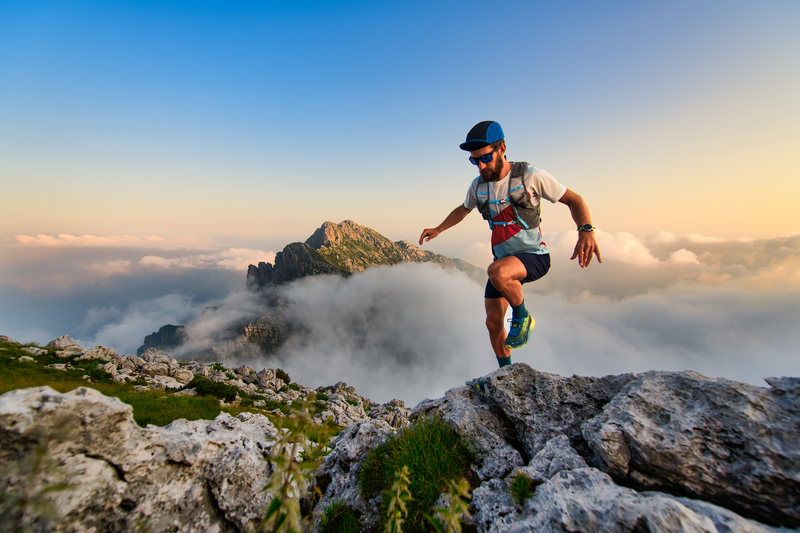
94% of researchers rate our articles as excellent or good
Learn more about the work of our research integrity team to safeguard the quality of each article we publish.
Find out more
ORIGINAL RESEARCH article
Front. Fish Sci. , 24 October 2024
Sec. Elasmobranch Science
Volume 2 - 2024 | https://doi.org/10.3389/frish.2024.1443923
Determining habitat associations and co-occurrence patterns among species can provide insight into their behavior and shared roles in ecosystem function. This study used a long-term gill-net survey to investigate habitat associations and co-occurrence patterns of three predatory fishes in a large estuarine complex in the northwestern Gulf of Mexico including two top predators alligator gar (Atractosteus spatula) and bull shark (Carcharhinus leucas) and a mesopredator Atlantic stingray (Hypanus sabina). Habitat suitability models were used to assess habitat conditions under which multiple conspecifics or species co-occurred. Environmental drivers of habitat suitability differed within and across species, but salinity and temperature were the most influential variables. Intraspecific co-occurrence of both alligator gars and bull sharks were primarily observed between conspecifics of similar size in low-salinity waters, but intraspecific co-occurrence was higher for alligator gars than for bull sharks. Although sample size was low, intraspecific co-occurrence of Atlantic stingrays was frequent in open-water regions of the estuary and consisted of both juveniles and adults. Interspecific co-occurrence between alligator gars and bull sharks was primarily observed in habitats commonly used by both species, particularly in low-salinity regions of the estuary. Even though there may be confounding effects of sampling gear, interspecific co-occurrence of Atlantic stingrays with either alligator gars or bull sharks was limited, suggesting there was limited overlap in estuarine habitats or environmental conditions used by Atlantic stingrays relative to the two other species investigated. These results clarify the influence of abiotic and biotic variables on the distribution and abundance of these species and suggest potential areas where interactions between them may shape their roles as predators.
Predators and their interactions with prey and other predator species play an important role in shaping the structure and function of estuarine ecosystems (1, 2). In turn, habitat loss, which is the most widespread and pressing threat to coastal and estuarine ecosystems, poses a threat to predator assemblages and the ecosystem services they offer (3). Recently, ecosystem-based fisheries management (EBFM) has been increasingly used to account for these losses and the interactive effects that can be ignored in species-specific management approaches, which is essential considering many marine and freshwater predators have declined across their range (3, 4). EBFM is a systematic approach of managing fisheries that considers the entirety of the natural resource system, including multispecies interactions, habitat requirements, anthropogenic impacts and environmental drivers that influence the broader community or ecosystem (5, 6). The identification of Essential Fish Habitats (EFHs), i.e., habitat necessary for breeding, foraging and growth of fishes, is a fundamental requirement for EBFM (7, 8). Identifying EFHs for multiple species in a given area offers a more comprehensive understanding of species interactions, thereby contributing to a more refined implementation of an ecosystem-based approach to management (9).
Estuaries are dynamic environments at the land–sea interface that are strongly affected by fluctuating environmental conditions across time and space (10). Estuaries encompass many distinctive habitat types, each supporting unique species assemblages (11, 12). Assemblage structure of fishes in an estuary is usually determined by the physiochemical tolerances and habitat associations of species, which can vary along salinity gradients within the estuary (13, 14). Salinity, as well as water temperature, dissolved oxygen, and other physical factors, can vary substantially on a seasonal basis in temperate and subtropical estuaries (15, 16), leading to spatiotemporal variability in assemblage structure. However, rapid changes may also occur on shorter time scales due to tidal cycles, shifts in freshwater inflow, and episodic climatic events (17). Habitat use by fishes in temperate and subtropical estuaries has been linked to fluctuating environmental conditions (18, 19). Consequently, delineating EFHs often requires long-term sampling strategies and modeling techniques that incorporate temporal and spatial variability of the physiochemical environment and species distributions.
Beyond understanding the physiochemical drivers of species abundance and distribution, biotic factors also play an important role in structuring estuarine fish assemblages. Competition exerts powerful selective forces in many contexts, with interactions among conspecifics (i.e., intraspecific competition) and/or other species (i.e., interspecific competition) shaping the abundance, distribution, and behavior of fishes (20). When resources are limited, niche segregation (e.g., food, space and time), may reduce competition within and across species promoting coexistence (20–22). Niche segregation is often measured in immediate returns such as alternative feeding strategies (23–25) and habitat use (26–28). However, such knowledge is often difficult to obtain considering long-term niche overlap across generations can lead to adaptations in these behaviors and reduce/eliminate competition, thereby compromising our ability to understand if differences in resource use are the product of evolution or temporary niche partitioning among co-occurring predators (29). Recently, an increase in the availability of co-occurrence data from long-term monitoring programs has renewed the interest of ecologists in the use of correlations and algorithms to infer species associations and the mechanism behind them (30–32). Inferring the potential for competitive interactions by delineating overlap in essential habitat of co-occurring predators can allow for some understanding of the biotic forces shaping ecosystem function in the absence of other data.
A common approach for modeling habitat uses in fishes has been based on the relationship between environmental variables and assemblage composition [random forest, RF (33)]. RF modeling methods used for predicting habitat suitability is highly accurate, and generates predictions based on machine learning techniques (34, 35). The model complexity of RF modeling can determine reliable and ecologically relevant species-specific habitat requirements, offering detailed management options for target species (36). RF modeling evaluates whether fishes are present near a habitat feature more frequently than anticipated based on the underlying distribution of the habitat feature in the environment. Because fish distributions are directly measured in their living environments, incorporating uncontrolled ecological factors, such as predation and competition, this approach delineates a species' realized niche, rather than the fundamental niche. Additionally, when habitat models operate at a spatial scale that is relevant to individuals within the environment, they can assess differences in habitat selection across age classes. While this approach has been applied to predict fish distribution based on abiotic variables (37, 38), less attention has been paid to environmental factors that lead to co-occurrence within and across species. Understanding when and where ecological interactions, such as predation and competition, are most likely to occur will elucidate the drivers and implications of co-occurrence and the potential for competitive interactions within and across species.
The present study aimed to construct habitat suitability models for the co-occurrence patterns of three estuarine predatory fishes using RF methods. Using data from a long-term sampling program in estuarine waters in a north Texas estuary, this study compares the habitat associations and co-occurrence patterns of three commonly occurring predators in the Galveston Bay Complex (GBC): alligator gar (Atractosteus spatula) and bull shark (Carcharhinus leucas) considered as top predators, and Atlantic stingray (Hypanus sabina) classified as mesopredator. The three species display different life history strategies and are generally assumed to primarily occur in different regions of the estuary along the salinity gradient, ranging from low [Atlantic stingray (39)] to high [alligator gar (40)] freshwater association, with bull sharks moving freely throughout estuaries and exhibiting size-specific affinities for certain salinities (41, 42). Ecosystems where predators co-occur across a gradient of environmental conditions provide an ideal study area to investigate the response of ecological communities to changing predator populations. Specific objectives of this study were to (1) determine what abiotic (i.e., environmental) and biotic (i.e., body size) factors correlate with conspecifics and species co-occurrence, and (2) delineate the extent of highly suitable habitat for conspecifics and species co-occurrence. Knowledge on the distribution patterns of co-occurring predatory fishes and the mechanisms driving these patterns are necessary for the successful conservation and management of predatory fishes and their surrounding environment. This information is important considering changes in abiotic and biotic factors attributed to climate change and human resource use in the region [e.g., (30)].
