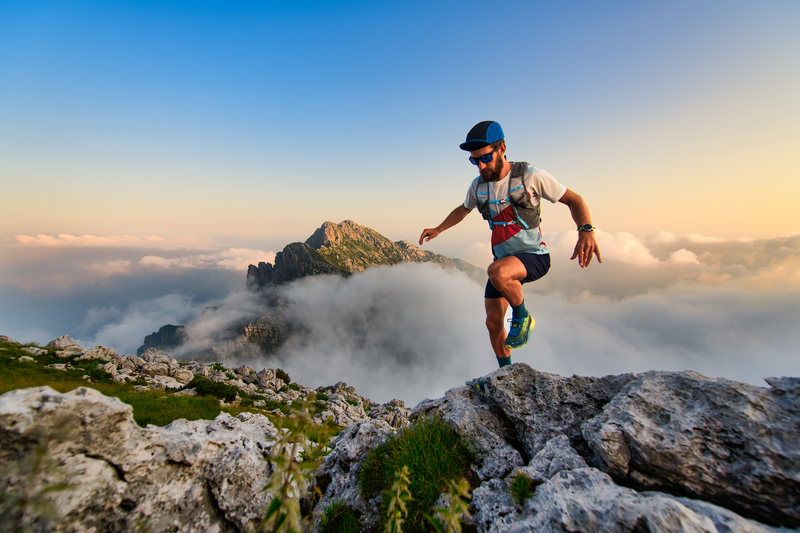
94% of researchers rate our articles as excellent or good
Learn more about the work of our research integrity team to safeguard the quality of each article we publish.
Find out more
SPECIALTY GRAND CHALLENGE article
Front. Internet Things , 22 July 2022
Sec. IoT Services and Applications
Volume 1 - 2022 | https://doi.org/10.3389/friot.2022.912388
The information era is coming. As a popular technology in the information age, Internet of Things (IoT) can collect various types of information in real time, realize the ubiquitous connection between things and people, and process the intelligent perception, identification, and management of things, processes, and information, and environment protection through various network access methods. Applying IoT to various fields is like wrapping the Earth with a layer of “digital skin” (Gubbi et al., 2013; Bauwens et al., 2020; Li and Da Xu, 2020). Moreover, under the trend of global warming, the emergence of the theme of Energy Saving and Emission Reduction (ESER) undoubtedly poses new challenges to the development of IoT.
When IoT is widely used in various life scenarios, its main purpose is to provide intelligent services and environments. IoT applications can connect any sensing device to the Internet for data transmission, so as to realize intelligent identification, tracking, positioning, and monitoring of sensing devices. In addition, IoT contains many types of Wireless Sensor Networks (WSN), which, as a part of IoT sensing layer, can meet the actual needs of people to obtain reliable data in special environments (Sadowski and Spachos, 2020). WSN is a multi-hop network which is self-organized by many sensor nodes. It has the characteristics of flexibility, fault tolerance, high awareness, low cost, strong survivability, and fast layout. Therefore, IoT has a wide range of applications, such as environmental monitoring, agriculture, military, and medical care, and can collect, process, and disseminate collected data deployed in various environments (Aman et al., 2020; Fortino et al., 2020). However, for some special scenes, many sensor nodes are often placed in areas that cannot be accessed by human beings. While enjoying the convenience brought by WSN, people are also faced with the problems that illegal personnel obtain illegitimate interests by intercepting the monitoring data transmitted in the public channel. Therefore, the attention to security issues in IoT cannot be ignored.
With the increasing commercial scope of 5th Generation Mobile Communication Technology (5G) communication technology in cities, the application of IoT in power, construction, industry, intelligent transportation, agriculture, logistics, intelligence, high efficiency, and ESER is being promoted (Verma et al., 2020). Of course, applying IoT is also inseparable from the combination of 5G communication, Cloud Computing (CC), Edge Computing (EC), blockchain, AI, and other technologies. IoT can collect many data from the environment, effectively monitor, analyze, and manage energy consumption, and reasonably improve IoT by identifying and analyzing opportunities of energy efficiency improvement (Khan et al., 2020; Liao et al., 2020; Saračević et al., 2020).
In summary, in today’s high-speed 5G communication technology, IoT is widely applied, and guaranteeing its performance is of high significance. Therefore, taking the practical application of IoT as the theme, this work explores the application of intelligent power, intelligent building, intelligent industry, intelligent transportation, intelligent agriculture, and intelligent logistics. In addition, it analyzes the intelligent prospect of IoT application based on the intelligent Information Security (IS) of IoT system and CE (carbon emission) reduction under the cooperation of Artificial Intelligence (AI), so as to provide a theoretical reference for the intelligent development of smart cities (SC) and social system and ESER.
With the penetration of “everyone connected” to “everything connected” in various industries, IoT is well-known as a symbol of SC in the Internet + era. IoT can equip Radio Frequency Identification Tag (RFID), sensors, two-dimensional code, and other types of sensors to a variety of real objects, through the interface and wireless network connection, and then run the original program for remote control or direct communication among objects, so as to realize the interconnection and dialogue between people and objects, and objects and objects, so that the urban environment realizes self-perception, and lays a foundation for data collection, mining analysis, and decision support (Abd El-Latif et al., 2020; Wang T et al., 2020). When IoT is applied in SC, its architecture is designed based on the initial three-tier architecture (Figure 1).
IoT system is mainly composed of collection, transmission, and application layers. Its terminal data-collection layer contributes to the construction of SC and IoT information system. The application layer is a critical component to give full play to the system function, and network transmission layer to connect the SC information system.
Therefore, the application of IoT system in all walks of life is described respectively for SC construction. It mainly classifies the applications of IoT in intelligent power, intelligent building, intelligent industry, intelligent transportation, intelligent agriculture, intelligent logistics, and other fields (Figure 2).
The IoT has been expounded in many fields such as intelligent power, building, industry, transportation, agriculture, and logistics.
Ubiquitous power IoT applies the IoT in power systems. Its essence is the resource-sharing of all professional perception layers, the aggregation and collaborative application of all kinds of data, the real-time online combination of data accommodation and businesses, the promotion of regional energy autonomy, and the better support of resource allocation, business collaboration, and risk prevention and control, to form a physical entity with self-perception and intelligent active operation (Adi and Kitagawa, 2019). Application of IoT in electric power has been studied extensively. Niu et al. (2019) proposed a power IoT load distribution mechanism for EC, which further realized the optimal distribution of workload. Balanced Initialization, Resource Allocation, and Task Allocation (BRT) algorithms were presented. The simulation results showed that this mechanism could minimize the service delay compared with Simulated Annealing Algorithm (SAA), LoAd Balancing (LAB), and Latency-awarE orkload offloaDing (LEAD). Fu et al. (2020) put forward a distributed User Cluster (UC) algorithm for clustering IoT devices into multiple UC and a system optimization model to lower the power consumption. Finally, many simulations suggested the effectiveness of the algorithm (Fu et al., 2020). Gomez et al. (2020) (Gomez et al., 2020) introduced the Static Context Header Compression and Fragmentation (SCHC) to solve insufficient self-adaption based on 6LoWPAN. Kaur et al. (2021), Lee et al. (2021) used transfer learning algorithm to deal with challenges related to data storage and processing and computational complexity in power IoT system, and finally found that the technology could bring innovation and higher productivity to intelligent changes in power systems.
It is found that most scholars optimize the performance of power systems based on the IoT, but there are few studies on fault occurrence, efficiency reduction, and function failure in power IoT system. Moreover, the accuracy and adaptability of operation state evaluation and Life Cycle (LC) prediction of complex power equipment in physical space are difficult to meet. It is unknown whether the industrial Big Data (BD) shared by various sensing equipment information and communication information is fully utilized in the power IoT. In view of the possible problems and faults in the power IoT mentioned above, the Digital Twins (DTs) technology is applied to it. The specific DTs-based power IoT system is shown in Figure 3.
