- 1Department of Chemistry, Biology, and Biotechnology, Università degli Studi di Perugia, Perugia, Italy
- 2Department of Biological and Environmental Sciences and Technologies (DISTeBA), University of Salento, Lecce, Italy
Inspired by some traits of human intelligence, it is proposed that wetware approaches based on molecular, supramolecular, and systems chemistry can provide valuable models and tools for novel forms of robotics and AI, being constituted by soft matter and fluid states as the human nervous system and, more generally, life, is. Bottom-up mimicries of intelligence range from the molecular world to the multicellular level, i.e., from the Ångström (
1 Introduction
The research field of AI and Robotics has the declared objective of building intelligent machines and the implicit purpose of understanding what intelligence is, especially that shown by humans (Russell and Norvig, 2010), and according to certain paradigms. Although,as humans, we have been meditating on our intelligence, at least, since the birth of philosophy in ancient Greece (i.e., since the far VI century BC), we are still missing a universally accepted definition. Minsky (2006) coined the expression “suitcase word” for terms like intelligence, which is multifaceted. Human intelligence has many features, and its definition might depend on the context. Attempting to define it from the Complexity Science viewpoint, it might be proclaimed that intelligence is an amazing emergent property of the human nervous system (HNS; Gentili, 2021a). As such, it is expected that by assembling artificial complex systems that are architectural mimicry of the HNS, some performances of human intelligence should pop up. This idea is supported by the methodology that the cognitive scientists Gallistel and King (2009) and the neuroscientist Marr (2010) have proposed to understand any biological complex system. Their methodology requires analysing the HNS at three distinct levels. The first analysis is at the “computational level” and requires determining inputs, outputs, and the system’s computations. The second one is at the “algorithmic level” and involves formulating algorithms reproducing the previously found computations. The final analysis is performed at the “implementation level” and requires designing and implementing artificial mechanisms that run the formulated algorithms. This methodology has been embraced by chemists who try to mimic some performances of human intelligence using chemistry: they contribute to AI and Robotics by tracing a new path and developing unconventional Chemical Artificial Intelligence (CAI; Gentili, 2013). In the first part of this series (Gentili and Stano, 2023b), it has been demonstrated—according to the current experimental investigations—that molecules and supramolecules can be exploited to reproduce some elementary functions, such as “sensing,” “computing,” “communicating,” and “working.” In this second part, it will be shown that it is feasible to imitate more elaborate performances of human intelligence by recurring to Systems Chemistry. Systems Chemistry refers to the design of complex mixtures of properly chosen chemical compounds that can give rise to emergent properties, i.e., properties that go beyond the sum of the characteristics of the system’s individual constituents (Ashkenasy et al., 2017). The emergent properties of artificial chemical mixtures, designed according to the three-level analysis mentioned above, can show some primary forms of intelligence.
2 Chemical robotics
The ultimate and ambitious goal of Systems Chemistry in the field of CAI is the development of the so-called Chemical Robotics (also named Molecular Robotics or Cybernetics; Hagiya et al., 2014; Murata et al., 2022). Chemical Robots are supposed to be autonomous and adaptive molecular assemblies, confined through a membrane, and provided with four other modules, which are also the prerogatives of every living cell (see Figure 1). The first is the sensory module, made of the molecular and supramolecular logic gates described in part I (Gentili and Stano, 2023b). The sensory module guarantees data collection related to the surrounding environment’s features and the robot’s internal state. The sensory data must be processed by an artificial neural network module, which also takes the decisions. The resolutions trigger the action of the effectors’ module, which is constituted by proper assemblies of molecular machines. Finally, the intelligent activities of any Chemical Robot should be sustained by a metabolic unit. An obvious application of Chemical Robots can be identified in nanomedicine, i.e., as smart drug-delivery (or drug-producing) agents. In such a scenario, miniaturized Chemical Robots will be implanted in living beings where they will interplay with living cells and organelles to perform bio-medical actions, such as releasing a drug in the right place and at the right moment. They should become “Medical Doctors within individual cells” (Shapiro, 2012) or auxiliary elements of the natural immune systems. These autonomous elements should be programmable molecular computing devices capable of computing synthetic biopolymers and releasing them in response to environmental inputs. It is convenient, then, to describe their design and operation in the computationalist paradigm, here based on and related to the central dogma of molecular biology (Crick, 1970). All the potential performances of any living being are encoded in the chemical composition of a polymer, which is the DNA. Its information is transcribed into a specific sequence of the polymer RNA through RNA polymerase. Finally, it is translated into a peculiar poly-aminoacidic sequence (i.e., a protein) through the ribosome. This naturally occurring way of processing information has striking similarities with the working principle of the universal computing machine proposed by Alan Turing in 1936. The Turing machine (Turing, 1936) works on an infinitely long tape (which can be a potentially unbounded DNA or RNA polymer). It contains a head (it can be RNA polymerase or the ribosome) that can write and read symbols while moving forward and backwards on the tape. A Chemical Robot, driven by a DNA- or RNA-based Turing machine, can face any solvable computing problem and regulate biomolecular processes in vivo because it can interact directly with the biochemical environment, offering new avenues for gene therapy (Varghese et al., 2015). Chemical Robots may also be employed to safeguard the environment and face problems related to energy and food supplies (Murata et al., 2013). Chemical Robot’s performances depend on the compartmentalisation of its modules and how well they are assembled and integrated. The perfect epitome is represented by any unicellular microorganism. Hence, the development of Chemical Robotics can be rightly embedded within the broad field of synthetic biology. In particular, the Chemical Robot we are referring to can be fabricated through the bottom-up approach (Luisi, 2002; Guindani et al., 2022), i.e., by assembling all the molecular elements required to ensure the expected functions. As a result, the so-called synthetic (or artificial) cells are obtained.
