molecular changes, such as increased expression of cell proliferation markers and lower expression of pro-apoptotic markers, mainly attributed to the epithelial component of this dental sac [8–12]. Some of these molecular and morphological alterations are considered as early signs of events that give rise to odontogenic lesions, such as developmental cysts and tumors. However, this is known to be a rare event [13].
Aging is a complex process that involves alterations in many cellular processes resulting in/from altered cell-cell interactions, loss of cell proteostasis, telomeric shortening, cell senescence, mitochondrial dysfunction, epigenetic alterations, genomic instability, stem cell exhaustion, among others [14]. The multi-level complexity underlying the aging process involves interactions between nucleic acids and proteins and is also influenced by environmental factors, constituting the aging phenotype [15]. Metabolomics is an emerging field among the so-called “-omics sciences” and correspond to a promising tool to understand the phenotype of cells, tissues, organs, and organisms in different conditions [16–18]. Thus, by exploring the metabolome, set of all metabolites in complex organisms, phenotypic changes related to biological functions can be revealed, especially when subtle changes in the concentration of metabolites can be targeted [19]. Some effective studies have been conducted in this context, unveiling the impact of aging on different tissues [15]. By exploring the aging physiology, the course of diseases in which the incidence increases with age, such as Alzheimer's disease, rheumatic disorders, cardiovascular, metabolic diseases, and, a prominent example, cancer, can be understood [20].
In the present study, we investigated the altered metabolic pathways in dental follicles of third-molar teeth from young and adult individuals by an untargeted metabolomics approach. We aimed to provide a list of predicted altered pathways, which can pave the way for further research that may use dental follicles/dental sacs as a model to understand aging, tooth eruption, bone physiology, and epithelium-mesenchymal interactions, as well as other phenomena that can be assessed in this peculiar tissue.
Materials and Methods
Patients and Tissue Specimens
A convenience sample (n = 20) of formalin-fixed paraffin-embedded (FFPE) tissue samples was retrieved from the archives of the Oral Pathology Service at Universidade Federal de Minas Gerais (UFMG, Brazil). Dental sacs associated with impacted/completely unerupted mandibular third molars with diagnosis confirmed by the histopathological analysis were used. The histopathological diagnosis of all samples was confirmed by three investigators (RSG, CCG, and VCB). After searching the files and retrieving the samples from the archives, we sought to select the best cases from the youngest and the oldest patients possible, based on tissue availability as well as histological features. Thus, samples showing intense inflammatory infiltrate or bony trabeculae were excluded since both could potentially affect the findings and/or metabolite extraction protocol. Samples with the clinical diagnosis of odontogenic cysts were also excluded.
Sample Preparation
Briefly, ~10 mg of tissue was obtained from FFPE specimens. Thick tissue sections of 20 μm were obtained using a manual microtome and collected into previously weighed microfuge tubes. The first two sections were discharged to remove potential surface contaminants. Tissues were then deparaffinized by four sequential incubations with cold xylene (Merck, Darmstadt, HE, Germany), each followed by centrifugations (15,000 × g, 15 min, 4°C). Residual solvent was dried using a dry bath incubator at 37°C and then weighed. The metabolite extraction solution was composed of a mixture of HPLC grade methanol:water:chloroform (3:1:1, v/v/v) (Sigma-Aldrich, San Luis, MO, USA). The volume of extraction solution was normalized per biomass of each tissue pellet (1 mg:20 μL of solution). Samples were homogenized using an ultrasonic bath for 10 min and centrifuged. The supernatant was filtered in nylon syringe-filters (13 mm diameter, 0.22 μm pore size), collected into inserts in glass vials, and sealed after sample preparation. A quality control (QC) sample was prepared by pooling 10 μL of each sample. Samples were stored at −80°C until analysis.
High-Performance Liquid Chromatography-Mass Spectrometry
Extracts were examined by HPLC-MS (Shimadzu HPLC System, LC 20A, Kyoto, Japan) coupled with a Bruker Quadrupole Time-of-Flight (QToF) mass spectrometer (Bruker micrOTOF QII, Billerica, MA, USA). A reverse phase, C18, 5 cm × 2.1 mm i.d., 1.9 μm chromatography column (Supelco Discovery HS, Bellefonte, PA, USA) was used for metabolite separation at 40°C. The HPLC was operated according to the following parameters: injection volume = 10 μL; flow rate = 0.3 mL/min. HPLC solvents were taken as follows: A, MilliQ Water with 0.1% (v/v) formic acid; and B, acetonitrile (ACN, Sigma-Aldrich, San Luis, MO, USA) with 0.1% (v/v) formic acid (Sigma-Aldrich, San Luis, MO, USA) for positive ionization mode; and A, MilliQ water; and B, ACN for negative ionization mode. The separation was conducted with gradient mode, in which the elution range was given as follows: 25–95% B (20 min); 95% B (3 min); 95–20%B (2 min); and 20% B for 5 min. Mass spectra were acquired using positive and negative-mode electrospray ionization (ESI+ and ESI-, respectively). The capillary voltage was 4,500 V for ESI+ and 3,500 V for ESI-. Nitrogen was used as cone and desolvation gas with a pressure of 2 bar and a flow rate of 7.0 L/h. The source temperature was 100°C, and the desolvation temperature was 180°C. Nitrogen was also used as collision gas and it was generated by a nitrogen generator (NM32LA, Peak Scientific, SP, Brazil). A full scan from 90–1200 m/z was obtained using sodium formate as calibrator. The QC sample was reinjected multiple times after each five sequential sample injections.
