- 1Faculty of Medical Sciences, Newcastle University, Newcastle Upon Tyne, United Kingdom
- 2The Royal Victoria Infirmary, Newcastle Upon Tyne Hospitals NHS Foundation Trust, Newcastle Upon Tyne, United Kingdom
- 3Moorfields Eye Hospital NHS Foundation Trust, London, United Kingdom
- 4Institute of Ophthalmology, University College London, London, United Kingdom
- 5Nuffield Department of Primary Care Health Sciences, Oxford University, Oxford, United Kingdom
- 6University of Leicester School of Business, University of Leicester, Leicester, United Kingdom
- 7Evidence Synthesis Group, Population Health Sciences Institute, Newcastle University, Newcastle Upon Tyne, United Kingdom
Introduction: Whilst a theoretical basis for implementation research is seen as advantageous, there is little clarity over if and how the application of theories, models or frameworks (TMF) impact implementation outcomes. Clinical artificial intelligence (AI) continues to receive multi-stakeholder interest and investment, yet a significant implementation gap remains. This bibliometric study aims to measure and characterize TMF application in qualitative clinical AI research to identify opportunities to improve research practice and its impact on clinical AI implementation.
Methods: Qualitative research of stakeholder perspectives on clinical AI published between January 2014 and October 2022 was systematically identified. Eligible studies were characterized by their publication type, clinical and geographical context, type of clinical AI studied, data collection method, participants and application of any TMF. Each TMF applied by eligible studies, its justification and mode of application was characterized.
Results: Of 202 eligible studies, 70 (34.7%) applied a TMF. There was an 8-fold increase in the number of publications between 2014 and 2022 but no significant increase in the proportion applying TMFs. Of the 50 TMFs applied, 40 (80%) were only applied once, with the Technology Acceptance Model applied most frequently (n = 9). Seven TMFs were novel contributions embedded within an eligible study. A minority of studies justified TMF application (n = 51,58.6%) and it was uncommon to discuss an alternative TMF or the limitations of the one selected (n = 11,12.6%). The most common way in which a TMF was applied in eligible studies was data analysis (n = 44,50.6%). Implementation guidelines or tools were explicitly referenced by 2 reports (1.0%).
Conclusion: TMFs have not been commonly applied in qualitative research of clinical AI. When TMFs have been applied there has been (i) little consensus on TMF selection (ii) limited description of selection rationale and (iii) lack of clarity over how TMFs inform research. We consider this to represent an opportunity to improve implementation science's translation to clinical AI research and clinical AI into practice by promoting the rigor and frequency of TMF application. We recommend that the finite resources of the implementation science community are diverted toward increasing accessibility and engagement with theory informed practices. The considered application of theories, models and frameworks (TMF) are thought to contribute to the impact of implementation science on the translation of innovations into real-world care. The frequency and nature of TMF use are yet to be described within digital health innovations, including the prominent field of clinical AI. A well-known implementation gap, coined as the “AI chasm” continues to limit the impact of clinical AI on real-world care. From this bibliometric study of the frequency and quality of TMF use within qualitative clinical AI research, we found that TMFs are usually not applied, their selection is highly varied between studies and there is not often a convincing rationale for their selection. Promoting the rigor and frequency of TMF use appears to present an opportunity to improve the translation of clinical AI into practice.
1. Introduction
Implementation science is a relatively young field drawing on diverse epistemological approaches and disciplines across a spectrum of research and practice (1). Its pragmatic goal of bridging know-do gaps to improve real-world healthcare necessitates this multi-disciplinary approach (2). A key aspect of implementation science is the application of theories, models or frameworks (TMF) to inform or explain implementation processes and determinants in a particular healthcare context (2, 3). In recent years TMFs addressing the implementation of interventions in healthcare organisations have accelerated and are pursued across a large and diverse literature which seeks to explore the factors shaping the implementation process (4). In line with the applications of TMFs, implementation researchers have variously employed qualitative research to explore the dynamic context and systems into which evidence-based interventions are embedded into practice by addressing the “hows and whys” of implementation (5). Drawing upon distinctive theoretical foundations, qualitative methodologies have offered a range of different analytical lenses to explore the complex processes and interactions shaping implementation through the recursive relationship between human action and the wider organisational and system context (4). Although this diversity of approach has allowed researchers to align specific research questions and objectives with particular context(s) at the policy, systems and organisational levels, at the same time it may pose challenges in informing the selection criteria for researchers to choose from the many TMFs in the field (6). This risks perpetuating or expanding implementation researchers' disconnect with practitioners, on whom implementation science's goal of improving real-world healthcare depends (7).
Healthcare interventions centering on clinical artificial intelligence (AI) appear in particular need of the proposed benefits of implementation science, as they are subject to a persistent know-do gap coined the “AI chasm” (8). Computer-based AI was conceived more than 50 years ago and has been incorporated into clinical practice through computerized decision support tools for several decades (9, 10). However, advancing computational capacity and the feasibility and potential of deep learning methods have galvanized public and professional enthusiasm for all applications of AI, including healthcare (11). The acknowledgment of this potential is formalized in the embedment of clinical AI into national healthcare strategic plans and by the recent surge of regulatory approvals issued for “software/AI as a medical device” (12–14). Despite this, there are few examples of clinical AI implemented in real-world patient care and little evidence of the benefits it has brought about (15, 16). This is in part because of the sensitivity of clinical AI interventions to technical, social and organizational variations in the context into which they are implemented and the paucity of research insights that go beyond the efficacy or effectiveness of the interventions themselves (17). TMFs offer a potential solution to this challenge as they allow insights from specific interventions and contexts to be abstracted to a degree through which they remain actionable whilst becoming transferrable across a wider range of interventions and contexts (18).
It is outside of the scope of the present study to directly assess the impact of implementation science on the translation of clinical AI to practice due to the bias and scarcity of reports of implementation success or failure (19). However, having been consistently proposed as an indicator of high-quality implementation research, the frequency and nature of TMF application to clinical AI research seem likely to influence the speed and extent of clinical AI interventions' real-world impact. To establish how the application of TMFs can most effectively support the realization of patient benefit from clinical AI, it will first be necessary to understand how they are currently applied. Given the early translational stage of most clinical AI research and the relatively low number of interventions that have been implemented to date, it seems unlikely that implementation science principals such as TMF usage are as well established as they are for other healthcare interventions. Implementation research focused on other categories of healthcare interventions has been characterized through descriptive summaries of TMF selection and usage. These studies act as a frame of reference, but to our knowledge none report on digital health interventions (20–22).