This study was conducted in the GBC, Texas located in the northwestern Gulf of Mexico from 1986 to 2018 (Figure 1). The GBC has four major sub-bays that are characterized by unique physiochemical properties (Figure 1). The complex also contains many smaller interconnecting sub-bays, rivers and streams around its periphery. The dominant freshwater sources for the GBC are inflows from the Trinity and San Jacinto Rivers (Upper Galveston Bay), and Chocolate Bayou (West Bay) (43). Galveston Island and Bolivar Peninsula separate the Gulf of Mexico from the GBC and most of the saltwater enters the system through the Bolivar Roads (Lower Galveston Bay) and San Luis Pass (West Bay). The tidal current through the narrow outlets is strong despite a limited tidal range (mean of 0.3 m) which leads to strong tidal pumping (44). The GBC is characterized by flat and shallow bottom (average 2 m), but deep man-made ship channels in the Lower Galveston Bay can reach a depth of up to 15 m (45). The waters of the GBC are usually turbid from inorganic suspended solids (46).
Figure 1. Location of the study area in the Galveston Bay Complex (GBC), Texas. Labels represent the four major sub-bays within the GBC used as strata in co-occurrence habitat suitability modeling. Arrows represent dominant freshwater and saltwater sources for the GBC.
Fishery-independent data were obtained from the Texas Parks and Wildlife Department (TPWD) long-term fishery-independent monitoring program (47). Gill-net sampling occurred seasonally in the GBC, with 45 gill-nets deployed in 10-week spring (April–June) and fall (September–November) sampling periods. Monofilament gill-nets (total length of 182.9 and 1.2 m deep comprised of four continuous 45.7 m panels with stretched mesh of 7.6, 10.2, 12.7, and 15.2 cm) were set perpendicular to the shoreline starting within 1 h before sunset and ending within 4 h after sunrise (mean soak time = 13.45 ± 1.5 h). Catch and environmental data (see more details below) were collected during gill-net sampling. To supplement these data, environmental data collected during monthly bag seine and otter trawl surveys were also used for this study, which occurred year-round, with half the monthly samples collected during the first half of the month, and the remaining collected the last 2 weeks of the month. While bag seines and gill nets were restricted to the vicinity of the shoreline, otter trawls took place in the open water region.
All organisms >5 mm TL caught in gill-nets were counted and measured (total length—TL or disc width—DW) to the nearest mm. Once all procedures were complete, each fish was monitored for signs of weakness or injury, and released if deemed healthy. Date, location, temperature (°C), salinity (psu), dissolved oxygen (mg l−1), turbidity (NTU), shallow water depth (m) and deep-water depth (m) were recorded at each sampling location while nets soaked. Water depths were recorded at the shoreline end of the net and the offshore end of the net. Abiotic parameters (i.e., water temperature, salinity, dissolved oxygen) were collected ~0.15 m below the water surface for both gill-nets, bag seines and otter trawls. A water sample was also collected for each net sample at the same depths as the other abiotic parameter and transported to TPWD laboratory for turbidity analysis for all sampling methods. Catch data from seine and trawl samples were not used in this analysis because they are not well-suited to quantify the abundance of all three study species, but environmental data were used from bag seine and otter trawl sampling, allowing for interpolating predictions across the GBC.
The total number of individuals of each species collected per gill-net set was calculated. For each gill-net set, sampling events in which at least two individuals were captured from the same species (i.e., intraspecific co-occurrence) were recorded as “1” while sampling events in which only one individual was captured were recorded as “0.” Similarly, interspecific co-occurrence events were recorded when at least two individuals from the three study species were captured in the same gill-net set. All data sets were sorted into decade (1980, 1990, 2000, and 2010's) and seasonal categories (Spring: April–June; Fall: September–November). A factor “sub-bay” was also created as a spatial variable, to represent the four major sub-bays within the GBC and were linked with all samples conducted within each sub-bay (Figure 1).
Random forest models were used to quantify the effect of abiotic factors on the likelihood of capturing at least two individuals of the same species during a sampling event (i.e., intraspecific co-occurrence). Predictor variables included temperature, salinity, dissolved oxygen, turbidity, shallow water depth, deep water depth, decade (categorical), season (categorical), and sub-bay (categorical). The variable fish size (i.e., TL and DW) was not used to create habitat suitability models, since interpolation was not possible using this variable, but additional statistical analysis (see statistical analysis procedure) was used to quantify the effects of size in predicting co-occurrence patterns. For each model, 500 unpruned classification trees were built, each using a random bootstrapped selection of data points with replacement. The default threshold value of 0.5 was used for all models (48). An identical analytical framework was used to quantify the effects of environmental factors leading to interspecific co-occurrence (i.e., when at least two individuals from different species were captured in the same sample).
Random forest is an ensemble collection of multiple decision trees that are constructed from bootstrap samples. The observations that are not part of the bootstrap sample are called out-of-bag (OOB, ~37% of the original data points each iteration). In random forest modeling, an accuracy test is estimated by OOB samples down through a tree as a form of cross-validation providing the prediction error. For the accuracy assessment, five methods were applied for each model: the overall average error rate, sensitivity (proportion of presences correctly classified), specificity (proportion of absences correctly classified), the true skill statistic (TSS), and the area under the receiver operating characteristic curve (AUC). TSS is calculated as follows: (sensitivity + specificity) – 1. TSS values range between 0 and 1 with values near 1 indicating nearly perfect model performance (49). Similarly, AUC values range from 0.5 to 1.0, with a value of 1.0 indicating perfect model (50). To determine the importance of each variable in predicting co-occurrence patterns, a variable importance metric is calculated using the Gini method. The Gini importance is directly derived from the Gini index on the resulting random forest trees (51). The Gini index measures the level of impurity/inequality of the samples assigned to a node based on a split at its parent. The purer a node is, the smaller the Gini value. Every time a split of a node is made using a certain feature attribute, the Gini value for the two descendant nodes is less than the parent node. A feature's Gini importance value in a single tree is then defined as the sum of the Gini index reduction (from parent to children) over all nodes in which the specific feature is used to split. The overall importance in the forest model is defined as the sum or the average of its importance value among all trees in the forest. To visualize the variable importance (see Figure 2), the Gini index was extracted for each predictor variable in each model. That value was then divided by the maximum value of that model, so that the most important variable was given a value of 1 and all the others were related to the most important variable.
Figure 2. Importance (standardized as a proportion of the maximum value) of each predictor variable for habitat suitability models for (A) intraspecific co-occurrence and (B) interspecific co-occurrence. Predictor variables are sorted by average importance across interactions.
Once all habitat suitability models were constructed (i.e., three intraspecific co-occurrence models and three interspecific co-occurrence models), abiotic data from seine and trawl sampling were used to predict the likelihood of co-occurrence within and across species. These new data points allowed for predictions of predator presence across the entire bay system since seine samples occurred along the shoreline, whereas trawl samples occurred in open waters throughout the bay. Interpolation of habitat use in open-water regions using models built with data exclusive to shoreline may introduce some uncertainty to these maps, such as differences in depth and access to structured habitats. However, these models apply to open regions considerably well since they are based primarily on abiotic quality variables and do not include structured habitats. To create habitat suitability maps for intra- and interspecific co-occurrence, predicted values were plotted in ArcGIS (ArcMap version 10.7.1) and projected to a standard coordinate system for spatial consistency (NAD 1983 UTM Zone 15N). Inverse distance weighted (IDW) interpolation was then used to create smoothed maps using a cell size of 100 x 100 m, a power of one, and a fixed search radius with a minimum of 15 points. The final habitat suitability maps (total of six maps) were then visualized using 10 bins of increasing probability of presence, each greater by 0.1.
Statistical analyses were performed using R [Version 4.0.2; (52)]. Normality and homoscedasticity of the data were tested using the Shapiro–Wilk and Levene's test, respectively. Data not normally distributed were compared using Kruskal–Wallis (H) test followed by a post-hoc Mann–Whitney U-test (Bonferroni corrected). For intraspecific co-occurrence analysis, significant differences in environmental variables and size among capture events (i.e., single individual capture events or >1 individual capture events) were compared using the Kruskal–Wallis (H) test. Chi-squared (X2) tests followed by post-hoc tests were used to test the null hypothesis that proportion of events with more than one individual captured did not differ significantly among decades, seasons and sub-bays. Results were used to determine the factors leading to intraspecific co-occurrence. A similar analytical framework was developed to identify the factors leading to interspecific co-occurrence.