Data Preprocessing
Raw data were converted into .mzXML open format with MSConvert software (ProteoWizard, v.3.0). After conversion, optimization of parameters for data treatment was performed using QC data files. Data were optimized separately for ESI+ and ESI- in IPO package version 1.16.0 (Libiseller et al., 2015) in R Statistic workplace (R version 3.6.2). A two-step workflow was employed: the first consisted of optimization of initial default XCMS parameters followed by optimization of the whole set of parameters for peak picking and retgroup functions. We edited the minfrac and minsample parameters (set to 0.7 and 2, respectively), since they contribute to the concordance of m/z features within each group, increasing analytical robustness [21]. Optimized values of each parameter are shown in Supplementary File 1. Files were then processed on the XCMS package [22] in R Statistical workplace. Features extracted at <2.5 min and >20 min were removed. Duplicity was also checked by Pearson's correlation of features coeluted within a 2.5 min interval, considering a 5 ppm mass error (for this purpose, r > 0.7 correlation of peak intensities were considered as features duplicities and were removed from the data matrix). After this preprocessing step, the data matrix consisted of 3,530 and 475 m/z features, in ESI+ and ESI- modes, respectively.
Data Processing and Statistical Analysis
Multivariate analysis was conducted on MetaboAnalyst 4.0 online platform [23, 24]. Principal Component Analysis (PCA) and Partial Least Square Discriminant Analysis (PLS-DA) models were constructed based on data input after data filtering and missing value imputation. Features presenting more than 20% of missing values were excluded, and the residual missing values were replaced by the half of minimum value of intensity for each feature; data filtering based on relative SD (features presenting RSD > 25% for the peak intensities in the QCs were excluded) and interquartile range (IQR), eliminating features with poor analytical consistency and non-informative variables. Data were then median normalized, log-transformed, and Pareto-scaled for multivariate analysis. For univariate statistical analysis, the same filters and normalizations were applied except for the Pareto scaling. Shapiro-Wilk normality test, followed by Independent Samples t-Test (Welch's t-Test) (accounting for Levene's test for equality of variances) or Mann-Whitney U Tests were conducted on IBM SPSS Statistics 26 (IBM Corporation, v.26.0.0.0) when applicable. False Discovery Rate (FDR) was applied to employ the Benjamini-Hochberg method and a cutoff of 5% was considered.
Pathway Enrichment
As a functional interpretation of global metabolomics data can be challenging [25], we used the MetaboAnalyst 4.0 module called “MS Peaks to Pathways,” which integrates mummichog and Gene Set Enrichment Analysis (GSEA) algorithms to predict changes in metabolic pathways in given conditions [26]. Mummichog [27] is an algorithm based on Over Representation Analysis (ORA), which assumes that a certain degree of random errors during individual peak assignment will not change the collective behavior jointly determined by all metabolites involved in the pathways [28]. This algorithm tests if statistically significant peaks of a given list are enriched compared with null models drawn from the user input list. GSEA [29] is a cutoff-free method that evaluates the overall differences between two distributions based on Kolmogorov–Smirnov tests and favors the detection of subtle and consistent alterations that can be lost by ORA approaches.
For this purpose, a five-column table was built consisting data of 2,068 remaining features retained after data filtering of MetaboAnalyst for both ionization modes. The input table presented the headers “m.z” for mass-to-charge ratios; “r.t” for retention time; “p-values” from both Independent Samples t-Test or Mann-Whitney U Test (raw p-values, non-FDR corrected); “mode” indicating the ionization mode (positive or negative); and “t.score” calculated for each feature. A mass error of 10 ppm was used since it seemed more reasonable with QToF mass resolution, as Ms peaks to Pathways developers encourage inputs derived from high-resolution analytical platforms, such as Orbitrap or other Fourier transform mass spectrometry family. For mummichog analysis, a p-value cutoff of 0.05 was used. The library selected was the Human MFN Model, which is manually curated and originated from different sources (KEGG, BiGG, and Edinburgh Model) [30].
Results
Sample Characterization
Thirteen samples were included in the young group of patients (13–16 years old, median = 15 years old), and seven in the adult group (26–38 years old, median = 28 years old). Although we originally intended to have equal numbers of samples in both groups, samples from dental follicles of older patients were difficult to obtain, as most of the surgical procedures to remove unerupted thirds molars occur in adolescents. The young group was comprised of six boys and seven girls. Four females and three males formed the adult group. The main histological findings are shown in Figure 1. Not all samples exhibited evident epithelial lining, but the presence of islets of the odontogenic epithelium was frequently observed, especially among younger individuals (Figure 1c). We observed the presence of reduced enamel epithelium in 6/13 samples in the young group of patients. Squamous epithelium was present in 3/7 samples among adult individuals and was also present in focal areas of 2/13 samples from young patients.
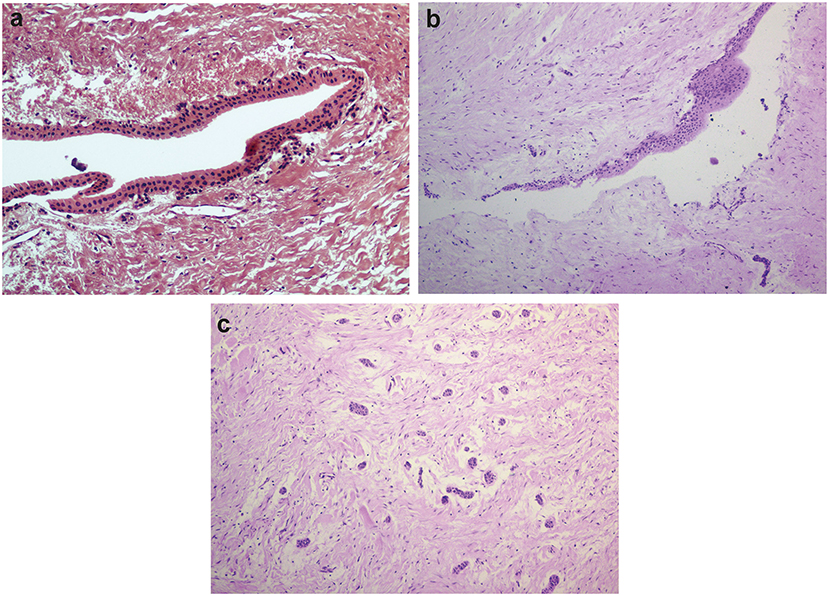
Figure 1. Histological features of dental follicles associated with unerupted mandibular third molars. (a) Reduced enamel epithelium lining connective tissue of dental follicle (x20 magnification). (b) Stratified squamous epithelial lining with focal thickening (x10 magnification). (c) Typical islets of the odontogenic epithelium were commonly observed in dental follicles from young individuals (x10 magnification). Slides were hematoxylin-eosin stained.