This bibliometric study aims to measure and characterize the application of TMFs in qualitative clinical AI research. These data are intended to (i) identify TMFs applied in contemporary clinical AI research, (ii) provide insight into implementation research practices in clinical AI and (iii) inform strategies which may improve the efficacy of implementation science in clinical AI research.
2. Methods
Mobilising a definition of implementation research, e.g., research “focused on the adoption or uptake of clinical interventions by providers and/or systems of care”, for a systematic search strategy is challenged by variation in approaches to article indexing and the framing which researchers from varied disciplines lend to their work (23–25). The present study aimed to mitigate this by targeting primary qualitative research of clinical AI. Qualitative research has a foundational relationship with the application of TMFs in implementation science and its focus on understanding how implementation processes shape and are shaped by dynamic contextual factors. Developing such an understanding requires an exploration of human behaviours, perceptions, experiences, attitudes and interactions. This approach was intended to maximise the sensitivity with which clinical AI implementation research using TMFs was identified whilst maintaining a feasible specificity of the search strategy (Figure 1).
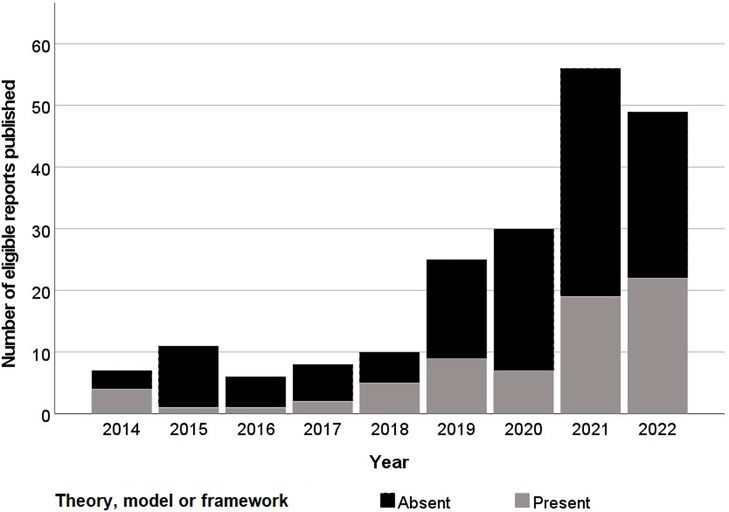
Figure 1. Histogram of year of publication of eligible reports and their application of a theory, model or framework.
This bibliometric study updates a pre-existent search strategy using AND logic to combine qualitative research with two other concepts; AI-enabled decision support including rule-based and non-rule-based tools and any healthcare context (17, 27). The earliest eligibility date of January 2014 was maintained from this prior work, marking the first FDA approvals for “Software as a Medical Device” (13), but the updated search execution included studies published up to October 2022. The five original target databases were maintained; Scopus, CINAHL (EBSCO), ACM Digital Library and Science Citation Index (Web of Science) to cover computer science, allied health, medical and grey literature (Supplementary File S1). Only English language indexing was required, there were no exclusion criteria relating to full-text language. The initial results were de-duplicated using Endnote x9.3.3 (Clarivate Analytics, PA, USA) and two independent reviewers (HDJH, MA) performed full title and abstract screening using Rayyan (28). The process was overseen by an information specialist (FB) and screening disagreements were arbitrated by a separate senior implementation researcher (GM). Eligible review and protocol manuscripts were included for reference hand searching only. Full-text review was performed independently by two independent reviewers (HDJH, MA), with the same arbiter (GM).
Two reviewers (HDJH, MA) extracted characteristics from articles independently following an initial consensus exercise. These characteristics included the year and type of publication, source field and impact factor, implementation context studied, TMF application, study methods and study participant type and number. For each study referring to a TMF in the body text, the stage of the research at which it had contributed and any justification for its selection was noted. The index article for the TMFs applied in eligible reports were sourced to facilitate characterization by a single reviewer (HDJH) following consensus exercises with a senior implementation researcher (GM). Nilsen's 5-part taxonomy of TMF types (process models, determinant frameworks, classic theories, implementation theories and evaluation frameworks) and Liberati's taxonomy of TMFs' disciplinary roots (usability, technology acceptance, organizational theories and practice theories) were applied to characterize each TMF along with its year of publication (29, 30).
3. Results
3.1. Eligible study characteristics
Following initial deduplication 6,653 potential eligible titles were returned by searches, 519 (7.8%) of which were included following title and abstract screening. Full-text screening identified 202 unique eligible studies (Figure 1). Three (1.5%) of these reports were theses with the remaining 198 (98.5%) consisting of articles in academic journals (Table 1). Excluding 2016, the frequency of eligible publication increased year-on-year, with a monthly rate of 4.9 publications averaged over January-October 2022 compared to 0.6 between January-December 2014 (Figure 2). Thirty-five different countries hosted the healthcare context under study, with the United States (n = 56, 27.7%), United Kingdom (n = 29, 14.4%), Canada (n = 16, 8.0%), Australia (n = 16, 7.9%) and Germany (n = 11, 5.4%) the most frequent countries studied. Six studies (3.0%) were based in countries categorized by the United Nations as having a medium or low human development index (31). Of the 172 studies focused on a single clinical specialty, primary care (n = 48, 27.9%) and psychiatry (n = 16, 9.3%) were the most common of 27 distinct clinical specialties.