The intelligent management system of DTs-based power IoT engine monitors the bearing vibration and rotating shaft speed of the entity in real time using current power sensors and angular speed sensors. The system uses DTs technology to map the specification data, stored historical data, and real-time collected data in the physical entity of power IoT engine into the virtual space (Kaur et al., 2021), then fuses and extracts the data, transmits the data features to the cloud platform, and describes the components of the engine from multiple dimensions such as physics, information, and behavior. The engine entity and its DTs information model operate together, and the twinning data generated includes the data perceived during operation, as well as the simulation data of shafting wear, torsional vibration, thermal stress, and other data generated by the information model (Montazerolghaem and Yaghmaee, 2020; Rahman et al., 2020). Finally, it is applied to the condition monitoring, fault diagnosis, optimized operation, and maintenance guidance services of power IoT engine.
In the construction field, as the “brain” of the intelligent control of the whole building, the IoT system can comprehensively and centrally manage and control each separate intelligence, including air conditioning, fresh air, lighting systems, energy metering monitoring, and PM 2.5 concentration monitoring, so as to realize intelligent, healthy, safe, efficient, and sustainable development (Spachos et al., 2018). Many scholars have explored using IoT in architecture. Plageras et al. (2018) put forward a new system for collecting and managing sensor data in operating intelligent buildings in the IoT environment. The results showed that the proposed solution for collecting and managing sensor data could lead to energy-saving intelligent buildings and green intelligent buildings. Kumar et al. (2018) mainly focused on the application of IoT sensor actuators in intelligent buildings, such as air quality, lighting, and heating/cooling, and proved that the system could understand the importance of various factors in intelligent buildings. Jia et al. (2019) summarized the current technologies of IoT applied to buildings and related fields, including three different levels based on the traditional IoT architecture, and discussed the priorities and challenges of successful seamless IoT integration in intelligent buildings. In addition, issues were discussed to promote the implementation of IoT in the construction and operation stages. Yu K et al. (2020) proposed a IoT-based intelligent building architecture, and found that the data aggregation of indoor electrical IoT and public facilities integration system formed building BD. Lv et al. (2021) constructed the physical world of an intelligent building physical information fusion system based on IoT, and used Back Propagation Neural Network (BPNN) for classification and pattern recognition. Finally, it was found that the combination of an information physical system and AI could provide a theoretical basis and practical reference for the intelligent building industry.
Through the research and analysis above, it is found that many scholars apply IoT and other intelligent technologies to the field of intelligent buildings, but the mature application of IoT in the construction industry has not been fully realized, so researchers in relevant fields need to pay more attention to its application.
The LC of intelligent building is predicted by using AI, BD, and IoT. Many scholars have explored its application in intelligent building. Zhao L et al. (2020) built an Intelligent Digital Twin-Software Defined Virtual Networks (IDT-SDVNs). The network architecture was simulated and analyzed to verify its effectiveness. White et al. (2021) found that, as the combination of Building Information Models (BIM) increased in size and accuracy in SC and the BD, various data in SC are becoming more accurate and transparent. Hou et al. (2018) pointed out a link prediction method for evaluation of the LC of intelligent buildings, which can predict the missing data. (Antoniadou-Plytaria et al., 2020) proposed a microgrid energy management system of intelligent building based on battery energy storage. Simulation results proved that the framework could more accurately estimate the building operation cost and improve the overall battery performance. (Bhamidi and Sivasubramani, 2020) presented a two-stage optimization model for home Renewable Energy Resources (RERs) and battery integration optimization. Its effectiveness was verified. (Du et al., 2021) predicted the LC of power transformer in intelligent building, and finally found that the Mean Absolute Percentage Error (MAPE) of the model was 5.20% on the test set, showing higher accuracy.
With the increasingly wide application of DTs technology, the combination of AI algorithm and DTs technology in the field of intelligent building is significant to its digital development and security maintenance.
After the proposal of “industry 4.0,” manufacturing in China has further developed in the direction of intelligence. At present, applying IoT to the manufacturing industry is generally believed to integrate electronic information technologies such as sensors, embedded systems, wireless networks, and actuators with manufacturing technology to realize reliable perception and real-time transmission of data in the production and manufacturing process, processing and integration of massive data, accurate control and optimization of production process, and trusted service and management of manufacturing products (Abdel-Basset et al., 2020; Wu, 2020). Liu et al. (2018) analyzed several key signal processing technologies to improve the performance of authorization free strategy and found that large-scale multiple input and multiple output was particularly suitable for large-scale IoT. This was because the multi measurement vector compressed sensing technology was adopted. When the number of antennas at the base station tended to infinity, the device detection error could asymptotically approach zero within the limit. Zhang et al. (2019a) proposed an updated framework to process the hypergraph signals. Through experimental analysis, it was found that, compared with some traditional signal processing solutions, using hypergraph signal processing framework could significantly improve the performance. Zhao Z et al. (2020) constructed a random game model to simulate the interaction between users and access points by the mobile EC network facing intelligent information services. By applying array signal processing scheme to users, the system is further optimized to reduce transmission delay. The simulation results indicated that the array signal processing scheme could significantly reduce the transmission delay. (Jbair et al., 2018) analyzed the information physics system technology, components, and relevant standards, and conducted relevant research on its potential fields (Tao et al., 2018); believed that intelligent interconnection was one of the important difficulties in realizing intelligent manufacturing instead of other aspects; then the Industrial IoT Hub (IIHub) integrating various other contents was optimized for intelligent interconnection. (Lyu et al., 2019) found that the state estimation of WSN exerted a key effect in the pervasive monitoring of industrial network physical systems. To meet the reliability requirements of state estimation, a redundant transmission strategy is proposed. This strategy combines different channels to provide sufficient spectrum opportunities for redundant transmission. Therefore, a joint optimization method of channel allocation and power control is pointed out to ensure the estimation performance and maximize the rate of WSN. (Dowdeswell et al., 2020) used machine learning to create a sufficiently complete model under the continuous and extensive use of model-based and data-driven AI in all fields; it was found that when the physical environment put forward requirements for the industrial physical information system, it was difficult to connect the industrial physical information system together to share enough telemetry data to diagnose and manage faults. (Li et al., 2020) suggested DTs driven architecture for the dynamic evolution of the whole LC in intelligent manufacturing in view of the challenges faced by the traditional manufacturing industry. The effectiveness of the architecture was verified. (Lv et al., 2020a) analyzed the typical information physical system control model based on spatio-temporal correlation detection model, and determined the analysis strategy of comprehensive reliability model. Finally, it was confirmed that the model can help in selecting appropriate defense resources against the attack and defense environment of information physical system.
In the industrial field, digital transformation is the trend. While comprehensively promoting digital industrialization and industrial digitization, it will comprehensively deploy the construction of a new generation of information and communication infrastructure. Thus, it will promote the full integration of the new generation of the deep integration of manufacturing and service industries, and accelerate the rapid development of digital economy and real economy. Industrial IoT (IIoT) takes data as the core, based on the massive data collected by sensors, combined with software platform and BD analysis technology to realize industrial automatic control and intelligent management. With the empowerment of IIoT, the productivity and work efficiency of enterprises have been improved, and the energy use and CE have been effectively reduced, to finally achieve energy conservation and efficiency increase. The IIoT system structure is shown in Figure 4.
During the intelligent transportation development, applications such as vehicle-road coordination, intelligent parking, and intelligent transportation planning are generally put on the agenda. The latest development in intelligent vehicles and Intelligent Transportation Systems (ITS) was realized after the sensor data was captured and processed (Cicconetti et al., 2020; Zhang et al., 2020). (Jan et al., 2019) designed a model for analyzing traffic data using Hadoop and Spark to process real-time traffic data. Aiming at the traffic data sets from various real sources, the constructed system was tested. The results revealed that the time of data processing and real-time communication with citizens was shorter. Yu L et al. (2020) proposed an intelligent transportation system of hybrid automatic and manual vehicles of traffic safety solution based on Deep Learning under 5G network. The experimental results proved the accuracy was improved greatly. (Arthurs et al., 2021) focused on the relationship between CC and intelligent transportation, and believed that CC and their sensors can solve delay and bandwidth constraints. (Hammoudeh et al., 2020) proposed an architecture aiming to realize the interoperability among various IoT platforms and support service-oriented modeling at a high level of abstraction. The results revealed that the architecture abstraction language allowed the combination of macro programming and node-centered programming, to realize the development of real-time and efficient intelligent transportation applications on the IoT.