Quality Assessment and Statistical Evaluation
Principal Component Analysis indicated good analytical stability during the HPLC-MS run, for both positive and negative ionization modes (Supplementary File 2). PLS-DA analysis showed some degree of group separation, but the generated models could not be interpreted as predictive and were considered overfitted (Supplementary File 2). The univariate analysis resulted in a total of 287 molecular features statistically significant (p < 0.05, non-significant after FDR correction) from a total of 2,068 features in both positive and negative ionization modes. Only two m/z features were retained after 5% correction of FDR (m/z 647.2999, adjusted p-value < 0.0001; m/z 379.1334, adjusted p-value < 0.0001, both detected on ESI+ mode). Since a single m/z feature can match multiple compounds [27], they were not individually examined.
Pathway Enrichment
We carried out pathway enrichment based on mummichog and its integration with the GSEA approach, currently available within the “MS peaks to pathways” module of MetaboAnalyst 4.0. It is important to emphasize that the prediction algorithms were solely used as tools to identify metabolic pathways, with no purpose of annotating metabolites. Mummichog v.2 was used, and it includes retention time information to increase the confidence and robustness of potential compound matches [30], thus resulting in 248 matched compounds (Supplementary File 3). By executing the same analysis without retention time information (mummichog v.1), 1,355 compounds were matched and resulted pathways were reported (Supplementary File 4). For the sake of clarity, the discussion will be based on the results of the analysis that accounted for retention time analysis, although we cannot entirely discharge any other results. This analysis identified several pathways, including the C-21 steroid hormone biosynthesis, the bile acid biosynthesis, the galactose metabolism, the androgen and estrogen metabolism, the starch and sucrose and lipoate metabolic pathways (Gamma-p-values < 0.05) in mummichog approach. Tables 1, 2 provide the results from the mummichog approach alone and integrated with GSEA, respectively. Figure 2 shows scatter plots derived from each analysis.
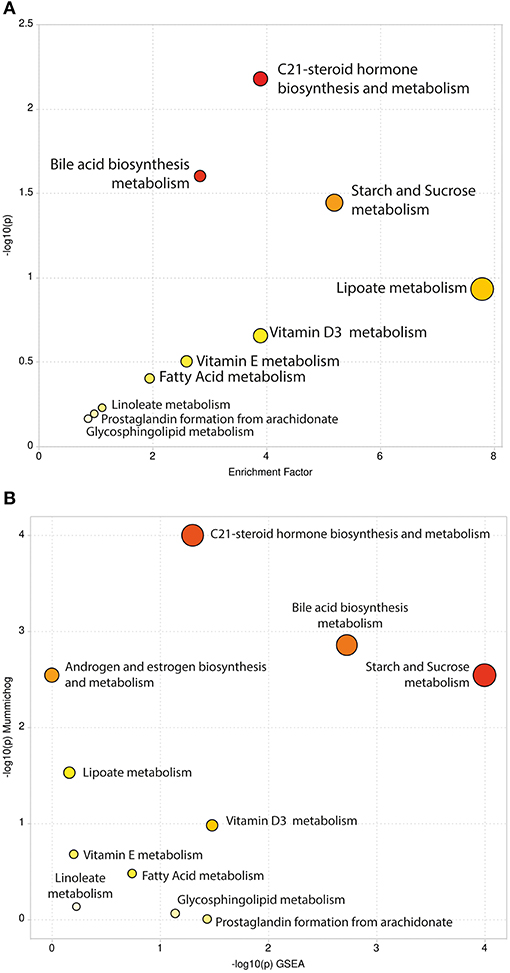
Figure 2. Scatter Plots of Pathway Enrichment Analysis provided by mummichog (A) and by its integration with GSEA (B). The color and size of each circle correspond to its p-value and enrichment factor, respectively. Darker tones indicate more statistically relevant predicted pathways. The size of each dot represents the ratio between significant pathway hits and the expected number of compound hits within the pathways.
Discussion
In the present study, we aimed to assess the metabolic alterations related to aging in dental sacs of impacted or unerupted mandibular third molars. These are the teeth that are the most frequently associated with odontogenic lesions development [13, 31] and are the last tooth to achieve occlusal plane, generally erupting between 17 and 26 years [13]. Considering that most of the third molar extraction surgeries are performed at a younger age [32, 33], it was difficult to retrieve dental follicle samples from patients above 30 years old. We observed the presence of reduced enamel epithelium in 6/13 samples in the young group of patients. Typical small islets and strands of odontogenic epithelium were most commonly observed in the young group, agreeing with the literature [7]. Squamous epithelium was present in 3/7 samples among adult individuals. If squamous metaplasia of the reduced enamel epithelium can represent an early sign of pathological change in dental follicles is debatable [8–12, 34], as tissues can naturally undergo morphological changes to adapt to new circumstances [7, 35–38]. The relevance of this debate relies on the absence of a consensus in the clinical practice guidelines justifying prophylactic surgical removal of asymptomatic disease-free impacted teeth [13].