From 1986 to 2018, 2,882 gill-nets were deployed in the GBC during which 4,381 alligator gars, 1,455 bull sharks, and 137 Atlantic stingrays were captured. Alligator gars ranged from 14 to 179 cm TL (mean ± SD = 92.4 ± 5.9 cm), bull sharks from 58 to 172 cm TL (mean ± SD = 105.3 ± 7.3 cm), and Atlantic stingrays from 12 to 67 cm DW (mean ± SD = 25.5 ± 8.7 cm). The average values of each abiotic variable used in the modeling and mapping procedures are detailed in Table 1. All environmental variables showed significant mean differences by decades expect deep water depth (H = 2.4, p = 0.49). Temperatures were higher in West Bay (H = 117.9, p < 0.01), and there was a seasonal shift in temperature with higher temperatures in the spring (H = 1, 325.9, p < 0.01). Similarly, salinity was higher in the fall than the spring (H = 281.2, p < 0.01) and displayed a northeast to southwest gradient from Upper Galveston Bay (lowest) to West Bay (highest) (H = 5,722.7, p < 0.01). Dissolved oxygen was higher in Upper Galveston Bay and lower in West Bay (H = 136.8, p < 0.01) with slightly higher dissolved oxygen during the fall (H = 378.1, p < 0.01). Turbidity was higher in East Bay and Upper Galveston Bay (H = 387.5, p < 0.01) with higher turbidity during the spring (H = 409.4, p < 0.01). Water depths (shallow and deep depths) were deeper in Lower Galveston Bay followed by Upper Galveston Bay, East Bay, and West Bay for both seasons (H test, all p < 0.05).
Table 1. Average water temperature (±SD°C), average salinity (±SD psu), average dissolved oxygen content (±SD mg L−1), average turbidity (±SD NTU), average shallow depth (±SD m), and average deep depth (±SD m) across season within each stratum. Abiotic variables in each stratum and season are based on gill-net, seine, and trawl collections.
Intraspecific co-occurrence in gill-net sets was highest for alligator gar (58.4% of capture events) followed by bull shark (50.7% of capture events) and Atlantic stingray (12.6% of capture events). Due to the high degree of intraspecific co-occurrence of alligator gars, the sensitivity (0.75) was higher than the specificity (0.50) for the random forest model (Table 2). The most important variable influencing the probability of alligator gar co-occurrence was salinity, but temperature also showed a high importance score (Figure 2). Individual alligator gars were caught at higher salinities (mean ± SE = 15.2 ± 0.4 psu, H = 41.0, p < 0.01) and deeper shallow-water depths (0.3 ± 0.1 m, H = 33.2, p < 0.01) than alligator gar caught with at least one conspecific (12.0 ± 0.3 psu, 0.2 ± 0.1 m, respectively). Intraspecific co-occurrence was more frequent when alligator gars were smaller (89.7 ± 6.2 cm vs. 96.2 ± 11.1 cm, H = 26.0, p < 0.01). Intraspecific co-occurrence of alligator gars varied spatially and temporally. The proportion of capture events with more than one alligator gar significantly varied across decades, with the highest frequency of intraspecific co-occurrence in the 2010's (, Supplementary Table 1). Intraspecific co-occurrence of alligator gars for individual gill-net sets was significantly higher during the spring (60.6% ± 1.5) than the fall . The frequency of alligator gar co-occurrence in capture events was lowest in Lower Galveston Bay (49.5% ± 1.3) and highest in East Bay ( 68.8, p < 0.01, Supplementary Table 1). The habitat suitability map for alligator gar intraspecific co-occurrence indicated generally low probability of co-occurrence throughout the GBC, with highest probabilities of co-occurrence in Upper Galveston Bay and East Bay shorelines (Figure 3A).
Table 2. Accuracy metrics from random forest models for intra- and interspecific co-occurrences. The average error rate of the model is based on out-of-bag (testing) data, sensitivity is the proportion of presences correctly classified, and specificity is the proportion of absences correctly classified. True skill statistic (TSS = sensitivity + specificity – 1) and area under the receiver operating characteristic curve (AUC = relationship between true positive rate and false positive rate) are measures of model predictive accuracy.
Figure 3. Intraspecific co-occurrence habitat suitability for: (A) alligator gar, (B) bull shark, and (C) Atlantic stingray, with warm colors depicting high probability of intraspecific co-occurrence and cold depicting low probability of intraspecific co-occurrence.
Similar to alligator gar, the intraspecific co-occurrence of bull sharks had higher sensitivity (0.63) than specificity (0.54). Bull shark intraspecific model had a lower AUC (0.62), a lower TSS (0.17), but a higher error rate (0.41) compared to the model predicting the intraspecific co-occurrence of alligator gars (error rate = 0.35, TSS = 0.25, AUC = 0.71, Table 2), meaning that co-occurrence absences were predicted less accurately for the bull shark model. Temperature and salinity were the two most important variables predicting bull shark co-occurrence (Figure 2). Solitary bull sharks were caught in significantly different environmental conditions than capture events that resulted in more than one individual. Individual bull sharks were caught at cooler temperatures (mean ± SE = 27.5 ± 0.2°C, H = 12.7, p < 0.01) and higher salinities (17.4 ± 0.5 psu, H = 23.7, p < 0.01) than bull sharks caught with at least one conspecific (28.4 ± 0.1°C and 14.2 ± 0.5 psu, respectively). There was no significant interaction between the likelihood of capturing more than one bull shark and bull shark size (H = 0.3, p = 0.6). Intraspecific co-occurrence increased across decades, with the highest frequency of intraspecific co-occurrence in the 2010's (55.8% ± 1.6 of capture events, 0.01, Supplementary Table 1) and was slightly higher in the spring (51.0 % ± 0.7) in comparison to the fall . The proportion of capture events with more than one bull shark was lowest in West Bay (33.0% ± 0.6) and Lower Galveston Bay (33.0% ± 0.7), and highest in East Bay and Upper Galveston Bay (, Supplementary Table 1). Overall, the probability of bull shark intraspecific co-occurrence was less pronounced than that of alligator gar throughout the GBC. However, the probability of bull shark intraspecific co-occurrence was highest along the shoreline closest to the mouth of the Trinity River in Upper Galveston Bay (Figure 3B).
Atlantic stingray had the lowest rate of intraspecific co-occurrence in the gill-nets resulting in higher specificity (0.98) than sensitivity (0.05) for the random forest model (Table 2). The model predicting the intraspecific co-occurrence of Atlantic stingrays also showed low accuracy for the average error rate (0.14), the TSS (0.04), and the AUC (0.58) (Table 2). The most important variable predicting the co-occurrence of Atlantic stingrays was salinity (Figure 2). Individual Atlantic stingrays were caught in significantly lower salinities (mean ± SE = 19.7 ± 0.8 psu) than Atlantic stingrays caught with at least one conspecific (24.4 ± 2.2 psu, H = 4.0, p < 0.05). The size of Atlantic stingray did not affect intraspecific co-occurrence (H = 1.8, p = 0.18). There was a significant decadal difference on the co-occurrence of Atlantic stingrays with higher proportions observed in the 1990's compared to the 1980's and 2000's (, Supplementary Table 1). The proportion of capture events with more than one individual was significantly higher during the spring (12.9% ± 1.4) than the fall . Intraspecific co-occurrence was highest in West Bay , Supplementary Table 1). Atlantic stingray probability of intraspecific co-occurrence was relatively high in open-water regions through the GBC, but it was reduced in East Bay and along the shoreline (Figure 3C).
When at least one alligator gar or at least one bull shark was captured, the proportion of interspecific co-occurrence of these two species across all gill-nets sets was 19.0%. In contrast, Atlantic stingray experienced lower instances of interspecific co-occurrence with alligator gar (3.8%) and bull shark (4.1%). The observed co-occurrence between alligator gars and bull sharks resulted in higher specificity (0.95) than sensitivity (0.29) for the random forest model (Table 2). The most important variable predicting the co-occurrence of alligator gars and bull sharks was temperature and had a higher importance score compared to the next highest variable, salinity (Figure 2). Alligator gars and bull sharks were caught together in warmer water temperatures (mean ± SE = 27.7 ± 0.2°C, H = 58.94, p < 0.01), lower salinities (12.7 ± 0.5 psu, H = 15.8, p < 0.01) and higher turbidities (25.2 ± 1.5 mg L−1, H = 6.6, p < 0.05) compared to homospecific events (i.e., when only one species was caught; 25.5 ± 0.1°C, 14.9 ± 0.3 psu, 21.4 ± 1.1 mg L−1, respectively). Among captured bull sharks, mean TL was higher during homospecific capture events (107.1 ± 11.1 cm) and smaller during heterospecific capture events (103.3 ± 9.1 cm, H = 2.8, p < 0.05). Co-occurrence of alligator gars and bull sharks varied spatially and temporally. Interspecific co-occurrence of alligator gars and bull sharks significantly increased across decades, with the highest frequency of interspecific co-occurrence in the 2010's (27.2% ± 1.3 of capture events,, Supplementary Table 1). Interspecific co-occurrence was also higher in the spring (27.0% ± 1.0 of capture events) than the fall (17.8% ± 0.7,, p < 0.01). Interspecific co-occurrence was higher in East Bay (18.1% ± 1.4) and Upper Galveston Bay (14.4% ± 1.6), and lower in West Bay (7.0% ± 0.8) and Lower Galveston Bay (, Supplementary Table 1) and this observation was supported by the habitat suitability map (Figure 4A). Upper Galveston Bay and East Bay represent together 66.8 and 59.3% of capture events for alligator gar and bull shark, potentially explaining higher encounter rates for these two species in these regions.