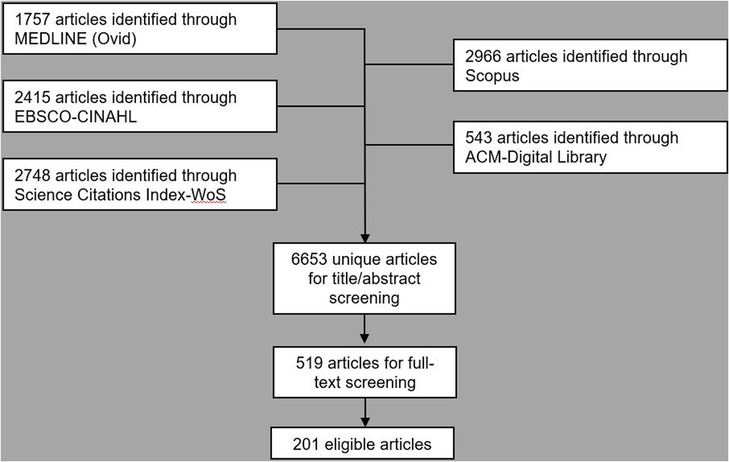
Figure 2. PRISMA style flowchart of database searching, de-duplication and title, abstract and full-text screening (26).
3.2. Theory, model or framework characteristics
Seventy eligible reports (34.7%) applied at least one of 50 distinct TMFs in the main text (Table 2), 7 (14.0%) of these were new TMFs developed within the eligible article itself. Theory application was increasingly prevalent as studies focused closer toward real-world use, with studies of hypothetical, simulated or active clinical use cases applying TMFs in 26.9%, 34.8% and 42.3% of studies respectively. There was no significant difference between the frequency of TMF application before and after the start of 2021, the median year of publication (Chi squared test, p = 0.17). Twelve (17.1%) of the 70 reports drawing on a TMF applied more than one [maximum 5 (82)]. Of the 87 instances that a TMF was applied it originated from the fields of technology acceptance (n = 36, 41.4%), practice theory (n = 21, 24.1%), organizational theory (n = 19, 21.8%) or usability (n = 11, 12.6%) according to Liberati's taxonomy (30). Similarly, under Nilsen's taxonomy of TMFs the purpose of each TMF applied could be classified as determinant framework (n = 49, 56.3%), process model (n = 18, 20.7%), classic theory (n = 10, 11.5%), evaluation framework (n = 9, 10.3%) or implementation theory (n = 1, 1.1%) (29).
3.3. Justification and application of theories, models and frameworks
The Technology Acceptance Model was the most frequent choice when a TMF was applied (n = 9, 12.9%), but 40 (80.0%) of the TMFs were only applied once across all eligible reports. Across the 87 instances of reports explicitly applying a TMF, 4 different modes of application emerged; to inform the study or intervention design (n = 9, 10.3%), to inform data collection (n = 29, 33.3%), to inform data analysis (n = 44, 50.6%) and to relate or disseminate findings to the literature (n = 25, 28.7%). The majority of instances in which a report applied a TMF carried no explanation or justification (n = 51, 58.6%). Five (5.7%) reports made isolated endorsement of the TMF's popularity or quality, e.g., “The sociotechnical approach has been applied widely…” (83). Thirty-one (35.6%) outlined the alignment of the TMF and the present research question, e.g., “our findings are consistent with disruptive innovation theory…” (84). Eleven (12.6%) reports discussed the disadvantages and alternatives that had been considered, e.g., “Because this model does not consider the unique characteristics of the clinical setting… we further adopted qualitative research techniques based on the CFIR [Consolidated Framework for Implementation Research] to further identify barriers and facilitators of the AI-based CDSS [Clinical Decision Support System]” (85).
4. Discussion
4.1. Principal findings
This study shows that a minority of clinical AI qualitative research applies TMFs, with no suggestion of a change in the relative frequency of TMF application over time. This appears to contrast with research funders and policy makers increasingly valuing more theory-based definitions of evidence and the consistent requirement for TMFs in related reporting guidelines and evaluation criteria (25, 86–88). Underlying this increasing appreciation of the contribution that TMFs can make, is a perception that specific research questions with unique configurations of complexity can draw on prior knowledge through the application of a well-matched theoretical approach (29). It is the great variety of unique research questions that may justify the ever-increasing variety of available TMFs. If considered matching of a specific research question's demands and a specific TMF's value is not taking place however, the ongoing proliferation of TMFs may only serve to further alienate practitioners trying to make sense of the shifting landscape of TMFs (7).
Within this study's relatively narrow eligibility criterion of qualitative clinical AI research, the variety and inconsistency of TMFs applied was striking, with 80% of the 50 TMFs encountered only applied once. This variation in TMF selection was also mirrored by the their varied purpose and mode of application. Across these applications of TMFs, a convincing rationale for their selection was usually absent. This heterogenous TMF selection coupled with little evidence of considered selection, suggests that current TMF application in qualitative clinical AI research usually fails to satisfy established definitions of good practice in implementation research (2, 25). If it is assumed that meeting these definitions of good practice would more effectively support implementation science's goal of bridging know-do-gaps, then it seems likely TMF application is currently under-delivering for efforts to translate clinical AI into practice. The observed heterogeneity in TMF selection is also set to grow, as 15% of the theories applied in eligible articles were novel. This may improve current practice in TMF application if these novel TMFs better serve the needs of research questions in clinical AI implementation. However, only 1 of these 7 novel TMFs has been applied within the other eligible reports of this bibliometric study and so there is a real risk of exacerbating unjustified heterogeneity in TMF usage (45).
4.2. Comparison with prior work
To the best of our knowledge, there are no other reviews of TMF application in qualitative implementation research of digital health. Smaller scoping reviews concerning specific disease areas and clinical guideline implementation, and a survey of implementation scientist practices are published, but their findings differ to the present study's in two important regards. Firstly, the heterogeneity of TMF application selection appears to be much greater in the present study, with half of guideline implementation studies applying at least one of the same 2 TMFs (20, 21). The preferences across implementation scientists in general also seem to differ from researchers working on clinical AI implementation as only 2 of the TMFs identified in the present study (Theoretical Domains Framework and Consolidated Framework for Implementation Research) appeared in the 10 most frequently applied TMFs from a survey of an international cohort of 223 implementation scientists (6). These differing preferences may be accounted for by the prominence of TMFs in qualitative clinical AI research from Technology Acceptance disciplines (40.9%), as described by Liberati's taxonomy, which do not have such natural relevance across implementation science as a whole (30). Secondly, the frequency with which any degree of rationale for TMF selection was described in the present study (42%) appears much lower than the 83% observed in guideline implementation research (21). Both of these differences seem to reflect the field of clinical AI and its nascent engagement with formally trained implementation scientists who have more established means of selecting TMFs (6). Taken together, the heterogenous and unjustified selection of TMFs suggests superficial use or misuse of TMFs is common and that clinical AI research is yet to benefit from the full value of TMF-research question alignment experienced by other areas of implementation research (18, 25, 86–89). Given the potential of unjustified heterogeneity to lower the accessibility of implementation research to relevant stakeholders, avoidance of TMF application may be preferable to their superficial use or misuse (6).