Differences between the young and adult dental follicle groups were observed in many metabolic pathways, such as the C21-steroid hormone biosynthesis and metabolism, the bile acid biosynthesis, the galactose metabolism, the androgen and estrogen biosynthesis and metabolism, the starch and sucrose metabolism, and the lipoate metabolism. Although we were not able to observe which metabolic pathways had decreased or increased activities between experimental groups, these metabolic pathways were already described in the aging context and will be briefly discussed. It is important to point out that puberty is, likewise, a complex process coupled to several hormonal and physiological changes [39], which must be kept in mind when dealing with metabolomic studies assessing young patients and may explain, for example, the fact that we found differences in androgen and estrogen biosynthesis and metabolism between our experimental groups. We encourage further validation studies on larger sample size and based on targeted approaches.
C21-steroid hormone biosynthesis and metabolism comprises progesterone-aldosterone and progesterone-cortisol/cortisone axes that mediate a wide variety of biological processes such as reproductive function, sexual development, electrolyte balance, blood pressure, and stress responses [40]. Steroidal metabolome was previously demonstrated to be influenced by sex, age, and circadian cycle [40–42].
Bile acids (BAs) comprise a group of important physiological agents for cholesterol metabolism, intestinal nutrient absorption, biliary secretion of lipids, toxic metabolites, and xenobiotics [43]. Through activation of signaling pathways triggered by the activation of G-protein-coupled receptors (GPCRs) or nuclear bile acid receptors (including farnesoid-X receptor, FXR, also known as NR1H4), bile acids have been shown to regulate not only their own synthesis and enterohepatic recirculation, but also regulate triglyceride, cholesterol, energy, and glucose homeostasis [44]. Changes in serum and blood plasma levels were already found to be age-related and sex-specific [43, 45]. Interestingly, genes related to the bile secretion pathway were reported to act in tooth germs development of rats at RNA and protein levels [46].
The main pathway of galactose metabolism in humans involves the conversion of galactose into glucose by galactokinase and galactose-1-phosphate uridyl-transferase for glycolysis [47, 48]. In animal models, long-term D-galactose exposure induces the acquisition of an aging phenotype, which has been recognized as being similar to those in naturally aged mice and rats [49]. Age-induced animals exhibit increased reactive oxygen species (ROS) formation and decreased antioxidant enzyme activity in the brain, poor immune responses, cognitive dysfunctions, weakened motor function, and shortened lifespan [48, 50], mainly the effects of the accumulation of senescent cells in naturally aged organisms [20, 51–53]. In these models, the impacts of impaired galactose metabolism have already been studied on the brain, liver, lungs, heart, kidney, skin, reproductive systems, and others [54]. Other carbohydrates cause oxidative stress by activation of mitochondrial metabolism of glucose, leading to ROS generation [55]. In this context, starch and sucrose metabolism may also be related. In this case, ROS is generated through mitochondrial respiratory chain enzymes, xanthine oxidases, lipoxygenases, cyclooxygenases, nitric oxide synthases, and peroxidases [55].
Lipoate metabolism plays a key role in mitochondrial functions [56]. Lipoate is a covalently bound cofactor essential for five redox reactions in humans: four 2-oxoacid dehydrogenases and the glycine cleavage system (GCS). Two enzymes are derived from the energy metabolism, α-ketoglutarate dehydrogenase and pyruvate dehydrogenase; and three are derived from the amino acid metabolism, branched-chain ketoacid dehydrogenase, 2-oxoadipate dehydrogenase, and the GCS [57]. Lipoate is the conjugate base of lipoic acid (LA), and the most prevalent form of LA under physiological conditions. It presents a highly negative reduction potential, increases the expression of antioxidant enzymes, and participates in the recycling of vitamins C and E. Due to these properties, LA is called the “universal antioxidant” [58]. LA displays anti-apoptotic and anti-inflammatory properties in in vivo and in vitro studies. Importantly, it has been shown that LA reverses the age-associated loss of neurotransmitters and their receptors, which can underlie its effects on cognitive functions [59].
Cellular, genetic, endocrine, molecular, and environmental factors were also involved in tooth eruption (as we are dealing with unerupted and impacted teeth) and were not entirely covered in our study design. Nutritional status, body mass index (BMI), socioeconomic status, and others should be stratified in further studies with larger sample size, since these are important factors affecting the metabolome and, accordingly, tooth eruption [60–63]. Moreover, causes of primary failure of eruption or delayed tooth eruption are not fully understood, but disruptions and/or total failure in the ability of dental follicles leading to bone resorption throughout the gubernacular canal are discussed [60, 61, 64]. From this perspective, metabolic alterations in dental follicles may lead tooth eruption to fail and should be explored.
The more deeply we understand tissue physiology, the more we become capable of reframing several biological questions, changing the status quo, and casting light into new perspectives. Recently, from genetic approaches, it has been observed that normal, pathology-free tissues also can harbor pathological mutations, some of which are known oncogenes, implicating a new way to look at genetic alterations [65–67]. Benign tumors often can exhibit hotspot mutations and still present indolent clinical behavior [67–69]. Regarding dental follicles, no hotspot mutations were found until now [70]. Global profiling of DNA methylation and hydroxymethylation was also recently explored, and, despite age-related decrease of global DNA hydroxymethylation found, the biological meaning of this epigenetic profile change in dental follicles remains to be elucidated [71].
Genotype-phenotype interactions are complex, and many variables can elicit different responses at cells, tissues, and organisms. Metabolomics can bridge the gap, making paradoxes to be reconciled. Recently, metabolism from a wide variety of sources (e.g., alcohol and microbial metabolism) was found to modify DNA and histones and exerts specific effects on cell biology, systemic physiology, and pathology [72]. Polyphenism, a peculiar sub-type of phenotypic plasticity present in several animal species were ultimately proposed to happen in humans at a metabolic level [73]. In our study, the C21-steroid hormone biosynthesis, the bile acid biosynthesis, the galactose metabolism, the androgen and estrogen biosynthesis, the starch and sucrose and lipoate metabolism have been found to correlate to aging in dental sacs.