Figure 4. Interspecific co-occurrence habitat suitability for: (A) alligator gar and bull shark, (B) alligator gar and Atlantic stingray, and (C) bull shark and Atlantic stingray, with warm colors depicting high probability of interspecific co-occurrence and cold depicting low probability of interspecific co-occurrence.
The frequency of events during which both alligator gars and Atlantic stingrays were captured was low, resulting in higher specificity (0.86) than sensitivity (0.17) for the random forest model. The alligator gar and Atlantic stingray interspecific model had also a lower AUC (0.54), a lower TSS (0.03), and a lower error rate (0.04) compared to the model predicting the interspecific co-occurrence of alligator gars and bull sharks (error rate = 0.17, TSS = 0.24, AUC = 0.76, Table 2), suggesting this model was less robust. The low predictive accuracy may also be related to the lack of data because the captures of both alligator gars and Atlantic stingrays on any one occasion was rare. Temperature was the most important variable influencing the co-occurrence of alligator gars and Atlantic stingrays, but it was not dominant. Salinity, dissolved oxygen and turbidity had similar importance scores (Figure 2). Alligator gars and Atlantic stingrays were caught together at increased water temperatures (26.9 ± 0.4°C) compared to homospecific events (25.6 ± 0.1°C, H = 4.2, p < 0.05). Alligator gar and Atlantic stingray sizes did not vary between homospecific and heterospecific capture events (H = 0.2, p = 0.7 and H = 0.8, p = 0.4, respectively). Interspecific co-occurrence exhibited significant changes across decades with highest frequencies in the 1990's (1.8% ± 0.4) and 2010's , Supplementary Table 1) and was higher in the spring (2.8% ± 0.6) than the fall . Interspecific co-occurrence of alligator gars and Atlantic stingrays showed significant differences across sub-bays , and was highest in West Bay (2.5% ± 0.1) and lowest in Lower Galveston Bay (0.4% ± 0.2, Supplementary Table 1). The habitat suitability map for alligator gar and Atlantic stingray co-occurrence showed extremely low probability of interspecific co-occurrence in all sub-bays (Figure 4B).
Capture events with at least one bull shark and one Atlantic stingray were low resulting in higher specificity (0.88) than sensitivity (0.12) for the model. The average error rate, the TSS and the AUC were respectively equal to 0.06, 0.04, and 0.55 for the Atlantic stingray and bull shark co-occurrence model. The most important variable predicting the co-occurrence of these two species was salinity followed by turbidity (Figure 2). Bull shark and Atlantic stingray were caught together at higher salinities (17.2 ± 1.7 psu, H = 4.2, p < 0.05) and lower turbidities (23.7 ± 4.8 NTU, H = 3.9, p < 0.05) compared to homospecific events (14.9 ± 0.3 psu, 29.6 ± 0.8 NTU, respectively). Interspecific co-occurrence was more frequent when bull sharks were larger (112.7 ± 9.5 cm vs. 105.1 ± 7.4 cm, H = 6.7, p < 0.05). Interspecific co-occurrence of bull sharks and Atlantic stingrays did not vary across decades (, Supplementary Table 1), but it was higher in the spring (2.8% ± 0.3 of capture events) than the fall . The proportion of capture events with at least one bull shark and one Atlantic stingray significantly differed among sub-bays and was highest in West Bay (1.6% ± 0.9) and lower in Lower Galveston bay (, Supplementary Table 1). The probability of co-occurrence of these two species was extremely low throughout the GBC, but slightly higher in the East Bay (Figure 4C).
Predators play important roles within food webs, however their co-occurrence with other predators can lead to shifts in behavior and trophic dynamics, thereby reshaping these roles and the response of prey species (1, 2). In this study, we used long-term gill-net data to better understand the co-occurrence patterns of two top predators (i.e., alligator gar and bull shark) and one mesopredator (i.e., Atlantic stingray) in a Texas estuary. By using habitat suitability models, we were able to examine the conditions under which alligator gar, bull shark and Atlantic stingray co-occur and potentially interact. The influence of environmental variables on the co-occurrence within and across species differed, but temperature and salinity were generally the most important drivers of these interactions. The regions in which intraspecific co-occurrence was observed most frequently aligned with the most suitable habitat. Intraspecific co-occurrence of alligator gars and bull sharks was largely restricted to regions characterized by low salinity waters, while Atlantic stingray intraspecific occurrence was higher in open-water regions throughout the GBC. Similarly, interspecific co-occurrence was largely attributed to habitat associations shared by co-occurring species. Alligator gar and bull shark co-occurrence was restricted to less saline regions, likely due to alligator gar osmoregulatory restrictions. Comparatively, interspecific co-occurrence for the Atlantic stingray was infrequent, which may be an artifact of sampling efficacy, or suggests movement away from habitat used by alligator gars and bull sharks, the latter of which feed on stingrays (53). The result of this study contributes toward our understanding of what drives concurrent distribution patterns of these predators, supporting ecosystem-based management strategies.
The presence of multiple conspecifics can lead to variations in predator behavior and ultimately alter their ecological roles (54). Determining regions, or environmental conditions that support multiple conspecifics can have implications for understanding ecosystem function and, managing systems to help maintain this function in light of human uses (e.g., fisheries, development). Habitat suitability maps of intraspecific co-occurrence in the GBC revealed major differences in the abundance and distribution of conspecifics for the three study species. Alligator gar intraspecific co-occurrence was present throughout the system, but highly suitable habitat appeared to be restricted to Upper Galveston Bay and East Bay, which receive more freshwater inflow than the other regions of the GBC. Bull shark intraspecific co-occurrence was also largely restricted to Upper Galveston Bay and East Bay, but intraspecific co-occurrence was more notable for alligator gars than for bull sharks. Overall, habitat suitability for the intraspecific co-occurrence of alligator gars and bull sharks expectantly coincided with suitable habitats previously described for these species (37). Interestingly, Atlantic stingray showed high habitat suitability in open-water regions throughout the GBC, and lower probabilities of intraspecific co-occurrence in East Bay. Although the result of accuracy metrics from the model predicting the intraspecific co-occurrence of Atlantic stingrays showed relatively good prediction accuracy, the low number of samples limits strong conclusions.
In estuaries like the GBC, salinity is often an important factor determining habitat suitability as it can vary dramatically with tidal state and inflow from rivers (55, 56). The variability in salinity in the GBC is attributed to the freshwater inflow from the Trinity and San Jacinto Rivers, leading to lower average salinities in Upper Galveston Bay and East Bay compared to the rest of the ecosystem (see Table 1). The most important variable predicting alligator gar co-occurrence was salinity, and co-occurrence was more frequent during the spring, when salinities were significantly lower. During spring flooding, large spawning aggregations of adult gars have been observed in shallow (<1 m water depth) areas with groups containing one large female accompanied by multiple smaller males (57–60). Wintering aggregations have also been reported for adult alligator gars in an apparent response to seasonal changes in temperature and hydrology (61). However, in our study, when intraspecific co-occurrence was reported the average size of alligator gars were 89.7 ± 6.2 cm, which is well below recorded length at maturity for both males and females (62). Juvenile alligator gars generally move less, use more off-channel habitats, and are less tolerant to higher salinities than adults (63–65). Therefore, expansive low salinity waters during the spring may allow for an increase in suitable habitats throughout the ecosystem for alligator gar. Adult alligator gars may be able to expand their habitat use throughout the entire ecosystem due to their lower surface area:volume reducing osmoregulatory costs of inhabiting higher salinity waters (e.g., Central and West Bays). Comparatively, juvenile alligator gars may remain in low salinity regions within the GBC, potentially explaining higher conspecific encounter rates for juveniles in these areas.
Ontogenetic shifts in movements and habitat use are common in estuarine fishes, therefore size-specific differences in alligator gar was not unexpected (66, 67). Bull sharks are one of few euryhaline shark species that inhabit estuaries and freshwater rivers throughout their range (68). Individuals found in low salinity habitats are typically juveniles, which use shallow estuarine areas as nursery grounds because they provide refuge from predators (42, 69). The average total length of bull sharks collected by gill-nets was 105.3 ± 7.3 cm suggesting that the majority of sharks caught during the study were juveniles based on total length (70, 71). Habitat suitability was highest in Upper Galveston Bay and East Bay, suggesting that intraspecific co-occurrence for bull sharks primarily occurs in low-salinity waters in the GBC where they may avoid predators (72, 73). However, the most important variable influencing bull shark co-occurrence was temperature. Temperature has previously been identified as the most important physical driver of bull shark abundance and distribution along the northwest Gulf of Mexico, with bull sharks presence positively correlated with temperatures above 25°C (74). Another study in the region also determined that bull shark co-occurrence was influenced by temperature, with bull sharks caught together at warmer temperatures (30). Recent work in the northern Gulf of Mexico indicates that warming waters attributed to climate change has increased habitat suitability of juvenile bull sharks (75) thus our findings are not unexpected, particularly the observed increase in intraspecific interactions from the 1980 to 2010's considering Gulf of Mexico waters continue to warm in response to climate change. In the GBC, colder conditions occur more frequently during episodic freshwater inflow events at the base of the Trinity River (Upper Galveston Bay) in the fall, which may force juvenile bull sharks to find refuge in warmer West Bay waters, which was likely responsible for seasonal differences in intraspecific co-occurrence.
