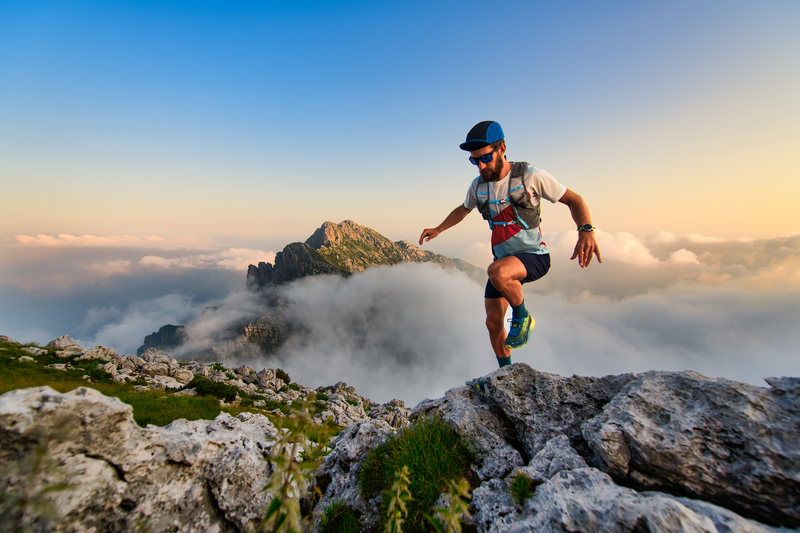
94% of researchers rate our articles as excellent or good
Learn more about the work of our research integrity team to safeguard the quality of each article we publish.
Find out more
ORIGINAL RESEARCH article
Front. Robot. AI , 27 July 2017
Sec. Humanoid Robotics
Volume 4 - 2017 | https://doi.org/10.3389/frobt.2017.00036
This article is part of the Research Topic Affective and Social Signals for HRI View all 14 articles
During occupational therapy for children with autism, it is often necessary to elicit and maintain engagement for the children to benefit from the session. Recently, social robots have been used for this; however, existing robots lack the ability to autonomously recognize the children’s level of engagement, which is necessary when choosing an optimal interaction strategy. Progress in automated engagement reading has been impeded in part due to a lack of studies on child-robot engagement in autism therapy. While it is well known that there are large individual differences in autism, little is known about how these vary across cultures. To this end, we analyzed the engagement of children (age 3–13) from two different cultural backgrounds: Asia (Japan, n = 17) and Eastern Europe (Serbia, n = 19). The children participated in a 25 min therapy session during which we studied the relationship between the children’s behavioral engagement (task-driven) and different facets of affective engagement (valence and arousal). Although our results indicate that there are statistically significant differences in engagement displays in the two groups, it is difficult to make any causal claims about these differences due to the large variation in age and behavioral severity of the children in the study. However, our exploratory analysis reveals important associations between target engagement and perceived levels of valence and arousal, indicating that these can be used as a proxy for the children’s engagement during the therapy. We provide suggestions on how this can be leveraged to optimize social robots for autism therapy, while taking into account cultural differences.
Autism spectrum conditions is a term for a group of complex neurodevelopmental conditions characterized by different challenges with social and reciprocal verbal and non-verbal communication, and repetitive and stereotyped behaviors (DSM-5, 2013). Social challenges are related to limitations in effective communication, social participation, social relationships, academic achievement, and/or occupational performance, individually or in combination. The onset of the symptoms occurs in the early developmental period, but deficits may not fully manifest until social communication demands exceed limited capacities. A recent meta-analysis based on 51 studies comparing children with autism and typically developing controls demonstrated a large effect size for motor issues in gait, postural control, motor coordination, upper limb control, and motor planning in autism (Fournier et al., 2010). Moreover, the overall motor performance is associated with the severity of diagnostic symptoms (Dziuk et al., 2007; Hilton et al., 2012), level of adaptive functioning (Kopp et al., 2010), and social withdrawal (Freitag et al., 2007). Children with autism also have difficulties in interpersonal synchrony (Marsh et al., 2013), which involves coordinating one’s actions with those of social partners, requiring appropriate social attention, imitation, and turn taking skills (Isenhower et al., 2012; Vivanti et al., 2014).
Early engagement with the world provides opportunities for learning and practicing new skills, and acquiring knowledge critical to cognitive and social development (Keen, 2009; Kishida and Kemp, 2009). However, in children with autism, displays of engagement are usually perceived as of low intensity, particularly in their social world (Keen, 2009). This limits the learning opportunities that occur naturally in their typically developing peers. For instance, Ponitz et al. (2009) showed that higher levels of engagement in typically developing children are correlated with better learning outcomes: kindergartners who were identified as more engaged in classroom activities had higher literacy achievement scores at the end of the year than those with lower levels of engagement. Odom (2002) found that the engagement level of children with autism in inclusive settings was comparable to children with other disabilities, and slightly lower when compared to children who were developing typically. However, Kemp et al. (2013) found that children with autism were engaged during free play activities only half of the time compared to children with other disabilities, who were engaged in free play activities. Likewise, Wong and Kasari (2012) found that preschoolers with autism in self-contained classrooms were disengaged for a lower amount of time during classroom activities (e.g., free play, centers, circle, and self-care activities). Possible reasons for the variability of engagement across these research studies include various definitions of engagement used, different activities/tasks (e.g., free play, circle time, and routines), type of classroom (e.g., self-contained versus inclusive), and child to adult ratio. This is in part due to the lack of consensus about both definition and measurement of engagement in population with autism (see e.g., McWilliam et al. (1992) and Keen (2009)). Furthermore, all the studies mentioned above assess the engagement via external observers and/or questionnaires, which can be lengthy and tedious. All this poses limitations for educators primarily, but also computer scientists aiming to build the technology (i.e., social and affective robots) that can be used to assess and measure the children’s engagement in a more effective and objective manner. To this end, we need first to find a suitable definition of engagement, investigate the related behavioral cues, and then build the tools and methods for its automatic measurement.
Russell et al. (2005) defines engagement as “the amount of time children spend interacting with the environment (with adults, children, or other materials) in a manner that is developmentally appropriate.” Engagement is also defined as “energy in action”—the connection between a person and activity; an active, constructive, focused interaction with one’s social and physical environment—consisting of three forms: behavioral, affective (emotional), and cognitive (Russell et al., 2005; Broughton et al., 2008). As described by Keen (2009), behavioral engagement refers to participation or involvement in learning activities and is related to on-task behavior, while affective engagement refers to the child’s interest in the activities (also expressed by different emotions and moods). For example, in the autism therapy with robots, Kim et al. (2012) defined behavioral engagement on a 0–5 Likert scale, each level corresponding to a set of the pre-defined responses by the child to the tasks and prompts from the therapist. Likewise, in robot interaction with typically developing children, affective engagement is defined as “concentrating on the task at hand and willingness to remain focused” (Ge et al., 2016). Cognitive engagement can best be described as the child’s eagerness or willingness to acquire and accomplish new skills and knowledge, and it relates to the goal directed behavior and self-regulated learning (Connell, 1990; Fredricks et al., 2004). As an example, Meece et al. (1988) measured the students’ performance in various learning tasks, providing evidence for the better school performance as a consequence of being focused on mastering a task, persisting longer, and expressing positive affect toward the task, thus, a combination of behavioral, affective, and cognitive engagement. While these three-dimensional constructs of engagement have been widely accepted, little attention has been paid to their contextual dimension. The latter is particularly important, as in order to measure engagement, we need to know whether the child is actively participating in target activity in a contextually appropriate manner (McWilliam et al., 1992; Eldevik et al., 2012). For this, we need also to gather information about the background context (Appleton et al., 2006), which can be described by a number of variables, such as the child’s demographics (age, gender, and cultural background), behavioral severity, individual vs. social interaction (Salam and Chetouani, 2015a), the use of tablets vs. robots, the type of therapy/tasks, and so on. To capture some aspects of this context taxonomy, Salam and Chetouani (2015b) proposed a model of human-robot engagement based on the context of the interaction (e.g., social, competitive, educative, etc.). A more recent work by Lemaignan et al. (2016) formalizes “with-me-ness,” a concept borrowed from the field of computer-supported collaborative learning, to measure to what extent the human is engaged with the robot (on a Likert scale 0–5) over the course of an interactive task. While useful in measuring the attentional focus of the children interacting with a robot, “with-me-ness” does not quantify the behavioral engagement that we address in this work. Specifically, we adapt the engagement definition from Kim et al. (2012), focusing on the task-response time to define engagement levels on a 0–5 Likert scale. We study how levels of this behavioral engagement vary as a function of (i) context (the task, culture, and behavioral severity of the children) and (ii) different facets of the children affective engagement (the perceived valence and arousal levels and the face expressivity, as described in Sec. 2). Note that most of the works on engagement in human-robot interaction (HRI) report binary engagement (engaged vs. disengaged) mainly due to the difficulty in capturing subtle changes in engagement displays. However, when more complex interactions are considered, such coarse definition is insufficient to explain differences in the children’s behavior. To the best of our knowledge, this is the first study that analyses the behavioral engagement (on a fine grained intensity scale) of children with autism, and in the context of assistive social robots deployed in different cultures.