It is found that the implementation of the Internet of Vehicles (IoV) depends more on edge devices. Therefore, compared with CC service, EC can further reduce the delay. It effectively reduces the prediction limitations, and predicts the road network in the SC effectively.
For further development of SC, traffic flow (TF) has to be predicted. (Lv et al., 2018) raised a new government service platform (Sodhro et al., 2019); verified that the Quality of Service (QoS) Aware, Green, Sustainable, Reliable, and Available (QGSRA) algorithm was superior to the traditional algorithm. Yu K et al. (2020) proposed an intelligent transportation system of hybrid, automatic, and manual vehicles of traffic safety solution based on Deep Learning under 5G network. (Qiu et al., 2019) raised a neighborhood-based Deep Learning method. By capturing the characteristics of each section, the trajectory characteristic of adjacent sections could be an interaction between road network topology and speed, the constructed model was proved to show a better performance than the existing models. (Jaleel et al., 2020) presented a Collaborative and Adaptive Signaling on the Edge (CASE) for traffic signals control. The performance comparison suggested that transplanting the controller based on Graphic Processing Units (GPU) can obtain better performance by greatly shortening the calculation time.
There are many pieces of research on Short-Term Traffic Flow (STTF) prediction. However, many defects still can be found in STTF prediction. This is why 5G network is commercial and popular. It combines advanced communication technology with the construction of urban road network transportation system, and further combines DTs technology to map the TF to establish an intelligent network integrating people, vehicles, roads, and the environment (Arthurs et al., 2021). It can finally better predict the urban TF, so it could be promoted in digital and intelligent development of intelligent transportation in the network physical society.
Precision agriculture helps to provide green ecological products. As a large agricultural country, China now relies more on traditional agricultural production, and land efficiency depends heavily on chemical fertilizers. The process of producing chemical fertilizer will also bring CE (Wang X et al., 2020; Rahman and Hossain, 2021). (Zulkifli and Noor, 2017) introduced the application of active RFID and Wireless Mesh Sensor Network (WMSN) technology in agriculture, and supported WSN by developing agricultural automatic IoT for irrigation systems. The automatic control system is very practical in agriculture, but most automatic control systems are based on schedules and timers, which is independent of soil conditions and temperature, and the wireless automatic irrigation system for water use and production has obvious efficiency. (Chen and Yang, 2019) built an intelligent agricultural system using the IoT technology. The system can screen the key technologies for production improvement and quality control of agricultural products. (Delnevo et al., 2021) used the social IoT to perceive environmental conditions (solar radiation, humidity, air temperature, and soil humidity) and communication, and predicted when plants would get sick with specific accuracy through data fusion and Deep Learning, to finally make the agricultural economy develop in the direction of sustainability. (Friha et al., 2021) comprehensively summarized the emerging technologies of intelligent agriculture based on IoT, and classified and compared the latest methods of supply chain management based on agricultural IoT Blockchain technology. It shows the excellent performance of IoT in intelligent agriculture. (Xing et al., 2021) introduced three models to accelerate the intelligent agriculture development. It is considered that new technologies in intelligent agriculture are the future directions of intelligent agriculture.
In summary, using IoT provides intelligent agricultural machinery interconnection for agricultural operators, carries out remote monitoring, operation and maintenance, and health management of Internet agricultural machinery equipment, and realizes accurate management of agricultural machinery. Therefore, in the follow-up development of agriculture, precision agriculture can automatically collect and analyze data that will help to improve farm efficiency and yield by combining intelligent algorithms such as Deep Learning with IoT and predictive analysis. Precision agriculture can help eliminate the excessive use of fertilizer, reduce the overall impact of agriculture on the environment, and improve the yield of crops. It can reduce the consumption of water, pesticides, and chemical fertilizers, reduce food production costs, reduce runoff, and minimize the impact on natural ecosystems (Gebremichael et al., 2020; Manogaran et al., 2020). Moreover, IoT devices provide farmers with a great opportunity to remotely monitor the condition of their livestock and obtain data about livestock and their health, which means that farm managers can make better decisions faster and bring higher profits.
Logistics is the driving force behind national and enterprise competitiveness and exerts a vital role in economic growth. The application of IoT in logistics transportation not only alleviates the high cost and low efficiency, but also provides opportunities for the development of intelligent logistics. (Luo et al., 2019) analyzed the impact of IoT environment on transportation, put forward a new IoT-based framework of intelligent transportation system, and introduced the element deployment, communication network, and three-tier architecture of the system in detail. It was found that the IoT-based intelligent transportation system could help decision-makers improve the utilization of traffic resources, improve scheduling efficiency, and reduce the travel time of users. (Song et al., 2020) conducted a comprehensive survey of the literature on applying IoT in intelligent logistics. The challenges and development direction of IoT in logistics and transportation were discussed. (Lv, 2020) discussed the gateway security of the designed edge device to enhance its reliability. It was found that the model could obviously lower the risk of data leakage. (Bhutta and Ahmad, 2021) developed an IoT-based security monitoring and reporting system to update the quality of perishable goods and supply chain management, focusing on transportation without any human intervention.
Wang et al. (2019), Wang T et al. (2020) designed a multi-objective and multi-cycle model for urban medical waste (MW) reverse logistics and constructed a dynamic method of MW reverse logistics network. The sensitivity analysis results verified the important impact of this method on network performance. (Liu et al., 2020) explored the mode of MW transfer station based on site selection, construction, and financing. The results verified the effectiveness of the proposed mode of MW transfer station, solved the key problems related to the operation of the transfer station, such as where to build, how to build, and the source of funds, and provided a certain reference for the actual operation and construction of the transfer station. (Torkayesh et al., 2021) analyzed the intelligent technology in the MW management system. The results show that the training of different stakeholders, market acceptance, transparency, and professionals are the main factors leading to the failure of intelligent technology adoption. The results verify the effectiveness of the proposed intelligent technology. (Kumar et al., 2021) used AI to classify and intelligently recycle relevant MW. The classifier based on Support Vector Machine performs best in classifying waste types under the background of circular manufacturing, with an accuracy of 96.5% and a sensitivity of 95.3%, showing the excellent ability of managing MW. (Zhao et al., 2021) compared 5 MW disposal technologies and their optimization schemes. The results indicated that the economic cost of pyrolysis incineration is the lowest. It is recommended to jointly incinerate the sterilized MW for optimization, and the impact on the environment can be reduced by at least 45%.
Although there have been relevant studies on the recovery of MW, the previous studies are aimed at the recovery and treatment of MW, but the recovery and treatment of waste medical instruments and equipment is very rare. Based on the previous exploration, it optimizes and uses the reverse logistics network to recycle the waste medical instruments and equipment, which contributes to the development of low-carbon economy and environmental protection.
Therefore, applying IoT to the logistics field can optimize the intelligent logistics supply chain management. In the intelligent supply chain system (SCS), the most important things can integrate the existing resources and form an efficient service system. It can strengthen the management of warehouses and goods and make enterprise management more convenient. Especially in product management, it can reduce wrong delivery or theft, and improve the management efficiency of products. It can know the product information rapidly by screening the bars. Therefore, under the background of IoT, logistics SCS can be managed intelligently. It has certain advantages for enterprises and customers, and has a positive impact. Among them, the architecture of IoT-based intelligent logistics system is shown in Figure 5.
Through the application of other simulation control, algorithm architecture, RFID, and other technical means, an intelligent logistics system with IoT as the core and supplemented by a variety of technologies is formed. However, in the intelligent logistics system, the essence of IoT is a centralized structure. With the increase of the number of mobile terminals and fixed terminals, the burden of the central server is increasing, and the security of information transmission cannot be effectively guaranteed.