There are a number of tools which have been designed, validated and disseminated to reduce the underuse, misuse and superficial use of TMFs demonstrated here and in implementation research generally (2, 90). To aid researchers in the rationalised selection of TMFs, interactive open access libraries and selection tools are available with embedded learning resources (91, 92). Following selection of a TMF, many of the authors of more prominent TMFs develop and maintain toolkits to support the appropriate and effective mobilization of their TMF to varied applications (93, 94). There are also reporting guidelines and quality criteria which support peer reviewers and academic journal editors in identifying quality research and incentivizing researchers to adopt good practices. Apart from occasional exceptions in the present study however, none of these tools were mentioned or used (86, 89, 95, 96). The present study adds to these resources for implementation researchers working in clinical AI by summarizing TMF use to date within the field, with examples of good practice (55, 56, 85). Paradoxically, it seems that the limitation on improving TMF application is not the presence of solutions, but their implementation.
4.3. Strengths and limitations
A strength of this study is the eligibility criteria, which facilitated the large number of eligible articles relative to pre-existent bibliometric studies of TMF applications in implementation research (20–22). The study also summarizes TMF applications in clinical AI research, a prominent and growing category of digital health implementation research which had not yet been subject to any similar bibliometric studies. Without clear incentives for authors to report the perceived impact, mode or rationale of TMF application, a lack of information in eligible articles for the present study does not exclude a theoretical foundation. This risk of over-interpreting negative findings is not unique to the present study but is a further limitation to hold in mind (97). A final limitation comes from the eligibility criteria for the present study which focus on qualitative research of clinical AI, to maximise the representation of TMFs among eligible articles at the cost of implementation studies which exclusively use quantitative methods. Whilst this does limit comparability to bibliometric studies of guideline implementation research or other areas, it appears to have succeeded in identifying a greater sample of TMF applications within clinical AI than found by alternative criteria in more established fields of research (20, 21).
4.4. Future directions
Firstly, the ambiguity over the value of ensuring that implementation research that is “theoretically informed”, in a well-characterized and reproducible way, should be minimized through adequately resourced programmes of research. This is not in order to generate more TMFs, but to establish the impact of TMF application under current definitions of good practice. Without it, the challenge laid out in one of the first issues of the journal Implementation Science will continue to limit support from stakeholders influencing the implementation of TMFs: “Until there is empirical evidence that interventions designed using theories are generally superior in impact on behavior choice to interventions not so designed, the choice to use or not use formal theory in implementation research should remain a personal judgment” (19). A negative finding would also prevent future research waste in championing the proliferation and application of TMFs.
Secondly, if TMFs are proven to improve implementation outcomes then scalable impact within clinical AI and elsewhere cannot depend upon the oversight of implementation experts on any more than a small number of high priority implementation endeavors. Therefore, work to improve the accessibility and apparent value of existent TMFs and tools to promote their uptake should be prioritized (2, 91, 92). A focus on training and capacity building across a wider community of researchers and practitioners may also be beneficial (92, 98). Academic journal editors and grant administrators could be influential in endorsing or demanding relevant tools and guidelines, helping to improve the quality, consistency and transparency of theoretically informed clinical AI implementation research. Improved accessibility across existent TMFs would also help to tighten the relationship between frequency of application and efficacy of TMFs, helping to reduce the potentially overwhelming variety of TMFs available. If such a shortlist of popular TMFs emerged, with a clearer rationale and value for application, it could improve the accessibility of TMFs to a greater breadth of the implementation community. This could establish a virtuous cycle of improving frequency and quality of TMF application, mitigating against the researcher-practitioner divide described in implementation science (7).
5. Conclusion
Around a third of primary qualitative clinical AI research draws on a TMF, with no evidence of change in that rate. The selection of TMFs in these studies is extremely varied and often unaccompanied by any explicit rationale, which appears distinct from other areas of implementation research. In the context of the continual proliferation of TMFs and well-validated tools and guidelines to support their application, these data suggest that it is the implementation of interventions to support theoretically informed research, not their development, that limits clinical AI implementation research. Attempts to capture the full value of TMFs to expedite the translation of clinical AI interventions into practice should focus on promoting the rigor and frequency of their application.
Author contributions
HH: contributed to the conception and design of the work, the acquisition, analysis and interpretation of the data and drafted the manuscript. MA: contributed to the acquisition and analysis of the data. PK: contributed to the design and conception of the work and revised the manuscript. GH: contributed to the interpretation of data and revised the manuscript. FB: contributed to the design of the work, the acquisition, analysis and interpretation of data and revised the manuscript. GM: contributed to the conception and design of the work, the data acquisition, analysis and interpretation of data and revised the manuscript. All authors approved the submitted version and all authors agree to be personally accountable for their own contributions and to ensure that questions related to the accuracy or integrity of any part of the work are appropriately investigated, resolved and the resolution documented in the literature. All authors contributed to the article and approved the submitted version.
Funding
This study is funded by the National Institute for Health Research (NIHR) through the academic foundation programme for the second author (MA) and through a doctoral fellowship (NIHR301467) for the first author (HDJH). The funder had no role in the design or delivery of this study.
Conflict of interest
The authors declare that the research was conducted in the absence of any commercial or financial relationships that could be construed as a potential conflict of interest.
Publisher's note
All claims expressed in this article are solely those of the authors and do not necessarily represent those of their affiliated organizations, or those of the publisher, the editors and the reviewers. Any product that may be evaluated in this article, or claim that may be made by its manufacturer, is not guaranteed or endorsed by the publisher.