The most commonly used model of affect Russell and Pratt (1980) suggests that all affective states arise from two fundamental neurophysiological systems, embedded in a circumplex with two orthogonal dimensions: valence (pleasure–displeasure continuum) and arousal (sleepiness–excitement continuum). Buckley et al. (2004) found that both positive valence and arousal are good indicators of emotional engagement in learning tasks. Conversely, musical engagement has been shown to be a good predictor of perceived valence and arousal (Olsen et al., 2014). A study on happiness found that the more the subjects were engaged and satisfied, the more they experienced positive valence and high arousal (Pietro et al., 2014). In the context of HRI, Habib (2014) provides analysis of relationships between the self-reported levels of valence and arousal, and the task difficulty, which was directly related to the user’s “mental” engagement with the task (engaged vs. being bored). Studies on the design of engaging personal robots emphasize the importance of these two dimensions for optimizing HRI (Breazeal, 2003). For instance, Castellano et al. (2014b) showed that in the child-robot interaction, the children’s valence, interest, and anticipatory behavior are strong predictors of the (social) engagement with the robot. Motivated by these findings and considering that expressions of affect are expected to differ significantly in children with autism (Volkmar et al., 2005); in this work, we investigate the relationship between (perceived) valence and arousal, as two components of affective engagement, and the target behavioral engagement in the context of autism therapy with social robots. Note that arousal (and other facets of affect such as the face expressivity) can be measured from outward behavioral cues, e.g., facial expressions (Gunes et al., 2011), as well as inward cues, e.g., physiological signals such as the skin conductance response of the autonomic nervous system (Picard, 2009; Hedman et al., 2012). In this study, we limit our consideration to the outward behavioral characteristics of valence and arousal.
Engagement with social robots for children with autism is about drawing their attention and interests toward both robot and social tasks, and maintaining the prolonged therapy sessions (Scassellati et al., 2012). Furthermore, educational, therapeutic, and assistive aspects of HRI are highly motivating environments for children with autism due to the simple, predictable, and non-intimidating nature of robots compared to humans (Robins et al., 2005; Scassellati, 2007). Also, the interaction mechanism in the field of assistive robots for children with autism is more focused on the social aspects of interaction than the physical interaction (Fong et al., 2003), such as joint attention, turn-taking, or imitation behavior, which are important target behavior for the children (Scassellati et al., 2012). In this context, the effectiveness of social interaction between robots and children increases when robotic systems have the capacity of generating coordinated and timely behaviors relevant to social surroundings (Breazeal, 2001). Such adaptive strategies for social interaction are expected to become the basis of a new class of interactive robots that act as “friends” and “mentors” to improve children’s experience during, for instance, the hospital stay, and support their learning (Kanda et al., 2007; Belpaeme et al., 2013). It is, therefore, critical that the robots are able to engage the children in target activities. In social robotics, engagement is usually approached from the perspective of the design of the robot’s appearance and its interaction capability. For instance, Tielman et al. (2014) showed that a robot that changed its voice, body pose, eye-color, and gestures in response to the emotions of children was perceived as more engaging than a robot that did not exhibit such adaptive behaviors. Similarly, Shen et al. (2015) showed that when the robot feedback based on the perceived user’s sentiment is provided, as part of an emotion mimicry interaction, the users’ were more engaged than when only a plain mimicking of the users was performed by the robot. In what follows, we review recent work providing evidence of engagement of children during interaction with robots and in the context of autism. We refer interested readers to Breazeal (2003, 2004) and Scassellati et al. (2012) for more detailed reviews of recent advances in social robotics.
The role of social robots in autism therapy is primarily (i) to act as a mediator between the therapist/caregiver and children with autism (Robins et al., 2010; Thill et al., 2012)—as in our study, (ii) to provide an interactive object to draw and maintain the children’s attention (Robins et al., 2006), and (iii) to be a playful device facilitating the children’s entertainment during the therapy (Scassellati et al., 2012). The advantages of using robots are, therefore, to help the children with autism to perceive and respond to the outside world through the least invasive exercises. This is mainly because the robots can modulate their behavioral responses according to the children’s internal dynamics and are capable of repetitive behavior, in contrast to humans (Wainer et al., 2014). The application of interactive robots for development of communication skills in children with autism has been shown in many studies to be effective (Robins et al., 2008). Scassellati et al. (2012) and Diehl et al. (2012) observed repeatedly that children who suffer from difficulties in communication with other people surprisingly started to interact with them more easily when the communication was assisted with the robots. In a comparison of the responses to a robot vs. virtual agent environments, Dautenhahn and Werry (2004) showed that the children with autism were more engaged in playing a chasing game with the robot.
Imitative behaviors such as “reach-to-grasp” tasks performed by a human and by a robot were found more engaging and motivating when a robot was used (Pierno et al., 2008; Suzuki et al., 2017). In a pilot study of child-robot interactions (age 2–4) with a toy robot, capable of showing signs of attention by changing its gaze direction, and of articulating the emotional displays of pleasure and excitement, Kozima et al. (2007) showed that these positively engaged and influenced the children’s emotional responses. Similarly, Stanton et al. (2008) reported that children with autism preferred to play with an interactive robotic dog (AIBO) rather than a toy having similar appearance but no interaction features. De Silva et al. (2009) proposed a therapeutic robot for children with autism, showing that children enjoyed interaction with the robot and that this approach enhanced their attention, based on an analysis of their eye-gaze. François et al. (2009) used a robot-assisted play, with the game designed in conformity with individual needs and abilities of each child. The authors validated their approach with a group of children with autism that were engaged in a non-directed play with a pet robot (Aibo ERS-7), which can assess the children’s progress across three dimensions: play, reasoning, and affect, showing that each child exhibited highly individual patterns of play. Wainer et al. (2010) assessed collaborative behaviors in a group of children with autism, showing that the interaction with robots was more engaging, fostering collaboration among the groups through a more active interaction with their peers during the robot sessions. Focusing on behavioral cues of children with autism and those of their typical peers during interaction with NAO, Anzalone et al. (2015) showed that the children with autism exhibited significantly lower yaw movements and less stable gaze, while the posture variance was significantly lower in typical children, during a joint attention task. To summarize, all these studies evidence the benefits of using robots for facilitating the learning and interaction of children with autism. One limitation is the difficulty in generalizing these findings and comparing them across studies as different settings and performance measures were used. For this reason, in our analysis of engagement across the two cultures, we focus on two identical situations set in similar contexts (as described in Sec. 6).
The importance of cultural diversity when studying different populations has been emphasized in a number of psychology studies (Russell, 1994; Scherer and Wallbott, 1994; Elfenbein and Ambady, 2002). For instance, the work by Scherer and Wallbott (1994) provides evidence for cultural variation in emotion elicitation, regulation, symbolic representation, and social sharing among populations from 37 countries. Likewise, Ekman (2005) found that whereas 95% of U.S. participants associated a smile with “happiness,” only 69% of Sumatran participants did. Similarly, 86% of U.S. participants associated wrinkling of the nose with “disgust,” but only 60% of Japanese did (Krause, 1987). Thus, subjective interpretation of specific emotions (i.e., primarily the cognitive component of emotion) differs across cultures (Uchida et al., 2004). These are seen as “cultural differences in perception, or rules about what emotions are appropriate to show in a given situation.” Culture also influences expressiveness of emotions (Immordino-Yang et al., 2016). There are rather consistent patterns across Eastern and Western cultures, although differences also exist across cultures, and sometimes even within cultures (An et al., 2017; McDuff et al., 2017). Recently, Lim (2016) explored cultural differences in emotional arousal level between the East and West, focusing on the observation that high arousal emotions are valued and promoted more than low arousal emotions in the West. On the other hand, in the East cultures, low arousal emotions are valued more than high arousal emotions, with people preferring to experience low rather than high arousal emotions. Nevertheless, apart from a handful of works, virtually all studies on cultural differences focus on the typically developing population. Below, we focus our studies on cultural differences in autism.
Since autism also involves social challenges, its treatment and interventions need to be tailored to target cultures (Dyches et al., 2004; Kitzhaber, 2012; Cascio, 2015). Several cross-cultural studies highlight that the culture-based treatments are crucial for individuals with autism (Tincani et al., 2009; Conti et al., 2015). For example, Daley (2002) argues that the transcultural1 supports are needed for the pervasive developmental conditions, including autism. So far, only a few studies have been conducted in this direction. Perepa (2014) conducted a study that investigated the cultural context in interventions for children with autism and with a diverse cultural background—British, Somali, West African, and south Asian. They found that the cultural background of the children’s parents is highly relevant to their social behavior, emphasizing the importance of transcultural treatments for children with autism. However, one limitation of this study is that the target children all lived in the UK, and, thus, the role of the cultural context may have been reduced. Libin and Libin (2004) showed that the children’s background, such as culture and/or psychological profile, can have a large impact on the robot therapy. Specifically, the authors conducted cross-cultural studies with Americans and Japanese in an interactive session using the robot cat called NeCoRo. Among other findings, they showed that, overall, Americans enjoyed more patting the robot than Japanese. Thus, accounting for cultural preferences is important when designing interactive games with robots. However, we are unaware of any published studies that looked into cultural differences in engagement, and, in particular, the social robots for autism therapy. A possible reason for the lack of such studies is that the heterogeneity in behavioral patterns of children with autism within cultures is already so pronounced (Happé et al., 2006). A famous adage says: “If you have met one person with autism, you have met one person with autism.” Therefore, attempting analysis of these differences from a higher level (particularly, in terms of different cultures) is a far-fetched goal. Yet, it is necessary to look at these differences at multiple levels: within and between cultures, where the former would focuses on differences within and between the children with the same cultural background. This, in turn, would potentially allow the robot solutions to be adapted to each culture first by accounting for the differences that may exist among children, followed by individual adaptation to each child within a culture (e.g., by focusing on its age, gender, and psychological profile). In this work, we analyze multiple facets of engagement at each level mentioned above.