Due to decentralization, openness, tamper resistance, anonymity, and traceability, Blockchain technology provides a new solution for intelligent logistics system based on IoT (Philip et al., 2021). (Perboli et al., 2018) combined Blockchain with the data flow of SCS, outlined how to use the Blockchain, and analyzed the opportunities and challenges in costs reduction and operation optimization. (Puthal et al., 2018) applied Blockchain in a distributed framework for convenience of users, and deeply analyzed its applicability in business applications (Choi et al., 2019); analyzed how Blockchain technology promoted the risk in SCS. (Humayun et al., 2020) explored if the IoT can be combined with Blockchain so that they could be applied in intelligent SCS and highlighted the advantages of them through the analysis of actual cases. To ensure the security and fast and efficient query of logistics information (Sun et al., 2021), proposed a logistics information query algorithm based on the characteristics of Blockchain. Finally, the correctness, integrity, and security of this scheme are analyzed, and the feasibility of this scheme is proved.
The application of Blockchain in intelligent logistics IoT system, the combination of Blockchain technology and IoT system, and the application of Blockchain technology in the intelligent processing and information traceability of data and information in the field of logistics are of great value to the guarantee of safety performance and the improvement of intelligent performance in the process of logistics transportation.
In the process of practical application of IoT, it is not only the all-round application in many fields, but also the intelligent IS, AI collaborative optimization, and ESER.
Firstly, 5G network has basically achieved full coverage. Moreover, the IoT is becoming more and more perfect. However, in the era of interconnection of all things, when the collected data can be transmitted and browsed more intelligently by using the IoT system, IS guarantee has also become the development trend.
Secondly, the IoT system can cooperate with AI algorithms to optimize the system and predict CE at the same time. For example, Blockchain has the characteristics of decentralization, traceability, and being tamper-proof. Its application in the IoT can effectively ensure the data IS (Nie et al., 2020). As one of the AI algorithms, Deep Learning can effectively extract the information from the collected data to realize energy optimization and CE reduction (Lv et al., 2020b). DTs technology can classify and integrate all kinds of data in physical objects with virtual spatial data (Haghighi et al., 2020).
When looking ahead to the intelligent application of IoT system in all walks of life, the analysis of intelligent IS, collaborative optimization by AI, and energy conservation and emission reduction in the fields of intelligent power, intelligent building, intelligent industry, intelligent transportation, intelligent agriculture, and intelligent logistics is shown in Figure 6.
While the IoT system shows its important roles in all aspects of human life, and although all systems are developing towards intelligence, its IS problem cannot be ignored. (Al-Turjman and Alturjman, 2018) found that in medical applications, the 5G-inspired IIoT paradigm, through secure WMSN, can realize interaction between users and machines. However, user self-verification before each interaction is a lengthy and time-consuming process, which will interfere with user’ activities and reduce the overall performance of the medical system. Therefore, a Context-sensitive Seamless Identity Provisioning (CSIP) framework is proposed to solve this problem. It was found that the framework can achieve the security of WMSN in a moment. (Anand et al., 2020) developed a double watermark based on compression and then encryption to protect the Electronic Patient Records (EPR) data of the medical system, which has some important characteristics. After using medical sensors to collect medical data, the proposed method was confirmed to be of use in intelligent medical treatment, and it provides better performance in robustness and security. Aiming at the energy imbalance in network systems (Zhu, 2021), introduced the calculation method of network energy consumption, and optimized network structure to enlarge the communication scale. The hardware of the cold chain collaborative monitoring (CCCM) system can work normally, the software design of the CCCM system can meet the enterprises’ basic requirements, and its accuracy has been improved to a higher degree. (Tan et al., 2020) presented a Blockchain-based BD access control scheme, which accessed BD in the Blockchain as the identity, redefined the access control permissions, and stored them in the Blockchain. The proposed system scheme is feasible and effective, and can protect privacy and realize secure access in the network. Yang et al. (2020) (Yang et al., 2020) reviewed the previous studies on applying visual perception in different industrial fields. Through investigation and discussion, it is found that the current challenges of visual perception are summarized, which is practical for predicting the future trend of intelligent perception. (Qu et al., 2020) believed that the Cyber-Physical-Social System (CPSS) realized human social interaction from cyberspace to the physical world by sharing more and more spatio-temporal data. Thus, a personalized model was put forward to realize differential privacy and spatiotemporal private data sharing by using Generative Adversarial Nets (GAN). The data can perfectly approximate the space-time trajectory and provide high-level privacy protection, which can guide the development of network physics and social intelligence. (Gielis and Prorok, 2021) aimed at the robot network in the real world, improved the competition-based network due to its highly dynamic communication topology and strict real-time transmission requirements, and realized safer robot motion control in a dense multi robot environment by using the navigation information between machines. (Xue et al., 2021) pointed out a fuzzy Operation Safety Assessment (OSA) and designed a multi model linkage mechanism to monitor the electrical or mechanical faults of the motor simultaneously and evaluate its operation safety intelligently. Through the experimental analysis, the effectiveness of this method is verified.
However, few studies have been made on the correlation of user data security interaction in IoT systems. Therefore, data privacy, similar to WSN, is regarded as the most important issue in the Block-chain-based Service Network (BSN), which needs to protect data from disclosure.
The secure location of the data can prevent an attacker from reporting the wrong signal strength to send the correct report about the user’s location. It avoids various security threats and attacks such as data modification, counterfeiting, eavesdropping, and playback. (Lai et al., 2019) proposed a real model to explore the cascading failure of CPPS, and proposed the deterministic and random coupling strategy of asymmetric CPPS with different subnet sizes to improve its robustness to random and intentional network attacks. It can improve the robustness of the system to network attacks. Aiming at malicious attacks on information physical systems (Hussain et al., 2020), suggested using deep Convolution Neural Networks (CNNs) to detect real network data, so that the Distributed Denial of Service (DDoS) attacks planned by botnets controlling malicious devices could be predicted as early as possible. Aiming at the security when the IoT system is applied to the power field (Kwon et al., 2020), introduced an anomaly detection system based on Bidirectional Recurrent Neural Network, incorporated various specific attack data in the power system, and of course verified the effectiveness. (Hu et al., 2021) selected various new technologies and application scenarios covering the field of CPS and IoT for analysis and finally their effectiveness was verified.
By eavesdropping on communication and related key information, an attacker may seriously harm the interests of users, because the attacker can use the obtained data for many illegal purposes. If an attacker changes the data by adding some fragments or processing packets, and forwards the changed data to the coordinator, it may cause great losses to society. Therefore, effective security monitoring on the IoT system is very valuable. The IS and attack detection of the IoT system are shown in Figure 7.
Under the trend of extensive application fields of AI algorithms such as Blockchain, DTs, and Deep Learning, its combination with IoT systems has also become an inevitable trend. (Kumar and Chaurasiya, 2018) undertook WSN as a necessary platform for data perception and communication to fully describe the environment or make robust decisions. In order to improve efficiency, spatio-temporal data mining is carried out on the sensing samples collected by sensor nodes, and a redundancy elimination strategy is proposed. Before forwarding to the base station or cluster head in WSN, the collected data is mined to select the appropriate information. The results show that the proposed scheme has better performance compared with other schemes in the same simulation scenario. (Khattak et al., 2019) described the key components of IoT (i.e., architecture, standards, and protocols) in the security environment of perception layer, and then the security requirements of IoT. Secondly, after describing the layered security of general IoT, the two key enabling technologies of sensing layer, namely RFID and sensor network, are discussed. Finally, the open research and challenges related to the perception layer are identified and analyzed. When applying Blockchain technology to the decentralized and untrusted environment of IIoT (Zhang et al., 2019b), pointed out that anonymous and trusted transactions could be realized, which helped to reduce system risks, alleviated financial fraud, and reduced operating costs. (Sodhro et al., 2020) proposed a 5G-based adaptive green algorithm to enhance the reliability. All transmitted packets must be transmitted in a specific active time slot. This method obtained 55% green and 41% reliable ITS platform. (Zhang S et al. 2019) proposed a Cognitive IoT (CIoT), which can improve the operation efficiency. Lv et al. (2020) (Lv and Kumar, 2020) analyzed the dual channel architecture using the wireless sensor software in 6G/Internet of Everything (IoE), and proposed a solution to reduce transmitting relevant signals. Therefore, it could decrease the traffic load. (Li et al., 2019) raised a computing framework and a strategy for resource scheduling so that the EC-supported intelligent manufacturing could be satisfied. (Shahbazi and Byun, 2021) combined Blockchain, EC, and Machine Learning for analysis on advanced data, which obviously shortened the computing time. (Zhang et al., 2021) presented a vehicle edge caching mechanism based on social perception, which dynamically coordinated the Roadside Units (RSUs) and intelligent vehicles according to user preference similarity and service availability. DTs technology is used to map the edge cache system to the virtual space to facilitate the construction of a social relationship model. The edge cache scheme has great advantages in optimizing the cache utility.
