- 1Department of Industrial Engineering and Management, Ben-Gurion University of the Negev, Beer-Sheva, Israel
- 2Department of Biomedical Engineering, Ben-Gurion University of the Negev, Beer-Sheva, Israel
- 3Zlotowski Center for Neuroscience, Ben-Gurion University of the Negev, Beer-Sheva, Israel
- 4Department of Psychology, Ben-Gurion University of the Negev, Beer-Sheva, Israel
Weber’s law is among the basic psychophysical laws of human perception. It determines that human sensitivity to change along a physical dimension, the just noticeable difference (JND), is linearly related to stimulus intensity. Conversely, in direct (natural), visually guided grasping, Weber’s law is violated and the JND does not depend on stimulus intensity. The current work examines adherence to Weber’s law in telerobotic grasping. In direct grasping, perception and action are synchronized during task performance. Conversely, in telerobotic control, there is an inherent spatial and temporal separation between perception and action. The understanding of perception–action association in such conditions may facilitate development of objective measures for telerobotic systems and contribute to improved interface design. Moreover, telerobotic systems offer a unique platform for examining underlying causes for the violation of Weber’s law during direct grasping. We examined whether, like direct grasping, telerobotic grasping with transmission delays violates Weber’s law. To this end, we examined perceptual assessment, grasp control, and grasp demonstration, using a telerobotic system with time delays in two spatial orientations: alongside and facing the robot. The examination framework was adapted to telerobotics from the framework used for examining Weber’s law in direct grasping. The variability of final grip apertures (FGAs) in perceptual assessment increased with object size in adherence with Weber’s law. Similarly, the variability of maximal grip apertures in grasp demonstration approached significance in adherence with Weber’s law. In grasp control, the variability of maximal grip apertures did not increase with object size, which seems to violate Weber’s law. However, unlike in direct grasping, motion trajectories were prolonged and fragmented, and included an atypical waiting period prior to finger closure. Therefore, in this condition, maximal grip aperture was an inappropriate indicator of JND. Instead, we calculated the aperture at the end of the opening phase, the initial grip aperture (IGA), and the FGA at the beginning of the waiting period, as more appropriate indicators for the JNDs. The IGAs adhered to Weber’s law. The FGAs approached significance in the same direction. This suggests that perception–action association during telerobotic grasping with transmission delays significantly diverges from direct grasping.
Introduction
Through the years, psychophysical research has uncovered several laws governing human perception–action integration. Among these is Weber’s law, which is considered a basic psychophysical principle of human perception (Baird and Noma, 1978). According to Weber’s law, the perceptual sensitivity, largely across all sensory modalities, to a change along a physical dimension is linearly related to the intensity of the stimulus, i.e., the just noticeable difference (JND) is larger for stronger stimuli. This finding was supported by numerous experiments. In striking contrast, visually guided, direct (natural) grasping violates Weber’s law.
When remotely controlling a robotic manipulator, the human operator is required to integrate cognition, perception, and action while accounting for the spatial separation of the local and remote sites. In such setups, the human operator controls a manipulator that differs in structure and dynamics from the operator’s limbs, sensory perceptions are limited and biased, and there are inherent transmission delays. These characteristics make both design and operation of telerobotic systems, challenging. Grasping is fundamental in most telerobotic tasks. It is especially challenging, as contact must be made between the robotic end-effector and the object to be grasped, which requires high spatiotemporal perception–action integration. It is, thus, important to study adherence to Weber’s law in the context of telerobotic grasping, as indication of the underlying internal processing mechanisms employed by the user. Additionally, the inherent spatial and temporal separation between perception and action in telerobotic systems offers a unique platform for examining underlying causes for the violation of Weber’s law in grasping.
It is common in psychophysics to use explicit methods to measure JNDs, such as the method of constant stimuli, i.e., extracting JND values from the psychophysical function. However, for the purpose of measuring JNDs during motor control, it is necessary to use a different method that directly taps onto movement trajectories. For this purpose, we and others (Ganel et al., 2008) use the classical method of adjustment. According to this method, the variance of the responses to a stimulus reflects an “area of uncertainty,” which is a measure of the JND for that stimulus. The use of the method of adjustment for measuring JNDs has not been limited to grasping or to motor control per se. Indeed, this method has been used for many years across different perceptual domains, such as time and auditory perception [for discussion, see Ganel et al. (2014)].
In grasping, the JND is measured as the within-participant variability of the maximal finger aperture during the reach-to-grasp movement and it remains invariant with object size, in violation of Weber’s law (Ganel et al., 2008). Several experimentally confirmed hypotheses have been suggested for explaining this phenomenon (Smeets and Brenner, 2008; Jazi et al., 2015; Löwenkamp et al., 2015; Utz et al., 2015; Jazi and Heath, 2016). The different perspectives from which these hypotheses have emerged have not been resolved thus far. However, it is commonly assumed that the immunity of the visuomotor system to Weber’s law reflects an absolute processing style during grasping, which is in sharp contrast to the relative processing style of the human perceptual system (Ganel and Goodale, 2003; Ganel et al., 2008; Jazi and Heath, 2016).
Perceptual–motor transparency is a major concern in telerobotic system interfaces as it determines system fidelity and usability (Preusche and Hirzinger, 2007; Nisky et al., 2013). It was analyzed extensively based on the characteristics of the communication channel. A three-layered human-centered measure of transparency was suggested, where the layers include perceptual transparency, local motor transparency, and remote transparency (Nisky et al., 2013). Perceptual transparency is assessed by quantifying perceptual bias and discrimination thresholds in the mechanical properties of the environment. Local (remote) motor transparency is assessed through comparison of human (remote manipulator) motion trajectories while teleoperating the robot, to those that would be executed if the operation was performed directly on the remote environment. Yet, even these measures are of external operation parameters, such as motion trajectories, and cannot ascertain internal processing similarity within the central nervous system (CNS). Indeed, only systems that can elicit such a degree of transparency, can be considered truly transparent and facilitate very high fidelity and usability. Similarity of the internal processing in natural (direct) and in telerobotic environments can be assessed only by uncovering the underlying mechanisms determining human perception–action operation during natural, direct motion and during telerobotic control. Such analysis is expected to improve the understanding of human operation, facilitate the development of objective measures for quantifying transparency, and lead to design of efficient telerobotic interfaces.
The Underlying Causes of Weber’s Law
For assessing the implications of violation or adherence to Weber’s law in telerobotic grasping, it is important to understand the underlying perception and action processing mechanisms. Hypotheses explaining the lack of Weber’s law in grasping vary considerably in their postulations regarding these mechanisms. These hypotheses relate to visual or haptic sensory perceptions, motion planning processes, and biomechanical constraints during motion execution. In the following, we detail the major hypotheses.
The violation of Weber’s law in grasping may stem from the functional separation of visual information processing. In the neuroscience literature, it is well established that, perception and action are mediated by separated neural networks. The two visual systems hypothesis proposed by Goodale and Milner (1992) provides a contemporary example for such an account that details the organization of the primate visual system. According to this proposal, the ventral “perception” pathway provides the rich and detailed visual representation of the world, and the dorsal “action” pathway enables flexible control of actions directed to objects. This proposal of a functional separation between visual systems underlying action and perception is supported by converging evidence from neuropsychological patient data and behavioral psychophysics. Behavioral studies provide evidence that, unlike visual perception, which is largely governed by relational and Gestalt representations of objects’ size and shape, visually guided action treats objects in a more analytic fashion. In-line with this view, the lack of adherence to Weber’s law in visually guided, direct grasping, results mainly from the functional separation between the visual systems (Ganel et al., 2008). This view is consistent with many other examples of dissociations between perception and action, such as in the case of the size–weight illusion (Flanagan and Beltzner, 2000) or the effect of delayed force information on perception of impedance and grip force adjustment (Leib et al., 2015).
