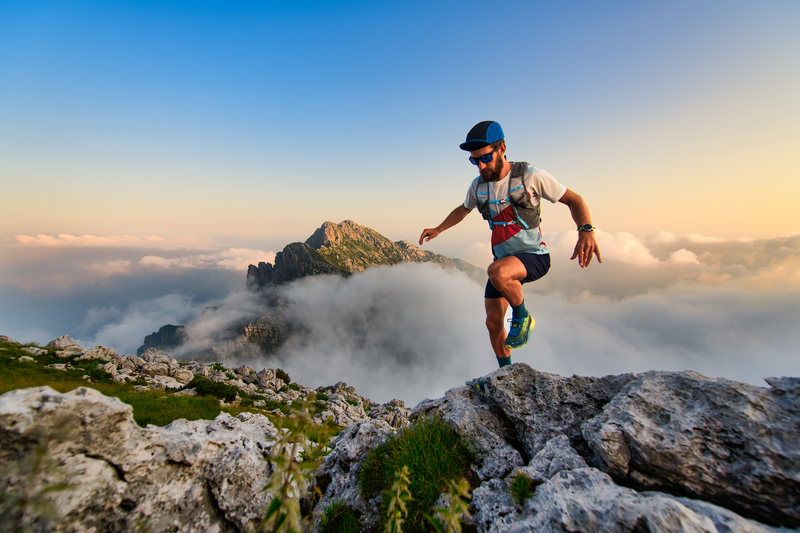
95% of researchers rate our articles as excellent or good
Learn more about the work of our research integrity team to safeguard the quality of each article we publish.
Find out more
ORIGINAL RESEARCH article
Front. Environ. Econ. , 02 July 2024
Sec. Agricultural Economics
Volume 3 - 2024 | https://doi.org/10.3389/frevc.2024.1305346
Horticulture has the potential to meet the development agenda of agrarian economies, like that of Cameroon, through the cultivation of high-value fruits and vegetables. Bananas and pineapples are two widely grown fruits in Cameroon for income, employment, and foreign earnings. Cameroon remains an important global player in the banana trade. To boost production, identifying important factors that drive the supply response of such crops is an important policy question. Here, we ask if non-price factors are important determinants of supply response of such horticultural crops, a question that has received very little attention. The objective of this study is thus to estimate the effect of non-price factors on the supply response of bananas and pineapples. To do this, a Nerlovian response function is directly estimated via an Error correction model using time series data, to capture long-run dynamics of production and supply. The results show that non-price factors such as rainfall, temperature, and land are the major drivers of the supply response of both crops. The effects of these factors, however, vary with the crops. We provide plausible explanations why non-price factors are salient. Our results suggest that improving the timely availability of weather and climate information, and input subsidies are possible areas for policy interventions.
Agriculture is Africa's heritage, and will remain vital in a foreseeable future for the continent's food and raw material supplies, as well as income and employment (Delgado, 1998; Koohafkan and Altieri, 2011; Lu and Horlu, 2019; Bjornlund et al., 2020). However, its agriculture is currently at a crucial juncture challenged by a plethora of complex problems (for instance, climate change, health, unequal markets, and unfair trading rules) impeding its progress and development. Africa is thus confronted with a multitude of complex difficulties, including the rapid growth of urban areas and a rising population in rural areas that need to be fed. The situation is recently worsened by the COVID-19 pandemic and the Russo-Ukrainian conflict, driving the skyrocketing of prices of staple foods like wheat, maize and barley (Ghufran et al., 2024; Kone et al., 2024; Ngwu et al., 2024). Notwithstanding, African agriculture has enormous potential to not only feed Africa, but also the world. African agriculture has shown promising signs of progress in recent years, with agricultural productivity increasing 13% on average every year between 2015 and 2020 (AfDB, 2021). Although there have been improvements, agriculture in the majority of African nations is still predominantly characterized by small-scale operations, limited use of advanced technologies, and reliance on rainfall. As a result, farmers and food production systems are extremely susceptible to climate-related, economic, and other shocks. Over the past few years, crop yields in Africa have been negatively impacted by droughts, cyclones, and floods. Additionally, the region is currently dealing with transboundary pest invasions and animal diseases such as desert locusts, fall armyworm, and ruminant pests. Many African farmers were significantly impacted by the disruption caused by COVID-19 in both input and output markets (Nhamo and Chapungu, 2024; Ongoma et al., 2024; Onyeaka et al., 2024).
The expanding horticulture subsector holds some promise in sub-Saharan Africa (Maertens et al., 2012; Keatinge et al., 2016; Kirsten et al., 2024). Horticulture is expanding through production intensification and by excavating new lands. With primarily fruits and vegetables, horticulture enriches diets, plays vital role in providing food and nutrition security, guarantees incomes, employment, foreign exchange and protects the environment (Weinberger and Lumpkin, 2007; Van den Broeck and Maertens, 2016; Resnick, 2024). Furthermore, horticulture can make a valuable contribution to sustainable development by cultivating fruit trees and plants that have the ability to absorb carbon dioxide, mitigate air pollution, and preserve soil fertility (Mkuna, 2022; Gelaye and Getahun, 2024; Haj-Amor et al., 2024; Sarkar et al., 2024). Additionally, it aids in the preservation of water and energy. The components of the value chain for horticulture crops generate employment and develop new market prospects for fruits and vegetables to play a crucial part in combating the triple challenges of hunger, micronutrient deficiencies, and overconsumption of starchy foods. Horticultural crops, which are primarily high-value crops, play a significant role in generating wealth. Lastly, horticulture significantly contributes to the empowerment of women and other vulnerable groups (Van den Broeck and Maertens, 2016; Mkuna, 2022; Resnick, 2024).