We present a study aimed at analysis of behavioral engagement of children with autism in the context of occupational therapy assisted with a humanoid robot NAO. By focusing on two culturally diverse groups: Asia (Japan) and Eastern Europe (Serbia), we provide insights into cultural differences of engagement among these two groups in terms of (i) the task difficulty, its relationships to (ii) the affective dimensions (valence and arousal), and (iii) behavioral cues (facial expressivity). We chose these three because they are important for the design of child-robot interactions: (i) is important for the robot’s ability to select a task respectful of the child’s abilities, while (ii), (iii) are critical when building computer vision and machine learning algorithms that can automatically estimate the child’s engagement, and, thus, enable robots to naturally engage the children in learning activities.
To the best of our knowledge, this is the first study of engagement in the context of social humanoid robots and therapy for children with autism across two cultures. Most previous work on engagement in autism focused on the discrete engagement (engaged vs. disengaged) (Hernandez et al., 2014) and within a culture. By contrast, we provide an analysis of engagement on a fine-grained scale (0–5) and in two cultural settings. Our exploratory analysis provides useful insights into the relationships between engagement dynamics as expressed within and between the two cultures, as well as its relationships to the perceived affect (valence and arousal). As one of the main findings, we provide evidence that outward displays of affect (valence and arousal) can be used as a proxy of target behavioral engagement. This confirms previous findings on the relationship between engagement and affect displays in typical individuals within a single culture (Buckley et al., 2004). Based on this, we provide suggestions for future research on automated measurement of children’s engagement during robot-assisted autism therapy, which takes the cultural diversity of the children into account. We also provide an overview of the most recent efforts in the field of social and affective robots for autism.
The rest of the paper is organized as follows: we first describe the data and methods used to elicit and analyze engagement of children with autism. We then present and discuss our results. In the light of these results, we provide insights into the current challenges of engagement measurement (with the focus on automated methods) and provide suggestions for future research.
We used the dataset produced by interactions between children with autism, specialized therapists, and NAO2 robot. The interaction was recorded as part of occupational therapy for children with autism, following steps designed based on the Theory of Mind (ToM) (Cohen, 1993) teaching approach to emotion recognition and expression (Howlin et al., 1999). In the original version, the children are asked by the therapist to pair the images of people’s expressive faces (see Figure 1) with four basic emotions (happiness, sadness, anger, and fear (Gross, 2004)) through storytelling. For the purpose of the study, the scenario was adapted to include NAO as an assistive tool in the tasks of emotion imitation and recognition.
Figure 1. The recording setting at the site in Japan (right) and Serbia (left). The NAO’s expressions of four basic emotions (sadness, fear, anger, and happiness), paired with the expression cards, are depicted in the middle. Note that in Japan the children were seated on the floor, while children in Serbia were using a chair—a reflection of the cultural preferences.
The interaction started with free play with NAO. Once the child felt comfortable, the following phases were attempted. (1) Pairing cards of static face images with the NAO’s expressions: the therapist shows the card of an emotion and then activates NAO, via a remote control using the wizard-of-Oz approach (Scassellati et al., 2012), to display its (bodily) expression of that emotion. This was repeated for all four emotion categories. (2) Recognition: the therapist shows the NAO’s expression of a target emotion and asks the child to select the correct emotion card. If the child selects the correct emotion card, the therapist moves to the next emotion, also providing a positive feedback; otherwise, the therapist moves to another emotion without the feedback. This is iterated until either the child correctly paired all emotions, or the therapist decided that the child was unable to do so. (3) Imitation: the therapist asks the child to imitate the NAO’s expressions. (4) Story: the therapist tells a short story involving NAO and asks the child to guess/show how NAO would feel in that particular situation. Note the increasing difficulty in executing each of the four phases: the Pairing phase requires minimal motor and cognitive performance. On the other hand, the Story phase is the most challenging, as it requires “social imagination,” which has been shown to be limited in children with autism (Howlin et al., 1999).
The whole interaction lasted, on average, 25 min per child. In cases when a child was not interested in the play, the therapist would use occasional prompts, calling her/his name, and/or activate NAO, who then waved at the child, saying “hi, hello,” and alike, to (re)engage the child. It is important to mention that the purpose of the designed scenario was not to improve existing therapies for autism, but rather induce a context in which the engagement can be measured more objectively (see Table 2). This protocol was reviewed and approved by relevant Institutional Review Boards (IRBs),3 and informed consent was obtained in writing from the parents of the children participating in the study.
The children participating in the study included 17 from Asia (Japan) and 19 from Eastern Europe (Serbia), age 3–13 (see Table 1). They were all referred based on a previous diagnosis of autism. After the interaction with the robot, the child’s behavioral severity was quantified using the Childhood Autism Rating Scale (CARS) (Baird, 2001), a diagnostic assessment method commonly used to differentiate children with autism from those with other developmental delays using scoring criteria (from non-autistic to severely autistic). The CARS is a practical and brief measure that encompasses both the social–communicative and the behavioral flexibility aspects of autism’s diagnostic triad (Chen et al., 2012). The CARS were filled out by the Japanese and Serbian therapist who interacted with the children. They both have 20+ years of working with children with autism and see regularly the children who participated in this study. Scores 30–36.5 are considered mild-to-moderate autism and scores of 37–60 as moderate-to-severe autism (Chen et al., 2012). As can be observed from Table 1, Japanese participants were slightly younger than Serbian participants. In both samples, the boys outnumbered the girls, reflective of the gender ratio in autism. From average CARS scores, we note that, within the selected groups, the participants from Serbia exhibited more obvious autistic traits. Note also that some of the children were below the autism threshold of 30, despite their autism diagnosis. Again, CARS is an indicative measure of the behavioral severity, and we report it to show the group differences obtained using the same scoring test. Note that we did not include typically developing children as controls, as there are no claims being made here about autism vs. typical development.
The interactions were recorded using a high-resolution webcam with a microphone (see Figure 1). To measure the children’s engagement during the interaction, each video was coded in terms of engagement levels defined based on the occurrence and relative timing of the children’s behavior (including learning-related behaviors), and the therapist’s requests and prompts. To this end, we adapted the coding approach proposed in Kim et al. (2012) and defined engagement on a 0–5 Likert scale, with 0 corresponding to the events when the child is fully non-compliant (evasive) and 5 when fully engaged (see Table 2 for description). Note that in Kim et al. (2012), 10 sec long fragments of target videos were coded in terms of the engagement levels. By contrast, we find it more objective to code the whole engagement episode: starting with the target task, e.g., the therapist asking the child to select the card corresponding to the NAO’s expression (the Recognition phase), until one of the conditions (Table 2) for scoring the engagement level has been met. We propose this task-driven coding of engagement4 as it preserves the context in which the engagement is measured. By coding short fixed-interval segments, as in Kim et al. (2012), the beginning and the end of target activity can easily be lost (since its duration varies across the tasks/children).
Table 2. Engagement coding [adaptation of the engagement definition proposed in Kim et al. (2012)].
After the engagement episodes were coded in the videos, each episode was further coded in terms of the affective dimensions (valence and arousal) and the children’s face-expressivity.5 As explained in Sec. 2, valence and arousal are well-studied affective dimensions and they have been related to a number of emotional states and moods; however, this has not been investigated much in autism. To analyze the relationship of the perceived valence and arousal, and target engagement levels, the engagement episodes were coded for valence and arousal on a 5-point ordinal scale [−2,2]. For example, the episodes were coded with high negative valence (−2) in cases when the child showed clear signs of experiencing unpleasant feelings (being unhappy, angry, visibly upset, showing dissatisfaction, frightened), dissatisfaction and disappointment (e.g., when NAO showed an expression that the child did not anticipate). The very positive valence (+2) was coded when the child showed signs of intense happiness (e.g., clapping hands), joy (in most cases followed with episodes of laughter), and delight (e.g., when NAO performed). The observable cues of arousal are directly related to the child’s level of excitement. The episodes in which the child seemed very bored or uninterested6 (e.g., looking away, not showing interest in the interaction, sleepy, passively observing) were coded as a very low arousal (−2). Note that this outward expression of a very low arousal could also be a consequence of intense internal arousal that led to a shut-down state (Picard and Goodwin, 2008). However, in this work, we focus on outward expressions of target affective states. The levels in between (−1,+1) for both dimensions just varied in their intensity (thus, being of lower intensity than the aforementioned). The neutral state of valence/arousal (0,0) corresponded to cases where the child seemed alert and/or attentive, with no obvious signs of any emotion, and/or physical activity (head, hand, and/or bodily movements). Note that the coders were instructed to base their judgments solely on the children’s outwards signs described above, and not their “intuition” about the children’s internal states, in order to focus on most objectively visible data. It is important to mention again the key difference between these two dimensions (facets of affective engagement) and the directly measured engagement levels: while the former are purely based on the behavioral cues, as reflection of the children’s level of joy (valence) and excitement (arousal), the latter is task driven (i.e., its score is based on a number of prompts and pre-defined activities, as defined in Table 2). Finally, the engagement episodes were also coded in terms of facial expressiveness of the children within the episodes. This was coded on a 0–5 Likert scale, from neutral (0) to very expressive (5) (regardless of the type of facial expression, such as positive or negative). Each episode was assigned a score based on the observed level of activation of facial muscles throughout the episode, thus, taking the total duration of the expressive video segments into account (and the parts where the face is mostly visible).
All video episodes of engagement were coded by two experienced occupational therapists (from Japan and Serbia, who did not participate in the recordings), with the percent agreement of 92.4%. This is expected as the coding scheme (Table 2) clearly defines the beginning/ending of the episodes. The disagreeing parts were caused by the language differences. For instance, in some cases, the coders needed the meaning of the vocalizations to make sure, e.g., that the therapist asked the child a question and not just made a statement. After the coding has been performed by each coder separately, the beginning/ending of each episode was adjusted by the coders together. The coding of the affective dimensions as well as face expressivity was done by the same coders (separately). Note that lower levels of agreements were obtained: valence (75.8%), arousal (67.4%), and face expressivity (69.8%). However, this is still widely accepted as a good level of agreement (Carmines and Zeller, 1979).