Supplementary material
The Supplementary Material for this article can be found online at: https://www.frontiersin.org/articles/10.3389/frhs.2023.1161822/full#supplementary-material
References
1. Eccles MP, Mittman BS. Welcome to implementation science. Implement Sci. (2006) 1(1):1. doi: 10.1186/1748-5908-6-32
2. Hull L, Goulding L, Khadjesari Z, Davis R, Healey A, Bakolis I, et al. Designing high-quality implementation research: development, application, feasibility and preliminary evaluation of the implementation science research development (ImpRes) tool and guide. Implement Sci. (2019) 14(1):80. doi: 10.1186/s13012-019-0897-z
3. Maniatopoulos G, Hunter DJ, Erskine J, Hudson B. Implementing the new care models in the NHS: reconfiguring the multilevel nature of context to make it happen. In: Nugus P, Rodriguez C, Denis JL, Chênevert D, editors. Transitions and boundaries in the coordination and reform of health services. Organizational behaviour in healthcare. Cham: Palgrave Macmillan (2020) p. 3–27. doi: 10.1007/978-3-030-26684-4_1
4. Maniatopoulos G, Hunter DJ, Erskine J, Hudson B. Large-scale health system transformation in the United Kingdom. J Health Organ Manag. (2020) 34(3):325–44. doi: 10.1108/JHOM-05-2019-0144
5. Hamilton AB, Finley EP. Qualitative methods in implementation research: an introduction. Psychiatry Res. (2019) 280:112516. doi: 10.1016/j.psychres.2019.112516
6. Birken SA, Powell BJ, Shea CM, Haines ER, Alexis Kirk M, Leeman J, et al. Criteria for selecting implementation science theories and frameworks: results from an international survey. Implement Sci. (2017) 12(1):124. doi: 10.1186/s13012-017-0656-y
7. Rapport F, Smith J, Hutchinson K, Clay-Williams R, Churruca K, Bierbaum M, et al. Too much theory and not enough practice? The challenge of implementation science application in healthcare practice. J Eval Clin Pract. (2022) 28(6):991–1002. doi: 10.1111/jep.13600
8. Topol EJ. High-performance medicine: the convergence of human and artificial intelligence. Nat Med. (2019) 25(1):44–56. doi: 10.1038/s41591-018-0300-7
9. Turing AM. Computing machinery and intelligence. Mind. (1950) 59:433–60. doi: 10.1093/mind/LIX.236.433
10. Miller A, Moon B, Anders S, Walden R, Brown S, Montella D. Integrating computerized clinical decision support systems into clinical work: a meta-synthesis of qualitative research. Int J Med Inform. (2015) 84(12):1009–18. doi: 10.1016/j.ijmedinf.2015.09.005
11. Krizhevsky A, Sutskever I, Hinton GE. Imagenet classification with deep convolutional neural networks. C3 Adv Neural Inf Process Syst. (2012) 2:1097–105. doi: 10.1145/3065386
12. Morley J, Murphy L, Mishra A, Joshi I, Karpathakis K. Governing data and artificial intelligence for health care: developing an international understanding. JMIR Form Res. (2022) 6(1):e31623. doi: 10.2196/31623
13. Muehlematter UJ, Daniore P, Vokinger KN. Approval of artificial intelligence and machine learning-based medical devices in the USA and Europe (2015–20): a comparative analysis. Lancet Digit Health. (2021) 3(3):e195–203. doi: 10.1016/S2589-7500(20)30292-2
14. Zhang J, Whebell S, Gallifant J, Budhdeo S, Mattie H, Lertvittayakumjorn P, et al. An interactive dashboard to track themes, development maturity, and global equity in clinical artificial intelligence research. Lancet Digit Health. (2022) 4(4):e212–3. doi: 10.1016/S2589-7500(22)00032-2
15. Yin J, Ngiam KY, Teo HH. Role of artificial intelligence applications in real-life clinical practice: systematic review. J Med Internet Res. (2021) 23(4):e25759. doi: 10.2196/25759
16. Sendak M, Gulamali F, Balu S. Overcoming the activation energy required to unlock the value of AI in healthcare. In: Agrawal A, editors. The economics of artificial intelligence: Health care challenges. Chicago, USA: University of Chicago Press (2022).
17. Hogg HDJ, Al-Zubaidy M, Technology Enhanced Macular Services Study Reference Group, Talks J, Denniston AK, Kelly CJ, et al. Stakeholder perspectives of clinical artificial intelligence implementation: systematic review of qualitative evidence. J Med Internet Res. (2023) 25:e39742. doi: 10.2196/39742
18. Kislov R, Pope C, Martin GP, Wilson PM. Harnessing the power of theorising in implementation science. Implement Sci. (2019) 14(1):103. doi: 10.1186/s13012-019-0957-4
19. Bhattacharyya O, Reeves S, Garfinkel S, Zwarenstein M. Designing theoretically-informed implementation interventions: fine in theory, but evidence of effectiveness in practice is needed. Implement Sci. (2006) 1:5. doi: 10.1186/1748-5908-1-5
20. Peters S, Sukumar K, Blanchard S, Ramasamy A, Malinowski J, Ginex P, et al. Trends in guideline implementation: an updated scoping review. Implement Sci. (2022) 17(1):50. doi: 10.1186/s13012-022-01223-6
21. Liang L, Bernhardsson S, Vernooij RW, Armstrong MJ, Bussières A, Brouwers MC, et al. Use of theory to plan or evaluate guideline implementation among physicians: a scoping review. Implement Sci. (2017) 12(1):26. doi: 10.1186/s13012-017-0557-0
22. Lima do Vale MR, Farmer A, Ball GDC, Gokiert R, Maximova K, Thorlakson J. Implementation of healthy eating interventions in center-based childcare: the selection, application, and reporting of theories, models, and frameworks. Am J Health Promot. (2020) 34(4):402–17. doi: 10.1177/0890117119895951
23. Stetler CB, Mittman BS, Francis J. Overview of the VA quality enhancement research initiative (QUERI) and QUERI theme articles: qUERI series. Implement Sci. (2008) 3:8. doi: 10.1186/1748-5908-3-8