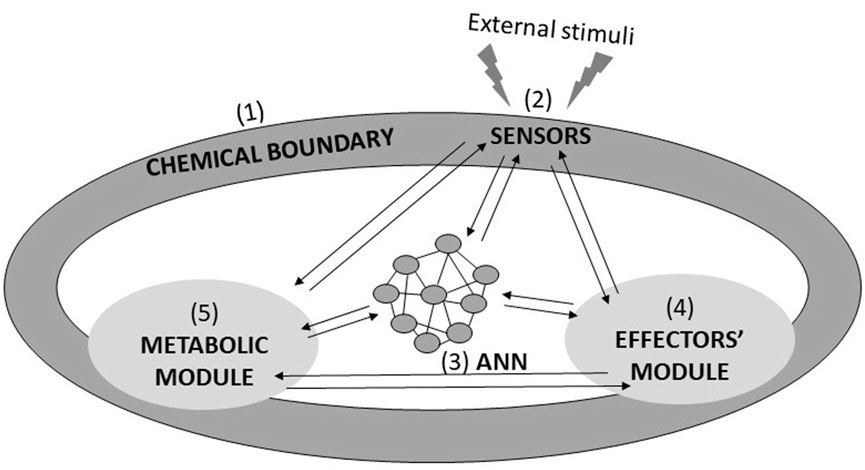
FIGURE 1. Sketch showing the required fundamental elements of a Chemical Robot, whose prototype is any unicellular organism. A Chemical Robot is a chemical system confined by (1) a chemical boundary along which are deployed (2) the sensors; it includes (3) the Artificial Neural Network (ANN), (4) the Effectors’ module, and (5) the metabolic unit. All the listed components must be strongly intertwined, conferring to the Chemical Robot the power of perceiving, generating knowledge, planning and acting: in a nutshell, autonomy and intelligence. It should perform analytical processing, practical, and regulatory functions (Zhang et al., 2019).
2.1 Artificial neural networks
A pivotal role in a Chemical Robot is played by its artificial neural network module. As mentioned above, it can be a DNA- or RNA-based Turing machine, or it might be more similar to the human brain. The human brain is a complex network of about
Proteins are valuable neural surrogates at the molecular level (Bray, 1995). They constitute the basic information-processing elements of the complex intracellular molecular reaction network that controls the physiology of living cells and organisms. In the signalling network of every cell, a protein, which links a substrate in its active site and transforms it chemically into a specific product, acts as a computational node. The information is encoded in the three-dimensional structure of the molecules, and it is primarily communicated through diffusion and, when possible, through advection of the liquid solution. The simple molecules that work as inputs and outputs of all the protein-based information-processing events establish most of the links among the proteins. Sometimes, the link is direct and implies protein-protein associations. In cellular signalling networks, a high degree of nonlinearity, mimicking that of neural networks, is guaranteed by allosteric proteins. A protein is allosteric when its activity towards a peculiar substrate is affected by other molecules (called effectors) that link to other sites of the same protein (Dokholyan, 2016). The effectors can accelerate or decelerate the reaction governed by the protein. Sometimes, the chemical species produced by a protein can play as its own effector and positive or negative feedback actions can be implemented. The protein-based signalling networks are the brains of living cells because they process the sensory data related to the external environment and internal cellular state and take the appropriate course of action, triggering specific epigenetic events, i.e., activating and/or inhibiting the expression of specific genes in DNA (Roederer, 2005).