An alternative account for the violation phenomena is based on the role of haptic feedback, as grasping a physical object involves haptic cues when the fingers touch the target object. Thus, integration of haptic and visual cues may be imperative for absolute specification of object size leading to the violation of Weber’s law in grasping. Moreover, the lack of haptic feedback may be the cause of Weber’s law appearing in pantomime grasping (Jazi et al., 2015; Jazi and Heath, 2016). Ozana and Ganel (2017) examined adherence to Weber’s law in direct grasping of physical objects placed beyond a transparent glass surface. They found that Weber’s law was maintained when subjects were instructed to end the motion close to the glass surface, but without touching it, and conversely, that Weber’s law was violated when subjects were instructed to touch the surface at the end of the motion. Their findings suggest that even indirect haptic information is sufficient to allow analytic processing during grasp.
Another alternative explanation of the violation of Weber’s law in grasping stems from motion planning mechanisms. Most contemporary research of reach-to-grasp motion asserts that it is comprised of two separately controlled, yet coordinated, functional components, the reaching motion bringing the hand toward the object, and the grasp formation shaping the hand according to object features (Jeannerod, 1981; Jeannerod et al., 1995). In contrast, Smeets and Brenner(1999, 2001) claim reach-to-grasp motion should be viewed as a coordination of separate finger motion plans. They suggest that reach-to-grasp motion planning is based on reaching with the finger to a position on the object rather than on the object’s size (Smeets and Brenner, 2008), and therefore, finger aperture during reach-to-grasp movements does not reflect the computation of size and is not expected to adhere to Weber’s law.
Finally, the violation of Weber’s law in grasping may be attributed to biomechanical constrains effecting motion execution rather than to planning or perceptual processing (Löwenkamp et al., 2015; Utz et al., 2015). Ceiling effects caused by the limited human finger span and human tendency to avoid large and uncomfortable apertures, can suppress variation in large finger apertures precluding the manifestation of Weber’s law. We note, however, that recent research has shown that the dissociation between perception and action in terms of their adherence to Weber’s law persists even when the possibility of biomechanical constrains are accounted for (Ganel et al., 2017; Heath and Manzone, 2017; Heath et al., 2017; Manzone et al., 2017).
The Effects of Transmission Delays
In natural grasping, reach-to-grasp motion profiles comprise two components, arm motion for moving the hand toward the object, and hand (finger) motion for grip formation (Lacquaniti and Soechting, 1982; Jeannerod, 1984; Marteniuk et al., 1990; Wallace et al., 1990; Santello et al., 2002). Arm motion profiles follow a stereotypical human motion path based on minimum jerk optimization (Flash and Hogan, 1985) and adhere to Fitts’ law for various object types and sizes (Crossman and Goodeve, 1983). Fitts’ law, among the basic psychophysical laws related to movement control, models the speed-accuracy tradeoff of human motion. It determines that reaching motion time is a logarithmic function of the ratio between the distance and the width of the target (Fitts, 1954). Grip formation has two stages, opening (finger stretching) and closing (closing fingers toward contact with the object). The formation of the finger grip occurs during arm motion (hand transportation), where maximum arm endpoint (wrist) velocity is typically reached in parallel to maximal aperture (Jeannerod, 1984; Marteniuk et al., 1990; Rand et al., 2000).
In teleoperation, transmission delays between control movements and feedback from the remote system response are inevitable, especially when the distances between the human operator and the controlled robotic device are long. The effects of such delays on operator performance have been extensively studied (Rohde and Ernst, 2016). It was shown that a modified form of Fitts’ law modeling a multiplicative relationship between movement time, an index of difficulty, and transmission delays, provides an accurate predictor of the experimental data (Hoffmann, 1992). Visuomotor delays increase errors in driving (Cunningham et al., 2001) distort drawing and writing (Kalmus et al., 1955; Morikiyo and Matsushima, 1990) and impede motor adaptation (Honda et al., 2012a,b). Moreover, a consistent exposure to delay eventually leads to adaptation (Foulkes and Miall, 2000; de la Malla et al., 2014; Farshchiansadegh et al., 2015; Rohde et al., 2014; Avraham et al., 2017; Leib et al., 2017), and aftereffects are evident upon delay removal (Smith and Bowen, 1980; Botzer and Karniel, 2013; Avraham et al., 2017). A delayed visual feedback also affects weight perception, with participants’ reports of an increased mass (Honda et al., 2013) or resistance (Takamuku and Gomi, 2015) in the presence of delay. Similarly, delayed force feedback biases perceived stiffness of elastic objects (Leib et al., 2015, 2016) where the effects of delay on actions with elastic objects are often different from their effects on perception (Nisky et al., 2011; Leib et al., 2016).
For long delays (above about 0.7 s), a change in control strategy was also found, from a more continuous form of control to a move-and-wait strategy. Experimental data in both long and short delays fit the modified Fitts’ model predictions with different coefficients (Sheridan and Ferrell, 1963; Ferrell, 1965). It was additionally shown that when participants were asked to track the motion of a visual bar with their hand, they were able to adjust to motion displacement only when there were no transmission delays. When the delays were longer than 0.3 s, participants were unable to adjust to their motion displacements, leading the authors to conclude that for such delays the correlation between visual feedback and motor control commands is disrupted (Held et al., 1966).
The amalgamation of these findings make the region of 0.3–0.7 s delays, where motion is continuous yet perception–action synchronization is disrupted, particularly interesting for analyzing telerobotic control. In the current work, we examined Weber’s law in a telerobotic control scenario with such transmission delays. We sought to determine if indeed participant behavior during telerobotic control with such delays would adhere to Weber’s law. We hypothesized that, as in direct conditions, in telerobotic perceptual-based tasks, participant behavior would adhere to Weber’s law. Indeed, establishing adherence to Weber’s law during perceptual assessment is crucial for establishing testbed validity. We further hypothesized that, when perceptual transformations are required during telerobotic control, e.g., when viewing and action directions are not aligned, participant behavior would also adhere to Weber’s law. To this end, we developed a telerobotic environment with transmission delays and telerobotic versions of direct tasks used for examining Weber’s law. A different study from our lab examined Weber’s law in a surgical robotic setup with negligible transmission delays (Milstein et al., submitted).1
Materials and Methods
Participants
Sixty-three healthy, right-handed participants (age 18–31 years, mean 24.3, 30 males) participated in the experiment. Participants had normal or corrected-to-normal vision with no neurological, sensorimotor, or orthopedic impairments. To avoid fatigue, the participants were divided into six groups (two perceptual assessment groups with 10 participants each, two grasp control groups with 11 participants each, and two grasp demonstration groups with 10 and 11 participants), where each group performed one of the experimental procedures described below. According to the requirements of the Helsinki declaration, the Human Subject Research Committee of Ben-Gurion University of the Negev approved the experimental protocol.
Apparatus
A unilateral telerobotic system (without force feedback to the user) was constructed based on a Motoman UP6 robotic manipulator (Yaskawa, Japan), a controlled jaw gripper, AVG 55 (Schunk, Germany), and a pair of Phantom Premium devices fitted with finger thimbles (Geomagic, USA). The human finger aperture determined the robotic gripper opening (without scaling) and the center of the human finger aperture determined the tool center point (TCP) position (with a 1:2.2 scaling). To simplify the task, robot motion toward (and away from) the object was possible only along a straight horizontal line (forward and backward). Similarly, lifting and placing the object back on the table were also possible only along the vertical axis.