Although price changes are a regular occurrence in properly functioning agricultural product markets, horticulture is particularly vulnerable to such fluctuations. Agricultural commodity markets are increasingly prone to significant price fluctuations (Gouel, 2012; Oduniyi, 2022). These changes are primarily driven by supply and demand factors, such as population growth and weather conditions, which directly impact output and consequently affect prices (Fafchamps, 2000; Lanfranchi et al., 2019). The issue of price fluctuation is a complex problem caused by multiple causes that, when combined, result in harmful effects for farmers who are at risk (Fafchamps, 2000; Gouel, 2012; Boyd and Bellemare, 2020). While high prices may initially benefit farmers, price volatility poses significant risk, as it jeopardizes the investments of farmers and other stakeholders in the food industry if prices decline (Boyd and Bellemare, 2020). However, when these fluctuations in size and unpredictability increase significantly (or become volatile), they can have a detrimental effect on the ability of consumers, farmers, and entire countries to maintain food security. Typically, impoverished farmers lack sufficient financial resources to endure such volatility. This can lead to less than optimal investment decisions and undermine long-term production. The increase in food prices has not automatically resulted in improved pricing for farmers in developing nations due to the concurrent rise in costs of non-food necessities, including cooking fuel, transportation, rent, fertilizers, kerosene, and agricultural inputs. Furthermore, intermediaries are experiencing elevated transportation expenses which are subsequently transferred on to farmers.
While price fluctuations have traditionally dominated the existing literature, non-price factors significantly influence the market dynamics of the horticultural industry. Market dynamics in the horticultural sector are shaped by various non-price factors, including consumer preferences, technological advancements, and globalization. Consumer demand for fresh, locally sourced produce has surged in recent years, driven by concerns over health, environmental sustainability, and food security (Food and Agriculture Organization, 2019). Consequently, farmers must adapt their cultivation practices and product offerings to meet evolving consumer expectations, such as organic or pesticide-free produce.
Moreover, technological advancements have significantly contributed to the horticulture industry by allowing farmers to optimize crop yields, minimize losses, and enhance product quality (Lastochkina et al., 2022; Singh et al., 2022; Wani et al., 2023). Technological innovations such as precision agriculture and vertical farming have revolutionized horticultural production methods, enabling year-round cultivation in controlled environments and optimizing resource utilization (Godfray et al., 2010). These technological improvements offer the potential for increased efficiency, decreased reliance on manual labor, and a transition toward data-driven and ecologically sensitive methods of agriculture. However, the adoption of these technologies often requires significant investments, posing challenges for small-scale farmers with limited financial resources.
Globalization has also transformed market dynamics, facilitating the international trade of horticultural products. The market for horticulture has been enhanced by the increased interconnectedness of countries under the dispensation of globalization. Globalization, propelled by international trade and investment, is intricately interwoven with the changes in economies, communities, and politics that are influenced by advancements in information technologies, facilitating more global interconnectedness (Von Braun and Diaz-Bonilla, 2008; Robinson, 2018). Anderson (2023) states that globalization has significantly reshaped the world's agrifood systems through the increasing functional integration of economies worldwide. This transformation has been facilitated by the internet, the growth of transnational corporations, and the elimination of various trade and investment barriers. However, while export opportunities present lucrative prospects for farmers, they also expose them to market volatility, regulatory complexities, and competition from low-cost producers (Gómez-Limón et al., 2018). Furthermore, fluctuations in currency exchange rates and trade policies can significantly impact the profitability of horticultural enterprises, necessitating risk management strategies and market diversification efforts.
Government policies, particularly on agriculture, play a crucial role in shaping the horticultural sector, influencing production practices, market access, and sustainability initiatives. Policy initiatives aimed at supporting smallholder farmers, promoting agro-industrial development, and enhancing food safety standards are integral to fostering a conducive operating environment (Tadesse et al., 2020). However, the effectiveness of these policies often hinges on their implementation, enforcement, and alignment with stakeholders' interests. In many developing countries, agricultural policies suffer from inadequate funding, bureaucratic inefficiencies, and lack of coordination between government agencies, hindering their impact on the ground (Gatzweiler et al., 2019). Moreover, policy interventions must strike a delicate balance between incentivizing agricultural productivity and safeguarding environmental sustainability, addressing trade-offs between short-term gains and long-term resilience.
In the midst of these varied drivers, sustainability has now emerged as a paramount concern in the horticultural sector, encompassing environmental stewardship, social equity, and economic viability. Intensive agricultural practices, such as mono-cropping, excessive pesticide use, and water depletion, have raised alarms over their adverse impacts on soil health, biodiversity, and ecosystem resilience (Foley et al., 2011). Sustainable horticulture entails adopting agro ecological principles, promoting biodiversity conservation, and enhancing resource efficiency throughout the value chain.
However, transitioning to sustainable production systems entails significant challenges, including knowledge gaps, investment barriers, and market constraints (Pretty et al., 2018). While certification schemes, such as organic or fair-trade labels, offer market premiums for sustainably produced goods, their adoption may entail additional costs and certification requirements for farmers. Additionally, the fragmented nature of global supply chains complicates traceability and transparency efforts, limiting consumers' ability to make informed choices about the sustainability of horticultural products.
Stakeholder engagement is pivotal in addressing the complex challenges facing the horticultural sector, fostering collaboration among farmers, policymakers, researchers, civil society organizations, and consumers. Farmers' perspectives on market access, input subsidies, and risk management strategies inform policy formulation and implementation, ensuring that interventions are tailored to their needs and realities (Van Kooten et al., 2019). Similarly, consumer preferences for ethically sourced, environmentally friendly produce drive market demand for sustainable horticultural products, incentivizing farmers to adopt responsible practices. However, conflicting interests and power asymmetries among stakeholders can impede collective action and compromise sustainability outcomes (Darnhofer et al., 2010). Policymakers must navigate these complex dynamics, facilitating inclusive decision-making processes that accommodate diverse perspectives and prioritize the long-term interests of society and the environment.