The other two fundamental macromolecular ingredients of every cell, i.e., DNA and RNA, have also been proposed as basic elements for implementing ANNs. DNA and RNA exist as strands made of sequences of four smaller molecules known as nucleotides: Adenine (A), thymine (T), guanine (G), and cytosine (C) in the case of DNA, and in RNA, uracil (U) substitutes T. Each nucleotide is complementary to another specific nucleotide due to the number and strength of hydrogen bonds that are established: A is complementary to T (or U in the case of RNA), and C to G. Based on this peculiar chemical complementarity, it is possible to exploit partially doubled-stranded molecules of DNA or RNA as neural surrogates. When a single-stranded DNA (or RNA) molecule finds a partially double-stranded molecule with a complementary sequence, they bind, causing the partially doubled-stranded molecule to shed the strand that was previously on it. The single-stranded DNA molecule that successfully binds to the partially double-stranded DNA molecule acts as the system’s input, whereas the strand kicked off by the bonding DNA molecule acts as the system’s output. Once released, an output strand can be input by interplaying with another partially double-stranded DNA molecule. ANNs built using DNA or RNA hybridisation reactions, in which two complementary single-stranded DNA or RNA molecules bond to form a double-stranded molecule, can run challenging computations (Qiu et al., 2013). They can be employed to solve NP-complete problems (Cox et al., 1999), such as the Travelling Salesman Problem (Adleman, 1994), or they can be appropriate for the recognition of variable patterns (Cherry and Qian, 2018).
Neural surrogates can be alternatively implemented through proper reactive chemical systems that, when maintained far from the thermodynamic equilibrium, reproduce the dynamics of real neurons (Przyczyna et al., 2020). They replicate the oscillatory, chaotic, or excitable dynamic regimes of real neurons (Izhikevich, 2007). A well-known instance is the Belousov-Zhabotinsky (BZ) reaction, which can proceed in oscillatory, chaotic, or excitable regimes depending on the physicochemical conditions (Epstein and Pojman, 1998). Information is encoded in the concentrations of specific chemical species, which determine the electric potential values and the UV-visible absorption properties of the macroscopic solutions. Distinct neural surrogates of the type of the BZ reaction can communicate through diffusion or advection, as in the case of the proteins, or, alternatively, through the propagation of chemical waves. Communication becomes ultrafast when the light transmitted or emitted by the neural surrogates is used as signals. Optical signals are quickly transferred among physically distant neural surrogates (Gentili et al., 2017; Gentili et al., 2018). When all the neural surrogates that are reciprocally linked are in their oscillatory regime, it is possible to implement Spiking Neural Networks (SNNs). SNNs, also called Oscillatory Neural Networks (ONNs), constitute a promising computing paradigm. It is analog because the information is encoded on the frequency of the oscillators and the phase relations between oscillators. It is low-power because the information is not encoded in the amplitude of the signals, and therefore the signal amplitude can be very low, reducing power consumption (Corentin et al., 2021). ONNs are helpful for recognising variable patterns. Memorised patterns are synchronised oscillatory states in which neurons fire periodically with certain relations between their phases (Hoppensteadt and Izhikevich, 2000). ONNs also promise to contribute to cutting-edge computing that should go beyond Moore’s law by devising alternative architectures to current electronic computers. Moore’s law plays a relevant role when we face the computation of hard problems, i.e., when we face exponential problems (or NP problems) with large dimensions. We can expect to solve accurately NP-problems only if we devise ultrafast computing machines. Since Moore’s law will stop holding soon because transistors are made of a few atoms, the only way to solve NP problems having large dimensions is to design novel computing architectures, revolutionizing the Von Neumann architecture of current electronic computers (Csaba and Porod, 2020). Such hard problems can also be faced through a top-down strategy (Gorecki, 2022): After choosing a proper computing chemical medium, how the input and the output information are encoded must be fixed. The properties of the medium must be controlled by some adjustable macroscopic parameters. Within this strategy, the values of parameters for which the output gives the most accurate solution must be found. To perform such optimization, several training examples are needed to verify the accuracy of computation performed by the medium.