24. Boulton R, Sandall J, Sevdalis N. The cultural politics of ‘implementation science’. J Med Humanit. (2020) 41(3):379–94. doi: 10.1007/s10912-020-09607-9
25. Pinnock H, Barwick M, Carpenter CR, Eldridge S, Grandes G, Griffiths CJ, et al. Standards for reporting implementation studies (StaRI) statement. Br Med J. (2017) 356:i6795. doi: 10.1136/bmj.i6795
26. Page MJ, McKenzie JE, Bossuyt PM, Boutron I, Hoffmann TC, Mulrow CD, et al. Updating guidance for reporting systematic reviews: development of the PRISMA 2020 statement. J Clin Epidemiol. (2021) 134:103–12. doi: 10.1016/j.jclinepi.2021.02.003
27. Al-Zubaidy M, Hogg HDJ, Maniatopoulos G, Talks J, Teare MD, Keane PA, et al. Stakeholder perspectives on clinical decision support tools to inform clinical artificial intelligence implementation: protocol for a framework synthesis for qualitative evidence. JMIR Res Protoc. (2022) 11(4):e33145. doi: 10.2196/33145
28. Ouzzani M, Hammady H, Fedorowicz Z, Elmagarmid A. Rayyan-a web and mobile app for systematic reviews. Syst Rev. (2016) 5(1):210. doi: 10.1186/s13643-016-0384-4
29. Nilsen P. Making sense of implementation theories, models and frameworks. Implement Sci. (2015) 10(1):53. doi: 10.1186/s13012-015-0242-0
30. Liberati EG, Ruggiero F, Galuppo L, Gorli M, González-Lorenzo M, Maraldi M, et al. What hinders the uptake of computerized decision support systems in hospitals? A qualitative study and framework for implementation. Implement Sci. (2017) 12(1):113. doi: 10.1186/s13012-017-0644-2
32. Pathman DE, Konrad TR, Freed GL, Freeman VA, Koch GG. The awareness-to-adherence model of the steps to clinical guideline compliance. The case of pediatric vaccine recommendations. Med Care. (1996) 34(9):873–89. doi: 10.1097/00005650-199609000-00002
33. Michie S, Richardson M, Johnston M, Abraham C, Francis J, Hardeman W, et al. The behavior change technique taxonomy (v1) of 93 hierarchically clustered techniques: building an international consensus for the reporting of behavior change interventions. Ann Behav Med. (2013) 46(1):81–95. doi: 10.1007/s12160-013-9486-6
34. Bandura A. Self-efficacy: toward a unifying theory of behavioral change. Psychol Rev. (1977) 84:191–215. doi: 10.1037/0033-295X.84.2.191
35. Michie S, van Stralen MM, West R. The behaviour change wheel: a new method for characterising and designing behaviour change interventions. Implement Sci. (2011) 6(1):42. doi: 10.1186/1748-5908-6-42
36. Pollock N, Williams R. e-Infrastructures: how do we know and understand them? Strategic ethnography and the biography of artefacts. Comput Support Coop Work (CSCW). (2010) 19(6):521–56. doi: 10.1007/s10606-010-9129-4
37. Damschroder LJ, Aron DC, Keith RE, Kirsh SR, Alexander JA, Lowery JC. Fostering implementation of health services research findings into practice: a consolidated framework for advancing implementation science. Implement Sci. (2009) 4:50. doi: 10.1186/1748-5908-4-50
38. Price M, Lau F. The clinical adoption meta-model: a temporal meta-model describing the clinical adoption of health information systems. BMC Med Inform Decis Mak. (2014) 14(1):43. doi: 10.1186/1472-6947-14-43
39. Brown B, Gude WT, Blakeman T, van der Veer SN, Ivers N, Francis JJ, et al. Clinical performance feedback intervention theory (CP-FIT): a new theory for designing, implementing, and evaluating feedback in health care based on a systematic review and meta-synthesis of qualitative research. Implement Sci. (2019) 14(1):40. doi: 10.1186/s13012-019-0883-5
40. Bower JL, Christensen CM. Disruptive technologies: catching the wave. Harv Bus Rev. (1995) 73(1):43–53
41. Croskerry P. Clinical cognition and diagnostic error: applications of a dual process model of reasoning. Adv Health Sci Educ Theory Pract. (2009) 14(Suppl 1):27–35. doi: 10.1007/s10459-009-9182-2
42. Wigfield A, Eccles JS. Expectancy–value theory of achievement motivation. Contemp Educ Psychol. (2000) 25(1):68–81. doi: 10.1006/ceps.1999.1015
43. Ammenwerth E, Iller C, Mahler C. IT-adoption and the interaction of task, technology and individuals: a fit framework and a case study. BMC Med Inform Decis Mak. (2006) 6:3. doi: 10.1186/1472-6947-6-3
44. Flottorp SA, Oxman AD, Krause J, Musila NR, Wensing M, Godycki-Cwirko M, et al. A checklist for identifying determinants of practice: a systematic review and synthesis of frameworks and taxonomies of factors that prevent or enable improvements in healthcare professional practice. Implement Sci. (2013) 8:35. doi: 10.1186/1748-5908-8-35
45. Barda AJ, Horvat CM, Hochheiser H. A qualitative research framework for the design of user-centered displays of explanations for machine learning model predictions in healthcare. BMC Med Inform Decis Mak. (2020) 20(1):257. doi: 10.1186/s12911-020-01276-x
46. Richardson JP, Curtis S, Smith C, Pacyna J, Zhu X, Barry B, et al. A framework for examining patient attitudes regarding applications of artificial intelligence in healthcare. Digit Health. (2022) 8:20552076221089084. doi: 10.1177/20552076221089084
47. Cooper A. About face: the essentials of user interface design. 1st ed. ed. I. USA: John Wiley & Sons (1995).
48. Nielsen J, Molich AR. Heuristic evaluation of user interfaces, in proceedings of the SIGCHI conference on human factors in computing systems; Seattle, Washington, USA (1990). p. 249–56
49. Madsen M, Gregor S. Measuring human-computer trust. In 11th Australasian Conference on Information Systems, vol. 53, p. 6–8. Citeseer: ACIS (2000).