Non-price factors are not new, although they have generally not received much attention in the supply response literature. However, they are growing in importance for at least two interrelated reasons. Firstly, they have been shown to affect price factors. Blake et al. (2018) show that poor weather conditions are a major driver of banana prices. Secondly, they have numerous effects on especially horticultural crops. Some of the most important non-price factors are varying rainfall and temperature, which are direct consequences of climate change. Several studies have highlighted that horticultural crops constitute one of the most vulnerable to the effects of climate change (Glenn et al., 2013; Gora et al., 2019; Agnolucci and De Lipsis, 2020; Abdoussalami et al., 2023). This occurs by altering important biological processes like flowering and fruiting (Joshi et al., 2023). Beyond such direct effects on production, horticultural crops are unique in that their sale depends on not only the quantity but also the quality. Hence, factors affecting fruits' quality may also affect their supply. Extreme climatic events may affect the quality of fruits. Availability of land is also an important non-price factor that may affect the production and supply of horticultural crops. In most parts of the developing world, increase in horticultural production has mostly been through land expansion. For example, Sharma et al. (2022) show that the area under cultivation of horticultural crops in India has been on a steady increase. On the one hand, this may be due to the lack of appropriate technology for intensification. On the other hand, the nature of most horticultural crops limit their growth in certain environments. Crops such as bananas are usually grown in large areas of land due to the wide area they cover and the spacing requirements for optimal growth. However, even with such expansions, the use of improved technologies like fertilizers and pesticides are still necessary.
Here, we ask if non-price factors are important drivers of supply response of horticultural crops. Agriculture hinges on important non-price factors like rainfall, temperature, and land, which have even become increasingly important in the face of climate change. However, they have received very little attention in supply response studies. Although some empirical studies Ayinde and Bessler (2014) and Tabe-Ojong et al. (2020) have shown that non-price factors are as important as price factors in supply response, price factors have been the focus of supply response in accordance with the dictates of microeconomic theory, which emphasizes prices as a major determinant of supply. Our objective is thus to estimate the effects of non-price factors on the supply response of banana and pineapples in Cameroon. We hypothesize that non-price factors are important drivers of the supply response of horticultural crops. With increasing importance of these crops to both smallholder farmers and government, identifying key non-price determinants may inform policy on the production and supply of such crops.
There is a large body of literature on the supply response of several crops such as tomato (Tabe-Ojong et al., 2020), sunflower (Nhundu et al., 2022), sorghum (Onono, 2018), rice (Ayinde and Bessler, 2014), apple and pear (Wani et al., 2015), and maize (Shoko et al., 2016; Sumathi et al., 2019). While some of these studies have focused on horticultural crops, to our knowledge, we are not aware of any studies on bananas and pineapple. We therefore contribute to the literature by focusing on these two important crops of worldwide significance. As annual crops, characterized by a longer gestation period, their supply response may vary, since farmers may take a longer time to respond to changes. Moreover, banana is globally a very popular fruit, not only for its nutritional value but also for their economic importance, especially to small and marginal farmers in developing countries. In terms of global importance and gross value of production, bananas are the fourth most important food crop after wheat, rice, and corn, being a dietary staple and providing a balanced diet to millions of people (Thrift et al., 2014). In Africa, it provides more than 25% of food energy requirements to more than 100 million people. Pineapple on the other hand is the third most important tropical fruit in the world after bananas and mangoes (Lobo and Paull, 2017). Both are extensively grown in Cameroon. Therefore, improving the supply response of these crops may play important roles in both food security and poverty, which remain serious challenges in Cameroon.
The remainder of the paper is structured as follows. Section two is the background of the study where we present the study context and conceptual framework. Section three provides the materials and methods while results are presented in section four. The paper concludes and provides some policy recommendations in section five.
The agricultural sector in Cameroon, particularly the horticultural industry such as bananas and pineapples, plays a pivotal role in the nation's economy. This industry makes a substantial contribution to employment in rural areas, export earnings, and food security. Nevertheless, in spite of its vital importance, this industry has many obstacles that impede its growth and have an impact on farmers' and agro-industrial stakeholders' supply responses (Anaciet, 2019). One of the primary challenges in Cameroon's horticultural sector is the lack of adequate infrastructure, such as roads and storage facilities, which significantly affects the supply chain efficiency. This infrastructure deficit leads to high post-harvest losses and restricts access to both local and international markets (Etame Kossi et al., 2023). Additionally, the vulnerability to climate change poses a significant threat, impacting crop yields and forcing farmers to adapt to unpredictable weather patterns (Abdoussalami et al., 2023).
The market dynamics in the banana and pineapple industries are influenced heavily by international trade agreements and local market structures. Cameroon's adherence to economic partnership agreements with the European Union has opened markets but also exposed local producers to fierce competition from Latin American banana exporters (Ollong, 2017). On the policy front, initiatives aimed at enhancing agricultural productivity through subsidies and technical support have had mixed results. While these policies intend to boost production, their implementation often suffers from administrative inefficiencies and a lack of coordination with local needs.
The perspectives of various stakeholders, including farmers, agribusinesses, government agencies, and NGOs, cannot be overemphasized in addressing the multifaceted challenges of the horticultural sector. Farmers often express concerns over the volatility of prices and the inadequacy of financial and technical support, whereas agribusinesses focus on improving productivity and market access. NGOs and international bodies emphasize the importance of sustainable practices and fair trade (Tabe-Ojong et al., 2023).
Incorporating non-price factors into supply response analysis in the context of Cameroon is crucial for several reasons that intersect economic viability, environmental sustainability, and social welfare. These factors often provide a more comprehensive understanding of agricultural dynamics that price mechanisms alone cannot explain, especially in the context of developing countries like Cameroon.
Economic diversification is a vital strategy for developing economies to stabilize against market shocks. Horticulture, involving the cultivation of fruits like bananas and pineapples, offers significant potential for diversification. Studies have shown that non-price factors such as market access and infrastructure significantly impact the economic outcomes of horticulture enterprises (Quentin et al., 2015). By understanding these factors, policymakers and stakeholders can better tailor strategies to bolster economic stability and growth, making the sector more resilient to external shocks.