For studying specifics of the participants’ interactions with NAO, throughout this section we provide qualitative as well as quantitative (statistical) analysis of relationships between the engagement levels, the affect dimensions, and corresponding contextual variables (tasks and culture). To measure association between these variables, we report Pearson’s correlation (r), as it is a commonly used dependence measure in HRI applications. Analysis of the group differences (within and between the two cultures) was performed using Welch’s t-test (Welch, 1947) due to its robustness to the unequal variances. If not said otherwise, only outcomes with significance levels p ≤ 0.05 were considered for interpretations.
We start by comparing the average engagement levels within each phase. In Table 3, we report the mean (M) and standard deviation (SD) for these values. Note that the average engagement in the Japanese did not vary as much as in the Serbs, in whom the highest (average) engagement levels occur in phases Pairing and Story. In the first phase, despite their behavioral severity, Serbs were able to perform the tasks fast because these were simple (see Sec. 6 (Protocol)). By contrast, children who reached the Story phase did not have much difficulty engaging, which explains the high average engagement (M = 4.54, SD = 0.68). By looking into the cross-cultural differences in the engagement levels per phase, we found that these were significantly different for all phases but the Story. Again, we suspect that this is because the majority of children, who reached the last phase, showed high levels of engagement. By looking into the mean duration (in seconds) of the engagement episodes per phase, we note that in phase Pairing, Serbs engaged much faster than their Japanese peers. Yet, we see in Serbs a much steeper increase in the time toward higher phases, with the duration of phases pairing/recognition being significantly different between the cultures. Table 4 reveals that the higher levels of engagement, on average, last much shorter than lower levels (<3). The high duration of phase story in Serbs was also biased by a frequent presence of lower (longer) engagement levels (episodes) (see Figure 2). Note also the significant differences in duration of the engagement episodes across levels 3–5 of the two cultures. A reason for level 5 being longer in Japanese is possibly because they had lower CARS, and, therefore, took longer to engage in the target activity.
Table 3. Average engagement levels (with one SD) within each phase, and the phase duration, computed per culture.
Figure 2. The number (left)/percentage (right) of the participants from the two cultures that showed in at least one of their engagement episodes, the target level. Note that less than 30% of Japanese have levels 0–3, while the remaining 70% have at least one instance of the higher levels of engagement. By contrast, more than 70% of Serbs showed levels of very low engagement. We also observe that, in Japanese, the engagement distribution is largely skewed toward higher levels, in contrast to Serbs, who have more uniformly distributed levels.
We next analyze the relationships between the engagement and perceived affect (valence and arousal, as well as the face expressivity) within target engagement episodes. Figure 3 shows the (normalized) distributions of the four dimensions. We first observe that the affect distributions are very close in shape when compared across the cultures, with the valence being uniformly distributed at the intermediate levels (−1,0,1). This indicates that highly positive/negative expressions were not perceived during the interaction. Distributions of arousal, on the other hand, are more Gauss-like, signaling the majority of episodes with low arousal, with a few having very low/high (perceived) arousal. The distribution of the face expressivity levels is highly skewed to the right—thus, very low levels (or no facial activity) were observed. However, this in line with (DSM-5, 2013) emphasizing the presence of “deficits in nonverbal communicative behaviors used for social interaction, ranging, for example, from poorly integrated verbal and nonverbal communication […] to a total lack of facial expressions and nonverbal communication” in children with autism. While these distributions are similar across the cultures, notice the differences in distributions of the engagement levels.
Figure 3. The (normalized) distribution of the levels for each coded dimension: valence, arousal, face expressivity, and engagement. Note that in both cultures, the affective dimensions exhibit similar distributions, while engagement distributions are highly skewed to the highest level in Japanese. The face expressivity in both cultures is skewed toward the neutral, confirming the expected low face expressivity in children with autism (DSM-5, 2013).
To get better insights into the relationships between the engagement levels and the affect dimensions, in Figure 4, we depict the (normalized) co-occurrence matrices. First, there is a strong positive correlation between the affective dimensions (valence and arousal) observed in both cultures (r = 0.73 and r = 0.56, respectively) and is more pronounced in Serbs. Lo et al. (2016) showed that (neurotypical) participants were more capable of distinguishing valence than arousal changes in emotion expressions, thus, capturing these when occurring together may be easier. Also, Brewer et al. (2016) showed that neurotypical persons have, in general, a difficulty in recognizing emotional expressions of persons with autism. This could, in part, explain why we obtained high correlations between the two affective dimensions: when both increase/decrease, it might be more obvious to the coders to perceive the change in the level. Also, this can be attributed to the way the persons with autism express their valence and arousal, which looks more obvious if both are very high or low, e.g., the child is expressing happiness with smiles and laughter, and fast movements of arms (flapping). Again, note that the coders were instructed to judge these two dimensions based on behavioral signs commonly observed in a neurotypical population (see Sec. 6). The dependence between valence and engagement is more spread in Serbs than Japanese, which is in part due to the highly imbalanced levels of engagement in the latter. However, we observe that in Japan, the positive valence occurs frequently at higher levels (3–4) and the negative (−1) is more present at lower engagement levels. By contrast, in Serbs, this is not that pronounced, since we can see that the valence levels are more smoothly distributed, with the negative valence occurring even at higher levels of engagement (e.g., the child sitting calm, might look bored or sad, looking around in the room but still participating in the task). We draw similar conclusions from the arousal–engagement relationships. We also note that in both cultures, high engagement never occurs with very low arousal, but mainly with the neutral and/or low positive arousal, as in cases when the child is sitting calm, showing no significant movements, and is being focused on the tasks. This may also be due to the coding bias. Finally, we see from the facial activity, that regardless of the engagement levels, the average face-expressivity was very low (mainly 0) in both cultures, showing very low (and insignificant) correlation (r < 0.20) with the engagement levels. In addition to the lack or atypical facial expressiveness in autistic population, as mentioned above, this could also be, in part, the consequence of scoring the whole engagement episode level rather than the image frames. This, in turn, may result in the coders ignoring subtle changes in the children’s facial expressions, the presence of which is obvious due to the perceived variation in the valence levels.
Figure 4. Dependence structures of the coded dimensions, computed as co-occurrence matrices of the corresponding levels. These are normalized across columns to remove the bias toward the highest engagement level (as can be seen from Figure 3). This, in turn, allows us to analyze the distribution of each level of affect dimensions within the target engagement levels. The Pearson correlation (r) with significance levels is shown above each plot.
Table 5 shows average levels of target affective dimensions w.r.t. the engagement levels of the two cultures. We observe that within the higher levels of engagement (specifically, level 5), the average valence level is much higher than in the levels below and significantly different between the cultures. This indicates that, on average, Serbs showed more pronounced expressions of positive states (e.g., joy and interest), as can also be noted from the face expressivity levels. However, while their average arousal levels were similar at the peak of engagement, Japanese showed significantly lower arousal levels across all engagement levels, with much lower arousal when being evasive (level 0). This can be ascribed to the differences in behavioral dynamics in these two cultures as well as the individual expressions of resistance to specific interactions with NAO. For instance, some children preferred to imitate expressions of certain emotions only, and not all. This (inner) resistance to comply with the task can also be related to their (in)ability to recognize all of the four emotions. However, a detailed analysis of the target stimulus is out of the scope of this work.
So far, we focused mainly on the group (the between culture) differences of the children under the study. It is, however, important to assess some of these differences within the cultures and also by looking at the individual variation. Figure 5 depicts the changes in the engagement levels, along with the affect dimensions, and the corresponding CARS for each child. Note the heterogeneity in the occurrences of the target levels per child. For example, we observe in Japanese (ID: 1, 16, and 12) and Serbs (ID: 7, 12, 15, and 18) that although valence and arousal are both (highly) negative, their average engagement was relatively high. This possibly is a consequence of idiosyncratic behavioral responses of the children: the same children had higher CARS, which means less functionality. This may be the reason for their expressions of valence and arousal being harder to perceive accurately (Brewer et al., 2016), although their engagement was high. We also observe from the children with ID:14 (Serb) and ID:3&15 (Japanese) that their high facial expressivity is typically followed with high engagement, valence, and arousal levels. This could indicate that these children are showing more obvious signs of positive emotions. We also report in Figure 5 (above each plot) the results of the t-test for the cultural differences w.r.t. the four target variables. Note that no significant differences were found (with p < 0.05), in valence, arousal, and face expressivity. However, we found a significant difference in the distribution of the average engagement levels in Serbs and Japanese.7
Figure 5. Changes in the average frequency of the corresponding levels of target dimensions, per child. The children’s ids were sorted according to the descending (average) engagement levels and the remaining dimensions aligned accordingly. The individual CARS are shown above the engagement bars.
We further investigate the between- and within-culture differences by looking into the Pearson (r) and Spearman scores (ρ) for dependencies between the average levels of valence, arousal, face expressivity, engagement, and CARS. We report both scores as the former assumes linear relationships and constant variance. As these can easily be violated due to the heterogeneity in the children, quantifying monotonic relationships (using the Spearman coefficient) may be a better indicator of target relationships (McDonald, 2009). For interpretations, we consider only the statistically significant scores (with p < 0.05). In Figure 4, the valence and arousal are highly (and linearly) correlated (as judged from similar r and ρ). There is also a high correlation between the perceived valence and face expressivity observed in both cultures (r > 0.65). This is expected, as both valence and arousal are directly related to the face modality (and facial expressions in particular), which provide the key cues when quantifying the (perceived) valence/arousal (Adolph and Georg, 2010). However, in contrast to the valence dimension, the face expressivity is not coded for the sign (positive/negative). Interestingly, despite the high (and significant) correlations between valence–face-expressivity (r = 0.67 in Serbs and r = 0.74 in Japanese) and valence-engagement (r = 0.69 in Serbs and r = 0.49 in Japanese), the correlations engagement–face-expressivity are relatively low and insignificant. This shows that the sign of valence (positive as when happy/negative as when sad) could be a good indicator of engagement. We also note that in Serbs, the engagement was highly correlated with the average valence levels per child. In Japanese, we observe the opposite from Table 6 (arousal is far more correlated with the engagement than valence). This may add evidence to the previous studies “showing variation as demonstrating cultural differences in ‘display rules,’ or rules about what emotions are appropriate o show in a given situation” (Ekman, 1972).