50. Battilana J, Casciaro T. The network secrets of great change agents. Harv Bus Rev. (2013) 91(7-8).24730170
51. Esmaeilzadeh P. Use of AI-based tools for healthcare purposes: a survey study from consumers’ perspectives. BMC Med Inform Decis Mak. (2020) 20(1):170. doi: 10.1186/s12911-020-01191-1
52. Singer SJ, Kellogg KC, Galper AB, Viola D. Enhancing the value to users of machine learning-based clinical decision support tools: a framework for iterative, collaborative development and implementation. Health Care Manage Rev. (2022) 47(2):E21–31. doi: 10.1097/HMR.0000000000000324
53. Kano N, Seraku N, Takahashi F, Tsuji S. Attractive quality and must-be quality. J Jpn Soc Qual Control. (1984) 14(2):39–48. doi: 10.20684/quality.14.2_147
54. Fernández-López M, Gómez-Pérez A, Juristo N. Methontology: from ontological art towards ontological engineering, in proc. Symposium on ontological engineering of AAAI. (1997) p. 33–40.
55. Pumplun L, Fecho M, Wahl N, Peters F, Buxmann P. Adoption of machine learning systems for medical diagnostics in clinics: qualitative interview study. J Med Internet Res. (2021) 23(10):e29301. doi: 10.2196/29301
56. Buck C, Doctor E, Hennrich J, Jöhnk J, Eymann T. General Practitioners’ attitudes toward artificial intelligence-enabled systems: interview study. J Med Internet Res. (2022) 24(1):e28916. doi: 10.2196/28916
57. Greenhalgh T, Wherton J, Papoutsi C, Lynch J, Hughes G, A'Court C, et al. Beyond adoption: a new framework for theorizing and evaluating nonadoption, abandonment, and challenges to the scale-up, spread, and sustainability of health and care technologies. J Med Internet Res. (2017) 19(11):e367. doi: 10.2196/jmir.8775
58. May C, Finch T, Mair F, Ballini L, Dowrick C, Eccles M, et al. Understanding the implementation of complex interventions in health care: the normalization process model. BMC Health Serv Res. (2007) 7:148. doi: 10.1186/1472-6963-7-148
59. May CR, Mair F, Finch T, MacFarlane A, Dowrick C, Treweek S, et al. Development of a theory of implementation and integration: normalization process theory. Implement Sci. (2009) 4:29. doi: 10.1186/1748-5908-4-29
60. Fisher AG. Uniting practice and theory in an occupational framework. 1998 eleanor clarke slagle lecture. Am J Occup Ther. (1998) 52(7):509–21. doi: 10.5014/ajot.52.7.509
62. Liberati EG, Galuppo L, Gorli M, Maraldi M, Ruggiero F, Capobussi M, et al. Barriers and facilitators to the implementation of computerized decision support systems in Italian hospitals: a grounded theory study. Recenti Prog Med. (2015) 106(4):180–91. doi: 10.1701/1830.20032
63. Söling S, Köberlein-Neu J, Müller BS, Dinh TS, Muth C, Pfaff H, et al. From sensitization to adoption? A qualitative study of the implementation of a digitally supported intervention for clinical decision making in polypharmacy. Implement Sci. (2020) 15(1):82. doi: 10.1186/s13012-020-01043-6
64. Luke DA, Calhoun A, Robichaux CB, Elliott MB, Moreland-Russell S. The program sustainability assessment tool: a new instrument for public health programs. Prev Chronic Dis. (2014) 11:130184. doi: 10.5888/pcd11.130184
65. Rasmussen J. Skills, rules, and knowledge; signals, signs, and symbols, and other distinctions in human performance models. IEEE Transactions Syst Man Cybern. (1983) SMC-13:257–66. doi: 10.1109/TSMC.1983.6313160
67. Shackel B, Richardson SJ. Human factors for informatics usability. Cambridge, UK: Cambridge University Press (1991).
68. Sittig DF, Singh H. A new sociotechnical model for studying health information technology in complex adaptive healthcare systems. Qual Saf Health Care. (2010) 19 Suppl 3(Suppl 3):i68–74. doi: 10.1136/qshc.2010.042085
69. Jack L, Kholeif AOR. Introducing strong structuration theory for informing qualitative case studies in organization, management and accounting research. Qual Res Organ Manag Int J. (2007) 2:208–25. doi: 10.1108/17465640710835364
70. Carayon P, Wooldridge A, Hoonakker P, Hundt AS, Kelly MM. SEIPS 3.0: human-centered design of the patient journey for patient safety. Appl Ergon. (2020) 84:103033. doi: 10.1016/j.apergo.2019.103033
71. Leveson NG. Applying systems thinking to analyze and learn from events. Saf Sci. (2011) 49:55–64. doi: 10.1016/j.ssci.2009.12.021
72. Davis FD. Perceived usefulness, perceived ease of use, and user acceptance of information technology. MIS Q. (1989) 13(3):319–40. doi: 10.2307/249008
73. Michie S, Johnston M, Abraham C, Lawton R, Parker D, Walker A, et al. Making psychological theory useful for implementing evidence based practice: a consensus approach. Qual Saf Health Care. (2005) 14(1):26–33. doi: 10.1136/qshc.2004.011155
74. Klein HK, Myers MD. A set of principles for conducting and evaluating interpretive field studies in information systems. MIS Q. (1999) 23:67–94. doi: 10.2307/249410
75. Santoni de Sio F, van den Hoven J. Meaningful human control over autonomous systems: a philosophical account. Front Robot AI. (2018) 5:15. doi: 10.3389/frobt.2018.00015
76. Ajzen I. The theory of planned behavior. Organ Behav Hum Decis Process. (1991) 50(2):179–211. doi: 10.1016/0749-5978(91)90020-T
77. Rosenberg MJ, Hovland CI. Cognitive, affective and behavioral components of attitudes. In: Press YU, editors. Attitude organization and change: an analysis of consistency among attitude components. New Haven: Yale University Press (1960).
78. Venkatesh V, Morris MG, Davis GB, Davis FD. User acceptance of information technology: toward a unified view. MIS Q. (2003) 27(3):425–78. doi: 10.2307/30036540
79. Scapin DL, Bastien JMC. Ergonomic criteria for evaluating the ergonomic quality of interactive systems. Behav Inf Technol. (1997) 16(4-5):220–31. doi: 10.1080/014492997119806
80. Schneider-Kamp A. The potential of AI in care optimization: insights from the user-driven co-development of a care integration system. Inquiry. (2021) 58:469580211017992. doi: 10.1177/00469580211017992
81. Wears RL, Hollnagel E, Braithwaite J. The resilience of everyday clinical work. Resilient health care, volume 2. Farnham, UK: Ashgate Publishing (2015). 328.