It is important to note that our predictions of altered metabolic pathways were performed based on untargeted HPLC-MS-based metabolomics of FFPE tissue samples, which has just recently become appreciated in the metabolomics field, inspiring protocols to be developed and optimized [74–76]. In this provocative and hypothesis-generator study, we shed light on few aspects of dental follicles physiology and so many others must be discovered. Dental follicles of unerupted/impacted teeth (i.e., dental sacs) are a unique tissue in the human body, hermetically encased within the alveolar bone. They are less exposed to exogenous environmental agents and are a reminiscence of tissue interactions dating back to early odontogenesis. We encourage scientists to consider this valuable tissue as a unique model and to explore its potential to answer a wide range of questions in the fields of physiology, developmental biology, and pathology.
Data Availability Statement
The original contributions generated for this study are included in the article/Supplementary Material, further inquiries can be directed to the corresponding author/s.
Ethics Statement
The studies involving human participants were reviewed and approved by Ethics Committee of Universidade Federal de Minas Gerais (UFMG, Brazil) (protocol number/approval: 30697120.9.0000.5149/4.082.478). Written informed consent to participate in this study was provided by the participants' legal guardian/next of kin.
Author Contributions
VB, JV, TP, FD-A, YL, and VM performed the experiments. VB, JV, RM-C, and FL-L performed the bioinformatic analyses. VB, JV, and RG performed data analyses. VB drafted the manuscript and generated the text and figures. LS and LL provided resources for HPLC-MS instrumentation. AM, GC, CG, and RG critically revised the manuscript. RG and CG provided leadership for the project. All authors contributed to the final manuscript.
Funding
This study was financed in part by the Coordination for the Improvement of Higher Education Personnel (CAPES)/Brazil [finance code 001] and by the Research Support Foundation of the State of Minas Gerais (FAPEMIG)/Brazil [grant number RED 00019-16]. VB, FD-A, RM-C, and TP received CAPES scholarship, and JV, YL, and VM received a National Council for Scientific and Technological Development (CNPq)/Brazil scholarship. CG and RG are research fellows at CNPq/Brazil.
Conflict of Interest
The authors declare that the research was conducted in the absence of any commercial or financial relationships that could be construed as a potential conflict of interest.
Acknowledgments
We would like to thank the entire staff of Oral Pathology Service (UFMG, Brazil).
Supplementary Material
The Supplementary Material for this article can be found online at: https://www.frontiersin.org/articles/10.3389/froh.2021.677731/full#supplementary-material
References
1. Cho M-I, and Garant PR. Development and general structure of the periodontium. Periodontol. (2000) 24:9–27. doi: 10.1034/j.1600-0757.2000.2240102.x
2. Cahill DR, and Marks SC. Tooth eruption: evidence for the central role of the dental follicle. J Oral Pathol Med. (1980) 9:189–200. doi: 10.1111/j.1600-0714.1980.tb00377.x
3. Marks SCJ. The basic and applied biology of tooth eruption. Connect Tissue Res. (1995) 32:149–57. doi: 10.3109/03008209509013718
4. Sarrafpour B, Swain M, Li Q, and Zoellner H. Tooth eruption results from bone remodelling driven by bite forces sensed by soft tissue dental follicles: a finite element analysis. PLoS ONE. (2013) 8:e58803. doi: 10.1371/journal.pone.0058803
5. Cate Ten AR, Mills C, and Solomon G. The development of the periodontium. A transplantation and autoradiographic study. Anat Rec. (1971) 170:365–79. doi: 10.1002/ar.1091700312
6. Larson EK, Cahill DR, Gorski JP, and Marks SC. The effect of removing the true dental follicle on premolar eruption in the dog. Arch Oral Biol. (1994) 39:271–5. doi: 10.1016/0003-9969(94)90116-3
7. Stanley HR, Krogh H, and Pannkuk E. Age changes in the epithelial components of follicles (dental sacs) associated with impacted third molars. Oral Surg Oral Med Oral Pathol. (1965) 19:128–39. doi: 10.1016/0030-4220(65)90226-4
8. Cabbar F, Güler N, Comunoglu N, Sençift K, and Çöloglu S. Determination of potential cellular proliferation in the odontogenic epithelia of the dental follicle of the asymptomatic impacted third molars. J Oral Maxillofac Surg. (2008) 66:2004–11. doi: 10.1016/j.joms.2008.06.011
9. Coşarcă AS, Mocan SL, Păcurar M, Fülöp E, and Ormenişan A. The evaluation of Ki67, p53, MCM3 and PCNA immunoexpressions at the level of the dental follicle of impacted teeth, dentigerous cysts and keratocystic odontogenic tumors. Rom J Morphol Embryol. (2016) 57:407–12.