3 Sophisticated reasonings: fuzzy logic and Bayesian inference
Chemical Robots will never be capable of tightly reproducing the power of human minds if they rely only upon Boolean logic. They might need to take rational decisions in environments dominated by uncertainty, partiality and relativity of truth. In these situations, other types of logic and inference are required. For instance, fuzzy logic is particularly helpful in the case of partially true statements and context-dependent decisions. It reproduces how humans make decisions using syllogistic statements of the type IF…, THEN… expressed through the natural language (Zadeh, 1997). Adjectives employed in the formulation of syllogistic statements are fuzzy sets granulating the numerical values of the variables. The meaning of any adjective is context-dependent, likewise the position and shape of fuzzy sets. A reason why fuzzy logic is a good model of human capability to compute with words has been attributed to some intrinsically fuzzy features of the HNS (Zadeh, 2002; Gentili, 2014). The information of any physicochemical stimulus is encoded hierarchically (Figure 2 shows the specific case of human vision): the modality at the molecular level, the intensity and its time-evolution at the cellular level, and the spatial distribution at the sensory organ level. These features of any stimulus are encoded as fuzzy information because the collections of molecules and cells involved in any sensory system work as ensembles of fuzzy sets, granulating the attributes of the physicochemical variables. The afferent neurons connecting the sensory cells to the brain operate further granulations of the variables through their peculiar receptive fields (Gentili, 2018). All the higher-level intelligent activities, such as sensory perception, knowledge generation, planning, and decision-making, are believed to take place in the neocortex, which comprises almost
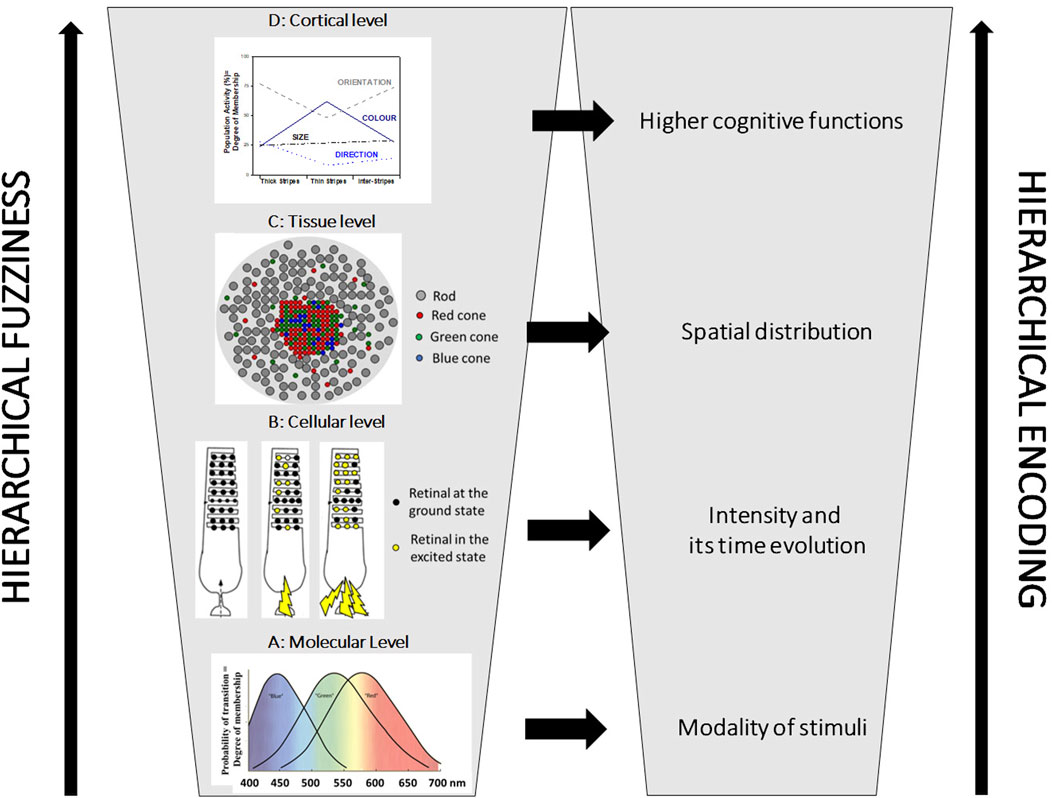
FIGURE 2. The hierarchical fuzziness and encoding in human vision. In the bottom left part, graph (A) shows the absorption bands of the three retinals that belong to the so-called “Blue,” “Green,” and “Red” cones. They are three molecular fuzzy sets granulating the visible spectral region. They allow encoding the modality of the light stimuli. Graph (B) shows the schematic structure of a rod with many retinals in its outer segment: lights (represented by the yellow lightning) with the same spectral compositions but different intensities excite different amounts of the retinals. The intensity of the light is encoded as the degree of membership to the cellular fuzzy set (see the right panel). Graph (C) represents a sketch of the retina. The spatial distribution of the light stimuli is encoded at the tissue level through the array of cellular fuzzy sets on the retina (see the right panel). Plot (D) shows that all three compartments (thick stripes, thin stripes, and inter-stripes) of the cortical visual area V2 participate, at different degrees, in the experience of colour, orientation, size, and direction of the objects that humans see [data extrapolated from Gegenfurtner (2003)]. The three compartments are intrinsically fuzzy. They are connected to the fuzzy Cortical Columns of the visual area V1.
It is a combination of the current information, represented by the likelihood
Such interpretation of sophisticated human reasoning blazes a trail for its chemical implementation. Chemistry will allow the imitation of the hierarchical fuzziness and information encoding of the HNS through a bottom-up approach. In the first part of this series of two papers (Gentili and Stano, 2023b), it has already been mentioned that single fuzzy sets can be implemented at the molecular level through those compounds that exist as ensembles of conformers (conformers are molecules that differ just in the 3D arrangement of their constitutive atoms). Any conformational collection has context-dependent features: the conformers’ identity and relative abundance (Gentili and Perez-Mercader, 2022). Mixing properly chosen molecular fuzzy sets enlarge the power of information encoding.