Table 6. The Pearson correlation (r) and Spearman rank correlation coefficient (ρ), with their significance levels (p), for the children’s average levels of valence, arousal, face expressivity, and engagement, as well as their CARS.
The findings described above can, in part, be attributed to the CARS of Japanese being lower, and, thus, them being more responsive (through timely body movements in response to target tasks). On the other hand, if we recall the engagement levels from Figure 3, in Serbs, the levels varied more than in Japanese, indicating that the former group showed more hesitation in doing the tasks. More importantly, note that there is a highly significant correlation between engagement and CARS (r = −0.52 and ρ = −0.71) in Japanese, while these are found to be insignificant in Serbs. To test whether these two groups are statistically different in engagement levels due to the cultural difference, and not due to the differences in the behavioral severity of the participants, we remove the CARS as a big causal factor, and one that is highly correlated with engagement on the Japanese side. After tossing out highest/lowest CARS, we match the Serbs and Japanese on CARS within the range [33–43], thus, with the mid behavioral severity. This range assured the best possible match between the CARS of the two cultures, which can also be seen from Figure 6, where we ranked the engagement from high to low CARS, resulting in 8 Serbs and 8 Japanese, with a very similar functionality levels. We ran the t-test on this sample and again obtained the statistically significant difference in the engagement levels between the two cultures [t(12) = −2.1, p = 0.05]. This shows that these differences are not only due to the variation in behavioral severity (CARS) solely but also due to other factors, the most likely being the culture. We also found highly strong relationships between CARS and engagement (ρ = −0.86, p <0.01 for Serbs, and ρ = − 0.82, p = 0.01 for Japanese—see Figure 6), thus, the Spearman scores are more consistent when the similar subgroups are matched based on CARS.
Figure 6. Engagement levels of the subset of children that are matched based on their CARS, ranking the average engagement levels from high CARS to low CARS. Note that the differences in the engagement levels between the two cultural groups are still statistically different with 5% significance level. Note also the strong relationships between the CARS and average engagement levels.
Before providing a further discussion of the study described, it is important to emphasize that this analysis was of the exploratory nature within a specific context: an occupational therapy for children with autism, using a social robot NAO, and recorded in two different cultures. Specifically, the participants in this study are 36 children from Japan and Serbia, who participated only once for a short duration. We note again that the aim of this study was not to propose a new therapy for autism, but induce a context in which the children’s engagement can be measured in a structured way. Our analysis of the relationships between the behavioral engagement and affective components of engagement (the perceived valence and arousal, as well as face expressivity) showed significant differences in a number of parameters considered. However, we restrain from making any conclusive statements about the causal cultural differences in these two groups. This is for the following reasons. First, although the parametric tests used in our analysis did find statistically significant relationships, a great variation was also found within the cultures and within the individuals (in terms of age, gender, and CARS). Therefore, larger-scale studies are needed to further confirm and extend our findings on the variations across cultures. Second, one of the goals of this study was to get better insights into the expression of engagement in naturalistic settings, where children with autism interact regularly with a therapist. This is in order to identify the key behavioral cues of engagement and its relationship to other parameters (in this case, task difficulty, affect, and CARS) that should be considered when designing social robots for autism therapy.
As noted by Keen (2009), “the ability to detect differences in engagement levels as a function of different program types, differences within programs, instructional methods, and other forms of ‘intervention’ is a first step toward establishing the validity of the measure for program evaluation purposes.” Also, children with autism (and/or other neurodevelopmental differences) spend less time actively engaged with adults and their peers, and less time in mastery-level engagement with materials than do the typically developing children (McWilliam and Bailey, 1995). This is why it is very important to develop techniques where the children can master social skills through therapeutic settings, with the aim of being able to translate those in the play with their (typically developing) peers (Keen, 2009). Toward this end, the role of social and affective robots is twofold: (i) to provide more efficient and reliable (standardized) means of measuring children’s engagement and (ii) to enable naturalistic interaction with the children by being able to automatically estimate their level of engagement and respond to it accordingly (e.g., timely giving a positive feedback and encouragement). Before this can be implemented, it is necessary to understand better the context: the children’s behavioral and other parameters that relate to their engagement. In what follows, we briefly discuss our findings from this perspective and provide suggestions for future work in this direction.
The main findings relate to how engagement levels differed as a function of the cultures, tasks, affective dimensions, and CARS-based behavioral severity. Our results indicate that there are statistically significant differences in duration and average levels of engagement between the two cultures, when compared in terms of the task difficulty. Japanese were able to engage and complete easier tasks faster, while Serbs (those who reached the last phase in the interaction) were engaged for a shorter time in the interaction. This can be a consequence of the latter group being affected more severely by the condition, as also reflected in their CARS and the distribution of the engagement levels. By matching the two groups based on CARS, we were still able to find significant differences between the engagement levels in the two cultures. Another important aspect to be considered (especially when comparing the task execution time) and that is not explored in detail in our analysis is the children’s age. While most of the typically developing children develop both motor and mental abilities by the age of four (Baron-Cohen et al., 1985), the lack of the same is indicated by the CARS scores in autism. By looking into the age of the children who performed the tasks faster than the others, we did not notice any significant dependencies on age.
These types of analyses are important because they provide a prior knowledge that can be used to adjust the dynamics of the robot interaction within each culture/age-range, with a possibility for further individual adjustment (Picard and Goodwin, 2008). For example, these could be used as priors for computer models, as part of the robot’s perception. This has recently been attempted in terms of the personality adjustment, with the focus on typically developing adults (Salam et al., 2017). Adding to this, one of the important findings of our study is the relatively high correlation between the CARS and engagement levels (e.g., in Japanese, Spearman coefficient ρ = −71%, p = 0.01). When the CARS range was matched across the cultures (Figure 6), these reached ρ = −82−86%, p = 0.01, in Japan and Serbia, respectively. This could potentially be a good calibrating parameter for social robots, derived from an easy- and fast-to-obtain measure of the current behavioral condition. However, it should be investigated whether the other standardized tests (e.g., specific questions of ADI-R and/or ADOS (Le Couteur et al., 2008)) would provide more universal indicators for (expected) engagement levels, resulting in a more stable input for choosing the therapy mode, establishing therapy expectations and follow ups on the individual progress. Moreover, this could potentially allow the medical doctors and therapists to more easily assess the therapeutic value of the whole procedure, through the common protocols, which could be adjusted to each culture and with assistance of the social robots.
Our analysis of the relationships between the directly measured behavioral engagement and indirectly measured affective engagement (from the valence and arousal levels perceived from outward behavioral cues, including the face expressivity) revealed statistically significant (p < 0.01) dependencies between the valence and arousal levels (average per child) and the behavioral engagement, with Spearman coefficient ρ = 68−75%. Therefore, these two affective dimensions can potentially be used as a proxy of the children’s behavioral engagement. The benefit of this is that these two affective dimensions can automatically be measured in human-robot interaction by means of existing models for affective computing (Picard, 1997; Castellano et al., 2014a). Specifically, a number of works on automated estimation of valence and arousal have been proposed (Zeng et al., 2009; Gunes et al., 2011). For instance, Nicolaou et al. (2011) showed that automatically estimated levels of valence/arousal can achieve the agreement similar to that of human coders when multi-modal behavioral cues are used as input (facial expressions, shoulder gestures, and audio cues). As we showed in our experiments (Table 6), while the face expressivity was highly correlated with valence/arousal levels, it did not relate strongly to the behavioral engagement. Investigating other facial cues such as the eye-gaze (typically used in autism studies (Chen et al., 2012; Jones et al., 2016)) in the context of engagement is a promising way to go. Moreover, it is critical to take into account the multi-modal nature of human behavior when estimating engagement. In a recent work, Salam et al. (2017) measured individual and group engagement by an automated multi-modal system that exploits outward behavioral cues (face and body gestures) as well as contextual variables (the personality traits of a user). They were able to improve significantly the engagement estimation when the individual features (face and body gestures) were included. While in this work, we focused on outward measures of the target affective dimensions, note that significant advances have been made in measuring the same from inward expressions (biosignals such as the heart rate, electrodermal activity (EDA), body temperature, and so on) (Picard, 2009). For instance, Hernandez et al. (2013) showed that there are significant relationships between autonomic changes in arousal levels (measured using a wristband sensor) and behavioral challenges in children with autism in a school setting. In another work, Hernandez et al. (2014) showed that automatically estimating the ease of engagement on a scale (0–2) of typical children, participating in interactions with the educator and the parent, can be achieved with an accuracy of up to 68% (from EDA solely). Likewise, but in the context of children with autism and human-computer interaction, Liu et al. (2008) achieved automatic detection of liking, anxiety, and engagement, from physiological signals (EDA, heart rate and temperature) with an accuracy of 82.9%. Further research on the use of social robots in autism should also closely examine these modalities for automated analysis of engagement.