10. Kucukkolbasi H, Esen A, and Erinanc O. Immunohistochemical analysis of Ki-67 in dental follicle of asymptomatic impacted third molars. J Oral Maxillofac Pathol. (2014) 18:189–93. doi: 10.4103/0973-029X.140737
11. Matsumoto MA, Filho HN, Jorge FM, Salvadori DMF, Marques MEA, and Ribeiro DA. Expression of cell cycle regulatory proteins in epithelial components of dental follicles. J Mol Histol. (2006). 37:127–31. doi: 10.1007/s10735-006-9048-3
12. Razavi SM, Hasheminia D, Mehdizade M, Movahedian B, and Keshani F. The relation of pericoronal third molar follicle dimension and bcl-2/ki-67 expression: an immunohistochemical study. Dent Res J. (2012) 9:S26–31. doi: 10.4103/1735-3327.107931
13. Ghaeminia H, Nienhuijs MEL, Toedtling V, Perry J, Tummers M, Hoppenreijs TJ, et al. Surgical removal versus retention for the management of asymptomatic disease-free impacted wisdom teeth. Cochrane Database Syst Rev. (2020) 5:CD003879. doi: 10.1002/14651858.CD003879.pub5
14. López-Otín C, Blasco MA, Partridge L, Serrano M, and Kroemer G. The hallmarks of aging. Cell. (2013) 153:1194–217. doi: 10.1016/j.cell.2013.05.039
15. Srivastava S. Emerging insights into the metabolic alterations in aging using metabolomics. Metabolites. (2019) 9:301. doi: 10.3390/metabo9120301
16. Nalbantoglu S. Metabolomics: basic principles and strategies. In: Nalbantoglu S, Amri H, editors. Molecular Medicine. Rijeka, Croatia: IntechOpen. p. 1–15. doi: 10.5772/intechopen.88563
17. Fiehn O. Metabolomics — the link between genotypes and phenotypes. In: Town C, editor. Functional Genomics. Dordrecht: Springer Netherlands (2002). p. 155–71. doi: 10.1007/978-94-010-0448-0_11
18. Patti GJ, Yanes O, and Siuzdak G. Metabolomics: the apogee of the omics trilogy. Nat Rev Mol Cell Biol. (2012) 13:263–9. doi: 10.1038/nrm3314
19. Armitage EG, and Barbas C. Metabolomics in cancer biomarker discovery: current trends and future perspectives. J Pharm Biomed Anal. (2014) 87:1–11. doi: 10.1016/j.jpba.2013.08.041
20. Campisi J. Aging, cellular senescence, and cancer. Annu Rev Physiol. (2013) 75:685–705. doi: 10.1146/annurev-physiol-030212-183653
21. Domingo-Almenara X, and Siuzdak G. Metabolomics Data Processing Using XCMS. In: Li S, editor. Computational Methods and Data Analysis for Metabolomics. New York, NY: Springer US (2020). p. 11–24. doi: 10.1007/978-1-0716-0239-3_2
22. Smith CA, Want EJ, O'Maille G, Abagyan R, and Siuzdak G. XCMS: processing mass spectrometry data for metabolite profiling using nonlinear peak alignment, matching, and identification. Anal Chem. (2006) 78:779–87. doi: 10.1021/ac051437y
23. Chong J, Wishart DS, and Xia J. Using MetaboAnalyst 4.0 for Comprehensive and integrative metabolomics data analysis. Curr Protoc Bioinforma. (2019) 68:1–128. doi: 10.1002/cpbi.86
24. Xia J, and Wishart DS. Web-based inference of biological patterns, functions and pathways from metabolomic data using MetaboAnalyst. Nat Protoc. (2011) 6:743–60. doi: 10.1038/nprot.2011.319
25. Schrimpe-Rutledge AC, Codreanu SG, Sherrod SD, and McLean JA. Untargeted Metabolomics strategies—challenges and emerging directions. J Am Soc Mass Spectrom. (2016) 27:1897–905. doi: 10.1007/s13361-016-1469-y
26. Chong J, Soufan O, Li C, Caraus I, Li S, Bourque G, Wishart DS, et al. MetaboAnalyst 4.0: towards more transparent and integrative metabolomics analysis. Nucleic Acids Res. (2018) 46:W486–94. doi: 10.1093/nar/gky310
27. Li S, Park Y, Duraisingham S, Strobel FH, Khan N, Soltow QA, et al. Predicting network activity from high throughput metabolomics. PLoS Comput Biol. (2013) 9:e1003123. doi: 10.1371/journal.pcbi.1003123
28. Chong J, and Xia J. Using MetaboAnalyst 4.0 for metabolomics data analysis, interpretation, and integration with other omics data. In: Li S, editor. Computational Methods and Data Analysis for Metabolomics. Methods in Molecular Biology. New York, NY: Springer US. p. 337–60. doi: 10.1007/978-1-0716-0239-3_17
29. Subramanian A, Tamayo P, Mootha VK, Mukherjee S, Ebert BL, Gillette MA, et al. Gene set enrichment analysis: a knowledge-based approach for interpreting genome-wide expression profiles. Proc Natl Acad Sci USA. (2005) 102:15545–50. doi: 10.1073/pnas.0506580102
30. Pang Z, Chong J, Li S, and Xia J. MetaboAnalystR 3.0: toward an optimized workflow for global metabolomics. Metabolites. (2020) 10:186. doi: 10.3390/metabo10050186
31. Mello FW, Melo G, Kammer PV, Speight PM, and Rivero ERC. Prevalence of odontogenic cysts and tumors associated with impacted third molars: a systematic review and meta-analysis. J Cranio-Maxillofacial Surg. (2019) 47:996–1002. doi: 10.1016/j.jcms.2019.03.026
32. Ventä I, Kylätie E, and Hiltunen K. Pathology related to third molars in the elderly persons. Clin Oral Investig. (2015) 19:1785–9. doi: 10.1007/s00784-014-1395-y
33. Kautto A, Vehkalahti MM, and Ventä I. Age of patient at the extraction of the third molar. Int J Oral Maxillofac Surg. (2018) 47:947–51. doi: 10.1016/j.ijom.2018.03.020
34. Adelsperger J, Campbell JH, Coates DB, Summerlin D-J, and Tomich CE. Early soft tissue pathosis associated with impacted third molars without pericoronal radiolucency. Oral Surg Oral Med Oral Pathol Oral Radiol Endodontol. (2000) 89:402–6. doi: 10.1016/S1079-2104(00)70119-3