Environmental sustainability in agriculture has become a pressing concern under the shadow of global climate change. The study of non-price factors such as farming practices, use of agrochemicals, and resource management is essential for promoting sustainable agriculture. The intensive use of agrochemicals in the banana and pineapple industries has raised environmental concerns, particularly regarding soil degradation and water pollution. Research has indicated that sustainable practices in horticulture not only help in preserving biodiversity but also improve soil health and reduce dependency on chemical inputs, which are critical in maintaining long-term productivity of the land (Njukwe et al., 2010). Addressing these factors can lead to more sustainable agricultural methods that align with global sustainability goals.
The horticultural sector is labor-intensive, making the study of labor conditions and social equity crucial. Socially, the labor conditions and economic benefits for local farmers remain areas requiring significant improvement to ensure that agricultural growth translates into broader community benefits. Non-price factors such as working conditions, wages, and access to social services influence the supply response by affecting labor availability and productivity. Research by Maertens and Swinnen (2012) suggest that improving labor conditions in horticultural industries in developing countries could lead to enhanced social equity and economic benefits for workers. Thus, studying these factors can help develop fair trade practices and improve living standards for workers in the sector.
Non-price factors are pivotal in evaluating the effectiveness of agricultural policies. For example, government initiatives aimed at providing technical support and enhancing market access are crucial for the growth of the horticultural sector. Studies by Minten et al. (2009) suggest that policy interventions that consider infrastructure development, technological dissemination, and market facilitation have a profound impact on the supply dynamics in agriculture. Understanding these influences can aid in crafting more effective policies that support sectoral growth and competitiveness.
Banana is an important crop in Cameroon. The Food and Agricultural Organization (2018) ranked Cameroon the first producer and exporter of bananas in Africa. The banana sector was one of the main providers of employment in Cameroon. It is produced by smallholder farmers and state-owned agro-industrial plantations. That produced by smallholders is destined for the local markets while that from plantations is exported to markets in Europe. It accounts for about 2% of agricultural products exported from the country. In 2011, about 297,210 tons of bananas were exported while the business generated about 170 billion FCFA while in 2020, production stood at over a million tons (FAOSTAT, 2022). The country is equally a major producer of pineapples with an annual production of about 300,000 tons, over a third of global production (Cameroon Radio Television, 2018; FAOSTAT, 2022; Wamuci, 2022). Like bananas, pineapples are also produced by both smallholder farmers and agro-industrial plantations. Smallholder production, however, predominates. The South, Littoral, and Center regions of Cameroon are the hub of pineapple production owing to the well-drained soils. Pineapple is sold in local markets for consumption, but some portion is equally sold for agro-industrial purposes. The country's production of banana and pineapple is shown in Figure 1A. The graph shows that banana and pineapple are major important agricultural products in Cameroon owing to their large production volumes. While production had been steadily increasing, a drop in production has characterized the last few years. It is possible that both price and non-price factors may be driving such reductions. However, there is no empirical evidence to support such claims, necessitating this study. Moreover, these crops also constitute important shares of the total value of Cameroon's agricultural production as shown in Figure 1B. Hence, understanding the drivers of their supply response is important for the country's agricultural production and exports.
Figure 1. (A) Production (in tons) of banana and pineapple in Cameroon. (B) Share of banana and pineapple in gross agricultural production value. Source: Authors' construction from FAOSTAT (2023).
Non-price factors also play an important role in Cameroon's agricultural sector. For example, the rain-fed system of agriculture implies that climatic factors are salient in determining farmers' responses. According to Tagang Tene (2022), there has been a decrease in rainfall and an increase in temperatures, despite significant heterogeneities across regions. These have significant effects on the production and supply of most crops in Cameroon. It further alters the production calendars and timing of important farming operations. Similarly, there is a constant reduction in available arable land in Cameroon owing to infrastructural development. This limits the available land for horticultural crops which are still grown on an extensive basis. These factors, in addition to limited technology use and exchange rate may play an important role in the supply response of banana and pineapple. It is worth re-iterating that there exists a dual horticultural sector in Cameroon wherein there are small-scale producers existing alongside large agro-industrial plantations.
This study is conceptualized in Figure 2. Figure 2 shows that price and non-price factors are basically determined by government agricultural policy, monetary policy instruments and climate variability factors. As per the law of supply, there is a positive relationship between the price of pineapples and bananas and the quantity supplied by farmers in Cameroon. For instance, government agricultural policy through its policy implementation on technology adoption of modern agricultural technologies, such as irrigation systems and improved crop varieties, could enhance pineapple and banana productivity in Cameroon given that technology adoption is crucial for increasing yields and improving efficiency.
As pointed out by Shoko et al. (2016) who explained that to the extent that such technologies are available and affordable to farmers, it may influence their supply response. Also, government grants and subsidies serve as incentive for producers to supply more pineapples and bananas, as it becomes more profitable to cultivate these crops compared to other substitute crops such as mango and oranges that may not receive the same attention. Monetary policy instruments such as exchange rate and consumer price influence banana and pineapple supply through non-price factors as well. For instance, fluctuations in the exchange rate can impact the competitiveness of pineapple and banana exports from Cameroon. A depreciating local currency may enhance export competitiveness, while a strengthening currency may reduce profitability (Smith, 2018). Similarly, consumer prices of alternative fruits, such as oranges and mangoes, could influence the demand for pineapples and bananas in Cameroon.
Higher prices of alternative fruits may shift consumer preferences toward pineapples and bananas, increasing demand. Finally, climate factors such as temperature and rainfall significantly affect banana and pineapple given that optimal temperature and adequate rainfall are essential for healthy plant growth and fruit development. This is in tandem with Joshi et al. (2023) who argue that temperature changes affect important processes like flowering and fruiting. Similarly, rainfall is another important non-price factor that may affect the supply response of a commodity by affecting biological processes. Also, Shoko et al. (2016) show that rainfall is one of the major drivers of supply response of maize in South Africa. Finally, the cost of inputs such as land, herbicides, fertilizers, and other agrochemicals also has a big impact on pineapple and banana supply responses.