Atlantic stingrays experienced higher habitat suitability throughout the GBC in comparison to alligator gars and bull sharks, suggesting that the entire complex may provide suitable habitat for this species. However, we are currently limited in our conclusions by the small number of individuals collected in the gill-nets. Habitat selection by batoids is primarily determined by substrata type and water temperature, with unconsolidated sediment substrata (i.e., mud and sand) and warm temperature favoring the presence of individuals (76). Substrata types are not recorded during gill-net sampling, but the GBC is mainly characterized by mud and sand, with the exception of Lower Galveston Bay, East Bay and West Bay that contain both oyster reefs and seagrass meadows (77, 78). This difference in substrata type may be responsible for the higher degree of intraspecific co-occurrence in West Bay, as habitat selection for stingrays is positively related to vegetated-sand habitat in other systems (76, 79, 80). However, this remains to be tested as Atlantic stingrays are also frequently caught outside of West Bay using other gear types. Temperature was the third most important variable in predicting Atlantic stingray co-occurrence after salinity and turbidity. Atlantic stingrays are found to migrate away from cold shallow waters and utilize warmer deeper waters during winter (81, 82). The lower score of temperature in addition to the absence of significant differences in water temperature between events could potentially be explained by the inability of gill-nets to collect this species in deeper or more topographically complex areas during the fall. It is therefore possible that Atlantic stingrays are present throughout the year in all regions but not collected using certain gear types, as suggested by Ramsden et al. (82). Size did not affect Atlantic stingray co-occurrence and the average disc width of individuals collected in this data set was 25.8 ± 8.9 cm potentially because co-occurrence for this species comprised both juveniles and adults based on disc width length (39). Juvenile dasyatids are known to use shallow sand flats to forage for prey and to avoid predators (83–85). With the increase of body size, predation risk is lowered and larger individuals can then exploit deeper waters for safety (86). However, when conditions are physiologically, and behaviorally advantageous conspecifics may periodically aggregate in specific areas. Similar aggregations have been observed in sympatric batoid communities with individuals feeding and resting in groups both with conspecifics and with the other species (80, 87). It is still unclear what shapes intraspecific co-occurrence of Atlantic stingrays in the GBC, but environmental conditions certainly play a significant role, which is important considering the expected changes in temperature and salinity attributed to natural disturbances and persistent human impacts in the system.
In addition to interacting with their conspecifics, estuarine predators also interact with other species. Understanding species interactions and how they are shaped by co-occurrence patterns provides insight into their individual and shared roles in ecosystem function (88, 89). In the GBC, the three study predators are not homogenously distributed throughout the estuary, and potential interactions between these species exhibited spatiotemporal variability. Co-occurrence of gars and bull sharks were primarily restricted to low salinity waters, typically in Upper Galveston Bay and East Bay. Suitable habitat for the interspecific co-occurrence of Atlantic stingrays with either alligator gars or bull sharks was low throughout the GBC, with the exception of discrete patches in East Bay. The spatial separation of these predators across much of the estuary suggests that competition for and compensatory predation on shared prey (i.e., Atlantic croaker [Micropogonias undulatus], gulf menhaden [Brevoortia patronus], brown shrimp [Farfantepenaeus aztecus], and white shrimp [Lito penaeus setiferus]) is heterogenous across the ecosystem. However, our data demonstrated that East Bay was relatively important to the three studied species and may offer an important area of EFH. In areas where co-occurrence between species was higher (i.e., Upper Galveston Bay and East Bay), the studied predators may play a larger role in shaping ecosystem function compared to areas where interactions were less abundant, such as West Bay where other predators may play a more important role (e.g., blacktip shark [Carcharhinus limbatus], gafftopsail catfish [Bagre marinus]).
Among the environmental factors considered, salinity and temperature played an especially important role in determining interspecific co-occurrence. Bull shark abundance has been found to be associated with low salinity waters, with freshwater disproportionately used by smaller juveniles and avoided by larger juveniles (64, 90). Interestingly, bull sharks were significantly larger when captured with at least one Atlantic stingray suggesting that the use of more saline waters by larger juvenile bull sharks increased the likelihood of encountering Atlantic stingrays, which could lead to predatory events. In Texas, bull shark prey upon stingrays (53, 91, 92). Thus, Atlantic stingray distributions could be driven by anti-predatory behavior, and our results support this hypothesis. Atlantic stingrays reduced the use of low-salinity areas, where larger predators were more abundant, in favor of shallow flats (i.e., West Bay) where the risk of encounter is lower. By using habitats where predator abundances are low, batoids can reduce their predation risk (93, 94). Bull sharks exhibited significantly lower average total length when captured with at least one alligator gar, potentially due to the preference exhibited by smaller bull sharks for low-salinity habitats [i.e., more suitable for alligator gars (90)]. In the GBC interspecific co-occurrence between alligator gars and bull sharks has increased over the last 30 years with significantly higher co-occurrence in the 2010's, which may be related to habitat quality and environmental conditions. Heavy rainfall events have become more frequent since the middle of the last century resulting in prolonged periods of low salinities water in estuaries (17). Extreme precipitation in Texas waters may have increased the availability of favorable habitats for alligator gars and bull sharks, potentially explaining increased co-occurrence between these species in recent decades. Human population growth in coastal Texas has led to stressors on natural variation in freshwater inflow due to increased demand for freshwater resources (95). Reduced freshwater inflow could predictably increase the use of riverine habitats by juvenile bull sharks in search of low-salinity waters (30, 73). Therefore, human induced change in hydrology may also explain the increased co-occurrence of alligator gars and juvenile bull sharks in low–moderate salinity habitats. Additionally, because temperature was the most important predictor variable, increased temperatures related to climate shift may also lead to an increase in spatial overlap between these two species (96).
Coexisting predators can exhibit depth-related habitat partitioning, which is often related to differences in trophic ecology between the species (97). Atlantic stingrays feed upon small benthic crustaceans, polychaetes and invertebrates associated with seagrass beds (39, 98). Diet of Atlantic stingray differ with those of alligator gars and bull sharks, which both prey at higher trophic positions (99), potentially explaining limited co-occurrence between Atlantic stingray and the two other predators. Interestingly, alligator gars and bull sharks exhibit significant dietary overlap in the northwestern Gulf of Mexico (100). Potential shared prey of alligator gars and bull sharks (i.e., Atlantic croaker, gulf menhaden) showed dramatic differences in their probability of presence between seasons in the GBC (37). Seasonality in prey populations could therefore affect interactions between predators, particularly in the spring when Atlantic croaker and gulf menhaden are abundant, resulting in significantly higher interspecific co-occurrence between these two apex predators. In Texas estuaries, while both alligator gars and bull sharks predominantly fed on mugilids, they are able to adjust their diets to local fitness trade-offs based on prey availability, potentially facilitating their coexistence (100).
Results of this study elucidate the habitat associations and coexistence patterns of alligator gars, bull sharks and Atlantic stingrays. This information can be beneficial for the management of multispecies systems by identifying habitats worth of protection if they are deemed essential for a variety of species. Our results suggest that temperature and salinity are among the most important environmental variables in predicting both intra- and interspecific co-occurrence, and the habitats in which conspecifics co-occurred most frequently coincided with habitat suitability of the related species. Similarly, the habitats in which species co-occurred generally aligned with the habitat associations that were shared by both species. However, complex interactions with other individuals in their surroundings are also important to consider. Additional work should explicitly test for and distinguish between the relative importance of abiotic process (e.g., freshwater runoff, extreme temperature events) and biological processes (e.g., competition and predation) in regulating the co-occurrence of estuarine fishes.
The raw data supporting the conclusions of this article will be made available by the authors, without undue reservation.
The animal study was approved by Texas Parks and Wildlife Department. The study was conducted in accordance with the local legislation and institutional requirements.
PF: Conceptualization, Formal analysis, Methodology, Writing – original draft, Writing – review & editing. CJ: Data curation, Methodology, Writing – review & editing. PM: Conceptualization, Methodology, Writing – review & editing. JR: Conceptualization, Methodology, Writing – review & editing. RW: Conceptualization, Funding acquisition, Methodology, Supervision, Writing – review & editing.