35. Galluzzi L, Yamazaki T, and Kroemer G. Linking cellular stress responses to systemic homeostasis. Nat Rev Mol Cell Biol. (2018) 19:731–45. doi: 10.1038/s41580-018-0068-0
36. Daley TD, and Wysocki GP. The small dentigerous cyst. Oral Surg Oral Med Oral Pathol Oral Radiol Endodontol. (1995) 79:77–81. doi: 10.1016/S1079-2104(05)80078-2
37. Kim J, and Ellis GL. Dental follicular tissue: misinterpretation as odontogenic tumors. J Oral Maxillofac Surg. (1993) 51:762–7. doi: 10.1016/S0278-2391(10)80417-3
38. de Mello Palma V, Danesi CC, Arend CF, Venturini AB, Blaya DS, Neto MM, et al. Study of pathological changes in the dental follicle of disease-free impacted third molars. J Maxillofac Oral Surg. (2018) 17:611–5. doi: 10.1007/s12663-018-1131-2
39. Wood CL, Lane LC, and Cheetham T. Puberty: normal physiology (brief overview). Best Pract Res Clin Endocrinol Metab. (2019) 33:101265. doi: 10.1016/j.beem.2019.03.001
40. Ackermann D, Groessl M, Pruijm M, Ponte B, Escher G, D'Uscio CH, et al. Reference intervals for the urinary steroid metabolome: the impact of sex, age, day and night time on human adult steroidogenesis. PLoS ONE. (2019) 14:e0214549. doi: 10.1371/journal.pone.0214549
41. Schiffer L, Barnard L, Baranowski ES, Gilligan LC, Taylor AE, Arlt W, et al. Human steroid biosynthesis, metabolism and excretion are differentially reflected by serum and urine steroid metabolomes: a comprehensive review. J Steroid Biochem Mol Biol. (2019) 194:105439. doi: 10.1016/j.jsbmb.2019.105439
42. Van Cauter E. Diurnal and ultradian rhythms in human endocrine function: a minireview. Horm Res. (1990) 34:45–53. doi: 10.1159/000181794
43. Xie G, Wang Y, Wang X, Zhao A, Chen T, Ni Y, et al. Profiling of serum bile acids in a healthy Chinese population using UPLC–MS/MS. J Proteome Res. (2015) 14:850–9. doi: 10.1021/pr500920q
44. Thomas C, Pellicciari R, Pruzanski M, Auwerx J, and Schoonjans K. Targeting bile-acid signalling for metabolic diseases. Nat Rev Drug Discov. (2008) 7:678–93. doi: 10.1038/nrd2619
45. Frommherz L, Bub A, Hummel E, Rist MJ, Roth A, Watzl B, et al. Age-related changes of plasma bile acid concentrations in healthy adults—results from the cross-sectional KarMeN study. PLoS ONE. (2016) 11:e0153959. doi: 10.1371/journal.pone.0153959
46. Yang J, Lu X, Liu S, and Zhao S. The involvement of genes related to bile secretion pathway in rat tooth germ development. J Mol Histol. (2020) 51:99–107. doi: 10.1007/s10735-020-09861-0
47. Song X, Bao M, Li D, and Li YM. Advanced glycation in d-galactose induced mouse aging model. Mech Ageing Dev. (1999) 108:239–51. doi: 10.1016/S0047-6374(99)00022-6
48. Li H, Zheng L, Chen C, Liu X, and Zhang W. Brain senescence caused by elevated levels of reactive metabolite methylglyoxal on D-galactose-induced aging mice. Front Neurosci. (2019) 13:1–11. doi: 10.3389/fnins.2019.01004
49. Shwe T, Bo-Htay C, Leech T, Ongnok B, Jaiwongkum T, Kerdphoo S, et al. D-galactose-induced aging does not cause further deterioration in brain pathologies and cognitive decline in the obese condition. Exp Gerontol. (2020) 138:111001. doi: 10.1016/j.exger.2020.111001
50. Zhou YY, Ji XF, Fu JP, Zhu XJ, Li RH, Mu CK, et al. Gene transcriptional and metabolic profile changes in mimetic aging mice induced by d-galactose. PLoS ONE. (2015) 10:1–16. doi: 10.1371/journal.pone.0132088
51. He S, and Sharpless NE. Senescence in health and disease. Cell. (2017) 169:1000–11. doi: 10.1016/j.cell.2017.05.015
52. Campisi J, and d'Adda di Fagagna F. Cellular senescence: when bad things happen to good cells. Nat Rev Mol Cell Biol. (2007) 8:729–40. doi: 10.1038/nrm2233
53. Hernandez-Segura A, Nehme J, and Demaria M. Hallmarks of cellular senescence. Trends Cell Biol. (2018) 28:436–53. doi: 10.1016/j.tcb.2018.02.001
54. Azman KF, and Zakaria R. d-Galactose-induced accelerated aging model: an overview. Biogerontology. (2019) 20:763–82. doi: 10.1007/s10522-019-09837-y
55. Umbayev B, Askarova S, Almabayeva A, Saliev T, Masoud A-R, and Bulanin D. Galactose-induced skin aging: the role of oxidative stress. Oxid Med Cell Longev. (2020) 2020:1–15. doi: 10.1155/2020/7145656
56. Cronan JE. Progress in the enzymology of the mitochondrial diseases of lipoic acid requiring enzymes. Front Genet. (2020) 11:1–6. doi: 10.3389/fgene.2020.00510
57. Mayr JA, Feichtinger RG, Tort F, Ribes A, and Sperl W. Lipoic acid biosynthesis defects. J Inherit Metab Dis. (2014) 37:553–63. doi: 10.1007/s10545-014-9705-8
58. Moura F, de Andrade K, Farias dos Santos J, and Fonseca Goulart M. Lipoic acid: its antioxidant and anti-inflammatory role and clinical applications. Curr Top Med Chem. (2015) 15:458–83. doi: 10.2174/1568026615666150114161358
59. Molz P, and Schröder N. Potential therapeutic effects of lipoic acid on memory deficits related to aging and neurodegeneration. Front Pharmacol. (2017) 8:849. doi: 10.3389/fphar.2017.00849
60. Wise GE, Frazier-Bowers S, and D'Souza RN. Cellular, molecular, and genetic determinants of tooth eruption. Crit Rev Oral Biol Med. (2002) 13:323–35. doi: 10.1177/154411130201300403
61. Almonaitiene R, Balciuniene I, and Tutkuviene J. Factors influencing permanent teeth eruption. Part one–general factors. Stomatologija. (2010) 12:67–72.