Suppose the intermingled molecular fuzzy sets are sensitive to the same physicochemical variables. In that case, they can carry out the granulation and graduation of the variables, like the three retinals (the “Blue,” “Green,” and “Red” ones) shown in Figure 2A. The mimicry of human colour vision at the level of the retina, implemented through mixtures of photochromic compounds, which absorb different portions of the UV and originate specific absorption bands in the visible region, has allowed extending human vision to the ultraviolet (Gentili et al., 2016).
When the molecular fuzzy sets, which are mixed in the same solution, participate in different chemical reactions and give rise to a chemical web because they share reagents and products, they allow the implementation of chemical fuzzy neural networks. Suppose these webs are recurrent because they include feedback actions. In that case, they become the chemical counterparts of the neuro-fuzzy algorithms that are good at modelling non-linear causal relationships, predicting aperiodic time series, and recognising variable patterns (Gentili and Stano, 2022; Braccini et al., 2023). The feedback actions that can alter the strength of the reciprocal connections confer learning abilities to the chemical network.
Higher cognitive functions can be achieved by hierarchically increasing the chemical fuzzy neural networks’ complexity. In synthetic cells, distinct modules playing different functions can be assembled through their compartmentalization, likewise in living cells (Gentili and Stano, 2023a). One step further towards the hierarchical implementation of chemical artificial intelligence can be taken by designing networks of synthetic cells. Their performances will depend on their network’s architecture, how the artificial cellular nodes communicate, and the adaptability of the reciprocal links. When the edges are strong enough, a sort of swarm or collective intelligence (Couzin, 2007; Watson and Levin, 2023) exhibiting Bayesian inference might emerge. Such powerful webs of synthetic cells might also establish strong connections with living cells and originate the so-called Internet of Bio-Nano Things (IoBNTs; Stano et al., 2023). The hybrid and collective intelligence of the IoBNTs promises to have a plethora of applications (Akyildiz, et al., 2015; Kuscu and Unluturk, 2021), such as diagnosis and therapies for human health and control and cleaning in natural ecosystems or urban areas. In the IoBNTs, even two- (Kagan et al., 2022) or three-dimensional cultures of human brain cells (brain organoids; Smirnova et al., 2023) might be involved. Brain organoids can more easily recapitulate the histoarchitecture and functionality of the fuzzy cortical columns. Therefore, it is reasonable to envisage that such IoBNTs will approach the power of human intelligence more closely to process complex information based on uncertain and context-dependent data, with the extraordinary energetic efficiency of the human brain (Herculano-Houzel, 2012).
4 Conclusive remarks
The potentialities of CAI presented in this article, and the previous one (Gentili and Stano, 2023b) are summarized in Table 1. Moving from Molecular to Supramolecular and finally to Systems Chemistry, the complexity of the problems domain that can be faced apparently increases. Although Chemical AI and robotics are still in their infancy, undoubtedly, it is worth pursuing their development. Among the most impressive achievements of traditional AI and robotics are those pushing robots where humans cannot arrive: the exploration of the marine abysses and the colonization of other planets. Chemical AI and robots can help humans to explore another space poorly investigated so far, i.e., the molecular world. As the same Kurzweil (2014) proclaimed, the “colonization” of the molecular world “will provide tools to effectively combat poverty, clean up the environment, overcome diseases, and extend human longevity.” Massively distributed intelligent chemical robots will greatly expand our memories and sensory and computational abilities. The authors hope these perspectives on Chemical AI and robotics (parts I and II) will spark a productive interdisciplinary collaboration among chemists, biotechnologists, physicists, computer scientists, engineers, neuroscientists, cognitive scientists, philosophers, and biologists. The development of inanimate intelligent chemical systems through a bottom-up approach will have not only remarkable technological repercussions on our societies but will probably unveil that outstanding event that occurred about 4.5 billion years ago, and that was the appearance of life on Earth: a sort of phase transition (Solé et al., 1996; Perez-Mercader, 2004) from an inanimate world devoid of agents to the appearance of the first chemical systems capable of exploiting matter and energy to “handle” information and pursue goals.
Data availability statement
The original contributions presented in the study are included in the article/Supplementary Material, further inquiries can be directed to the corresponding author.
Author contributions
PG: Conceptualization, Funding acquisition, Writing–original draft, Writing–review and editing. PS: Conceptualization, Writing–review and editing.
Funding
The authors declare financial support was received for the research, authorship, and/or publication of this article. This work has been funded by Università degli Studi di Perugia (Fondo Ricerca di Base 2022) and the European Union—NextGenerationEU under the Italian Ministry of University and Research (MUR) National Innovation Ecosystem grant ECS00000041—VITALITY. We acknowledge Università degli Studi di Perugia and MUR for support within the project Vitality.