The author(s) declare financial support was received for the research, authorship, and/or publication of this article. This work was funded by the U.S. Fish and Wildlife Service through the Texas Parks and Wildlife Department under the State Wildlife Grant Program (Grant #CA-0002944 to RW).
All data were provided by TPWD Coastal Fisheries Division and we thank the many TPWD staff that were involved with field collections and data management. The authors also thank Mariah Livernois for assistance with the data analyses.
The authors declare that the research was conducted in the absence of any commercial or financial relationships that could be construed as a potential conflict of interest.
All claims expressed in this article are solely those of the authors and do not necessarily represent those of their affiliated organizations, or those of the publisher, the editors and the reviewers. Any product that may be evaluated in this article, or claim that may be made by its manufacturer, is not guaranteed or endorsed by the publisher.
The Supplementary Material for this article can be found online at: https://www.frontiersin.org/articles/10.3389/frish.2024.1443923/full#supplementary-material
1. Heithaus MR, Frid A, Wirsing AJ, Worm B. Predicting ecological consequences of marine top predator declines. Trends Ecol Evol. (2008) 23:202–10. doi: 10.1016/j.tree.2008.01.003
2. Heithaus MR, Frid A, Vaudo JJ, Worm B, Wirsing AJ. Unraveling the ecological importance of elasmobranchs. In: Carrier JC, Musick JA, Heithaus MR, editors. Sharks and Their Relatives II: Biodiversity, Adaptative Physiology, and Conservation. Boca Raton, FL: CRC Press (2010). p. 627–54.
3. Lotze HK, Lenihan HS, Bourque BJ, Bradbury RH, Cooke RG, Kay MC, et al. Depletion, degradation, and recovery potential of estuaries and coastal seas. Science. (2006) 312:1806–9. doi: 10.1126/science.1128035
4. Kroodsma DA, Mayorga J, Hochberg T, Miller NA, Boerder K, Ferretti F, et al. Tracking the global footprint of fisheries. Science. (2018) 359:904–8. doi: 10.1126/science.aao5646
5. Plagányi ÉE, Punt AE, Hillary R, Morello EB, Thébaud O, Hutton T, et al. Multispecies fisheries management and conservation: tactical applications using models of intermediate complexity. Fish Fisher. (2014) 15:1–22. doi: 10.1111/j.1467-2979.2012.00488.x
6. Craig JK, Link JS. It is past time to use ecosystem models tactically to support ecosystem-based fisheries management: case studies using Ecopath with Ecosim in an operational management context. Fish Fisher. (2023) 24:381–406. doi: 10.1111/faf.12733
7. Marasco RJ, Goodman D, Grimes CB, Lawson PW, Punt AE, Quinn II, et al. Ecosystem-based fisheries management: some practical suggestions. Can J Fisher Aquat Sci. (2007) 64:928–39. doi: 10.1139/f07-062
8. Link JS, Bundy A, Overholtz WJ, Shackell N, Manderson J, Duplisea D, et al. Ecosystem-based fisheries management in the Northwest Atlantic. Fish Fisher. (2011) 12:152–70. doi: 10.1111/j.1467-2979.2011.00411.x
9. Bellido JM, Brown AM, Valavanis VD, Giráldez A, Pierce GJ, Iglesias M, et al. Identifying essential fish habitat for small pelagic species in Spanish Mediterranean waters. Essent Fish Habit Map Mediter. (2008) 13:171–84. doi: 10.1007/978-1-4020-9141-4_13
10. James NC, Cowley PD, Whitfield AK, Lamberth SJ. Fish communities in temporarily open/closed estuaries from the warm-and cool-temperate regions of South Africa: a review. Rev Fish Biol Fish. (2007) 17:565–80. doi: 10.1007/s11160-007-9057-7
11. Pihl L, Cattrijsse A, Codling I, Mathieson S, McLusky D, Roberts C. Habitat use by fishes in estuaries and other brackish areas. Fish Estuar. (2002) 2:10–53. doi: 10.1002/9780470995228.ch2
12. McLusky DS, Elliott M. The Estuarine Ecosystem: Ecology, Threats and Management. Oxford: OUP Oxford (2004).
13. Martino EJ, Able KW. Fish assemblages across the marine to low salinity transition zone of a temperate estuary. Estuar Coast Shelf Sci. (2003) 56:969–87. doi: 10.1016/S0272-7714(02)00305-0
14. Allen LG, Pondella DJ, Horn MH. The Ecology of Marine Fishes: California and Adjacent Waters. Berkeley, CA: University of California Press (2006).
15. Sutherland DA, O'Neill MA. Hydrographic and dissolved oxygen variability in a seasonal Pacific Northwest estuary. Estuar Coast Shelf Sci. (2016) 172:47–59. doi: 10.1016/j.ecss.2016.01.042
16. Hoeksema SD, Chuwen BM, Tweedley JR, Potter IC. Factors influencing marked variations in the frequency and timing of bar breaching and salinity and oxygen regimes among normally-closed estuaries. Estuar Coast Shelf Sci. (2018) 208:205–18. doi: 10.1016/j.ecss.2018.04.010
17. Wetz MS, Yoskowitz DW. An “extreme” future for estuaries? Effects of extreme climatic events on estuarine water quality and ecology. Mar Pollut Bullet. (2013) 69:7–18. doi: 10.1016/j.marpolbul.2013.01.020
18. Kupschus S, Tremain D. Associations between fish assemblages and environmental factors in nearshore habitats of a subtropical estuary. J Fish Biol. (2001) 58:1383–403. doi: 10.1111/j.1095-8649.2001.tb02294.x
19. Mattone C, Bradley M, Barnett A, Konovalov DA, Sheaves M. Environmental conditions constrain nursery habitat value in Australian sub-tropical estuaries. Mar Environ Res. (2022) 175:105568. doi: 10.1016/j.marenvres.2022.105568
21. Pianka ER. Niche overlap and diffuse competition. Proc Natl Acad Sci USA. (1974) 71:2141–5. doi: 10.1073/pnas.71.5.2141
22. Schoener TW. Resource partitioning in ecological communities: research on how similar species divide resources helps reveal the natural regulation of species diversity. Science. (1974) 185:27–39. doi: 10.1126/science.185.4145.27
23. Papastamatiou YP, Wetherbee BM, Lowe CG, Crow GL. Distribution and diet of four species of carcharhinid shark in the Hawaiian Islands: evidence for resource partitioning and competitive exclusion. Mar Ecol Progr Ser. (2006) 320:239–51. doi: 10.3354/meps320239
24. Matley J, Heupel M, Fisk A, Simpfendorfer C, Tobin A. Measuring niche overlap between co-occurring Plectropomus spp. using acoustic telemetry and stable isotopes. Mar Freshw Res. (2016) 68:1468–78. doi: 10.1071/MF16120
25. Matich P, Kiszka JJ, Mourier J, Planes S, Heithaus MR. Species co-occurrence affects the trophic interactions of two juvenile reef shark species in tropical lagoon nurseries in Moorea (French Polynesia). Mar Environ Res. (2017) 127:84–91. doi: 10.1016/j.marenvres.2017.03.010
26. Lea JS, Humphries NE, von Brandis RG, Clarke CR, Sims DW. Acoustic telemetry and network analysis reveal the space use of multiple reef predators and enhance marine protected area design. Proc Royal Soc B Biol Sci. (2016) 283:20160717. doi: 10.1098/rspb.2016.0717
27. Matley J, Tobin A, Lédée E, Heupel M, Simpfendorfer C. Contrasting patterns of vertical and horizontal space use of two exploited and sympatric coral reef fish. Mar Biol. (2016) 163:1–12. doi: 10.1007/s00227-016-3023-7