Price fluctuations for inputs have the potential to impact farmers' production costs and profitability, which in turn might impact their choices about crop cultivation. Furthermore, market factors that affect farmers' capacity to supply customers with bananas and pineapples include price volatility, market accessibility, and transportation infrastructure. Influenced heavily by international trade agreements and local market structures, these factors have heavily affected banana and pineapple supply following Cameroon's membership in regional economic blocs, such as the Economic Community of Central African States (ECCAS) and the African Continental Free Trade Area (AfCFTA), that presents opportunities for small businesses to access larger markets within the Central African region and beyond.
As such, understanding how these factors influence supply response opens up new avenues for policy interventions aimed at horticultural production. We therefore exploit this framework to contribute to the extant literature, by estimating values that provide new information to guide practices in the industry.
The role of price and non-price factors in understanding horticultural crop supply response is becoming increasingly important in light of the most recent trade policies influencing horticulture globally under the new economic order. Siegle et al. (2024) provides an extensive methodological review of the different techniques employed in the supply response analysis. The estimation technique for this study hinges on the Nerlovian supply response framework. Yield, area, or output response can be used to model crop supply response. Perennial crops require long-term capital investment to yield short-term outputs, so an analytical approach that internalizes short-run response (within year adjustments), long-run response, and management behavior is needed. The Nerlovian supply response technique determines perennial crop short-term and long-term elasticities (French and Matthews, 1971; Akiyama and Trivedi, 1987; Siegle et al., 2024). Nerlovian models analyze farmers' output reaction based on price expectations and partial area adjustment (Nerlove, 1958; French and Matthews, 1971; Askari and Cummings, 1977). The model can also include non-price production shift variables. The Nerlovian supply response technique uses a Nerlovian expectation model to analyze the rate and level of adjustment of actual acreage and yield toward targeted acreage. Time series econometrics, including error correction models, may aptly determine short-run and long run supply sensitivity when time series data are utilized to investigate production dynamics (Nickell, 1985). In our current study, supply response would therefore, indicate the changes in output with changes in prices and non-price factors and estimation of the long-run equilibrium in the sector (Tripathi and Anubhuti, 2008).
Supply response has been estimated directly by including partial adjustments and restrictive expectations (Nerlove, 1958). This model describes the dynamics of supply by incorporating price expectations and partial area adjustment. Suppose we postulate the model, where output in period t, is Yt, as a function of expected price in the same period, P* as shown in Equation 1.
Where Yt = Banana or pineapple output in period t, P* = Expected banana or pineapple price at period t, νt = unobserved random effects affecting the banana or pineapple output and has expected value zero and constant variance, α0 = intercept and α1 = Long-run banana or pineapple price elasticity coefficient of output.
Nerlove (1958) proposes a relationship between the expected or long run and actual banana or pineapple output which holds at any time, t. Since the long-run output is not directly observable, at any time, t, actual output is adjusted in relation to the difference between the expected output in the long-run equilibrium and actual output. Equation 2 shows such a relationship.
Where Yt the actual output in period t, δ is the coefficient of adjustment, and wt is an error term. Thus, the actual output in period t is adjusted by a fraction of the difference between the long-run equilibrium or expected output and the actual output in the lagged period. If δ = 0, present and previous periods' outputs remain the same. This does not correspond to the partial adjustment hypothesis and thus a restriction is set as δ > 0. On the other hand, if δ = 1, actual output equals expected output. That is, long-rung run equilibrium is achieved fast. Generally, 0 < δ < 1 and the adjustment to long-run equilibrium is likely to be incomplete in one period for reasons as varied as toughness, inaction, enabling environment, and technical constraints, and these factors influence the dynamics of supply response. Considering this adjustment, the above equation can be rewritten as:
Equation (3) implies that actual output in period t, is a weighted average of desired output at the same time and actual output in the previous period, weighted by δ and (1- δ), respectively. If δ = 0, the actual output in period t is the same as in the previous period. Conversely, if δ = 1, actual output adjusts to long-run equilibrium within one period.
Combining price expectations and adjustment yields Equation (4)
Where ϑt = δμt+wt and all other variables are as previously defined. This model is a short-run function where short-run output, Y, may be different from its long-run output, Y*.
Agricultural output variables, such as Banana and Pineapple outputs, are essential components of the supply response model as they represent the quantity of goods produced by farmers. According to Just and Pope (2001), incorporating output variables allows for the assessment of farmers' production behavior in response to changes in input prices, technology adoption, and market conditions. Modeling the supply response of banana and pineapple producers provides insights into crop-specific production dynamics, resource allocation decisions, and market competitiveness.
Expected price variables reflect farmers' anticipations of future market conditions and price levels for bananas and pineapples. According to Orden (2002), incorporating expected price variables into supply response models enables the evaluation of farmers' forward-looking behavior and strategic decision-making in response to price signals. By including expected price variables, the Nerlovian model captures the influence of price expectations on farmers' production choices and supply responses, thereby enhancing the model's predictive accuracy and policy relevance.
The adaptive expectation model appears similar to the partial adjustment model but is very different in theory. The adaptive is based on the uncertainty of formulation of expected price, while the partial is based on technical or institutional toughness, inertia, and so on. In empirical studies, the two models are suitable for various situations. Adaptive expectations without partial adjustment (δ = 1), is suitable for the study of crop production where there are few fixed factors, and adaptive can be completed in one period; that is, Y* = Yt. Partial adjustment without adaptive expectations (δ = 1) is suitable for situations where crop prices are known at the beginning of the planting season. If we put equations in logarithmic form, the short-run price elasticities of the supply response of these models are the same, δα1, while the long-run price elasticity for both is α1.
An advantage of the Nerlovian framework is that it is quite flexible and allows other supply shifters to be incorporated into the model directly. In this light, several studies have used the Nerlovian framework to directly estimate supply response. A major weakness, however, is the problem of spurious regressions, which may arise from the characteristic non-stationary nature of most time series data. That is, variables may show a relationship even when none exists (Granger and Newbold, 1974; Granger et al., 2001). Since we use time series data in this study, we obviate this problem by employing several techniques. Firstly, we test for stationarity and employ several approaches to cater to it. We then test for the presence of co-integration vectors whose presence necessitates the estimation of an error correction model. These tests are discussed below.