Acknowledgments
PG acknowledges the Università degli Studi di Perugia for the “Ricerca di Base” fund.
Conflict of interest
The authors declare that the research was conducted in the absence of any commercial or financial relationships that could be construed as a potential conflict of interest.
The authors declared that they were an editorial board member of Frontiers, at the time of submission. This had no impact on the peer review process and the final decision.
Publisher’s note
All claims expressed in this article are solely those of the authors and do not necessarily represent those of their affiliated organizations, or those of the publisher, the editors and the reviewers. Any product that may be evaluated in this article, or claim that may be made by its manufacturer, is not guaranteed or endorsed by the publisher.
References
Adleman, L. M. (1994). Molecular computation of solutions to combinatorial problems. Science 266, 1021–1024. doi:10.1126/science.7973651
Akyildiz, I. F., Pierobon, M., Balasubramaniam, S., and Koucheryavy, Y. (2015). The internet of bio-nano things. IEEE Commun. Mag. 53, 32–40. doi:10.1109/MCOM.2015.7060516
Ashkenasy, G., Hermans, T. M., Otto, S., and Taylor, A. F. (2017). Systems chemistry. Chem. Soc. Rev. 46 (9), 2543–2554. doi:10.1039/C7CS00117G
Braccini, M., Collinson, E., Roli, A., Fellermann, H., and Stano, P. (2023). Recurrent neural networks in synthetic cells: a route to autonomous molecular agents? Front. Bioeng. Biotechnol. 11, 1210334. doi:10.3389/fbioe.2023.1210334
Bray, D. (1995). Protein molecules as computational elements in living cells. Nature 376, 307–312. doi:10.1038/376307a0
Cherry, K. M., and Qian, L. (2018). Scaling up molecular pattern recognition with DNA-based winner-take-all neural networks. Nature 559, 370–376. doi:10.1038/s41586-018-0289-6
Christensen, D. V., Dittmann, R., Linares-Barranco, B., Sebastian, A., Le Gallo, M., Redaelli, A., et al. (2022). 2022 roadmap on neuromorphic computing and engineering. Neuromorphic Comput. Eng. 2 (2), 022501. doi:10.1088/2634-4386/ac4a83
Corentin, D., Carapezzi, S., Abernot, M., Boschetto, G., Azemard, N., Salles, J., et al. (2021). “Oscillatory neural networks for edge ai computing,” in 2021 IEEE computer society annual symposium on VLSI (ISVLSI) (IEEE), 326–331. doi:10.1109/ISVLSI51109.2021.00066
Cox, J. C., Cohen, D. S., and Ellington, A. D. (1999). The complexities of DNA computation. Trends Biotechnol. 17 (4), 151–154. doi:10.1016/S0167-7799(99)01312-8
Csaba, G., and Porod, W. (2020). Coupled oscillators for computing: a review and perspective. Appl. Phys. Rev. 7 (1), 011302. doi:10.1063/1.5120412
Dokholyan, N. V. (2016). Controlling allosteric networks in proteins. Chem. Rev. 116 (11), 6463–6487. doi:10.1021/acs.chemrev.5b00544
Epstein, I. R., and Pojman, J. A. (1998). An introduction to nonlinear chemical dynamics: oscillations, waves, patterns, and chaos. Oxford University Press.
Gallistel, C. R., and King, A. (2009). Memory and the computational brain: why cognitive science will transform neuroscience. New York (USA): Blackwell/Wiley.