To support robustness and modularity, the system was developed as a distributed system with each hardware component constituting a separate agent. The control was implemented in a data-driven approach, where communication between the components was established over the internet. The communication apparatus was developed using the data distribution service (RTI, USA). The data transmission rate from the Phantom devices was set to 100 Hz and the control cycle delays of the robot and gripper were 0.6 and 0.3 s, respectively. The delays were determined based on hardware constraints and preliminary examination of system operation. The system’s transmission delays are determined by the control cycle delays. For such delays motion is expected to be continuous yet perception–action synchronization is disrupted.
Five cylinders with different diameters ranging from 20 to 40 mm in 5-mm steps (XS, S, M, L, XL) were used in the experiments (Figure 1D). A small table was placed in front of the robot inside the robot’s work-volume for placing the cylinder to be grasped. A single cylinder was placed on the table for each experimental run.
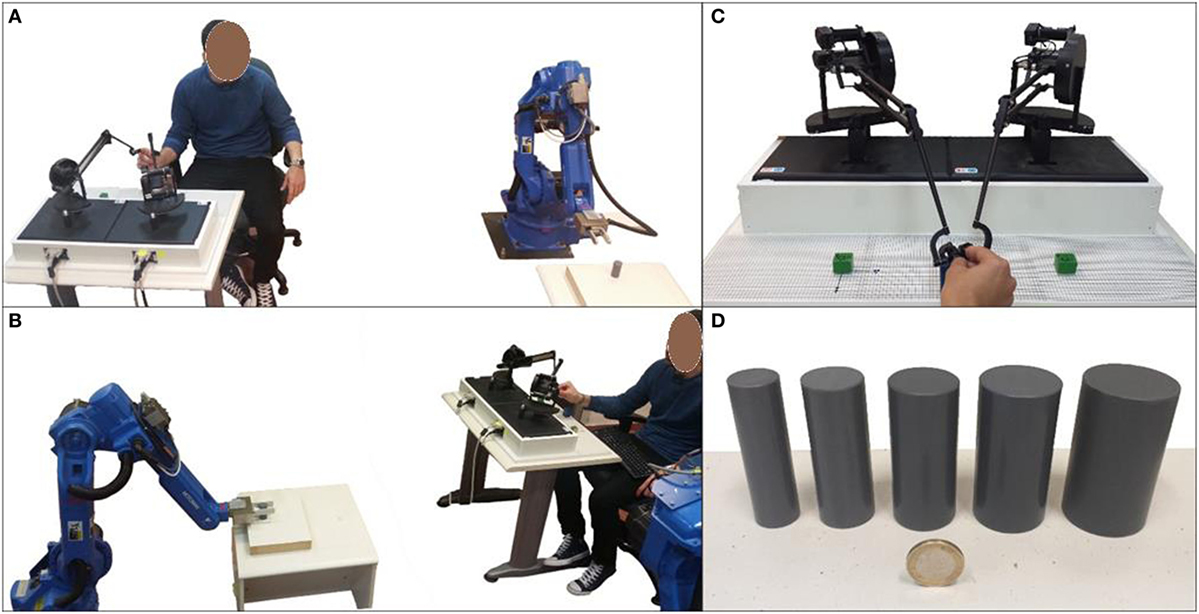
Figure 1. Experimental setup. (A) Alongside, (B) across, (C) initial position, (D) tested cylinders. Written and informed consent has been obtained from the depicted individual for the publication of his identifiable image.
Experimental Procedure
The experiment comprised three tasks: perceptual assessment, grasp control, and grasp demonstration. The tasks were constructed as a telerobotic version of classical (direct) visual perception, visually guided grasping, and pantomimed grasping tasks typically used for assessing Weber’s law (Ganel et al., 2008; Smeets and Brenner, 2008; Jazi et al., 2015; Löwenkamp et al., 2015; Utz et al., 2015; Jazi and Heath, 2016). In the grasp control task, the subject is required to grasp the object. In the visual perception task, the subject indicates her perception of the size of the object with finger aperture. So this task includes finger motion, though not a grasping movement. In pantomimed grasping, the subject pantomimes a grasping motion, so the task includes grasping motion, but not toward a physical object.
Each task was conducted in two orientations of the operator with respect to the robot: alongside (Figure 1A) and across (Figure 1B). These two orientations were selected because they provide different control directions and viewing conditions. When alongside each other the robot and operator have aligned control directions, as would be the case in direct grasping (when the participant grasps the object using his/her own hand), yet unlike direct grasping, the view of the grasp contact point on the object of the remote robotic finger is obscured. When facing each other in the across orientation the movement directions of the operator and robot are mirrored, i.e., different from direct grasping conditions, yet grasp contact points on the object of both fingers are clearly visible. In both orientations, the participants sat outside the robotic work-volume, about 2 m away from the robot base. Two pseudorandom sets of object order were prepared. In each condition (task and orientation combination), the participants were equally divided and performed the experiment according to one of the two sets. In all tasks at the beginning of each trial, participants placed their fingers at the initial position (Figure 1C) with their eyes closed, waiting for a computerized audio cue to open their eyes and start moving. Then, they performed the task, paused, and then returned to the initial position. Transitions between the stages were marked by a computerized audio cue. Participants performed the trials for each of the five objects and were allowed to rest at will twice during the experiment. Before starting the experiment, participants practiced the task for a few repetitions until they reported feeling comfortable in performing it.
In the perceptual assessment task, participants were asked to indicate the cylinder’s width by opening the gripper to an equivalent aperture during a 5.1-s time window. In this task, only the gripper opening was controlled by the participants, and the robot manipulator did not move. The choice of such pantomimed reporting of a perceptual assessment is consistent with prior studies of perception–action dissociations in their adherence to Weber’s law. It is important for making sure that the perceptual assessment and the grasp control are performed using similar finger motion and, therefore, attributing any differences in violation or adherence to Weber’s law to the underlying neural processing. The adaptation to the telerobotic environment is in that the aperture of the robotic figures, rather than the aperture of the participant’s fingers, is the object size indicator. Participants performed 20 trials for each of the five objects (100 trials overall).
In the grasp control task, participants were requested to teleoperate the robot, and to use it to grasp and lift the object in three consecutive stages, pausing between stages until they received a computerized audio command to continue. The stages were reach and grasp the object (during a 7.2-s time window), raise the object and place it back on the table (during a time window of 3.9 s), and release the object and return to the initial position. Participants performed 20 trials for each of the five objects (100 trials overall).
In the grasp demonstration task, there were two experimental stages. In the first stage, participants practiced remotely controlling the robot with the Phantom interface. They remotely grasped and lifted a cylinder placed on the table using the robotic system (as was performed in the grasp control task). This was done for several minutes until they reported feeling comfortable with the task. This stage was introduced to assure that the participants attain an understanding of the robotic task and appreciate the capabilities of the robotic system. In the second stage, participants were asked to demonstrate reach-to-grasp motion to the robot (in a 4.2-s time window), while their fingers were placed in the Phantom thimbles, just as in the grasp control stage. During the demonstrations the robot or gripper did not move. The adaptation of the pantomime task to the telerobotic demonstration has three components: acquainting the participants with the capabilities of the robotic system; the use of the Phantom interface, and requesting the participants to demonstrate the task to the robot, which is important for placing their actions in context of the robotic operation, rather than their own direct operation. Participants performed 15 demonstration trials for each of the five objects (75 trials overall).