The use of intelligent technologies can effectively reduce the energy consumption of IoT, thus reducing CE.
Analyzing the physical objects faithfully can also reflect the real changes of the whole digital system. This can not only reduce the energy consumption of objects, but also reflect the real changes of the whole digital system. (Laamarti et al., 2020) established an ISO/IEEE11073 standardized DTs framework architecture, which could form the basis of the development of intelligent medical DTs. (Fuller et al., 2020) analyzed the challenges in AI, IoT, and DTs, and explored the use, challenges, and role of DTs technology in the field of SC transportation. (White et al., 2021) found that, with the BIM combined with BD generated by IoT sensors, a large amount of data in SC was more accurate and clearer. A DTs model makes urban planning more accurate. (Lv et al., 2020c) combined the K-means and fuzzy theory and BD technology to enhance the performance of the vehicle transportation network and reduce the network data transmission performance delay, change the path, and effectively curb the spread of congestion. (Bhamidi and Sivasubramani, 2020) presented a two-stage optimization model for home Renewable Energy Resources (RERs) and battery integration optimization. Its effectiveness was verified. (Du et al., 2021) forecasted the LC of power transformer in intelligent transportation, and finally found that MAPE of the model was only 5.20%, suggesting that the accuracy is extremely high. (Francisco et al., 2020) used smart meters to adjust building energy in the DTs of SC, making smart energy management within the geographical scope of medium and large buildings in SC a key step in SC construction.
DTs technology is of great significance to reduce energy consumption in a SC. Further, by using the BD backplane and “urban hub” of spatiotemporal correlation integrated by IoT system and intelligent CC, it can enable the comprehensive integration of physical cities and digital cities and the collaborative management of all fields, and make it easier for urban managers to conduct urban simulation and management in many fields, guiding and optimizing various industries in the SC. Among them, the system architecture of the combination of DTs technology and IoT is shown in Figure 8.
The practical application of IoT has helped to realize the intelligent drive in many fields of society. Aiming at the development status of the practical application of IoT, it makes a summary analysis from the perspectives of intelligent IoT systems in various fields. In addition, various security threats and attacks such as data modification, counterfeiting, eavesdropping, and playback in the IoT system are effectively monitored to ensure the IS when the IoT system is applied. Through effective cooperation with AI such as Blockchain, Deep Learning, DTs, and EC, the IoT system is continuously optimized to develop towards intelligence, high efficiency, and ESER.
The author confirms being the sole contributor of this work and has approved it for publication.
The author declares that the research was conducted in the absence of any commercial or financial relationships that could be construed as a potential conflict of interest.
All claims expressed in this article are solely those of the authors and do not necessarily represent those of their affiliated organizations, or those of the publisher, the editors and the reviewers. Any product that may be evaluated in this article, or claim that may be made by its manufacturer, is not guaranteed or endorsed by the publisher.
Abd El-Latif, A. A., Abd-El-Atty, B., Mazurczyk, W., Fung, C., and Venegas-Andraca, S. E. (2020). Secure data encryption based on quantum walks for 5G Internet of Things scenario. IEEE Trans. Netw. Serv. Manage. 17 (1), 118–131. doi:10.1109/tnsm.2020.2969863
Abdel-Basset, M., El-Shahat, D., Elhoseny, M., and Song, H. (2020). Energy-aware metaheuristic algorithm for industrial-Internet-of-Things task scheduling problems in fog computing applications. IEEE Internet Things J. 8 (16), 12638–12649. doi:10.1109/jiot.2020.3012617
Adi, P. D. P., and Kitagawa, A. (2019). Quality of Service and power consumption optimization on the IEEE 802.15. 4 pulse sensor node based on Internet of Things. Int. J. Adv. Comput. Sci. Appl. 10 (5), 144–154. doi:10.14569/ijacsa.2019.0100518
Al-Turjman, F., and Alturjman, S. (2018). Context-sensitive access in industrial internet of things (IIoT) healthcare applications. IEEE Trans. Ind. Inf. 14 (6), 2736–2744. doi:10.1109/tii.2018.2808190
Aman, A. H. M., Yadegaridehkordi, E., Attarbashi, Z. S., Hassan, R., and Park, Y. J. (2020). A survey on trend and classification of internet of things reviews. Ieee Access 8, 111763–111782. doi:10.1109/access.2020.3002932
Anand, A., Singh, A. K., Lv, Z., and Bhatnagar, G. (2020). Compression-then-encryption-based secure watermarking technique for smart healthcare system. IEEE Multimed. 27 (4), 133–143. doi:10.1109/mmul.2020.2993269
Antoniadou-Plytaria, K., Steen, D., Carlson, O., Ghazvini, M. A. F., and Fotouhi Ghazvini, M. A. (2020). Market-based energy management model of a building microgrid considering battery degradation. IEEE Trans. Smart Grid 12 (2), 1794–1804. doi:10.1109/tsg.2020.3037120
Arthurs, P., Gillam, L., Krause, P., Wang, N., Halder, K., Mouzakitis, A., et al. (2021). A taxonomy and survey of edge cloud computing for intelligent transportation systems and connected vehicles. IEEE Trans. Intell. Transp. Syst., 1–16. doi:10.1109/tits.2021.3084396
Bauwens, J., Ruckebusch, P., Giannoulis, S., Moerman, I., and De Poorter, E. (2020). Over-the-air software updates in the internet of things: An overview of key principles. IEEE Commun. Mag. 58 (2), 35–41. doi:10.1109/mcom.001.1900125
Bhamidi, L., and Sivasubramani, S. (2020). Optimal sizing of smart home renewable energy resources and battery under prosumer-based energy management. IEEE Syst. J. 15 (1), 105–113. doi:10.1109/jsyst.2020.2967351
Bhutta, M. N. M., and Ahmad, M. (2021). Secure identification, traceability and real-time tracking of agricultural food supply during transportation using internet of things. IEEE Access 9, 65660–65675. doi:10.1109/access.2021.3076373
Chen, J., and Yang, A. (2019). Intelligent agriculture and its key technologies based on internet of things architecture. IEEE Access 7, 77134–77141. doi:10.1109/access.2019.2921391
Choi, T. M., Wen, X., Sun, X., and Chung, S. H. (2019). The mean-variance approach for global supply chain risk analysis with air logistics in the blockchain technology era. Transp. Res. Part E Logist. Transp. Rev. 127, 178–191. doi:10.1016/j.tre.2019.05.007
Cicconetti, C., Conti, M., and Passarella, A. (2020). A decentralized framework for serverless edge computing in the internet of things. IEEE Trans. Netw. Serv. Manage. 18 (2), 2166–2180. doi:10.1109/tnsm.2020.3023305
Delnevo, G., Girau, R., Ceccarini, C., and Prandi, C. (2021). A deep learning and social IoT approach for plants disease prediction toward a sustainable agriculture. IEEE Internet Things J., 1–9.