However, none of the methods that could potentially be used for automated estimation of engagement and/or valence and arousal, were evaluated before in the context of autism and child-robot interaction. Therefore, there are at least a few important questions for the future work toward building a system for automated estimation of engagement of children with autism, and in the context of therapies involving social robots. First, how can multiple modalities of children’s behavior (including inward and outward expressions) be modeled efficiently using models for affective computing (Picard, 2009; Castellano et al., 2014a) to take the full advantage of their complimentary nature? This, in turn, would not only provide better insights into behavioral cues of engagement but also enable a more accurate and reliable perception of engagement by social robots. How to account for the contextual aspect of engagement is another important challenge in automating engagement estimation. As our results suggest, the culture (among other factors) may play an important role in modulating the time each child spends in a target activity, as well as the distributions and average levels of engagement. One approach would be to define affective computing models so that they embed this contextual information via priors on the model parameters, which can then be adjusted to each child as the therapy progresses. This brings us to our final and the most important challenge: how do we personalize the models to each child and obtain the child-specific estimation of target engagement levels? Although, in our study, we did not find statistically significant differences in engagement levels within either of the two cultures, we did, however, observe high levels of individual variation. Therefore, personalizing the models to each child with autism is, perhaps, the most challenging aspect of automated engagement estimation that the future work will face (Picard and Goodwin, 2008). Another important factor not addressed in this study is the influence of culture on the annotation process. While annotators in our study achieved a high level of agreement, as shown in several other studies (e.g., see Engelmann and Pogosyan (2013)), handling the annotators bias effectively is of paramount importance when designing social robots that can automatically estimate engagement. Likewise, CARS is the standard, and, thus, its scoring should not be affected by cultural background of the therapists (but it still may be affected). While the presence of cultural biases in autism screening is inevitable, Mandell and Novak (2005) showed that it is mostly due to the different perception of autistic traits by parents with different cultural backgrounds. Since the therapists did the CARS in our study, we do not expect these differences to be as affected by the culture.8
Taken together, the findings of this study and the questions raised clearly indicate that more research is needed in the field of social and affective robotics for autism. While our study focused on a single day recordings of the children, future research should focus on longitudinal studies of engagement, if more reliable conclusions are to be drawn and data for automating the engagement estimation collected. There is an overall lack of such studies and data (especially across cultures); yet, they are of critical importance for building more effective technology that could facilitate, augment, and scale, rather than replace, the efforts by medical doctors and therapists working directly with individuals with autism. We hope that this work will increase awareness for the need of such studies and also provide useful insights into computer scientists in the field of affective computing and social robotics, and also neuroscientists, psychologists, therapists, and educators working in the autism field. Finally, we must keep our sights on the main goal: building technology and insights that ultimately bring benefit to users on the autism spectrum, especially those who seek to sustain engagement more successfully in learning experiences.
This research has been approved by institutional review board (IRB) of Chubu University, Japan; Mental Health Institute, Serbia; and MIT COUHES, USA.
OR and JL are the lead authors of this work: they conducted the study and organized the data collection. LM-M contributed via constant discussions and literature review. BS provided useful insights about the study and feedback during the preparation of the manuscript. RP helped to define the structure of the manuscript, interpret the results, and improve the content of the manuscript.
The authors declare that the research was conducted in the absence of any commercial or financial relationships that could be construed as a potential conflict of interest.
This work was supported by MEXT Grant-in-Aid for Young Scientists B grant no. 16763279 and Chubu University Grant I grant no. 27IS04I (Japan). The work of OR was funded by EU HORIZON 2020 under grant agreement no. 701236 (ENGAGEME)—Marie Skłodowska-Curie Individual Fellowship, and the work of BS under grant agreement no. 688835 (DE-ENIGMA). We thank the Serbian Autism Society (Ms. V. Petrovic and Ms. S. Petrovic), the occupational therapists: Ms. S. Babovic, Ms. N. Selic, Ms. Y. Takeuchi, Ms. K. Hara and Prof. M. Kojima, and Prof. M. Pejovic, MD (Mental Health Institute – MHI, Belgrade), for their help and discussions over the course of this study. We also thank Prof. G. Obinata, Prof. M. Pantic, and Dr. J. Shen for their support and feedback in the early stage of this study. Our special thanks go to all the children and their parents who participated in this study—without them, this research would not be possible.
Adolph, D., and Georg, W. A. (2010). Valence and arousal: a comparison of two sets of emotional facial expressions. Am. J. Psychol. 123, 209–219. doi: 10.5406/amerjpsyc.123.2.0209
An, S., Ji, L.-J., Marks, M., and Zhang, Z. (2017). Two sides of emotion: exploring positivity and negativity in six basic emotions across cultures. Front. Psychol. 8:610. doi:10.3389/fpsyg.2017.00610
Anzalone, S. M., Boucenna, S., Ivaldi, S., and Chetouani, M. (2015). Evaluating the engagement with social robots. Int. J. Soc. Robot. 7, 465–478. doi:10.1007/s12369-015-0298-7
Appleton, J. J., Christenson, S. L., Kim, D., and Reschly, A. L. (2006). Measuring cognitive and psychological engagement: validation of the student engagement instrument. J. Sch. Psychol. 44, 427–445. doi:10.1016/j.jsp.2006.04.002
Baird, G. (2001). Screening and surveillance for autism and pervasive developmental disorders. Arch. Dis. Child. 84, 468–475. doi:10.1136/adc.84.6.468
Baron-Cohen, S., Leslie, A. M., and Frith, U. (1985). Does the autistic child have a “theory of mind”? Cognition 21, 37–46. doi:10.1016/0010-0277(85)90022-8
Belpaeme, T., Baxter, P. E., Read, R., Wood, R., Cuayáhuitl, H., Kiefer, B., et al. (2013). Multimodal child-robot interaction: building social bonds. J. Hum. Robot Interact. 1, 33–53. doi:10.5898/JHRI.1.2.Belpaeme
Breazeal, C. (2001). “Affective interaction between humans and robots,” in Advances in Artificial Life, Vol. 2159. (Berlin, Heidelberg: Springer), 582–591.
Breazeal, C. (2003). Emotion and sociable humanoid robots. Int. J. Hum. Comput. Stud. 59, 119–155. doi:10.1016/S1071-5819(03)00018-1
Brewer, R., Biotti, F., Catmur, C., Press, C., Happé, F., Cook, R., et al. (2016). Can neurotypical individuals read autistic facial expressions? atypical production of emotional facial expressions in autism spectrum disorders. Autism Res. 9, 262–271. doi:10.1002/aur.1508
Broughton, M., Stevens, C., and Schubert, E. (2008). “Continuous self-report of engagement to live solo marimba performance,” in Int’l Conference on Music Perception and Cognition, Sapporo, 366–371.
Buckley, S., Hasen, G., and Ainley, M. (2004). Affective Engagement: A Person-Centered Approach to Understanding the Structure of Subjective Learning Experiences. Melbourne, Australia: Australian Association for Research in Education, 1–20.
Cascio, M. A. (2015). Cross-cultural autism studies, neurodiversity, and conceptualizations of autism. Cult. Med. Psychiatry 39, 207–212. doi:10.1007/s11013-015-9450-y
Castellano, G., Gunes, H., Peters, C., and Schuller, B. (2014a). “Multimodal affect recognition for naturalistic human-computer and human-robot interactions,” in The Oxford Handbook of Affective Computing, eds R. Calvo, S. D’Mello, J. Gratch, and A. Kappas (USA: Oxford University Press), 246.
Castellano, G., Leite, I., Pereira, A., Martinho, C., Paiva, A., and Mcowan, P. W. (2014b). Context-sensitive affect recognition for a robotic game companion. ACM Trans. Interact. Intell. Syst. 4, 1–25. doi:10.1145/2622615
Chen, G. M., Yoder, K. J., Ganzel, B. L., Goodwin, M. S., and Belmonte, M. K. (2012). Harnessing repetitive behaviours to engage attention and learning in a novel therapy for autism: an exploratory analysis. Front. Psychol. 3:12. doi:10.3389/fpsyg.2012.00012
Cohen, S. B. (1993). “From attention-goal psychology to belief-desire psychology: The development of a theory of mind and its dysfunction,” in Understanding Other Minds: Perspectives from Autism (Oxford: Oxford University Press), 59–82.
Connell, J. P. (1990). Context, self, and action: a motivational analysis of self-system processes across the life span. Self Trans. Infancy Child. 8, 61–97.
Conti, D., Cattani, A., Di Nuovo, S., and Di Nuovo, A. (2015). “A cross-cultural study of acceptance and use of robotics by future psychology practitioners,” in IEEE Int’l Symposium on Robot and Human Interactive Communication (RO-MAN), Kobe, 555–560.
Daley, T. C. (2002). The need for cross-cultural research on the pervasive developmental disorders. Transcult. Psychiatry 39, 531–550. doi:10.1177/136346150203900409
Dautenhahn, K., and Werry, I. (2004). Towards interactive robots in autism therapy: background, motivation and challenges. Pragmat. Cogn. 12, 1–35. doi:10.1075/pc.12.1.03dau
De Silva, R. S., Tadano, K., Higashi, M., Saito, A., and Lambacher, S. G. (2009). “Therapeutic-assisted robot for children with autism,” in IEEE Int’l Conf. on Intelligent Robots and Systems (IROS), St. Louis, MO, 3561–3567.
Diehl, J. J., Schmitt, L. M., Villano, M., and Crowell, C. R. (2012). The clinical use of robots for individuals with autism spectrum disorders: a critical review. Res. Autism Spectr. Disord. 6, 249–262. doi:10.1016/j.rasd.2011.05.006
DSM-5. (2013). Diagnostic and Statistical Manual of Mental Disorders: DSM-5. American Psychiatric Association.