Data Analysis
Motion trajectories were recorded at 100 Hz and were filtered using a standard two-way, low-pass Butterworth filter (n = 3) with a 5.54-Hz cutoff (verified against the data). For the assessment task (Figure 2B), Maximal aperture speed was determined over all the movement. Movement start was determined as the time at which the aperture speed exceeded and remained above 10% of the maximal aperture speed, for 0.1 s. To ensure inclusion of final motion corrections movement end was similarly determined as the time at which the aperture speed decreased and remained below 10% of the maximal aperture speed, for 0.5 s. For the grasp control and grasp demonstration tasks (Figure 2A), only the reach-to-grasp phase was analyzed. Two maximal speeds were determined, maximal opening speed, during the first part of the movement in which finger aperture increased, and maximal closing speed during the final part of the movement (after reaching maximal aperture) in which finger aperture decreased. Movement start was determined as the time at which the aperture speed exceeded and remained above 10% of the maximal aperture opening speed, for 0.1 s. The end of the aperture opening phase was determined as the time at which the aperture opening speed decreased and remained below 10% of the maximal aperture opening speed, for 0.1 s. The start of the aperture closing phase was determined as the time at which the aperture closing speed increased and remained above 10% of the maximal aperture closing speed, for 0.1 s. Movement end was determined as the time at which the aperture closing speed decreased and remained below 10% of the maximal aperture closing speed, for 0.1 s.
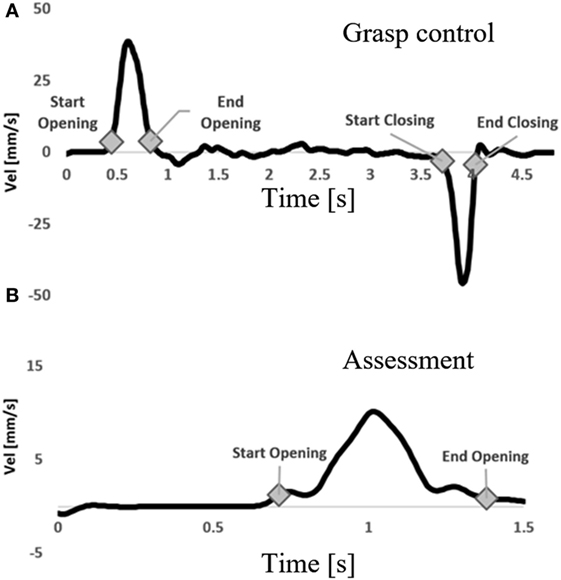
Figure 2. Aperture velocity. (A) Grasp control task, start and end of aperture opening and closing marked. (B) Assessment task, start and end of aperture opening marked.
Two measures were defined for all tasks and additional four measures were defined for the grasp control and grasp demonstrations tasks. For all tasks, movement time was computed as the time difference between movement start and end. The mean aperture opening speed was computed as the mean speed during the aperture opening time. For the grasp control and grasp demonstration tasks, aperture opening time ratio (OTR) was computed as the time between movement start and the end of the aperture opening divided by movement time. Aperture transport time ratio (TTR) was computed as the time between the end of the aperture opening and the beginning of the aperture closing divided by movement time. The final waiting time (FWT) was computed as the time between the end of both aperture opening and forward TCP motion, and the beginning of aperture closing. When aperture closing started prior to the end of the forward TCP motion, the FWT was set as 0, i.e., the FWT is a non-negative measure. The scaled sagittal TCP transport distance (STCPD) was calculated as the difference between the TCP position at movement start and end, multiplied by the robot movement scaling-factor (which was 1:2.2 in the experimental apparatus).
For the assessment task, the final grip aperture (FGA) was computed as the aperture at the end of the movement. For the grasp control and grasp demonstration tasks the maximum grip aperture (MGA) was computed over all the aperture motion. For the grasp control task the initial grip aperture (IGA), and the FGA were also calculated. IGA was determined as the aperture after the end of the opening phase, when aperture speed additionally decreased to 3.3% of the global mean maximum aperture opening speed where, the global mean maximum aperture opening speed was computed over all the movements of all the subjects who performed the grasp control task. This value was chosen to ensure the aperture was sampled after the end of the opening phase in a speed that is not related to object size. This is important for verifying that aperture variability is not affected by aperture velocity, which may lead to an indirect dependence on object size (Ganel et al., 2014; Ganel, 2015). FGA for the grasp control task was determined as the aperture at the end of both aperture opening and the forward TCP motion (when aperture closing had not yet started), i.e., the aperture at the beginning of the FWT.
Statistical Analysis
Failure in the task was defined as failure to complete the task within the designated time window or, additionally in the grasp control task, if the robot collided with the object. Participants were excluded from the analysis if more than 10% of their movements resulted in failure. Data distribution was symmetrical, and therefore, outliers were determined for each remaining participant using the interquartile range of the MGA.
A mixed model ANOVA analysis was conducted for movement time with task (assessment, control, demonstration) and orientation (alongside, across) as between-subjects independent factors, and movement set, as the within-subject independent factor. A similar analysis was conducted separately for each task with orientation as the between-subjects independent factor, and movement set, as the within-subject independent factor for all other measurers (movement time, aperture OTR, aperture TTR, FWT, and STCPD) except for mean aperture opening speed. Mean aperture opening speed was analyzed for each task with orientation as the between-subjects independent factor, and movement set and object size as within-subject independent factors. A confidence interval was determined for the mean STCPD for facilitating comparison to the physically required distance. A linear trend analysis was conducted for the mean and SD of FGA, MGA, and IGA for each task. The analysis of the mean was conducted to verify that the participants were sensitive to object size. Similar to other experiments for assessing Weber’s law in grasping, participants were excluded from the analysis when linear trend analysis of the mean did not show a significant linear trend, i.e., analysis showed they were not sensitive to object size. For the perceptual assessment task, the analysis was based on FGA, for grasp control and grasp demonstration the analysis was based on MGA. The analysis of the SD was conducted to test for the adherence to Weber’s law. The coefficients used for the linear components of the trend analysis were: −2, −1, 0, 1, 2 for object sizes XS, S, M, L, XL, respectively. These are the coefficients commonly used for linear trend analysis for a set of size five.
Results
Two participants in the grasp control task were excluded from the analysis as they had many failures and outliers (one alongside 14%, one across 12%). All participants in the perceptual assessment and the grasp demonstration group succeeded in completing the task. Six additional participants (perceptual assessment: one alongside and one across; grasp control: one across; grasp demonstration: one alongside and two across) were excluded from the analysis as statistical analysis showed they were not sensitive to object size (they did not show a linear relationship for MGA or FGA as a function of object size, with a significance threshold of 0.05) and, therefore, they had failed to comply with the experimental task. For the remaining participants (perceptual assessment: nine alongside, nine across, grasp control: 10 alongside, nine across, grasp demonstration: 10 alongside, eight across), failure and outlier ratio ranged between 0 and 7%, with a mean ratio of 1.6% and they performed all tasks without major retractions (Figures 3 and 4).