Dowdeswell, Barry, MacDonell, Stephen G., and MacDonell, S. G. (2020). Finding faults: A scoping study of fault diagnostics for industrial cyber–physical systems. J. Syst. Softw. 168, 110638. doi:10.1016/j.jss.2020.110638
Du, M., Zhao, Y., Liu, C., and Zhu, Z. (2021). Lifecycle cost forecast of 110 kV power transformers based on support vector regression and gray wolf optimization. Alexandria Eng. J. 60 (6), 5393–5399. doi:10.1016/j.aej.2021.04.019
Fortino, G., Savaglio, C., Spezzano, G., and Zhou, M. (2020). Internet of things as system of systems: A review of methodologies, frameworks, platforms, and tools. IEEE Trans. Syst. Man. Cybern. Syst. 51 (1), 223–236. doi:10.1109/tsmc.2020.3042898
Francisco, A., Mohammadi, N., and Taylor, J. E. (2020). Smart city digital twin–enabled energy management: Toward real-time urban building energy benchmarking. J. Manage. Eng. 36 (2), 04019045. doi:10.1061/(asce)me.1943-5479.0000741
Friha, O., Ferrag, M. A., Shu, L., Maglaras, L. A., and Wang, X. (2021). Internet of things for the future of smart agriculture: A comprehensive survey of emerging technologies. IEEE/CAA J. Autom. Sin. 8 (4), 718–752. doi:10.1109/jas.2021.1003925
Fu, S., Tang, Y., Zhang, N., Zhao, L., Wu, S., Jian, X., et al. (2020). Joint unmanned aerial vehicle (UAV) deployment and power control for Internet of Things networks. IEEE Trans. Veh. Technol. 69 (4), 4367–4378. doi:10.1109/tvt.2020.2975031
Fuller, A., Fan, Z., Day, C., and Barlow, C. (2020). Digital twin: Enabling technologies, challenges and open research. IEEE Access 8, 108952–108971. doi:10.1109/access.2020.2998358
Gebremichael, T., Ledwaba, L. P., Eldefrawy, M. H., Hancke, G. P., Pereira, N., Gidlund, M., et al. (2020). Security and privacy in the industrial internet of things: Current standards and future challenges. IEEE Access 8, 152351–152366. doi:10.1109/access.2020.3016937
Gielis, J., and Prorok, A. (2021). Improving 802.11 p for delivery of safety-critical navigation information in robot-to-robot communication networks. IEEE Commun. Mag. 59 (1), 16–21. doi:10.1109/mcom.001.2000545
Gomez, C., Minaburo, A., Toutain, L., Barthel, D., and Zuniga, J. C. (2020). IPv6 over LPWANs: Connecting low power wide area networks to the Internet (of Things). IEEE Wirel. Commun. 27 (1), 206–213. doi:10.1109/mwc.001.1900215
Gubbi, J., Buyya, R., Marusic, S., and Palaniswami, M. (2013). Internet of things (IoT): A vision, architectural elements, and future directions. Future gener. Comput. Syst. 29 (7), 1645–1660. doi:10.1016/j.future.2013.01.010
Haghighi, M. S., Nader, O., and Jolfaei, A. (2020). A computationally intelligent hierarchical authentication and key establishment framework for the internet of things. IEEE Internet Things M. 3 (4), 36–39. doi:10.1109/iotm.0001.2000014
Hammoudeh, M., Epiphaniou, G., Belguith, S., Unal, D., Adebisi, B., Baker, T., et al. (2020). A service-oriented approach for sensing in the internet of things: Intelligent transportation systems and privacy use cases. IEEE Sens. J. 21 (14), 15753–15761. doi:10.1109/jsen.2020.2981558
Hou, P., Cai, J., Qu, S., and Xu, M. (2018). Estimating missing unit process data in life cycle assessment using a similarity-based approach. Environ. Sci. Technol. 52 (9), 5259–5267. doi:10.1021/acs.est.7b05366
Hu, S., Shi, Y., Colombo, A., Karnouskos, S., and Li, X. (2021). Cloud-edge computing for cyber-physical systems and internet-of-things. IEEE Trans. Industrial Inf., 1.
Humayun, M., Jhanjhi, N. Z., Hamid, B., and Ahmed, G. (2020). Emerging smart logistics and transportation using IoT and blockchain. IEEE Internet Things M. 3 (2), 58–62. doi:10.1109/iotm.0001.1900097
Hussain, B., Du, Q., Sun, B., and Han, Z. (2020). Deep learning-based DDoS-attack detection for cyber–physical system over 5G network. IEEE Trans. Ind. Inf. 17 (2), 860–870. doi:10.1109/tii.2020.2974520
Jaleel, A., Hassan, M. A., Mahmood, T., Ghani, M. U., and Rehman, A. U. (2020). Reducing congestion in an intelligent traffic system with collaborative and adaptive signaling on the edge. IEEE Access 8, 205396–205410. doi:10.1109/access.2020.3037348
Jan, B., Farman, H., Khan, M., Talha, M., and Din, I. U. (2019). Designing a smart transportation system: An internet of things and big data approach. IEEE Wirel. Commun. 26 (4), 73–79. doi:10.1109/mwc.2019.1800512
Jbair, M., Ahmad, B., Ahmad, M. H., and Harrison, R. (2018). “Industrial cyber physical systems: A survey for control-engineering tools,” in international conference on pervasive services, 270–276.
Jia, M., Komeily, A., Wang, Y., and Srinivasan, R. S. (2019). Adopting internet of things for the development of smart buildings: A review of enabling technologies and applications. Automation Constr. 101, 111–126. doi:10.1016/j.autcon.2019.01.023
Kaur, K., Guo, S., Chen, M., and Rawat, D. B. (2021). Guest editorial: Special section on transfer learning for 5G-aided industrial internet of things. IEEE Trans. Ind. Inf. 17 (10), 7070–7074. doi:10.1109/tii.2021.3071310
Khan, S., Alvi, A. N., Khan, M. Z., Javed, M. A., Alhazmi, O. H., Bouk, S. H., et al. (2020). A novel superframe structure and optimal time slot allocation algorithm for IEEE 802.15. 4–based Internet of things. Int. J. Distributed Sens. Netw. 16 (12), 155014772098464. doi:10.1177/1550147720984645
Khattak, H. A., Shah, M. A., Khan, S., Ali, I., and Imran, M. (2019). Perception layer security in internet of things. Future Gener. Comput. Syst. 100, 144–164. doi:10.1016/j.future.2019.04.038
Kumar, A., Singh, A., Kumar, A., Singh, M. K., Mahanta, P., Mukhopadhyay, S. C., et al. (2018). Sensing technologies for monitoring intelligent buildings: A review. IEEE Sens. J. 18 (12), 4847–4860. doi:10.1109/jsen.2018.2829268
Kumar, N. M., Mohammed, M. A., Abdulkareem, K. H., Damasevicius, R., Mostafa, S. A., Maashi, M. S., et al. (2021). Artificial intelligence-based solution for sorting COVID related medical waste streams and supporting data-driven decisions for smart circular economy practice. Process Saf. Environ. Prot. 152, 1–9. doi:10.1016/j.psep.2021.06.026
Kumar, S., and Chaurasiya, V. K. (2018). A strategy for elimination of data redundancy in internet of things (IoT) based wireless sensor network (wsn). IEEE Syst. J. 13 (2), 1650–1657. doi:10.1109/jsyst.2018.2873591
Kwon, S., Yoo, H., and Shon, T. (2020). IEEE 1815.1-based power system security with bidirectional RNN-based network anomalous attack detection for cyber-physical system. IEEE Access 8, 77572–77586. doi:10.1109/access.2020.2989770
Laamarti, F., Badawi, H. F., Ding, Y., Arafsha, F., Hafidh, B., El Saddik, A., et al. (2020). An ISO/IEEE 11073 standardized digital twin framework for health and well-being in smart cities. IEEE Access 8, 105950–105961. doi:10.1109/access.2020.2999871
Lai, R., Qiu, X., and Wu, J. (2019). Robustness of asymmetric cyber-physical power systems against cyber attacks. IEEE Access 7, 61342–61352. doi:10.1109/access.2019.2915927
Lee, I. G., Kim, D. B., Choi, J., Park, H., Lee, S. K., Cho, J., et al. (2021). WiFi HaLow for long-range and low-power internet of things: System on chip development and performance evaluation. IEEE Commun. Mag. 59 (7), 101–107. doi:10.1109/mcom.001.2000815
Li, L., Qu, T., Liu, Y., Zhong, R. Y., Xu, G., Sun, H., et al. (2020). Sustainability assessment of intelligent manufacturing supported by digital twin. IEEE Access 8, 174988–175008. doi:10.1109/access.2020.3026541
Li, X., and Da Xu, L. (2020). A review of internet of things—Resource allocation. IEEE Internet Things J. 8 (11), 8657–8666. doi:10.1109/jiot.2020.3035542
Li, X., Wan, J., Dai, H. N., Imran, M., Xia, M., Celesti, A., et al. (2019). A hybrid computing solution and resource scheduling strategy for edge computing in smart manufacturing. IEEE Trans. Ind. Inf. 15 (7), 4225–4234. doi:10.1109/tii.2019.2899679
Liao, Z., Peng, J., Huang, J., Wang, J., Wang, J., Sharma, P. K., et al. (2020). Distributed probabilistic offloading in edge computing for 6g-enabled massive internet of things. IEEE Internet Things J. 8 (7), 5298–5308. doi:10.1109/jiot.2020.3033298
Liu, H., Meijer, S., and Yao, Z. (2020). Study of transfer station model for medical waste based on location, construction and financing. Fresenius Environ. Bull. 29 (9), 7228–7238.