28. Heupel MR, Lédée EJ, Simpfendorfer CA. Telemetry reveals spatial separation of co-occurring reef sharks. Mar Ecol Progr Ser. (2018) 589:179–92. doi: 10.3354/meps12423
29. Matich P, Bizzarro JJ, Shipley ON. Are stable isotope ratios suitable for describing niche partitioning and individual specialization? Ecol Appl. (2021) 31:e02392. doi: 10.1002/eap.2392
30. Matich P, Mohan JA, Plumlee JD, TinHan T, Wells RD, Fisher M. Factors shaping the co-occurrence of two juvenile shark species along the Texas Gulf Coast. Mar Biol. (2017) 164:1–16. doi: 10.1007/s00227-017-3173-2
31. Livernois MC, Powers SP, Albins MA, Mareska JF. Habitat associations and co-occurrence patterns of two estuarine-dependent predatory fishes. Mar Coast Fisher. (2020) 12:64–77. doi: 10.1002/mcf2.10104
32. Schumann DA, Colvin ME, Miranda LE, Jones-Farrand DT. Occurrence and co-occurrence patterns of gar in river-floodplain habitats: methods to leverage species coexistence to benefit distributional models. North Am J Fisher Manag. (2020) 40:622–37. doi: 10.1002/nafm.10402
33. Brownscombe JW, Midwood JD, Cooke SJ. Modeling fish habitat: model tuning, fit metrics, and applications. Aquat Sci. (2021) 83:1–14. doi: 10.1007/s00027-021-00797-5
34. Garzon MB, Blazek R, Neteler M, De Dios RS, Ollero HS, Furlanello C. Predicting habitat suitability with machine learning models: the potential area of Pinus sylvestris L. in the Iberian Peninsula. Ecol Model. (2006) 197:383–93. doi: 10.1016/j.ecolmodel.2006.03.015
35. Lawler JJ, White D, Neilson RP, Blaustein AR. Predicting climate-induced range shifts: model differences and model reliability. Glob Change Biol. (2006) 12:1568–84. doi: 10.1111/j.1365-2486.2006.01191.x
36. Levin PS, Stunz GW. Habitat triage for exploited fishes: can we identify essential “Essential Fish Habitat?” Estuar Coast Shelf Sci. (2005) 64:70–8. doi: 10.1016/j.ecss.2005.02.007
37. Livernois MC, Fujiwara M, Fisher M, Wells RD. Seasonal patterns of habitat suitability and spatiotemporal overlap within an assemblage of estuarine predators and prey. Mar Ecol Progr Ser. (2021) 668:39–55. doi: 10.3354/meps13700
38. Wang Y, Wu X, Zheng L, Wu J, Zhang S, Wang X. Modeling seasonal changes in the habitat suitability of Coilia nasus in the Yangtze River Estuary using tree-based methods. Region Stud Mar Sci. (2023) 67:103212. doi: 10.1016/j.rsma.2023.103212
39. Snelson Jr FF, Williams-Hooper SE, Schmid TH. Reproduction and ecology of the Atlantic stingray, Dasyatis sabina, in Florida coastal lagoons. Copeia. (1988) 1988:729–39. doi: 10.2307/1445395
40. Daugherty DJ, Schlechte JW, McDonald DL. Alligator gars in Texas coastal bays: long-term trends and environmental influences. Trans Am Fish Soc. (2018) 147:653–64. doi: 10.1002/tafs.10041
41. Heupel MR, Simpfendorfer CA. Movement and distribution of young bull sharks Carcharhinus leucas in a variable estuarine environment. Aquat Biol. (2008) 1:277–89. doi: 10.3354/ab00030
42. Froeschke JT, Stunz GW, Sterba-Boatwright B, Wildhaber ML. An empirical test of the “shark nursery area concept” in Texas bays using a long-term fisheries-independent data set. Aquat Biol. (2010) 11:65–76. doi: 10.3354/ab00290
43. Villalon A, Lynch A, Awwad H, Gooch T, Albright J. Galveston Bay Freshwater Inflows Study. Trans-Texas Water Program. Austin, TX: Southeast Area Technical Memorandum, Texas Water Development Board (1998).
44. Du J, Park K, Shen J, Zhang YJ, Yu X, Ye F, et al. A hydrodynamic model for Galveston Bay and the shelf in the northern Gulf of Mexico. Ocean Sci. (2019) 15:951–66. doi: 10.5194/os-15-951-2019
45. Lester J, Gonzalez LA, Sage T, Gallaway A. The State of the Bay: A Characterization of the Galveston Bay Ecosystem. Houston, TX: Galveston Bay Estuary Program (2002).
46. Santschi PH. Seasonality in nutrient concentrations in Galveston Bay. Mar Environ Res. (1995) 40:337–62. doi: 10.1016/0141-1136(95)92644-J
47. Martinez-Andrade F. Marine Resource Monitoring Operations Manual. Austin, TX: Texas Parks and Wildlife Department, Coastal Fisheries Division (2018).
49. Allouche O, Tsoar A, Kadmon R. Assessing the accuracy of species distribution models: prevalence, kappa and the true skill statistic (TSS). J Appl Ecol. (2006) 43:1223–32. doi: 10.1111/j.1365-2664.2006.01214.x
50. Raes N, ter Steege H. A null-model for significance testing of presence-only species distribution models. Ecography. (2007) 30:727–36. doi: 10.1111/j.2007.0906-7590.05041.x
53. TinHan TC, Wells RD. Spatial and ontogenetic patterns in the trophic ecology of juvenile Bull Sharks (Carcharhinus leucas) from the Northwest Gulf of Mexico. Front Mar Sci. (2021) 8:664316. doi: 10.3389/fmars.2021.664316
54. Chase JM, Abrams PA, Grover JP, Diehl S, Chesson P, Holt RD, et al. The interaction between predation and competition: a review and synthesis. Ecol Lett. (2002) 5:302–15. doi: 10.1046/j.1461-0248.2002.00315.x
55. Barletta M, Barletta-Bergan A, Saint-Paul U, Hubold G. The role of salinity in structuring the fish assemblages in a tropical estuary. J Fish Biol. (2005) 66:45–72. doi: 10.1111/j.0022-1112.2005.00582.x
56. Cloern JE, Jassby AD, Schraga TS, Nejad E, Martin C. Ecosystem variability along the estuarine salinity gradient: examples from long-term study of San Francisco Bay. Limnol Oceanogr. (2017) 62:S272–91. doi: 10.1002/lno.10537
57. Brinkman EL. Contributions to the Life History of Alligator Gar, Atractosteus spatula (Lacépède), in Oklahoma. Stillwater, OK: Oklahoma State University (2008).
58. Mendoza Alfaro R, González CA, Ferrara AM. Gar biology and culture: status and prospects. Aquacult Res. (2008) 39:748–63. doi: 10.1111/j.1365-2109.2008.01927.x
59. Keevin TM, Lopinot NH. Historical record of an alligator gar, Atractosteus spatula (Lacépède, 1803), captured on the floodplain of the Middle Mississippi River at Columbia, Illinois, USA. J Appl Ichthyol. (2019) 35:871–5. doi: 10.1111/jai.13925
60. Kreiser BR, Daugherty DJ, Buckmeier DL, Smith NG, Newsome EB. Sibship analysis to characterize alligator gar reproductive contributions in two Texas systems. North Am J Fisher Manag. (2020) 40:555–65. doi: 10.1002/nafm.10400
61. Kluender ER, Adams R, Lewis L. Seasonal habitat use of alligator gar in a river-floodplain ecosystem at multiple spatial scales. Ecol Freshw Fish. (2017) 26:233–46. doi: 10.1111/eff.12270
62. Binion GR, Daugherty DJ, Bodine KA. Population dynamics of alligator gar in Choke Canyon Reservoir, Texas: implications for management. J Southeast Assoc Fish Wildlife Agenc. (2015) 2:57–63.
63. Suchy MD. Effects of Salinity on Growth and Survival of Larval and Juvenile Alligator Gar Atractosteus spatula, and on Plasma Osmolality of Non-teleost Actinoperygiian Fishes. Thibodaux, LA: Nicholls State University (2009).