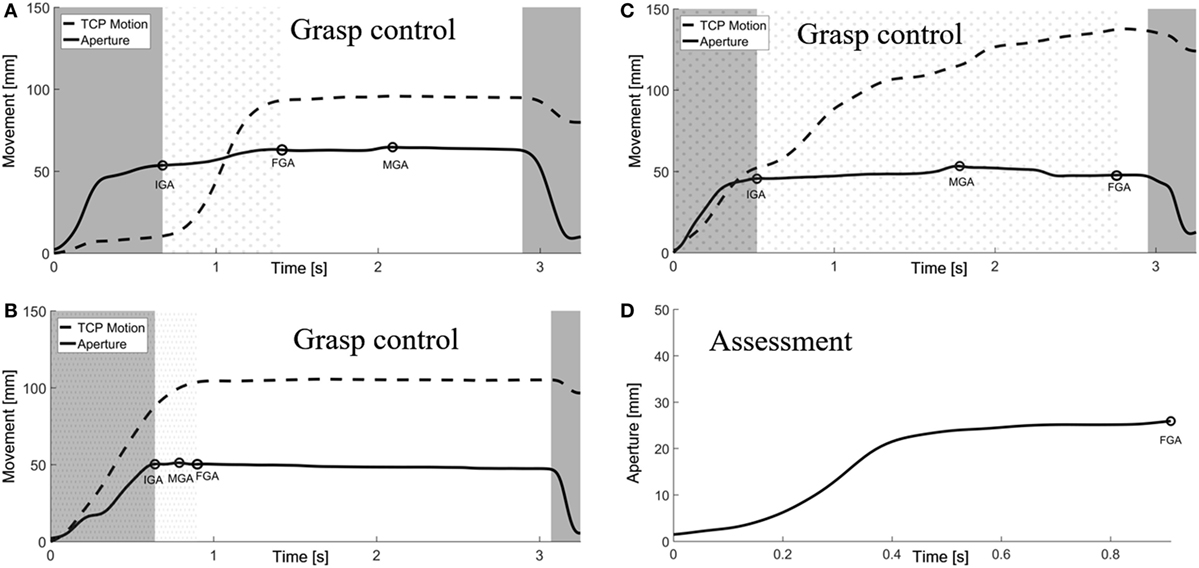
Figure 3. Perceptual assessment and grasp control: representative motion profiles for tool center point motion (TCP) toward the object (dotted line) and for the grip aperture (full line). (A) Grasp control, across, object L (Participant 1). (B) Grasp control, alongside, object L (Participant 2). (C) Grasp control, alongside, object XL (Participant 3). (D) Assessment, across, orientation object M (Participant 4). For grasp control movements gray background marks aperture opening and closing durations. Dotted background marks motion forward duration.
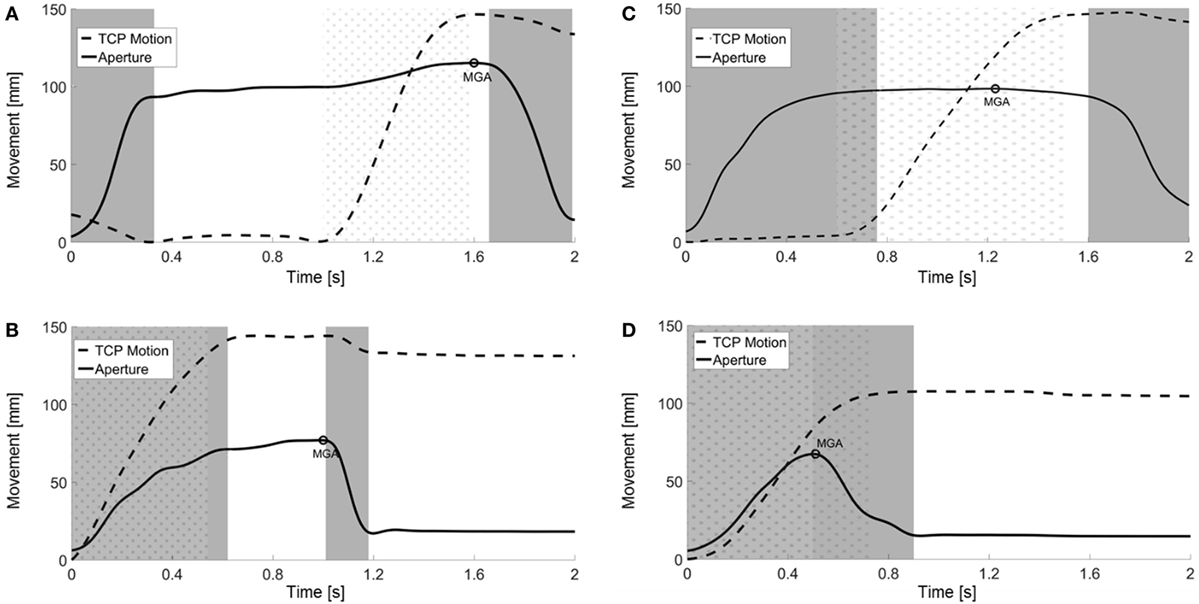
Figure 4. Grasp demonstration: representative motion profiles for tool center point motion (TCP) toward the object (dotted line) and for the grip aperture (full line). (A) Alongside, object L (Subject 1). (B) Across, object XL (Subject 2). (C) Across, object M (Subject 3). (D) Alongside, object L (Subject 4). Gray background marks aperture opening and closing epochs. Dotted background marks TCP motion epochs.
Statistical tests showed that all measures in each task had similar values for both orientations and both movement sets; therefore, all subsequent analysis of the data from each task was conducted jointly for participants from both orientations and both movement sets. Mean values and SD for each task for movement time, aperture OTR, aperture TTR, FWT, STCPD, and mean aperture opening speed are presented in Table 1.
The mean movement time significantly differed between tasks [F(2,52) = 197.5, p < 0.0001]. Mean movement time for grasp control was longer (3.67 s) then grasp demonstration (1.45 s), and perceptual assessment (0.77 s). Mean aperture opening speed for grasp control and grasp demonstration was significantly larger for wider cylinders [grasp control p < 0.001, F(4,1852) = 18.78; grasp demonstration p < 0.001, F(4,1321) = 74.32].
Taking the length of the gripper’s fingers into account (90 mm finger length), the physical distance the robot has to transverse for performing the grasp successfully is 180–270 mm. That is, when the object is grasped at the tip of the gripper’s fingers, the required travel distance is 180 mm and when it is grasped near the wrist, the distance is 270 mm. For grasp control the 95% confidence interval of STCPD was 231.9–236.0 mm and for grasp demonstration, it was 271.7–280.6 mm.
Aperture motion in the grasp control task was fragmented and had a clear opening stage, a transport stage in which participants kept their fingers open, and finally a short closing stage. The forward motion of the TCP started with the aperture opening (Figures 3B,C) or after the end of the opening phase (Figure 3A). Almost all of the trials (98.93%, 1,855 of the trials) included a significant FWT (mean 1.74 s), at the end of the transport stage, after the end of the aperture opening and TCP forward movement, and before aperture closing. MGAs were found in various time points along the transport stage (Figure 3).
In the grasp demonstration task, grip formation had three stages: opening, transport, and closing in only 20% of the movements (263 trials) (Figures 4A,B). Other movement trials had either two stages, where the transport occurred simultaneously with the opening (35%, 469 trials) or the closing (8%, 110 trials) of the fingers (Figure 4C), or one stage where the transport occurred simultaneously with the finger opening and closing movements (36%, 483 trials) (Figure 4D). Many of the movements (52.67%, 710 of the trials) had a distinguishable waiting time, yet waiting time (mean 0.11 s) was significantly smaller than for grasp control [p < 0.0001, F(1,35) = 211].
During grasp control, for all objects, mean MGAs were larger than mean FGAs which in turn, were larger than mean IGAs [p < 0.0001, F(2,5592) = 312.8] (Figure 5). The mean MGAs for grasp control were smaller than the mean MGAs for grasp demonstration [p < 0.001, F(1,35) = 15.188], and mean IGAs for grasp control were larger than mean FGAs for perceptual assessment [p < 0.0001, F(1,35) = 31.34].
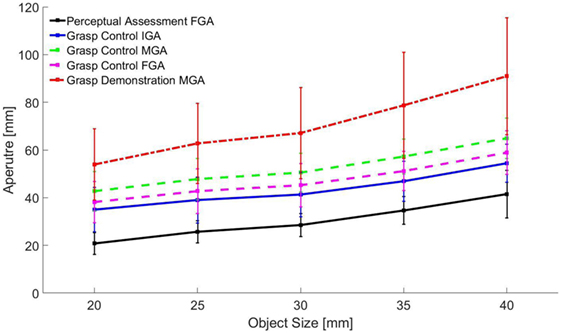
Figure 5. Mean of means (SD marked by crosshair) for assessment final grip aperture (FGA), grasp control initial grip aperture (IGA), FGA, and maximum grip aperture (MGA), and grasp demonstration MGA.