A major problem that arises with the use of time series data is non-stationarity. That is, a series may contain a unit root. Failure to correct for this non-stationarity may lead to spurious regressions. Several approaches such as the Dickey-Fuller, augmented Dickey-Fuller and Phillip-Perrons test are used to test for stationarity. The Dickey-fuller tests, however, fails to use lags of variables, which limits the application of the test. However, the augmented Dickey Fuller (ADF) does and is thus argued to be a better test for stationarity (Gujarati, 2022). Moreover, it produces similar results like that Phillip-Perrons test. We employ the augmented Dickey-Fuller test (ADF) to test for stationarity. However, we support our results by also providing results of the Phillip-Perrons test. The model for the ADF test is presented as in Equation (5).
Where Y is the process to be tested, β is the test coefficient, and j is the lag length chosen for ADF such that ut is a white noise process. Here the parameter of interest is β whose statistical significance suggests the presence of a unit root and hence, non-stationarity. Therefore, if the null hypothesis of not weak stationary cannot be rejected, the variables are differenced until they become stationary.
Upon confirming the stationarity of variables, the next step consists in testing the presence of a long-run relationship between the stationary series. The presence or not of a long run relationship is primordial in determining the appropriate analysis to follow viz vector error correction (VEC) or vector autoregression (VAR; Gujarati, 2022). To test for the presence of long-run relationship, we employ the Johansen co-integration approach as shown below.
In order to test for the existence of co-integration (CI) between the series, we employ the Engle-Granger Augmented Dickey-Fuller co-integration test (EG-ADF test), which is one of the most robust co-integration tests (Punshi, 2020). This test involves two steps. The first step consists of a relation from which the residual process (zt) must be extracted. The second step is to look for a unit root in the residual process of the co-integration regression. To this end, we specify a model for the test as shown in Equation 6.
Where k is the shift chosen according to the criteria of Akaike and Schwartz. The assumptions are as follows:
H0: v = 0 (no existence of a co-integration relationship).
H1: v < 0 (existence of a co-integration relationship).
The decision rule is such that the null hypothesis of no co-integration is rejected if the calculated Mckinnon statistic is greater than the corresponding critical value. Otherwise, there would be no long-term link between variables considered (Johansen, 1988). The existence of a long-term relationship paves the way for the estimation of the error correction model (ECM).
In this study, only the standard case of CI (1,1) has been treated. Using the Engle-Granger representation theorem, if a set of variables are co-integrated in an order of I (0), there exists an ECM describing the relationship. The theorem explains that co-integration and an ECM can be used as a unified theoretical and empirical framework to analyze both short-run and long run behavior. If the series Yjt and χit are I (1) and co-integrated, then the ECM is represented in the following form shown in Equations 7 and 8.
Where, Δ is the difference operator, χt and Yt represent different series indexed by i and j, α and β are parameters to be estimated, ut and εt are white noise error-term, and ECMt − 1 is the error correction-term (adjusted vector) derived from the long-run co-integrating relationship.
Modeling Banana and Pineapple outputs in the ECM allows for the examination of production responses to changes in exogenous factors. The inclusion of output variables enables the model to capture the impact of productivity shocks, technology adoption, and market conditions on fruit production dynamics.
Consumer prices for different fruits play a crucial role in shaping demand patterns and market equilibrium. Incorporating consumer price variables into the ECM, enables to assess the price elasticity of demand for various fruits and analyze how changes in prices affect market equilibrium, producer revenues, and consumer welfare.
The exchange rate is a significant determinant of agricultural trade dynamics and market competitiveness. Including the exchange rate variable in the ECM enables us to examine the impact of currency movements on fruit exports, import substitution, and trade balance, thereby assessing the sector's vulnerability to external shocks and policy changes.
Technological innovation plays a critical role in driving productivity growth and efficiency improvements in the agricultural sector. Modeling technology variables into the ECM, enables to evaluate the effects of technological advancements, and extension services on fruit production levels, yield variability, and resource utilization.
Climate variability, represented by temperature and rainfall variables, significantly influences agricultural production and crop yields. Modeling temperature and rainfall variables in the ECM enables us to analyze the sensitivity of fruit production to climate variability, identify adaptation strategies, and assess the sector's resilience to climate change impacts.
The data for this study were obtained from secondary sources. We use production volumes of banana and pineapple as our outcome variables. Other studies have used the area harvested to estimate supply response. However, literature suggests that measuring area of cultivation is fraught with measurement errors. Hence, we prefer production quantity that is measured with relatively more precision. Moreover, production quantity has been used in estimating supply response by other studies (Ogundari, 2018; Abodi et al., 2021). Banana and pineapple production (in tons) data and their harvested areas (in hectares), were obtained from FAOSTAT.1 The consumer price for pineapple (in $/kg), the exchange rate between FCFA and US$ was equally obtained from FAOSTAT, while the consumer price of banana, mangoes, and oranges came from the World Bank commodity price data (PINK SHEET). Technology (proxied by added value into agriculture, forestry, and fisheries in million dollar/mt) was obtained from the World Bank Development Indicators. Climate variables like temperature variation in degree fahrenheit and rainfall in mm were obtained from the National oceanic and atmospheric administration and climate change knowledge portal. Our choice of variables is influenced by existing literature as well as theoretical insights (Shoko et al., 2016; Ogundari, 2018; Tabe-Ojong et al., 2020; Abodi et al., 2021).
In this section, we present the results of the diagnostic tests, which inform the final model. Since we are using time series data, it is conventional to perform diagnostic tests such as the stationarity and co-integration tests since they serve as guides to the final model to be employed. We discuss these tests and their implications for the estimation of the final model.