Gegenfurtner, K. R. (2003). Cortical mechanisms of colour vision. Nat. Neurosci. 4, 563–572. doi:10.1038/nrn1138
Gentili, P. L. (2021b). Establishing a new link between fuzzy logic, neuroscience, and quantum mechanics through bayesian probability: perspectives in artificial intelligence and unconventional computing. Molecules 26, 5987. doi:10.3390/molecules26195987
Gentili, P. L., Giubila, M. S., Germani, R., and Heron, B. M. (2018). Photochromic and luminescent compounds as artificial neuron models. Dyes Pigments 156, 149–159. doi:10.1016/j.dyepig.2018.04.006
Gentili, P. L., Giubila, M. S., Germani, R., Romani, A., Nicoziani, A., Spalletti, A., et al. (2017). Optical communication among oscillatory reactions and photo-excitable systems: UV and visible radiation can synchronize artificial neuron models. Angew. Chem. Int. Ed. 56 (26), 7535–7540. doi:10.1002/anie.201702289
Gentili, P. L., and Perez-Mercader, J. (2022). Quantitative estimation of chemical microheterogeneity through the determination of fuzzy entropy. Front. Chem. 10, 950769. doi:10.3389/fchem.2022.950769
Gentili, P. L., Rightler, A. L., Heron, B. M., and Gabbutt, C. D. (2016). Extending human perception of electromagnetic radiation to the UV region through biologically inspired photochromic fuzzy logic (BIPFUL) systems. Chem. Comm. 52, 1474–1477. doi:10.1039/C5CC09290F
Gentili, P. L. (2013). Small steps towards the development of chemical artificial intelligent systems. RSC Adv. 3 (48), 25523–25549. doi:10.1039/C3RA44657C
Gentili, P. L., and Stano, P. (2022). Chemical neural networks inside synthetic cells? A proposal for their realization and modeling. Front. Bioeng. Biotechnol. 10, 927110. doi:10.3389/fbioe.2022.927110
Gentili, P. L., and Stano, P. (2023a). Monitoring the advancements in the technology of artificial cells by determining their complexity degree: hints from complex systems descriptors. Front. Bioeng. Biotechnol. 11, 1132546. doi:10.3389/fbioe.2023.1132546
Gentili, P. L., and Stano, P. (2023b). Tracing a new path in the field of AI and robotics: mimicking human intelligence through chemistry. Part I: molecular and supramolecular chemistry. Front. Robot. Ai. 10, 1238492. doi:10.3389/frobt.2023.1238492
Gentili, P. L. (2018). The fuzziness of the molecular world and its perspectives. Molecules 23 (8), 2074. doi:10.3390/molecules23082074
Gentili, P. L. (2014). The human sensory system as a collection of specialized fuzzifiers: a conceptual framework to inspire new artificial intelligent systems computing with words. J. Intell. Fuzzy Syst. 27, 2137–2151. doi:10.3233/IFS-141179
Gentili, P. L. (2021a). Why is Complexity Science valuable for reaching the goals of the UN 2030 Agenda? Rend. Fis. Acc. Lincei 32, 117–134. doi:10.1007/s12210-020-00972-0
Gorecki, J. (2022). Information processing using networks of chemical oscillators. Entropy 24 (8), 1054. doi:10.3390/e24081054
Guindani, C., Silva, L. C., Cao, S., Ivanov, T., and Landfester, K. (2022). Synthetic cells: from simple bio-inspired modules to sophisticated integrated systems. Angew. Chem. Int. Ed. 61, e202110855. doi:10.1002/anie.202110855
Ha, S. D., and Ramanathan, S. (2011). Adaptive oxide electronics: a review. J. Appl. Phys. 110 (7), 14. doi:10.1063/1.3640806
Hagiya, M., Konagaya, A., Kobayashi, S., Saito, H., and Murata, S. (2014). Molecular robots with sensors and intelligence. Acc. Chem. Res. 47 (6), 1681–1690. doi:10.1021/ar400318d
Herculano-Houzel, S. (2009). The human brain in numbers: a linearly scaled-up primate brain. Front. Hum. Neurosci. 3, 31. doi:10.3389/neuro.09.031.2009
Herculano-Houzel, S. (2012). The remarkable, yet not extraordinary, human brain as a scaled-up primate brain and its associated cost. PNAS 109, 10661–10668. doi:10.1073/pnas.1201895109
Hoppensteadt, F. C., and Izhikevich, E. M. (2000). Pattern recognition via synchronization in phase-locked loop neural networks. IEEE Trans. Neural Netw. 11, 734–738. doi:10.1109/72.846744
Kagan, B. J., Kitchen, A. C., Tran, N. T., Habibollahi, F., Khajehnejad, M., Parker, B. J., et al. (2022). In vitro neurons learn and exhibit sentience when embodied in a simulated game-world. Neuron 110 (23), 3952–3969.e8. doi:10.1016/j.neuron.2022.09.001
Kuscu, M., and Unluturk, B. D. (2021). Internet of bio-nano things: a review of applications, enabling technologies and key challenges. ITU J. Future Evol. Technol. 2, 1–24. doi:10.52953/CHBB9821
Luisi, P. L. (2002). Toward the engineering of minimal living cells. Anat. Rec. 268, 208–214. doi:10.1002/ar.10155
Marr, D. V. (2010). A computational investigation into the human representation and processing of visual information. USA): The MIT Press.
Minsky, M. (2006). The emotion machine: commonsense thinking, artificial intelligence, and the future of human mind. New York: Simon & Schuster.
Mountcastle, V. B. (1997). The columnar organization of the neocortex. Brain 120, 701–722. doi:10.1093/brain/120.4.701
Murata, S., Konagaya, A., Kobayashi, S., Saito, H., and Hagiya, M. (2013). Molecular robotics: a new paradigm for artifacts. New Gener. comput. 31, 27–45. doi:10.1007/s00354-012-0121-z
Murata, S., Toyota, T., Nomura, S. I. M., Nakakuki, T., and Kuzuya, A. (2022). Molecular Cybernetics: challenges toward cellular chemical artificial intelligence. Adv. Funct. Mat. 32 (37), 2201866. doi:10.1002/adfm.202201866
Pérez-Mercader, J. (2004). Physical phenomena underlying the origin of life. InLife in the universe. Dordrecht: Springer, 27–51.