82. Terry AL, Kueper JK, Beleno R, Brown JB, Cejic S, Dang J, et al. Is primary health care ready for artificial intelligence? What do primary health care stakeholders say? BMC Med Inform Decis Mak. (2022) 22(1):237. doi: 10.1186/s12911-022-01984-6
83. Cresswell K, Callaghan M, Mozaffar H, Sheikh A. NHS Scotland's decision support platform: a formative qualitative evaluation. BMJ Health Care Inform. (2019) 26(1):e100022. doi: 10.1136/bmjhci-2019-100022
84. Morgenstern JD, Rosella LC, Daley MJ, Goel V, Schünemann HJ, Piggott T. AI's gonna have an impact on everything in society, so it has to have an impact on public health": a fundamental qualitative descriptive study of the implications of artificial intelligence for public health. BMC Public Health. (2021) 21(1):40. doi: 10.1186/s12889-020-10030-x
85. Fujimori R, Liu K, Soeno S, Naraba H, Ogura K, Hara K, et al. Acceptance, barriers, and facilitators to implementing artificial intelligence-based decision support systems in emergency departments: quantitative and qualitative evaluation. JMIR Form Res. (2022) 6(6):e36501. doi: 10.2196/36501
86. Skivington K, Matthews L, Simpson SA, Craig P, Baird J, Blazeby JM, et al. A new framework for developing and evaluating complex interventions: update of medical research council guidance. Br Med J. (2021) 374:n2061. doi: 10.1136/bmj.n2061
87. Crable EL, Biancarelli D, Walkey AJ, Allen CG, Proctor EK, Drainoni ML. Standardizing an approach to the evaluation of implementation science proposals. Implement Sci. (2018) 13(1):71. doi: 10.1186/s13012-018-0770-5
88. Albrecht L, Archibald M, Arseneau D, Scott SD. Development of a checklist to assess the quality of reporting of knowledge translation interventions using the workgroup for intervention development and evaluation research (WIDER) recommendations. Implement Sci. (2013) 8:52. doi: 10.1186/1748-5908-8-52
89. Hoffmann TC, Glasziou PP, Boutron I, Milne R, Perera R, Moher D, et al. Better reporting of interventions: template for intervention description and replication (TIDieR) checklist and guide. BMJ. (2014) 348:g1687. doi: 10.1136/bmj.g1687
90. Michie S, Abraham C. Interventions to change health behaviours: evidence-based or evidence-inspired? Psychol Health. (2004) 19(1):29–49. doi: 10.1080/0887044031000141199
91. Birken SA, Rohweder CL, Powell BJ, Shea CM, Scott J, Leeman J, et al. T-CaST: an implementation theory comparison and selection tool. Implement Sci. (2018) 13(1):143. doi: 10.1186/s13012-018-0836-4
92. Rabin B., Swanson K, Glasgow R, Ford B, Huebschmann A, March R, et al. Dissemination and Implementation Models in Health Research and Practice (2021). Available at: https://dissemination-implementation.org
93. Allen CG, Barbero C, Shantharam S, Moeti R. Is theory guiding our work? A scoping review on the use of implementation theories, frameworks, and models to bring community health workers into health care settings. J Public Health Manag Pract. (2019) 25(6):571–80. doi: 10.1097/PHH.0000000000000846
94. Greenhalgh T, Maylor H, Shaw S, Wherton J, Papoutsi C, Betton V, et al. The NASSS-CAT tools for understanding, guiding, monitoring, and researching technology implementation projects in health and social care: protocol for an evaluation study in real-world settings. JMIR Res Protoc. (2020) 9(5):e16861. doi: 10.2196/16861
95. Santillo M, Sivyer K, Krusche A, Mowbray F, Jones N, Peto TEA, et al. Intervention planning for antibiotic review kit (ARK): a digital and behavioural intervention to safely review and reduce antibiotic prescriptions in acute and general medicine. J Antimicrob Chemother. (2019) 74(11):3362–70. doi: 10.1093/jac/dkz333
96. Patel B, Usherwood T, Harris M, Patel A, Panaretto K, Zwar N, et al. What drives adoption of a computerised, multifaceted quality improvement intervention for cardiovascular disease management in primary healthcare settings? A mixed methods analysis using normalisation process theory. Implement Sci. (2018) 13(1):140. doi: 10.1186/s13012-018-0830-x
97. Sendak MP, Gao M, Ratliff W, Nichols M, Bedoya A, O'Brien C, et al. Looking for clinician involvement under the wrong lamp post: the need for collaboration measures. J Am Med Inform Assoc. (2021) 28(11):2541–2. doi: 10.1093/jamia/ocab129
Keywords: artificial intelligence, clinical decision support tools, implementation, qualitative research, theory, theoretical approach, bibliometric study
Citation: Hogg HDJ, Al-Zubaidy M, Keane PA, Hughes G, Beyer FR and Maniatopoulos G (2023) Evaluating the translation of implementation science to clinical artificial intelligence: a bibliometric study of qualitative research. Front. Health Serv. 3:1161822. doi: 10.3389/frhs.2023.1161822
Received: 8 February 2023; Accepted: 26 June 2023;
Published: 10 July 2023.
Edited by:
Nick Sevdalis, National University of Singapore, SingaporeReviewed by:
Per Nilsen, Linköping University, SwedenHarry Scarbrough, City University of London, United Kingdom
© 2023 Hogg, Al-Zubaidy, Keane, Hughes, Beyer and Maniatopoulos. This is an open-access article distributed under the terms of the Creative Commons Attribution License (CC BY). The use, distribution or reproduction in other forums is permitted, provided the original author(s) and the copyright owner(s) are credited and that the original publication in this journal is cited, in accordance with accepted academic practice. No use, distribution or reproduction is permitted which does not comply with these terms.
*Correspondence: H. D. J. Hogg jeffry.hogg@ncl.ac.uk