We first present the variables prior to testing for stationarity. Figures 3A and B show a summary of all the series that were tested for unit root and stationarity using the Augmented Dickey-Fuller test and confirmed with the Philip-Perron test. They were found to have a unit root, non-stationary and with drift. Indicative of non-stationarity are oscillation of the series away from zero. Using the series in this nature may lead to spurious regressions. Hence, the series must be made stationary. In order to render them stationary, we perform a first difference. Upon first differencing, all the series became stationary, integrated of order 1. They vibrate around zero. Similar results were obtained for pineapple.
Figure 3. (A) Differenced and stationary data for banana series. (B) Differenced and stationary data for pineapple series. Source: Authors' construction (2023).
The results of the unit root (stationarity) tests are presented in Tables 1 and 2, which represent banana and pineapple, respectively. Two types of tests are performed to confirm the stationarity of the variables. The ADF tests and the Philip-Perron tests. The 1% critical values of both tests are greater than the associated test statistics, which implies that the null hypothesis of the presence of a unit root, can be rejected, confirming the stationarity of the variables. This indicates a long term relationship among the selected variables. The results show that upon first differencing, all the variables become stationary. That is, the series become integrated of order 1 [I (1)].
Co-integration tests analyze non-stationary time-series processes that have variance and means that vary over time. Two sets of variables are co-integrated if a linear combination of those variables has a lower order of integration. For example, co-integration exists if a set of I(1) variables can be modeled with linear combinations that are I(0). The order of integration here- I(1)—indicates that a single set of differences can transform the non-stationary variables to stationarity. The co-integration tests result for banana and pineapple are presented below as trace and eigen-value tests.
Using the co-integration tests, we compare the trace statistic and the eigenvalue with the 5% critical value. We can see that there are only two co-integrating equations for bananas and only one for pineapple. Therefore, we reject the null hypothesis if the trace or eigenvalue is higher than the 5% critical value. Thus, from Tables 3 and 4, the null hypothesis of no co-integration is rejected at 5% level. The Granger representation theorem, states that if the variables are co-integrated, the relationship between them can be expressed as an ECM.
In this section, we present the estimated results of the price and non-price drivers of bananas and pineapple. The drivers of the supply response of bananas are presented in Table 5 while those of pineapple are presented in Table 6. These results are discussed in turn starting with banana. This is followed by a discussion of the error correction terms in the associated models.
Specifically, the coefficient of lagged rainfall is –0.206 and statistically significant at the 10%. This implies that a unit increase in rainfall will reduce the banana supply by 20.66%. Other studies such as Nhundu et al. (2022) also show that lagged rainfall affects supply response. While rainfall is an important input for banana production, excess rainfall may rather have deleterious effects.2 Excess rainfall leads to choking of the root network, which may result in poor absorption of nutrients. This is in line with the findings of Meza-Pale and Yunez-Naude (2015) and Salau et al. (2016) who argue that extreme rainfall conditions either in the form of drought or floods can have disturbing impacts on rural homes engaged in agriculture. Li et al. (2019) also showed that excessive rainfall could reduce maize yield up to −34%.
Temperature negatively affects supply response as indicated by its negative and statistically significant coefficient of −0.071. Hence, every Fahrenheit increase in temperature in the previous period reduces banana supply by 7%. This result can be explained by the fact that extremes of temperature greatly reduce the yield of most crops. This is in line with findings from other studies showing that temperature increases may lead to crop losses. High temperatures for example provide favorable conditions for the proliferation of pests, especially insect pests which may serve as vectors of diseases, affecting production (Hendricks and Peterson, 2014; Wang et al., 2017; Arora et al., 2020). Insect pests may equally reduce the aesthetic value of bananas.
The coefficient of lagged land is –0.346. This indicates that a hectare increase in land in the previous period reduces banana production by 34%. A plausible explanation is that banana production, especially for exports, like most horticultural crops, is capital intensive. Increasing land area may warrant an increase in capital to cultivate this land. If such capital is not available, the resources initially used in other areas will have to be apportioned to accommodate the new land area. This may reduce the output since resources may not be applied at optimal levels. This result suggests that increases in the land should be accompanied by an increase in other inputs to fully utilize the new land. For a country like Cameroon with swathes of arable land, such area can be brought into production, accompanied by sufficient quantities of other inputs like fertilizers and pesticides in order to boost production.
Turning to pineapple, lagged land negatively affects supply response. Its coefficient is negative and statistically significant suggesting that a hectare increase in land in the previous period reduces pineapple supply by 74.4%. Pineapple production is generally capital-intensive. As land increases, more capital is needed for optimal production. The absence of this may instead reduce supply.
Like land, lagged temperature equally has a negative effect on the supply of pineapple. With a negative coefficient of −2.61, one-Fahrenheit increase in temperature in the previous period reduces the supply of pineapple by 26.1%. Temperature is an important climatic variable that greatly affects agricultural production. Excess temperatures are argued to favor insect pests and diseases that affect production. Also, excess temperatures affect some important biological processes of plant growth.
Rainfall on its part positively affects supply response. A millimeter increase in rainfall in the previous period increases pineapple supply by 16.4%. This result is in line with Shoko et al. (2016) who showed that rainfall is an important determinant of supply response. Rainfall is one of sub-Sahara Africa's most important resources for crop production. This is especially important for smallholder farmers who rely on rainfall to embark on agricultural production. Since pineapple production is in the hands of smallholders with an average farm size of about 2 hectares, an increase in rainfall has important implications for pineapple production.
The error correction term [ECTt − 1(1)]; the adjustment coefficient is negative, implying that at time t-1; actual (realized) banana and pineapple production are lower than their long-run value. Banana and pineapple production will therefore adjust forward to restore equilibrium in the next period. The values of the error correction terms are −0.220 and −1.199 for banana and pineapple, respectively, meaning that 22% of errors that occurred in the short run were corrected in the long run to restore equilibrium per annum. The model adjusted itself fast (4.5 years) for all disequilibrium to realign fully to the long-run equilibrium. The value of the error correction term for pineapple is (−1.19), meaning that 11.9% of errors that occurred in the short run were corrected in the long run to restore equilibrium per annum. Therefore, the model adjusts itself (1.19 years) for all disequilibrium to realign fully to the long-run equilibrium.