For grasp assessment, the SD of FGAs increased linearly with object size [p < 0.01, F(1,84) = 9.08] (Figure 6A). For grasp control, the SD of IGAs increased linearly with object size [p < 0.05, F(1,89) = 4.26], and the SD of FGAs had an approaching significance linear trend with object size [p = 0.08, F(1,89) = 3.16], but the SD of MGAs did not change with object size (Figures 6C,D). For grasp demonstration, the SD of MGAs had an approaching significance linear trend with object size [p = 0.09, F(1,84) = 2.93] (Figure 6B).
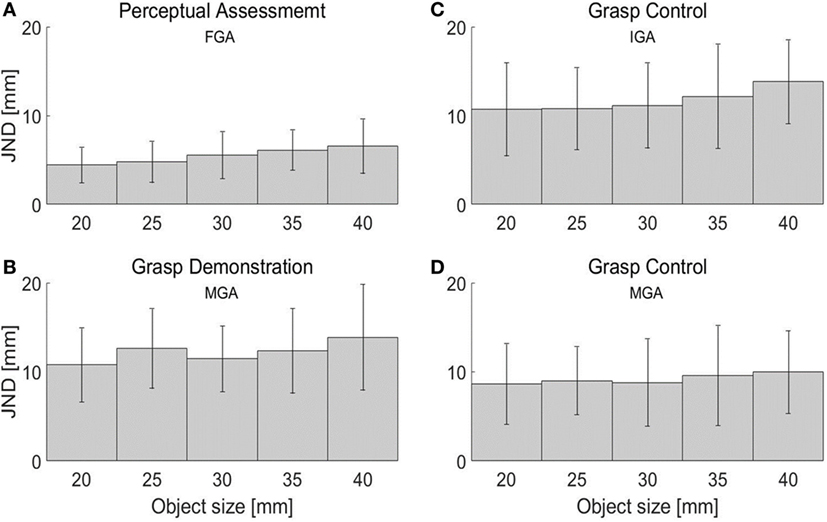
Figure 6. Mean of SD marked by crosshair. (A) final grip aperture (FGA), perceptual assessment. (B) maximum grip aperture (MGA), grasp demonstration. (C) initial grip aperture (IGA), grasp control. (D) MGA, grasp control.
Discussion
As in direct conditions (Ganel et al., 2008), the motion of participants in the perceptual assessment task adhered to Weber’s law, i.e., the JND, as measured by the SD of FGA, increased linearly with the size of the object. Similar to pantomime-grasping conditions (Jazi et al., 2015; Jazi and Heath, 2016), the motion of participants during the grasp demonstration task adhered to Weber’s law, i.e., the JND, as measured by the SD of MGA, approached significance for the linear increase with the size of the object. The SD of MGA in the grasp control task did not linearly increase with the size of the object. Yet, movement fragmentation and the prolonged aperture transport stage in the grasp control task have most likely influenced MGA, and therefore, reduced the validity of the SD of MGA as an indicator for the JND. Accordingly, we argue that the lack of the linear trend for the MGA in the grasp control task cannot be considered as reliable indicator for the violation of Weber’s law. In contrast, IGA, the grip aperture after the opening stage, and FGA, the grip aperture after the end of both the opening and forward motion, are less influenced by fatigue and random movement fluctuations, and thus their SD provide a better indicator for JNDs. Our results indicate that the SD of IGA increased linearly with the size of the object and the SD of FGA approached significance for the linear increase with the size of the object. Therefore, the results of the current study show, for the first time that, unlike direct grasping, telerobotic grasping with transmission delays adheres to Weber’s law. This suggests that telerobotic grasping with transmission delays is mediated by different perception–action associations compared to direct grasping. The evidence for the use of different perception–action mechanisms points at an inherent lack of transparency. Such a lack of transparency may indicate an inefficient visuomotor control during telerobotic operation.
In a related study, by Milstein et al. (see text footnote 1), the authors report that telerobotic control without transmission delays violates Weber’s law. These findings indicate that the time delays may be the most probable cause of the disruption to the ability of the operator to effectively utilize dorsal-stream computations when performing grasping tasks. Although a clear grasp success indication was attained from the lifting stage following object grasping, the participants did not receive haptic feedback during trials, as under the system’s time delays such feedback was highly unnatural and confusing. Therefore, an additional disruption of natural grasp processing may have been contributed by the lack of complete haptic and visual sensory integration in conveying grasp success. Yet, this was also the case in Milstein et al. (see text footnote 1), where violation of Weber’s law was found during telerobotic grasping without transmission delays.
The two additional mechanisms suggested as possible causes for the violation of Weber’s law in grasping, namely motion planning and biomechanical constraints, were not supported in the current study. While biomechanics may have influenced MGAs during the aperture transport stage, it clearly did not affect IGAs, or grasp control FGAs, i.e., the preparation of the finger aperture for grasping. Yet, biomechanics cannot be fully ruled out, as MGAs were larger than the IGAs and FGAs. As for motion planning for grasping, movement fragmentation and the similarity of results in both orientations support the assertion that, at least for remote manipulation with time delays, participants plan two motion components, reach and grasp formation, and that the planned grasp formation is based on object size.
In almost all cases, the participants were able to complete the tasks successfully. Most of the participants were clearly sensitive to object size as shown by the linear increase in mean aperture value for MGA, IGA, and FGA. This is also strengthened by the demonstrated dependence of the mean opening speed on the object size, as predicted by Fitts’ law (Hoffmann, 1992). These results indicate that the experimental setup and protocol were suitably adapted to the capabilities of the participants.
The participants in the grasp demonstration task controlled the telerobotic system for a few minutes during the first, training stage of the experiment. This stage was constructed to familiarize the users with the capabilities of the system. During the training, they were exposed to the system’s transmission time delays. It is well documented that consistent exposure to delay leads to adaptation (Foulkes and Miall, 2000; de la Malla et al., 2014; Farshchiansadegh et al., 2015; Rohde and Ernst, 2016; Avraham et al., 2017; Leib et al., 2017). Demonstrated movement characteristics were slower and larger (larger aperture opening, longer forward TCP motion) than required for successful task completion. Mean movement time was much longer (approximately twice) than movement time in natural reach-to-grasp motion (Jeannerod, 1984; Marteniuk et al., 1990; Wallace et al., 1990), and considerably longer than the movement time attainable by the robot. The spatial motion parameters, i.e., MGA and STCPD, were larger than the values required for performing task. It seems that participants have mistakenly assigned the temporal control error induced by the transmission delays to system dynamics. Identifying a lag in visual response as an increased system mass, force, or inertia is a well-known phenomenon (Smith, 1972; Vercher and Gauthier, 1992; Sarlegna et al., 2010; Honda et al., 2013; Takamuku and Gomi, 2015; Leib et al., 2017). In addition, compensating for inertial perturbations can be achieved by slowing down, which has been frequently found as a way to compensate for sensory delays, albeit being suboptimal (Rohde and Ernst, 2016). Regardless, the demonstrated movement profiles were easily adapted for successfully programing a robot to perform the task (Davidowitz and Berman, 2016).
The current research highlights the impacts of telerobotic system characteristics, specifically the transmission delay, on the operator’s motion. We show differences in adherence to Weber’s law between telerobotic operation with transmission delays, and operation without transmission delays, or direct grasping. Such differences may be related to differences internal perception–action mechanisms within the CNS. Telerobotic operation with transmission delays has been shown to lack transparency and to be more largely dependent on high-level cognitive control mechanisms than direct operation. In such conditions, telerobotic operators are more likely to be subjected to higher cognitive loads, a potential factor which should be accounted for in the design of telerobotic systems.