Liu, L., Larsson, E. G., Yu, W., Popovski, P., Stefanovic, C., De Carvalho, E., et al. (2018). Sparse signal processing for grant-free massive connectivity: A future paradigm for random access protocols in the internet of things. IEEE Signal Process. Mag. 35 (5), 88–99. doi:10.1109/msp.2018.2844952
Luo, X. G., Zhang, H. B., Zhang, Z. L., Yu, Y., and Li, K. (2019). A new framework of intelligent public transportation system based on the internet of things. IEEE Access 7, 55290–55304. doi:10.1109/access.2019.2913288
Lv, Z., Chen, D., Lou, R., and Alazab, A. (2021). Artificial intelligence for securing industrial-based cyber–physical systems. Future gener. Comput. Syst. 117, 291–298. doi:10.1016/j.future.2020.12.001
Lv, Z., Han, Y., Singh, A. K., Manogaran, G., and Lv, H. (2020a). Trustworthiness in industrial IoT systems based on artificial intelligence. IEEE Trans. Ind. Inf. 17 (2), 1496–1504. doi:10.1109/tii.2020.2994747
Lv, Z., Qiao, L., Li, J., and Song, H. (2020b). Deep-learning-enabled security issues in the internet of things. IEEE Internet Things J. 8 (12), 9531–9538. doi:10.1109/jiot.2020.3007130
Lv, Z., Qiao, L., Cai, K., and Wang, Q. (2020c). Big data analysis technology for electric vehicle networks in smart cities. IEEE Trans. Intell. Transp. Syst. 22 (3), 1807–1816. doi:10.1109/tits.2020.3008884
Lv, Z., and Kumar, N. (2020). Software defined solutions for sensors in 6G/IoE. Comput. Commun. 153, 42–47. doi:10.1016/j.comcom.2020.01.060
Lv, Z., Li, X., Wang, W., Zhang, B., Hu, J., Feng, S., et al. (2018). Government affairs service platform for smart city. Future Gener. Comput. Syst. 81, 443–451. doi:10.1016/j.future.2017.08.047
Lv, Z. (2020). Security of internet of things edge devices. Softw. Pract. Exper. 51 (12), 2446–2456. doi:10.1002/spe.2806
Lyu, L., Chen, C., Yan, J., Lin, F., Hua, C., and Guan, X. (2019). State estimation oriented wireless transmission for ubiquitous monitoring in industrial cyber-physical systems. IEEE Trans. Emerg. Top. Comput. 7 (1), 187–201. doi:10.1109/tetc.2016.2573719
Manogaran, G., Srivastava, G., Muthu, B. A., Baskar, S., Shakeel, P. M., Hsu, C. H., et al. (2020). A response-aware traffic offloading scheme using regression machine learning for user-centric large-scale internet of things. IEEE Internet Things J. 8 (5), 3360–3368. doi:10.1109/jiot.2020.3022322
Montazerolghaem, A., and Yaghmaee, M. H. (2020). Load-balanced and QoS-aware software-defined internet of things. IEEE Internet Things J. 7 (4), 3323–3337. doi:10.1109/jiot.2020.2967081
Nie, L., Ning, Z., Obaidat, M. S., Sadoun, B., Wang, H., Li, S., et al. (2020). A reinforcement learning-based network traffic prediction mechanism in intelligent internet of things. IEEE Trans. Ind. Inf. 17 (3), 2169–2180. doi:10.1109/tii.2020.3004232
Niu, X., Shao, S., Xin, C., Zhou, J., Guo, S., Chen, X., et al. (2019). Workload allocation mechanism for minimum service delay in edge computing-based power Internet of Things. IEEE Access 7, 83771–83784. doi:10.1109/access.2019.2920325
Perboli, G., Musso, S., and Rosano, M. (2018). Blockchain in logistics and supply chain: A lean approach for designing real-world use cases. Ieee Access 6, 62018–62028. doi:10.1109/access.2018.2875782
Philip, N. Y., Rodrigues, J. J., Wang, H., Fong, S. J., and Chen, J. (2021). Internet of things for in-home health monitoring systems: Current advances, challenges and future directions. IEEE J. Sel. Areas Commun. 39 (2), 300–310. doi:10.1109/jsac.2020.3042421
Plageras, A. P., Psannis, K. E., Stergiou, C., Wang, H., and Gupta, B. B. (2018). Efficient IoT-based sensor BIG Data collection–processing and analysis in smart buildings. Future Gener. Comput. Syst. 82, 349–357. doi:10.1016/j.future.2017.09.082
Puthal, D., Malik, N., Mohanty, S. P., Kougianos, E., and Yang, C. (2018). The blockchain as a decentralized security framework [future directions]. IEEE Consum. Electron. Mag. 7 (2), 18–21. doi:10.1109/mce.2017.2776459
Qiu, J., Du, L., Zhang, D., Su, S., and Tian, Z. (2019). Nei-TTE: Intelligent traffic time estimation based on fine-grained time derivation of road segments for smart city. IEEE Trans. Ind. Inf. 16 (4), 2659–2666. doi:10.1109/tii.2019.2943906
Qu, Y., Yu, S., Zhou, W., and Tian, Y. (2020). Gan-driven personalized spatial-temporal private data sharing in cyber-physical social systems. IEEE Trans. Netw. Sci. Eng. 7 (4), 2576–2586. doi:10.1109/tnse.2020.3001061
Rahman, M. A., and Hossain, M. S. (2021). An internet-of-medical-things-enabled edge computing framework for tackling Covid-19. IEEE Internet Things J. 8 (21), 15847–15854. doi:10.1109/jiot.2021.3051080
Rahman, S. A., Tout, H., Talhi, C., and Mourad, A. (2020). Internet of things intrusion detection: Centralized, on-device, or federated learning? IEEE Netw. 34 (6), 310–317. doi:10.1109/mnet.011.2000286
Sadowski, S., and Spachos, P. (2020). Wireless technologies for smart agricultural monitoring using internet of things devices with energy harvesting capabilities. Comput. Electron. Agric. 172, 105338. doi:10.1016/j.compag.2020.105338
Saračević, M. H., Adamović, S. Z., Miškovic, V. A., Elhoseny, M., Maček, N. D., Selim, M. M., et al. (2020). Data encryption for Internet of Things applications based on Catalan objects and two combinatorial structures. IEEE Trans. Reliab. 70 (2), 819–830. doi:10.1109/tr.2020.3010973
Shahbazi, Z., and Byun, Y. C. (2021). Improving transactional data system based on an edge computing–blockchain–machine learning integrated framework. Processes 9 (1), 92. doi:10.3390/pr9010092
Sodhro, A. H., Obaidat, M. S., Abbasi, Q. H., Pace, P., Pirbhulal, S., Fortino, G., et al. (2019). Quality of service optimization in an IoT-driven intelligent transportation system. IEEE Wirel. Commun. 26 (6), 10–17. doi:10.1109/mwc.001.1900085
Sodhro, A. H., Pirbhulal, S., Sodhro, G. H., Muzammal, M., Zongwei, L., Gurtov, A., et al. (2020). Towards 5G-enabled self adaptive green and reliable communication in intelligent transportation system. IEEE Trans. Intelligent Transp. Syst., 1–9.