Pouget, A., Beck, J. M., Ma, W. J., and Latham, P. E. (2013). Probabilistic brains: knowns and unknowns. Nat. Neurosci. 16, 1170–1178. doi:10.1038/nn.3495
Przyczyna, D., Zawal, P., Mazur, T., Strzelecki, M., Gentili, P. L., and Szaciłowski, K. (2020). In-materio neuromimetic devices: dynamics, information processing and pattern recognition. Jpn. J. Appl. Phys. 59 (5), 050504. doi:10.35848/1347-4065/ab82b0
Qiu, M., Khisamutdinov, E., Zhao, Z., Pan, C., Choi, J. W., Leontis, N. B., et al. (2013). RNA nanotechnology for computer design and in vivo computation. Phil. Trans. R. Soc. A 371, 20120310. doi:10.1098/rsta.2012.0310
Rakic, P. (2008). Confusing cortical columns. Proc. Natl. Acad. Sci. U. S. A. 105, 12099–12100. doi:10.1073/pnas.0807271105
Russell, S., and Norvig, P. (2010). Artificial intelligence. A modern approach. New Jersey (USA): Prentice Hall.
Shapiro, E. (2012). A mechanical Turing machine: blueprint for a biomolecular computer. Interface focus 2 (4), 497–503. doi:10.1098/rsfs.2011.0118
Smirnova, L., Caffo, B. S., Gracias, D. H., Huang, Q., Morales Pantoja, I. E., Tang, B., et al. (2023). Organoid intelligence (OI): the new frontier in biocomputing and intelligence-in-a-dish. Front. Sci. 1, 1017235. doi:10.3389/fsci.2023.1017235
Solé, R. V., Manrubia Cuevas, S., Luque, B., Delgado, J., and Bascompte, J. (1996). Phase transitions and complex systems:Simple, nonlinear models capture complex systems at the edge of chaos. Complexity 1, 13–26. doi:10.1002/cplx.6130010405
Stano, P., Gentili, P. L., Damiano, L., and Magarini, M. (2023). A role for bottom-up synthetic cells in the internet of bio-nano things? Molecules 28 (14), 5564. doi:10.3390/molecules28145564
Turing, A. (1936). On computable numbers, with an application to the entscheidungsproblem. Proc. Lond. Math. Soc. 42, 230–265. doi:10.1112/plms/s2-42.1.230
Varghese, S., Elemans, J. A., Rowan, A. E., and Nolte, R. J. (2015). Molecular computing: paths to chemical Turing machines. Chem. Sci. 6 (11), 6050–6058. doi:10.1039/C5SC02317C
Watson, R., and Levin, M. (2023). The collective intelligence of evolution and development. Collect. Intell. 2 (2), 263391372311683–22. doi:10.1177/26339137231168355
Wells, R. B. (2005). Cortical neurons and circuits: a tutorial introduction. Unpublished work. Available online: https://citeseerx.ist.psu.edu/viewdoc/download?doi=10.1.1.573.3117&rep=rep1&type=pdf (Accessed June 25, 2023).
Zadeh, L. A. (2002). Toward a perception-based theory of probabilistic reasoning with imprecise probabilities. J. Stat. Plan. Inf. 105, 233–264. doi:10.1016/s0378-3758(01)00212-9
Zadeh, L. A. (1997). Toward a theory of fuzzy information granulation and its centrality in human reasoning and fuzzy logic. Fuzzy Sets Syst. 90, 111–127. doi:10.1016/S0165-0114(97)00077-8
Keywords: chemical artificial intelligence, chemical robots, artificial neural networks, proteins, DNA, fuzzy logic, Bayesian inference
Citation: Gentili PL and Stano P (2023) Tracing a new path in the field of AI and robotics: mimicking human intelligence through chemistry. Part II: systems chemistry. Front. Robot. AI 10:1266011. doi: 10.3389/frobt.2023.1266011
Received: 24 July 2023; Accepted: 09 October 2023;
Published: 17 October 2023.
Edited by:
Elio Masciari, University of Naples Federico II, ItalyReviewed by:
Jerzy Gorecki, Polish Academy of Sciences, PolandCopyright © 2023 Gentili and Stano. This is an open-access article distributed under the terms of the Creative Commons Attribution License (CC BY). The use, distribution or reproduction in other forums is permitted, provided the original author(s) and the copyright owner(s) are credited and that the original publication in this journal is cited, in accordance with accepted academic practice. No use, distribution or reproduction is permitted which does not comply with these terms.
*Correspondence: Pier Luigi Gentili, pierluigi.gentili@unipg.it