Ethics Statement
This study was carried out in accordance with the recommendations of “The Human Subject Research Committee of Ben-Gurion University of the Negev” with written informed consent from all subjects. All subjects gave written informed consent in accordance with the Declaration of Helsinki. The protocol was approved by the “The Human Subject Research Committee of Ben-Gurion University of the Negev.”
Author Contributions
OA, NS, SB, IN, and TG, each made substantial contributions to the study design, revised it critically for important intellectual content, and approved the final version of this written work. All authors listed meet the authorship criteria and no one qualified for authorship has been omitted. The first two authors, OA and NS, equally contributed to the manuscript, they collected data, aided in the design of data analysis procedures, performed data analysis, generated the first draft of this manuscript, and contributed to edits and updates to the document. The third author, IN, contributed to edits and updates of the manuscript. The fourth author, TG, aided in the design of data analysis procedures and contributed to edits and updates of the manuscript. The fifth author, SB, provided guidance for refining the data collection method, aided in the design of data analysis procedures, generated the first draft of this manuscript, and contributed to edits and updates of the manuscript.
Conflict of Interest Statement
The authors declare that the research was conducted in the absence of any commercial or financial relationships that could be construed as a potential conflict of interest.
Acknowledgments
The authors thank Prof. Yisrael Parmet for his help with the statistical analysis.
Funding
Research supported by the Helmsley Charitable Trust through the Agricultural, Biological and Cognitive Robotics Center of Ben-Gurion University of the Negev. IN is supported by the Israeli Science Foundation (grant 823/15), and the Binational United States–Israel Science Foundation (grant 2016850).
Abbreviations
CNS, central nervous system; FGA, final grip aperture. The aperture at the end of the movement (mm); IGA, initial grip aperture. The aperture after the end of the opening time (mm); JND, just noticeable difference; MGA, maximum grip aperture. The maximum aperture during the movement (mm); STCPD, The scaled sagittal TCP transport distance; TCP, Robotic Tool center point.
Footnote
- ^Milstein, A., Ganel, T., Berman, S., and Nisky, I. (submitted). The effect of gripper scaling on human-centered transparency of grasping in robot-assisted minimally invasive surgery.
References
Avraham, G., Mawase, F., Karniel, A., Shmuelof, L., Donchin, O., Mussa-Ivaldi, F. A., et al. (2017). Representing delayed force feedback as a combination of current and delayed states. J. Neurophysiol. 118, 2110–2131. doi: 10.1152/jn.00347.2017
Baird, J. C., and Noma, E. J. (1978). Fundamentals of Scaling and Psychophysics. New York: John Wiley & Sons.
Botzer, L., and Karniel, A. (2013). Feedback and feedforward adaptation to visuomotor delay during reaching and slicing movements. Eur. J. Neurosci. 38, 2108–2123. doi:10.1111/ejn.12211
Crossman, E. R. F. W., and Goodeve, P. J. (1983). Feedback control of hand-movement and Fitts’ law. Q. J. Exp. Psychol. 35, 251–278. doi:10.1080/14640748308402133
Cunningham, D. W., Chatziastros, A., von der Heyde, M., and Bülthoff, H. H. (2001). Driving in the future: temporal visuomotor adaptation and generalization. J. Vis. 1, 3. doi:10.1167/1.2.3
Davidowitz, I., and Berman, S. (2016). “Robot motion learning and adaptation,” in Computational Motor Control Workshop (CMCW), Jun 19–21; (Beer-Sheva).
de la Malla, C., Lopez-Moliner, J., and Brenner, E. (2014). Dealing with delays does not transfer across sensorimotor tasks. J. Vis. 14, 8. doi:10.1167/14.12.8
Farshchiansadegh, A., Ranganathan, R., Casadio, M., and Mussa-Ivaldi, F. A. (2015). Adaptation to visual feedback delay in a redundant motor task. J. Neurophysiol. 113, 426–433. doi:10.1152/jn.00249.2014
Ferrell, W. R. (1965). Remote manipulation with transmission delay. IEEE Trans. Hum. Factors Electron. HFE-6, 24–32. doi:10.1109/thfe.1965.6591253
Fitts, P. M. (1954). The information capacity of the human motor system in controlling the amplitude of movement. J. Exp. Psychol. 47, 381–391. doi:10.1037/h0055392
Flanagan, J. R., and Beltzner, M. A. (2000). Independence of perceptual and sensorimotor predictions in the size-weight illusion. Nat. Neurosci. 3, 737–741. doi:10.1038/76701
Flash, T., and Hogan, N. (1985). The coordination of arm movements: an experimentally confirmed mathematical model. J. Neurosci. 5, 1688–1703.
Foulkes, A. J., and Miall, R. C. (2000). Adaptation to visual feedback delays in a human manual tracking task. Exp. Brain Res. 131, 101–110. doi:10.1007/s002219900286
Ganel, T., Chajut, E., and Algom, D. (2008). Visual coding for action violates fundamental psychophysical principles. Curr. Biol. 18, R599–R601. doi:10.1016/j.cub.2008.04.052
Ganel, T., Freud, E., and Meiran, N. (2014). Action is immune to the effects of Weber’s law throughout the entire grasping trajectory. J. Vis. 14, 11. doi:10.1167/14.7.11
Ganel, T., and Goodale, M. A. (2003). Visual control of action but not perception requires analytical processing of object shape. Nature 426, 664–667. doi:10.1038/nature02156
Ganel, T., Namdar, G., and Mirsky, A. (2017). Bimanual grasping does not adhere to Weber’s law. Sci. Rep. 7, 6467. doi:10.1038/s41598-017-06799-4
Goodale, M. A., and Milner, A. D. (1992). Separate visual pathways for perception and action. Trends Neurosci. 15, 20–25. doi:10.1016/0166-2236(92)90344-8
Heath, M., and Manzone, J. (2017). Manual estimations of functionally graspable target objects adhere to Weber’s law. Exp. Brain Res. 235, 1701–1707. doi:10.1007/s00221-017-4913-8
Heath, M., Manzone, J., Khan, M., and Davarpanah Jazi, S. (2017). Vision for action and perception elicit dissociable adherence to Weber’s law across a range of ‘graspable’ target objects. Exp. Brain Res. 235, 3003–3012. doi:10.1007/s00221-017-5025-1
Held, R., Efstathiou, A., and Greene, M. (1966). Adaptation to displaced and delayed visual feedback from the hand. J. Exp. Psychol. 72, 887–891. doi:10.1037/h0023868
Hoffmann, E. R. (1992). Fitts’ law with transmission delay. Ergonomics 35, 37–48. doi:10.1080/00140139208967796
Honda, T., Hagura, N., Yoshioka, T., and Imamizu, H. (2013). Imposed visual feedback delay of an action changes mass perception based on the sensory prediction error. Front. Psychol. 4:760. doi:10.3389/fpsyg.2013.00760
Honda, T., Hirashima, M., and Nozaki, D. (2012a). Adaptation to visual feedback delay influences visuomotor learning. PLoS ONE 7:e37900. doi:10.1371/journal.pone.0037900
Honda, T., Hirashima, M., and Nozaki, D. (2012b). Habituation to feedback delay restores degraded visuomotor adaptation by altering both sensory prediction error and the sensitivity of adaptation to the error. Front. Psychol. 3:540. doi:10.3389/fpsyg.2012.00540
Jazi, S. D., and Heath, M. (2016). Pantomime-grasping: advance knowledge of haptic feedback availability supports an absolute visuo-haptic calibration. Front. Hum. Neurosci. 10:197. doi:10.3389/fnhum.2016.00197
Jazi, S. D., Yau, M., Westwood, D. A., and Heath, M. (2015). Pantomime-grasping: the ‘return’ of haptic feedback supports the absolute specification of object size. Exp. Brain Res. 233, 2029–2040. doi:10.1007/s00221-015-4274-0
Jeannerod, M. (1981). Intersegmental coordination during reaching at natural visual objects. Atten. Perform. 9, 153–168.