Song, Y., Yu, F. R., Zhou, L., Yang, X., and He, Z. (2020). Applications of the internet of things (IoT) in smart logistics: A comprehensive survey. IEEE Internet Things J. 8 (6), 4250–4274. doi:10.1109/jiot.2020.3034385
Spachos, P., Papapanagiotou, I., and Plataniotis, K. N. (2018). Microlocation for smart buildings in the era of the internet of things: A survey of technologies, techniques, and approaches. IEEE Signal Process. Mag. 35 (5), 140–152. doi:10.1109/msp.2018.2846804
Sun, Y., Li, X., Lv, F., and Hu, B. (2021). Research on logistics information blockchain data query algorithm based on searchable encryption. IEEE Access 9, 1–12. doi:10.1109/ACCESS.2021.3054557
Tan, L., Shi, N., Yang, C., and Yu, K. (2020). A blockchain-based access control framework for cyber-physical-social system big data. IEEE Access 8, 77215–77226. doi:10.1109/access.2020.2988951
Tao, F., Cheng, J., and Qi, Q. (2018). IIHub: An industrial internet-of-things hub toward smart manufacturing based on cyber-physical system. IEEE Trans. Ind. Inf. 14 (5), 2271–2280. doi:10.1109/tii.2017.2759178
Torkayesh, A. E., Deveci, M., Torkayesh, S. E., and Tirkolaee, E. B. (2021). Analyzing failures in adoption of smart technologies for medical waste management systems: A type-2 neutrosophic-based approach. Environ. Sci. Pollut. Res. Int., 1–14. doi:10.1007/s11356-021-16228-9
Verma, S., Kaur, S., Khan, M. A., and Sehdev, P. S. (2020). Toward green communication in 6G-enabled massive internet of things. IEEE Internet Things J. 8 (7), 5408–5415. doi:10.1109/jiot.2020.3038804
Wang, X., Wang, C., Li, X., Leung, V. C., and Taleb, T. (2020). Federated deep reinforcement learning for Internet of Things with decentralized cooperative edge caching. IEEE Internet Things J. 7 (10), 9441–9455. doi:10.1109/jiot.2020.2986803
Wang, T., Qiu, L., Sangaiah, A. K., Liu, A., Bhuiyan, M. Z. A., Ma, Y., et al. (2020). Edge-computing-based trustworthy data collection model in the internet of things. IEEE Internet Things J. 7 (5), 4218–4227. doi:10.1109/jiot.2020.2966870
Wang, Z., Huang, L., and He, C. X. (2019). A multi-objective and multi-period optimization model for urban healthcare waste’s reverse logistics network design. J. Comb. Optim. 42, 785–812. doi:10.1007/s10878-019-00499-7
White, G., Zink, A., Codecá, L., and Clarke, S. (2021). A digital twin smart city for citizen feedback. Cities 110, 103064. doi:10.1016/j.cities.2020.103064
Wu, Y. (2020). Cloud-edge orchestration for the internet of things: Architecture and AI-powered data processing. IEEE Internet Things J. 8 (16), 12792–12805. doi:10.1109/jiot.2020.3014845
Xing, Y., Lei, S., Jianing, C., Amine, F. M., Jun, W., Edmond, N., et al. (2021). A survey on smart agriculture: Development modes, technologies, and security and privacy challenges. IEEE/CAA J. Autom. Sin. 8 (2), 273–302. doi:10.1109/jas.2020.1003536
Xue, H., Ding, D., Zhang, Z., Wu, M., and Wang, H. (2021). A fuzzy system of operation safety assessment using multi-model linkage and multi-stage collaboration for in-wheel motor. IEEE Trans. Fuzzy Syst., 1–9.
Yang, J., Wang, C., Jiang, B., Song, H., and Meng, Q. (2020). Visual perception enabled industry intelligence: State of the art, challenges and prospects. IEEE Trans. Ind. Inf. 17 (3), 2204–2219. doi:10.1109/tii.2020.2998818
Yu, L., Nazir, B., and Wang, Y. (2020). Intelligent power monitoring of building equipment based on Internet of Things technology. Comput. Commun. 157, 76–84. doi:10.1016/j.comcom.2020.04.016
Yu, K., Lin, L., Alazab, M., Tan, L., and Gu, B. (2020). Deep learning-based traffic safety solution for a mixture of autonomous and manual vehicles in a 5G-enabled intelligent transportation system. IEEE Trans. Intelligent Transp. Syst., 1–11.
Zhang, K., Cao, J., Maharjan, S., and Zhang, Y. (2021). Digital twin empowered content caching in social-aware vehicular edge networks. IEEE Trans. Comput. Soc. Syst., 1–13.
Zhang, K., Long, J., Wang, X., Dai, H. N., Liang, K., Imran, M., et al. (2020). Lightweight searchable encryption protocol for industrial internet of things. IEEE Trans. Ind. Inf. 17 (6), 4248–4259. doi:10.1109/tii.2020.3014168
Zhang, S., Ding, Z., and Cui, S. (2019). Introducing hypergraph signal processing: Theoretical foundation and practical applications. IEEE Internet Things J. 7 (1), 639–660. doi:10.1109/jiot.2019.2950213
Zhang, Y., Ma, X., Zhang, J., Hossain, M. S., Muhammad, G., Amin, S. U., et al. (2019a). Edge intelligence in the cognitive Internet of Things: Improving sensitivity and interactivity. IEEE Netw. 33 (3), 58–64. doi:10.1109/mnet.2019.1800344
Zhang, Y., Zheng, Z., Dai, H. N., and Svetinovic, D. (2019b). Guest editorial: Special section on “blockchain for industrial internet of things” in IEEE transactions on industrial informatics. IEEE Trans. Ind. Inf. 15 (6), 3514–3515. doi:10.1109/tii.2019.2913698
Zhao, H. L., Wang, L., Liu, F., Liu, H. Q., Zhang, N., Zhu, Y. W., et al. (2021). Energy, environment and economy assessment of medical waste disposal technologies in China. Sci. Total Environ. 796, 148964. doi:10.1016/j.scitotenv.2021.148964
Zhao L, L., Han, G., Li, Z., and Shu, L. (2020). Intelligent digital twin-based software-defined vehicular networks. IEEE Netw. 34 (5), 178–184. doi:10.1109/mnet.011.1900587
Zhao, Z., Zhou, W., Deng, D., Xia, J., and Fan, L. (2020). Intelligent mobile edge computing with pricing in Internet of Things. IEEE Access 8, 37727–37735. doi:10.1109/access.2020.2974249
Zhu, X. (2021). Complex event detection for commodity distribution Internet of Things model incorporating radio frequency identification and Wireless Sensor Network. Future Gener. Comput. Syst. 125, 100–111. doi:10.1016/j.future.2021.06.024
Keywords: IoT, ESER, intelligent logistics, CE, is, intelligent transportation
Citation: Lv Z (2022) Practical Application of Internet of Things in the Creation of Intelligent Services and Environments. Front. Internet Things 1:912388. doi: 10.3389/friot.2022.912388
Received: 05 April 2022; Accepted: 20 June 2022;
Published: 22 July 2022.
Edited and reviewed by:
Rajkumar Buyya, The University of Melbourne, AustraliaCopyright © 2022 Lv. This is an open-access article distributed under the terms of the Creative Commons Attribution License (CC BY). The use, distribution or reproduction in other forums is permitted, provided the original author(s) and the copyright owner(s) are credited and that the original publication in this journal is cited, in accordance with accepted academic practice. No use, distribution or reproduction is permitted which does not comply with these terms.
*Correspondence: Zhihan Lv, bHZ6aGloYW5AZ21haWwuY29t
Disclaimer: All claims expressed in this article are solely those of the authors and do not necessarily represent those of their affiliated organizations, or those of the publisher, the editors and the reviewers. Any product that may be evaluated in this article or claim that may be made by its manufacturer is not guaranteed or endorsed by the publisher.
Research integrity at Frontiers
Learn more about the work of our research integrity team to safeguard the quality of each article we publish.