62. Rattray NJW, Deziel NC, Wallach JD, Khan SA, Vasiliou V, Ioannidis JPA, et al. Beyond genomics: understanding exposotypes through metabolomics. Hum Genomics. (2018) 12:4. doi: 10.1186/s40246-018-0134-x
63. Robinson O, Carter AR, Ala-Korpela M, Casas JP, Chaturvedi N, Engmann J, et al. Metabolic profiles of socio-economic position: a multi-cohort analysis. Int J Epidemiol. (2020) 1–15. doi: 10.1093/ije/dyaa188
64. Kjær I. Mechanism of human tooth eruption: review article including a new theory for future studies on the eruption process. Scientifica. (2014) 2014:1–13. doi: 10.1155/2014/341905
65. Martincorena I, Roshan A, Gerstung M, Ellis P, Van Loo P, McLaren S, et al. High burden and pervasive positive selection of somatic mutations in normal human skin. Science (80-). (2015) 348:880–6. doi: 10.1126/science.aaa6806
66. Lac V, Nazeran TM, Tessier-Cloutier B, Aguirre-Hernandez R, Albert A, Lum A, et al. Oncogenic mutations in histologically normal endometrium: the new normal? J Pathol. (2019) 249:173–81. doi: 10.1002/path.5314
67. Marino-Enriquez A, and Fletcher CDM. Shouldn't we care about the biology of benign tumours? Nat Rev Cancer. (2014) 14:701–2. doi: 10.1038/nrc3845
68. Coura BP, Bernardes VF, de Sousa SF, França JA, Pereira NB, Pontes HAR, et al. KRAS mutations drive adenomatoid odontogenic tumor and are independent of clinicopathological features. Mod Pathol. (2019) 32:799–806. doi: 10.1038/s41379-018-0194-4
69. Coura BP, Bernardes VF, de Sousa SF, Diniz MG, Moreira RG, de Andrade BAB, et al. Targeted next-generation sequencing and allele-specific quantitative PCR of laser capture microdissected samples uncover molecular differences in mixed odontogenic tumors. J Mol Diagnostics. (2020) 22:1393–9. doi: 10.1016/j.jmoldx.2020.08.005
70. Coura BP, de Resende TAC, de Menezes VCB, Bernardes VF, de Sousa SF, Diniz MG, et al. Assessing pathogenic mutations in dental follicles as an attempt to identify early events in odontogenic tumours tumourigenesis. Arch Oral Biol. (2020) 113:104523. doi: 10.1016/j.archoralbio.2019.104523
71. de Menezes VCB, Siqueira de EC, Costa dos SFS, de Souza FTA, de Souza RP, Gomez RS, et al. Effects of aging on DNA hydroxymethylation and methylation in human dental follicles. Arch Oral Biol. (2020) 118:104856. doi: 10.1016/j.archoralbio.2020.104856
72. Dai Z, Ramesh V, and Locasale JW. The evolving metabolic landscape of chromatin biology and epigenetics. Nat Rev Genet. (2020) 21:737–53. doi: 10.1038/s41576-020-0270-8
73. Yang C-H, and Andrew Pospisilik J. Polyphenism – a window into gene-environment interactions and phenotypic plasticity. Front Genet. (2019) 10:132. doi: 10.3389/fgene.2019.00132
74. Kelly AD, Breitkopf SB, Yuan M, Goldsmith J, Spentzos D, and Asara JM. Metabolomic profiling from formalin-fixed, paraffin-embedded tumor tissue using targeted LC/MS/MS: application in sarcoma. PLoS ONE. (2011) 6:e25357. doi: 10.1371/journal.pone.0025357
75. Donczo B, and Guttman A. Biomedical analysis of formalin-fixed, paraffin-embedded tissue samples: the Holy Grail for molecular diagnostics. J Pharm Biomed Anal. (2018) 155:125–34. doi: 10.1016/j.jpba.2018.03.065
Keywords: aging, dental follicle, dental sac, developmental biology, oral pathology, untargeted metabolomics, LC-MS
Citation: Bastos VC, Vitório JG, Martins-Chaves RR, Leite-Lima F, Lebron YAR, Moreira VR, Duarte-Andrade FF, Pereira TSF, Santos LVS, Lange LC, Macedo AN, Canuto GAB, Gomes CC and Gomez RS (2021) Age-Related Metabolic Pathways Changes in Dental Follicles: A Pilot Study. Front. Oral. Health 2:677731. doi: 10.3389/froh.2021.677731
Received: 08 March 2021; Accepted: 12 May 2021;
Published: 18 June 2021.
Edited by:
Eliete Neves Da Silva Guerra, University of Brasilia, BrazilReviewed by:
Elena Riet Correa Rivero, Federal University of Santa Catarina, BrazilRogelio González-González, Juárez University of the State of Durango, Mexico
Celeste Sanchez-Romero, University of the Republic, Uruguay
Copyright © 2021 Bastos, Vitório, Martins-Chaves, Leite-Lima, Lebron, Moreira, Duarte-Andrade, Pereira, Santos, Lange, Macedo, Canuto, Gomes and Gomez. This is an open-access article distributed under the terms of the Creative Commons Attribution License (CC BY). The use, distribution or reproduction in other forums is permitted, provided the original author(s) and the copyright owner(s) are credited and that the original publication in this journal is cited, in accordance with accepted academic practice. No use, distribution or reproduction is permitted which does not comply with these terms.
*Correspondence: Ricardo Santiago Gomez, cnNnb21lekB1Zm1nLmJy
†These authors have contributed equally to this work and share senior authorship