Jeannerod, M. (1984). The timing of natural prehension movements. J. Mot. Behav. 16, 235–254. doi:10.1080/00222895.1984.10735319
Jeannerod, M., Arbib, M. A., Rizzolatti, G., and Sakata, H. (1995). Grasping objects: the cortical mechanisms of visuomotor transformation. Trends Neurosci. 18, 314–320. doi:10.1016/0166-2236(95)93921-j
Kalmus, H., Denes, P., and Fry, D. B. (1955). Effect of delayed acoustic feed-back on some non-vocal activities. Nature 175, 1078. doi:10.1038/1751078a0
Lacquaniti, F., and Soechting, J. F. (1982). Coordination of arm and wrist motion during a reaching task. J. Neurosci. 2, 399–408.
Leib, R., Karniel, A., and Mussa-Ivaldi, F. A. (2017). The mechanical representation of temporal delays. Sci. Rep. 7, 7669. doi:10.1038/s41598-017-07289-3
Leib, R., Karniel, A., and Nisky, I. (2015). The effect of force feedback delay on stiffness perception and grip force modulation during tool-mediated interaction with elastic force fields. J. Neurophysiol. 113, 3076–3089. doi:10.1152/jn.00229.2014
Leib, R., Mawase, F., Karniel, A., Donchin, O., Rothwell, R., Nisky, I., et al. (2016). Stimulation of PPC affects the mapping between motion and force signals for stiffness perception but not motion control. J. Neurosci. 36, 10545–10559. doi:10.1523/JNEUROSCI.1178-16.2016
Löwenkamp, C., Gärtner, W., Haus, I. D., and Franz, V. H. (2015). Semantic grasping escapes Weber’s law. Neuropsychologia 70, 235–245. doi:10.1016/j.neuropsychologia.2015.02.037
Manzone, J., Jazi, S. D., Whitwell, R. L., and Heath, M. (2017). Biomechanical constraints do not influence pantomime-grasping adherence to Weber’s law: a reply to Utz et al. (2015). Vision Res. 130, 31–35. doi:10.1016/j.visres.2016.09.018
Marteniuk, G. G., Leavitt, J. L., MacKenzie, C. L., and Athenes, S. (1990). Functional relationships between grasp and transport components in a prehension task. Hum. Mov. Sci. 9, 149–176. doi:10.1016/0167-9457(90)90025-9
Morikiyo, Y., and Matsushima, T. (1990). Effects of delayed visual feedback on motor control performance. Percept. Mot. Skills 70, 111–114. doi:10.2466/pms.1990.70.1.111
Nisky, I., Mussa-Ivaldi, F. A., and Karniel, A. (2013). Analytical study of perceptual and motor transparency in bilateral teleoperation. IEEE Trans. Hum. Mach. Syst. 43, 570–582. doi:10.1109/tsmc.2013.2284487
Nisky, I., Pressman, A., Pugh, C. M., Mussa-Ivaldi, F. A., and Karniel, A. (2011). Perception and action in teleoperated needle insertion. IEEE Trans. Haptics 4, 155–166. doi:10.1109/TOH.2011.30
Ozana, A., and Ganel, T. (2017). Weber’s law in 2D and 3D grasping. Psychol. Res. doi:10.1007/s00426-017-0913-3
Preusche, C., and Hirzinger, G. (2007). Haptics in telerobotics. Vis. Comput. 23, 273–284. doi:10.1007/s00371-007-0101-3
Rand, M. K., Shimansky, Y., Stelmach, G. E., Bracha, V., and Bloedel, J. R. (2000). Effects of accuracy constraints on reach-to-grasp movements in cerebellar patients. Exp. Brain Res. 135, 179–188. doi:10.1007/s002210000528
Rohde, M., and Ernst, M. O. (2016). Time, agency, and sensory feedback delays during action. Curr. Opin. Behav. Sci. 8, 193–199. doi:10.1016/j.cobeha.2016.02.029
Rohde, M., van Dam, L. C. J., and Ernst, M. O. (2014). Predictability is necessary for closed-loop visual feedback delay adaptation. J. Vis. 14, 4. doi:10.1167/14.3.4
Santello, M., Flanders, M., and Soechting, J. F. (2002). Patterns of hand motion during grasping and the influence of sensory guidance. J. Neurosci. 22, 1426–1435.
Sarlegna, F. R., Baud-Bovy, G., and Danion, F. (2010). Delayed visual feedback affects both manual tracking and grip force control when transporting a handheld object. J. Neurophysiol. 104, 641–653. doi:10.1152/jn.00174.2010
Sheridan, T. B., and Ferrell, W. R. (1963). Remote manipulative control with transmission delay. IEEE Trans. Hum. Factors Electron. HFE-4, 25–29. doi:10.1109/thfe.1963.231283
Smeets, J. B. J., and Brenner, E. (1999). A new view on grasping. Motor Control 3, 237–271. doi:10.1123/mcj.3.3.237
Smeets, J. B. J., and Brenner, E. (2001). Independent movements of the digits in grasping. Exp. Brain Res. 139, 92–100. doi:10.1007/s002210100748
Smeets, J. B. J., and Brenner, E. (2008). Grasping Weber’s law. Curr. Biol. 18, R1089–R1090. doi:10.1016/j.cub.2008.10.008
Smith, W. M. (1972). Feedback: real-time delayed vision of one’s own tracking behavior. Science 176, 939–940. doi:10.1126/science.176.4037.939
Smith, W. M., and Bowen, K. F. (1980). The effects of delayed and displaced visual feedback on motor control. J. Mot. Behav. 12, 91–101. doi:10.1080/00222895.1980.10735209
Takamuku, S., and Gomi, H. (2015). What you feel is what you see: inverse dynamics estimation underlies the resistive sensation of a delayed cursor. Proc. Biol. Sci. 282, 20150864. doi:10.1098/rspb.2015.0864
Utz, K. S., Hesse, C., Aschenneller, N., and Schenk, T. (2015). Biomechanical factors may explain why grasping violates Weber’s law. Vision Res. 111, 22–30. doi:10.1016/j.visres.2015.03.021
Vercher, J. L., and Gauthier, G. (1992). Oculo-manual coordination control: ocular and manual tracking of visual targets with delayed visual feedback of the hand motion. Exp. Brain Res. 90, 599–609. doi:10.1007/BF00230944
Keywords: Weber’s law, telerobotics, grasping, motor control, human factors
Citation: Afgin O, Sagi N, Nisky I, Ganel T and Berman S (2017) Visuomotor Resolution in Telerobotic Grasping with Transmission Delays. Front. Robot. AI 4:54. doi: 10.3389/frobt.2017.00054
Received: 30 July 2017; Accepted: 06 October 2017;
Published: 25 October 2017
Edited by:
Matteo Bianchi, University of Pisa, ItalyReviewed by:
Alessandro Moscatelli, Università degli Studi di Roma Tor Vergata, ItalyRyad Chellali, Nanjing University of Technology, China
Simone Toma, Arizona State University, United States
Leonard James Smart, Miami University, United States
Copyright: © 2017 Afgin, Sagi, Nisky, Ganel and Berman. This is an open-access article distributed under the terms of the Creative Commons Attribution License (CC BY). The use, distribution or reproduction in other forums is permitted, provided the original author(s) or licensor are credited and that the original publication in this journal is cited, in accordance with accepted academic practice. No use, distribution or reproduction is permitted which does not comply with these terms.
*Correspondence: Sigal Berman, sigalbe@bgu.ac.il