Dyches, T. T., Wilder, L. K., Sudweeks, R. R., Obiakor, F. E., and Algozzine, B. (2004). Multicultural issues in autism. J. Autism Dev. Disord. 34, 211–222. doi:10.1023/B:JADD.0000022611.80478.73
Dziuk, M. A., Larson, J. C. G., Apostu, A., Mahone, E. M., Denckla, M. B., and Mostofsky, S. H. (2007). Dyspraxia in autism: association with motor, social, and communicative deficits. Dev. Med. Child Neurol. 49, 734–739. doi:10.1111/j.1469-8749.2007.00734.x
Ekman, P. (1972). “Universals and cultural differences in facial expressions of emotion,” in Nebraska Symposium on Motivation. ed. J. Cole (Lincoln, NE: University of Nebraska Press) 207–282.
Eldevik, S., Hastings, R. P., Jahr, E., and Hughes, J. C. (2012). Outcomes of behavioral intervention for children with autism in mainstream pre-school settings. J. Autism Dev. Disord. 42, 210–220. doi:10.1007/s10803-011-1234-9
Elfenbein, H. A., and Ambady, N. (2002). On the universality and cultural specificity of emotion recognition: a meta-analysis. Psychol. Bull. 128, 203–235. doi:10.1037/0033-2909.128.2.203
Engelmann, J. B., and Pogosyan, M. (2013). Emotion perception across cultures: the role of cognitive mechanisms. Front. Psychol. 4:118. doi:10.3389/fpsyg.2013.00118
Fong, T., Nourbakhsh, I., and Dautenhahn, K. (2003). A survey of socially interactive robots. Rob. Auton. Syst. 42, 143–166. doi:10.1016/S0921-8890(02)00372-X
Fournier, K. A., Hass, C. J., Naik, S. K., Lodha, N., and Cauraugh, J. H. (2010). Motor coordination in autism spectrum disorders: a synthesis and meta-analysis. J. Autism Dev. Disord. 40, 1227–1240. doi:10.1007/s10803-010-0981-3
François, D., Powell, S., and Dautenhahn, K. (2009). A long-term study of children with autism playing with a robotic pet: taking inspirations from non-directive play therapy to encourage children’s proactivity and initiative-taking. Interact. Studies 10, 324–373. doi:10.1075/is.10.3.04fra
Fredricks, J. A., Blumenfeld, P. C., and Paris, A. H. (2004). School engagement: potential of the concept, state of the evidence. Rev. Educ. Res. 74, 59–109. doi:10.3102/00346543074001059
Freitag, C. M., Kleser, C., Schneider, M., and von Gontard, A. (2007). Quantitative assessment of neuromotor function in adolescents with high functioning autism and Asperger syndrome. J. Autism Dev. Disord. 37, 948–959. doi:10.1007/s10803-006-0235-6
Ge, B., Park, H. W., and Howard, A. M. (2016). “Identifying engagement from joint kinematics data for robot therapy prompt interventions for children with autism spectrum disorder,” in Int’l Conference on Social Robotics, 531–540.
Gross, T. F. (2004). The perception of four basic emotions in human and nonhuman faces by children with autism and other developmental disabilities. J. Abnorm. Child Psychol. 32, 469–480. doi:10.1023/B:JACP.0000037777.17698.01
Gunes, H., Schuller, B., Pantic, M., and Cowie, R. (2011). “Emotion representation, analysis and synthesis in continuous space: A survey,” in IEEE Int’l Conference on Automatic Face & Gesture Recognition (FG’W), 827–834.
Happé, F., Ronald, A., and Plomin, R. (2006). Time to give up on a single explanation for autism. Nat. Neurosci. 9, 1218–1220. doi:10.1038/nn1770
Hedman, E., Miller, L., Schoen, S., Nielsen, D., Goodwin, M., and Picard, R. (2012). “Measuring autonomic arousal during therapy,” in Proceedings of Design and Emotion, 11–14.
Hernandez, J., Riobo, I., Rozga, A., Abowd, G. D., and Picard, R. W. (2014). “Using electrodermal activity to recognize ease of engagement in children during social interactions,” in ACM Int’l Joint Conference on Pervasive and Ubiquitous Computing, 307–317.
Hernandez, J., Sano, A., Zisook, M., Deprey, J., Goodwin, M., and Picard, R. W. (2013). “Analysis and visualization of longitudinal physiological data of children with ASD,” in The Extended Abstract of IMFAR, 2–4.
Hilton, C. L., Zhang, Y., Whilte, M. R., Klohr, C. L., and Constantino, J. (2012). Motor impairment in sibling pairs concordant and discordant for autism spectrum disorders. Autism 16, 430–441. doi:10.1177/1362361311423018
Howlin, P., Baron-Cohen, S., and Hadwin, J. (1999). Teaching Children with Autism to Mind-Read: A Practical Guide for Teachers and Parents. New York: Wiley.
Immordino-Yang, M. H., Yang, X.-F., and Damasio, H. (2016). Cultural modes of expressing emotions influence how emotions are experienced. Emotion 16, 1033–1039. doi:10.1037/emo0000201
Isenhower, R. W., Marsh, K. L., Richardson, M. J., Helt, M., Schmidt, R., and Fein, D. (2012). Rhythmic bimanual coordination is impaired in young children with autism spectrum disorder. Res. Autism Spectr. Disord. 6, 25–31. doi:10.1016/j.rasd.2011.08.005
Jones, R. M., Southerland, A., Hamo, A., Carberry, C., Bridges, C., Nay, S., et al. (2016). Increased eye contact during conversation compared to play in children with autism. J. Autism Dev. Disord. 47, 607–614. doi:10.1007/s10803-016-2981-4
Kanda, T., Sato, R., Saiwaki, N., and Ishiguro, H. (2007). A two-month field trial in an elementary school for long-term human-robot interaction. IEEE Trans. Robot. 23, 962–971. doi:10.1109/TRO.2007.904904
Keen, D. (2009). Engagement of children with autism in learning. Aust. J. Spec. Educ. 33, 130–140. doi:10.1375/ajse.33.2.130
Kemp, C., Kishida, Y., Carter, M., and Sweller, N. (2013). The effect of activity type on the engagement and interaction of young children with disabilities in inclusive childcare settings. Early Child. Res. Q. 28, 134–143. doi:10.1016/j.ecresq.2012.03.003
Kim, E., Paul, R., Shic, F., and Scassellati, B. (2012). Bridging the research gap: making HRI useful to individuals with autism. J. Hum. Robot Interact. 1, 26–54. doi:10.5898/JHRI.1.1.Kim
Kishida, Y., and Kemp, C. (2009). The engagement and interaction of children with autism spectrum disorder in segregated and inclusive early childhood center-based settings. Topics Early Child. Spec. Educ. 29, 105–118. doi:10.1177/0271121408329172
Kitzhaber, S. (2012). Interventions for multicultural children with autism. Master Soc. Work Clin. Res. Papers 118, 1–67.
Kopp, S., Beckung, E., and Gillberg, C. (2010). Developmental coordination disorder and other motor control problems in girls with autism spectrum disorder and/or attention-deficit/hyperactivity disorder. Res. Dev. Disabil. 31, 350–361. doi:10.1016/j.ridd.2009.09.017
Kozima, H., Nakagawa, C., and Yasuda, Y. (2007). Children–robot interaction: a pilot study in autism therapy. Prog. Brain Res. 164, 385–400. doi:10.1016/S0079-6123(07)64021-7
Krause, R. (1987). Universals and cultural differences in the judgments of facial expressions of emotion. J. Pers. Soc. Psychol. 5, 4–712.
Le Couteur, A., Haden, G., Hammal, D., and McConachie, H. (2008). Diagnosing autism spectrum disorders in pre-school children using two standardised assessment instruments: the ADI-R and the ADOS. J. Autism Dev. Disord. 38, 362–372. doi:10.1007/s10803-007-0403-3
Lemaignan, S., Garcia, F., Jacq, A., and Dillenbourg, P. (2016). “From real-time attention assessment to with-me-ness in human-robot interaction,” in The Int’l Conference on Human Robot Interaction (HRI) (IEEE Press), 157–164.
Libin, A., and Libin, E. (2004). Person-robot interactions from the robopsychologists’ point of view: the robotic psychology and robotherapy approach. Proc. IEEE 92, 1789–1803. doi:10.1109/JPROC.2004.835366
Lim, N. (2016). Cultural differences in emotion: differences in emotional arousal level between the east and the west. Integr. Med. Res. 5, 105–109. doi:10.1016/j.imr.2016.03.004
Liu, C., Conn, K., Sarkar, N., and Stone, W. (2008). Physiology-based affect recognition for computer-assisted intervention of children with autism spectrum disorder. Int. J. Hum. Comput. Stud. 66, 662–677. doi:10.1016/j.ijhsc.2008.04.003
Lo, L., Hung, N., and Lin, M. (2016). Angry versus furious: a comparison between valence and arousal in dimensional models of emotions. J. Psychol. 150, 949–960. doi:10.1080/00223980.2016.1225658
Mandell, D. S., and Novak, M. (2005). The role of culture in families’ treatment decisions for children with autism spectrum disorders. Ment. Retard. Dev. Disabil. Res. Rev. 11, 110–115. doi:10.1002/mrdd.20061
Marsh, K. L., Isenhower, R. W., Richardson, M. J., Helt, M., Verbalis, A. D., Schmidt, R. C., et al. (2013). Autism and social disconnection in interpersonal rocking. Front. Integr. Neurosci. 7:4. doi:10.3389/fnint.2013.00004
McDonald, J. H. (2009). Handbook of Biological Statistics. Baltimore, MD: Sparky House Publishing, 2.
McDuff, D., Girard, J. M., and El Kaliouby, R. (2017). Large-scale observational evidence of cross-cultural differences in facial behavior. J. Nonverbal Behav. 41, 1–19. doi:10.1007/s10919-016-0244-x
McWilliam, R., Bailey, D., Bailey, D., and Wolery, M. (1992). Promoting engagement and mastery. Teach. Infants Preschool. Disabil. 2, 230–255.
