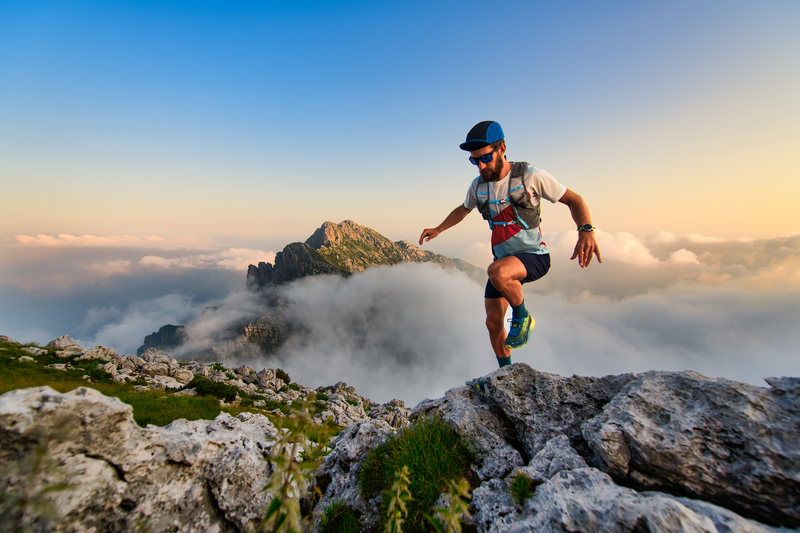
94% of researchers rate our articles as excellent or good
Learn more about the work of our research integrity team to safeguard the quality of each article we publish.
Find out more
CORRECTION article
Front. Robot. AI , 05 May 2021
Sec. Biomedical Robotics
Volume 8 - 2021 | https://doi.org/10.3389/frobt.2021.691570
This article is part of the Research Topic Robotics, Autonomous Systems and AI for Nonurgent/Nonemergent Healthcare Delivery During and After the COVID-19 Pandemic View all 35 articles
This article is a correction to:
Deep Learning-Based Haptic Guidance for Surgical Skills Transfer
A corrigendum on
Deep Learning-Based Haptic Guidance for Surgical Skills Transfer
by Fekri, P., Dargahi, J., and Zadeh, M., (2021). Front. Robot. AI 7:586707. doi:10.3389/frobt.2020.586707
In the original article, there was a mistake in Figure 7 as published. The figure included “Dropout”, which was not used in our reported results but can be applied to the “Dense Layers” before the output layer. Since we have not utilized “Dropout” in the reported results, the corresponding information has been removed from the caption of Figure 7 and a correction has been made to the Evaluation and Discussion section, subsection Result and Discussion, Paragraph 3:
“Configuration 1 had 128 memory size through a one-layer LSTM network. The aim of this setup was mapping the input vector of size nine to an output vector with three elements related to the haptic force feedback prediction. Configuration 2 is the intended architecture for the DHG. This setup reached to the best result in comparison with the others. Figure 7 demonstrates the architecture of the DHG. The prepared data (section 3.1) is fed to an LSTM, which is unrolled over e = 20. Every hidden state of the unrolled unit enters to another LSTM unit in layer 2. In this unit, only the output of the hidden state in time t goes to a dense layer. Since the DHG aims at estimating the forces as a regression problem, the activation function for the dense layer is a linear one.”
The corrected Figure 7 and caption appear below.
FIGURE 7. The diagram shows the intended architecture for the DHG. The input is a tensor containing the data from different sources (section 2.2). The LSTM is unrolled over e previously generated data. The cell state h is the input of its corresponding unrolled unit in the next layer. However, using the latest LSTM unit’s output, the DHG squeezes the prediction vector through a dense layer. Finally, the output of the network is a vector with three elements corresponding to forces in x, y, and z direction.
The authors apologize for this error and state that this does not change the scientific conclusions of the article in any way. The original article has been updated.
Keywords: deep learning, recurrent neural network, LSTM, haptic, force feedback, bone drilling, surgical skill transfer, COVID-19
Citation: Fekri P, Dargahi J and Zadeh M (2021) Corrigendum: Deep Learning-Based Haptic Guidance for Surgical Skills Transfer. Front. Robot. AI 8:691570. doi: 10.3389/frobt.2021.691570
Received: 07 April 2021; Accepted: 12 April 2021;
Published: 05 May 2021.
Edited and reviewed by:
S. Farokh Atashzar, New York University, United StatesCopyright © 2021 Fekri, Dargahi and Zadeh. This is an open-access article distributed under the terms of the Creative Commons Attribution License (CC BY). The use, distribution or reproduction in other forums is permitted, provided the original author(s) and the copyright owner(s) are credited and that the original publication in this journal is cited, in accordance with accepted academic practice. No use, distribution or reproduction is permitted which does not comply with these terms.
*Correspondence: Pedram Fekri, cF9mZWtyaUBlbmNzLmNvbmNvcmRpYS5jYQ==
Disclaimer: All claims expressed in this article are solely those of the authors and do not necessarily represent those of their affiliated organizations, or those of the publisher, the editors and the reviewers. Any product that may be evaluated in this article or claim that may be made by its manufacturer is not guaranteed or endorsed by the publisher.
Research integrity at Frontiers
Learn more about the work of our research integrity team to safeguard the quality of each article we publish.