64. Buckmeier DL, Smith NG, Daugherty DJ. Alligator gar movement and macrohabitat use in the lower Trinity River, Texas. Trans Am Fish Soc. (2013) 142:1025–35. doi: 10.1080/00028487.2013.797494
65. Solomon LE, Phelps QE, Herzog DP. Juvenile alligator gar movement patterns in a disconnected floodplain habitat in southeast Missouri. Am Midland Natural. (2013) 169:336–44. doi: 10.1674/0003-0031-169.2.336
66. Able KW. A re-examination of fish estuarine dependence: evidence for connectivity between estuarine and ocean habitats. Estuar Coast Shelf Sci. (2005) 64:5–17. doi: 10.1016/j.ecss.2005.02.002
67. Reis-Filho JA, Schmid K, Harvey ES, Giarrizzo T. Coastal fish assemblages reflect marine habitat connectivity and ontogenetic shifts in an estuary-bay-continental shelf gradient. Mar Environ Res. (2019) 148:57–66. doi: 10.1016/j.marenvres.2019.05.004
68. Gausmann P. Synopsis of global fresh and brackish water occurrences of the bull shark Carcharhinus leucas Valenciennes, 1839 (Pisces: Carcharhinidae), with comments on distribution and habitat use. Integr Systemat. (2021) 4:55–213. doi: 10.18476/2021.423083
69. Heupel MR, Simpfendorfer CA. Estuarine nursery areas provide a low-mortality environment for young bull sharks Carcharhinus leucas. Mar Ecol Progr Ser. (2011) 433:237–44. doi: 10.3354/meps09191
70. Cruz-Martínez A, Chiappa-Carrara X, Arenas-Fuentes V. Age and growth of the bull shark, Carcharhinus leucas, from southern Gulf of Mexico. J Northw Atlant Fish Sci. (2005) 35:367–74. doi: 10.2960/J.v35.m481
71. Natanson LJ, Adams DH, Winton MV, Maurer JR. Age and growth of the bull shark in the western North Atlantic Ocean. Trans Am Fisher Soc. (2014) 143:732–43. doi: 10.1080/00028487.2014.892537
72. Wade MW, Fisher M, Matich P. Comparison of two machine learning frameworks for predicting aggregatory behaviour of sharks. J Appl Ecol. (2022) 59:2767–78. doi: 10.1111/1365-2664.14273
73. Lofthus AJ, Beach-Mehrotra M, Fisher MR, Wozniak JR, Matich P. Factors influencing the nursery dynamics of bull sharks Carcharhinus leucas in two estuaries along the Texas coast. Mar Ecol Progr Ser. (2024) 728:43–58. doi: 10.3354/meps14495
74. Plumlee JD, Dance KM, Matich P, Mohan JA, Richards TM, TinHan TC, et al. Community structure of elasmobranchs in estuaries along the northwest Gulf of Mexico. Estuar Coast Shelf Sci. (2018) 204:103–13. doi: 10.1016/j.ecss.2018.02.023
75. Mullins L, Cartwright J, Dykstra SL, Evans K, Mareska J, Matich P, et al. Warming waters lead to increased habitat suitability for juvenile bull sharks (Carcharhinus leucas). Sci Rep. (2024) 14:4100. doi: 10.1038/s41598-024-54573-0
76. Meese EN, Lowe CG. Finding a resting place: how environmental conditions influence the habitat selection of resting batoids. Bullet South Calif Acad Sci. (2019) 118:87–101. doi: 10.3160/0038-3872-118.2.87
77. Klinck JM, Hofmann EE, Powell EN, Dekshenieks MM. Impact of channelization on oyster production: a hydrodynamic-oyster population model for Galveston Bay, Texas. Environ Model Assess. (2002) 7:273–89. doi: 10.1023/A:1020954502355
78. Sheridan P, McMahan G, Hammerstrom K, Pulich J, Warren A. Factors affecting restoration of Halodule wrightii to Galveston Bay, Texas. Restorat Ecol. (1998) 6:144–58. doi: 10.1111/j.1526-100X.1998.00625.x
79. Costa T, Thayer J, Mendes L. Population characteristics, habitat and diet of a recently discovered stingray Dasyatis marianae: implications for conservation. J Fish Biol. (2015) 86:527–43. doi: 10.1111/jfb.12572
80. Kanno S, Schlaff AM, Heupel MR, Simpfendorfer CA. Stationary video monitoring reveals habitat use of stingrays in mangroves. Mar Ecol Progr Ser. (2019) 621:155–68. doi: 10.3354/meps12977
81. Brinton CP, Curran MC. Tidal and diel movement patterns of the Atlantic stingray (Dasyatis sabina) along a stream-order gradient. Mar Freshw Res. (2017) 68:1716–25. doi: 10.1071/MF16073
82. Ramsden S, Cotton CF, Curran MC. Using acoustic telemetry to assess patterns in the seasonal residency of the Atlantic stingray Dasyatis sabina. Environ Biol Fish. (2017) 100:89–98. doi: 10.1007/s10641-016-0498-5
83. Davy LE, Simpfendorfer CA, Heupel MR. Movement patterns and habitat use of juvenile mangrove whiprays (Himantura granulata). Mar Freshw Res. (2015) 66:481–92. doi: 10.1071/MF14028
84. Elston C, Cowley PD, von Brandis RG, Lea J. Residency and habitat use patterns by sympatric stingrays at a remote atoll in the Western Indian Ocean. Mar Ecol Progr Ser. (2021) 662:97–114. doi: 10.3354/meps13632
85. Martins A, Matley J, Heupel M, Fisk A, Chin A, Simpfendorfer C. Trophic ecology of sympatric juvenile stingrays within a nursery area. Mar Freshw Res. (2022) 73:678–88. doi: 10.1071/MF21292
86. Oh BZ, Thums M, Babcock RC, Meeuwig JJ, Pillans RD, Speed C, et al. Contrasting patterns of residency and space use of coastal sharks within a communal shark nursery. Mar Freshw Res. (2017) 68:1501–17. doi: 10.1071/MF16131
87. Vaudo JJ, Heithaus MR. Diel and seasonal variation in the use of a nearshore sandflat by a ray community in a near pristine system. Mar Freshw Res. (2012) 63:1077–84. doi: 10.1071/MF11226
88. Gonzalez JG, Ménard F, Le Loc'h F, De Andrade HA, Viana AP, Ferreira V, et al. Trophic resource partitioning of two snook fish species (Centropomidae) in tropical estuaries in Brazil as evidenced by stable isotope analysis. Estuar Coast Shelf Sci. (2019) 226:106287. doi: 10.1016/j.ecss.2019.106287
89. Maitra S, Harikrishnan M, Nidhin B. Feeding strategy, dietary overlap and resource partitioning among four mesopredatory catfishes of a tropical estuary. J Fish Biol. (2020) 96:130–9. doi: 10.1111/jfb.14194
90. Matich P, Nowicki RJ, Davis J, Mohan JA, Plumlee JD, Strickland BA, et al. Does proximity to freshwater refuge affect the size structure of an estuarine predator (Carcharhinus leucas) in the north-western Gulf of Mexico? Mar. Freshw Res. (2020) 71:1501–16. doi: 10.1071/MF19346
91. Hernout B, Leleux J, Lynch J, Ramaswamy K, Faulkner P, Matich P, et al. The integration of fatty acid biomarkers of trophic ecology with pollutant body-burdens of PAHs and PCBs in four species of fish from Sabine Lake, Texas. Environ Adv. (2020) 1:100001. doi: 10.1016/j.envadv.2020.100001
92. Cottrant E, Matich P, Fisher MR. Boosted regression tree models predict the diets of juvenile bull sharks in a subtropical estuary. Mar Ecol Progr Ser. (2021) 659:127–41. doi: 10.3354/meps13568
93. Vaudo JJ, Heithaus MR. Spatiotemporal variability in a sandflat elasmobranch fauna in Shark Bay, Australia. Mar Biol. (2009) 156:2579–90. doi: 10.1007/s00227-009-1282-2
94. Bond M, Valentin-Albanese J, Babcock E, Heithaus M, Grubbs R, Cerrato R, et al. Top predators induce habitat shifts in prey within marine protected areas. Oecologia. (2019) 190:375–85. doi: 10.1007/s00442-019-04421-0
95. Montagna P, Vaughan B, Ward G. The importance of freshwater inflows to Texas estuaries. In: Griffin RC, editor. Water Policy in Texas: Responding to the Rise of Scarcit. Washington, DC: The RFF Press (2012). p. 107–27.
96. Matich P, Plumlee JD, Bubley W, Curtis TH, Drymon JM, Mullins LL, et al. Long-term effects of climate change on juvenile bull shark migratory patterns. J Anim Ecol. (2024) 2024:14140. doi: 10.1111/1365-2656.14140
97. Ross ST. Resource partitioning in fish assemblages: a review of field studies. Copeia. (1986) 1986:352–88. doi: 10.2307/1444996
98. Schwartz FJ, Dahlberg MD. Biology and ecology of the Atlantic stingray, Dasyatis sabina (Pisces: Dasyatidae) in North Carolina and Georgia. Gulf Mexico Sci. (1978) 2:1. doi: 10.18785/negs.0201.01
99. Livernois MC, Rezek RJ, Wells RD. Spatial and ontogenetic trophic dynamics of co-occurring predatory fishes in a Northern Gulf of Mexico Estuary. Estuar Coast. (2024) 8:1–20. doi: 10.1007/s12237-024-01361-8
Keywords: Atlantic stingray, alligator gar, bull shark, habitat use, random forest model
Citation: Fontaine P, Jensen CC, Matich P, Rooker JR and Wells RJD (2024) Predicting habitat suitability for the co-occurrence of an estuarine mesopredator and two top predatory fishes. Front. Fish Sci. 2:1443923. doi: 10.3389/frish.2024.1443923
Received: 04 June 2024; Accepted: 30 September 2024;
Published: 24 October 2024.
Edited by:
Julia L. Y. Spaet, University of Cambridge, United KingdomReviewed by:
Taryn Murray, South African Institute for Aquatic Biodiversity, South AfricaCopyright © 2024 Fontaine, Jensen, Matich, Rooker and Wells. This is an open-access article distributed under the terms of the Creative Commons Attribution License (CC BY). The use, distribution or reproduction in other forums is permitted, provided the original author(s) and the copyright owner(s) are credited and that the original publication in this journal is cited, in accordance with accepted academic practice. No use, distribution or reproduction is permitted which does not comply with these terms.
*Correspondence: Paco Fontaine, cGFjby5mb250YWluZUB0YW11LmVkdQ==
Disclaimer: All claims expressed in this article are solely those of the authors and do not necessarily represent those of their affiliated organizations, or those of the publisher, the editors and the reviewers. Any product that may be evaluated in this article or claim that may be made by its manufacturer is not guaranteed or endorsed by the publisher.
Research integrity at Frontiers
Learn more about the work of our research integrity team to safeguard the quality of each article we publish.