McWilliam, R., and Bailey, D. B. Jr. (1995). Effects of classroom social structure and disability on engagement. Topics Early Child. Spec. Educ. 15, 123–147. doi:10.1177/027112149501500201
Meece, J. L., Blumenfeld, P. C., and Hoyle, R. H. (1988). Students’ goal orientations and cognitive engagement in classroom activities. J. Educ. Psychol. 80, 514–523. doi:10.1037/0022-0663.80.4.514
Nicolaou, M. A., Gunes, H., and Pantic, M. (2011). Continuous prediction of spontaneous affect from multiple cues and modalities in valence-arousal space. IEEE Trans. Affect. Comput. 2, 92–105. doi:10.1109/T-AFFC.2011.9
Odom, S. L. (2002). Widening the Circle: Including Children with Disabilities in Preschool Programs. Early Childhood Education Series. Teachers College Press.
Olsen, K. N., Dean, R. T., and Stevens, C. J. (2014). A continuous measure of musical engagement contributes to prediction of perceived arousal and valence. Psychomusicology 24, 147–156. doi:10.1037/pmu0000044
Perepa, P. (2014). Cultural basis of social ‘deficits’ in autism spectrum disorders. Eur. J. Spec. Needs Educ. 29, 313–326. doi:10.1080/08856257.2014.908024
Picard, R. W. (2009). Future affective technology for autism and emotion communication. Philos. Trans. R. Soc. B 364, 3575–3584. doi:10.1098/rstb.2009.0143
Picard, R. W., and Goodwin, M. S. (2008). Innovative technology: the future of personalized autism research and treatment. Autism 50, 32–39.
Pierno, A. C., Mari, M., Lusher, D., and Castiello, U. (2008). Robotic movement elicits visuomotor priming in children with autism. Neuropsychologia 46, 448–454. doi:10.1016/j.neuropsychologia.2007.08.020
Pietro, C., Silvia, S., and Giuseppe, R. (2014). The pursuit of happiness measurement: a psychometric model based on psychophysiological correlates. Sci. World J. 2014, 1–15. doi:10.1155/2014/139128
Ponitz, C., Rimm-Kaufman, S., Grimm, K., and Curby, T. (2009). Kindergarten classroom quality, behavioral engagement, and reading achievement. School Psych. Rev. 38, 102–120.
Robins, B., Dautenhahn, K., Boekhorst, R. T., and Billard, A. (2005). Robotic assistants in therapy and education of children with autism: can a small humanoid robot help encourage social interaction skills? Univ. Access Inform. Soc. 4, 105–120. doi:10.1007/s10209-005-0116-3
Robins, B., Dautenhahn, K., and Dubowski, J. (2006). Does appearance matter in the interaction of children with autism with a humanoid robot? Interact. Stud. 7, 509–542. doi:10.1075/is.7.3.16rob
Robins, B., Ferrari, E., and Dautenhahn, K. (2008). “Developing scenarios for robot assisted play,” in IEEE Int’l Symposium on Robot and Human Interactive Communication (RO-MAN), 180–186.
Robins, B., Ferrari, E., Dautenhahn, K., Kronreif, G., Prazak-Aram, B., Gelderblom, G.-J., et al. (2010). Human-centred design methods: developing scenarios for robot assisted play informed by user panels and field trials. Int. J. Hum. Comput. Stud. 68, 873–898. doi:10.1016/j.ijhcs.2010.08.001
Russell, J. A. (1994). Is there universal recognition of emotion from facial expression? A review of the cross-cultural studies. Psychol. Bull. 115, 102. doi:10.1037/0033-2909.115.1.102
Russell, J. A., and Pratt, G. (1980). A description of the affective quality attributed to environments. J. Pers. Soc. Psychol. 38, 311–322. doi:10.1037/0022-3514.38.2.311
Russell, V., Ainley, M., and Frydenberg, E. (2005). Student motivation and engagement. School. Issues Digest 2, 1–11.
Salam, H., Celiktutan, O., Hupont, I., Gunes, H., and Chetouani, M. (2017). Fully automatic analysis of engagement and its relationship to personality in human-robot interactions. IEEE Access 5, 705–721. doi:10.1109/ACCESS.2016.2614525
Salam, H., and Chetouani, M. (2015a). “Engagement detection based on mutli-party cues for human robot interaction,” in Int’l Conference on Affective Computing and Intelligent Interaction (ACII), 341–347.
Salam, H., and Chetouani, M. (2015b). “A multi-level context-based modeling of engagement in human-robot interaction,” in IEEE Int’l Conference on Automatic Face and Gesture Recognition (FG’W), Vol. 3, 1–6.
Scassellati, B. (2007). “How social robots will help us to diagnose, treat, and understand autism,” in In Robotics Research (Springer), 552–563.
Scassellati, B., Admoni, H., and Matarić, M. (2012). Robots for use in autism research. Annu. Rev. Biomed. Eng. 14, 275–294. doi:10.1146/annurev-bioeng-071811-150036
Scherer, K. R., and Wallbott, H. G. (1994). Evidence for universality and cultural variation of differential emotion response patterning. J. Pers. Soc. Psychol. 66, 310–328. doi:10.1037/0022-3514.66.2.310
Shen, J., Rudovic, O., Cheng, S., and Pantic, M. (2015). “Sentiment apprehension in human-robot interaction with NAO,” in Int’l Conference on Affective Computing and Intelligent Interaction (ACII), 867–872.
Stanton, C. M., Kahn, P. H., Severson, R. L., Ruckert, J. H., and Gill, B. T. (2008). “Robotic animals might aid in the social development of children with autism,” in Int’l Conference on Human-Robot Interaction (HRI), 271–278.
Suzuki, R., Lee, J., and Rudovic, O. (2017). “Nao-dance therapy for children with ASD,” in Int’l Conference on Human-Robot Interaction (HRI), 295–296.
Thill, S., Pop, C. A., Belpaeme, T., Ziemke, T., and Vanderborght, B. (2012). Robot-assisted therapy for autism spectrum disorders with (partially) autonomous control: challenges and outlook. Paladyn 3, 209–217.
Tielman, M., Neerincx, M., Meyer, J.-J., and Looije, R. (2014). “Adaptive emotional expression in robot-child interaction,” in Int’l Conference on Human-Robot Interaction (HRI), 407–414.
Tincani, M., Travers, J., and Boutot, A. (2009). Race, culture, and autism spectrum disorder: understanding the role of diversity in successful educational interventions. Res. Pract. Pers. Sev. Disabil. 34, 81–90. doi:10.2511/rpsd.34.3-4.81
Uchida, Y., Norasakkunkit, V., and Kitayama, S. (2004). Cultural constructions of happiness: theory and empirical evidence. J. Happiness Stud. 5, 223–239. doi:10.1007/s10902-004-8785-9
Vivanti, G., Trembath, D., and Dissanayake, C. (2014). Mechanisms of imitation impairment in autism spectrum disorder. J. Abnorm. Child Psychol. 42, 1395–1405. doi:10.1007/s10802-014-9874-9
Volkmar, F. R., Paul, R., Klin, A., and Cohen, D. J. (2005). Handbook of Autism and Pervasive Developmental Disorders, Diagnosis, Development, Neurobiology, and Behavior, Vol. 1. John Wiley & Sons.
Wainer, J., Dautenhahn, K., Robins, B., and Amirabdollahian, F. (2014). A pilot study with a novel setup for collaborative play of the humanoid robot KASPAR with children with autism. Int. J. Soc. Robot. 6, 45–65. doi:10.1007/s12369-013-0195-x
Wainer, J., Ferrari, E., Dautenhahn, K., and Robins, B. (2010). The effectiveness of using a robotics class to foster collaboration among groups of children with autism in an exploratory study. Pers. Ubiquit. Comput. 14, 445–455. doi:10.1007/s00779-009-0266-z
Welch, B. L. (1947). The generalization of student’s problem when several different population variances are involved. Biometrika 34, 28–35. doi:10.2307/2332510
Wong, C., and Kasari, C. (2012). Play and joint attention of children with autism in the preschool special education classroom. J. Autism Dev. Disord. 42, 2152–2161. doi:10.1007/s10803-012-1467-2
Keywords: autism, engagement, social robots, affective computing, human-robot interaction
Citation: Rudovic O, Lee J, Mascarell-Maricic L, Schuller BW and Picard RW (2017) Measuring Engagement in Robot-Assisted Autism Therapy: A Cross-Cultural Study. Front. Robot. AI 4:36. doi: 10.3389/frobt.2017.00036
Received: 01 February 2017; Accepted: 03 July 2017;
Published: 27 July 2017
Edited by:
Hatice Gunes, University of Cambridge, United KingdomReviewed by:
Hanan Salam, University of Pittsburgh Medical Center, United StatesCopyright: © 2017 Rudovic, Lee, Mascarell-Maricic, Schuller and Picard. This is an open-access article distributed under the terms of the Creative Commons Attribution License (CC BY). The use, distribution or reproduction in other forums is permitted, provided the original author(s) or licensor are credited and that the original publication in this journal is cited, in accordance with accepted academic practice. No use, distribution or reproduction is permitted which does not comply with these terms.
*Correspondence: Ognjen (Oggi) Rudovic, b3J1ZG92aWNAbWl0LmVkdQ==
Disclaimer: All claims expressed in this article are solely those of the authors and do not necessarily represent those of their affiliated organizations, or those of the publisher, the editors and the reviewers. Any product that may be evaluated in this article or claim that may be made by its manufacturer is not guaranteed or endorsed by the publisher.
Research integrity at Frontiers
Learn more about the work of our research integrity team to safeguard the quality of each article we publish.