Thus, far, our results have shown that non-price factors are the main drivers of the supply response of both crops. Land, rainfall, and temperature have significant effects on the supply response of bananas and pineapples in Cameroon. They negatively affect the supply response of bananas while their effect on pineapple supply response is mixed. These results suggest that non-price factors play an important role in the supply response of crops in Cameroon. Our results contradict findings from Onono (2018) who shows that non-price factors do not affect the supply of sorghum; rather, public investments are far more significant. A plausible explanation of our results is that non-price factors such as land, rainfall and temperature may be far more important for horticultural crops. For example, rainfall and temperatures may affect key biological processes that are essential for the production of these crops. This may not be the case with sorghum, which may be more resilient to drought conditions. Land is also an important factor for the production of pineapples and banana. For example in Cameroon, land expansion is one of the main sources of production increase. Hence, producers rely a lot on available land to boost their production. Reduction in such land may thus affect their supply response. Also, there is limited infrastructural support such as available greenhouses or storage facilities that could improve the production and storage of these crops. This further increases the reliance on non-price factors such as land and temperatures. Moreover, these horticultural crops have a relatively long gestation period, which means farmers cannot quickly adjust to changes in temperature, rainfall and even land. Our findings are similar to Wani et al. (2015) who found that non-price factors are important drivers of the supply response of maize in South Africa. For example, most systems are rainfed, highlighting the importance of rainfall in production decisions. In a similar vein, land is argued to be one of the most important assets for smallholders in developing countries. Hence, changes in any of these have far-reaching implications on crop production.
Price factors on their part do not have any significant effects on the supply response. Our results contrast those by Tabe-Ojong et al. (2020) who showed that price affects the supply response of tomatoes in Cameroon, Sumathi et al. (2019) who show that price and lagged production are the main drivers of the supply response of maize, and Wani et al. (2015) who equally revealed that price factors are the main drivers of the supply response of pear in India. A plausible explanation is that bananas and pineapples are perennials. In line with microeconomic theory, producers can quickly adjust to price changes of short-term crops as opposed to perennials. Once planted, perennials continuously produce which makes it difficult for producers to adjust. More so, these crops have a longer gestation period, making it even more difficult for producers to respond to price changes. However, this finding may be peculiar to Cameroon given the nature of agricultural production in the country. Production of these crops are linked to agricultural production patterns in the country. As such, whether prices change or not, it may not affect the production of such crops. For example, huge spates of land have been particularly designed for the production of such crops such that it is difficult for other crops to be cultivated in the same area. Hence, changes in prices cannot quickly alter farmers' decisions. This is also closely related to the fact that such crops are perennials, wherein once planted, it takes a long time to change decisions. These reasons may explain why our results contradict those by Tabe-Ojong et al. (2020) who found positive effects of price factors as drivers of supply response in Cameroon. They focus on tomato, which is an annual crop with a relatively short gestation period. Farmers can quickly adjust to price changes.
Amidst climate change where non-price factors have become increasingly strained, our results suggest that they should receive more attention. Given that the banana and pineapple now constitute major important crops in Cameroon as indicated by increased investment in the production, the government should focus on non-price factors.
It is possible that our results are influenced by autocorrelation and heteroscedasticity, which may affect inference. We therefore test for their presence. To test for autocorrelation in this short-run dynamic model we employed the Breusch-Godfrey Serial Correlation Lagrange Multiplier Test since the lagged dependent variable appears in the model as an explanatory variable. The results are presented in the Tables A1 and A2 for pineapple and banana, respectively in the online supplementary material. Our test shows that there is no autocorrelation indicating that the values in the series are independent of each other at different time lags. This means there is no linear relationship between a value and its past values. The absence of autocorrelation is significant in these models as it suggests that the model residuals are random, supporting the validity of the model's assumptions and improving the reliability of its forecasts.
We employed the ARCH test for heteroscedasticity in the short-run model for banana and pineapple against the null hypothesis that there is no ARCH effect. The results shown in the online supplementary material in Tables A3 and A4 for pineapple and banana, respectively, equally show that there is no heteroscedasticity. This implies the variability of independent variables are consistent across all levels of the dependent variable. In simpler terms, it indicates that the spread or scatter of the residuals (the differences between observed and predicted values) remains constant throughout the series. It assures that the model provides unbiased and efficient estimates.
Lastly, we employ Ramsey regression equation specification error test (RESET) test for the appropriate model specification. The test results are in the online supplementary material in Tables A5 and A6 for pineapple and banana, respectively. It confirms that the functional form is in order. That is, the model is correctly specified.
We employ the recursive residual test to evaluate structural breaks in our models. The graphs of the tests are presented in Figures 4 and 5 for banana and pineapple, respectively. An application of the recursive residual test demonstrates that the sample curve for banana and pineapple residual lies within the confidence band in the plot. The parameters lie approximately plus or minus two (±2) in the confidence interval for bananas and plus or minus six (±6) for pineapple.
To test the stability of coefficients, we employ the cusum test. This is a parameter stability test and it demonstrates that the parameters are stable over the sampled period for all the equations. The cusum graph shows that the test statistic is < 5% critical value. Moreover, the cusum curve lies within the confidence band in the plot suggesting that the coefficients are stable. The cusum graphs for banana and pineapple are presented in Figures 6 and 7, respectively.
Figure 7. Cusum graph for the stability of pineapple estimates. Source: Authors' construction (2023).
Horticulture provides an important avenue for fighting poverty and nutrition-related problems, especially in developing countries. Cultivation of high-value fruits and vegetables can boost the income of farmers as well as generate foreign earnings. To benefit from these, farmers need to produce in response to changes in factors; price and non-price factors that affect the production of these crops. Which of these factors drives the supply of these crops? This important question will inform policy on relevant interventions aimed at boosting supply. This paper uses time series data from 1960 to 2019 to study the supply response of two important horticultural crops: bananas and pineapples. In line with time series analysis, we performed several diagnostics tests like the unit root and co-integration tests to inform our final analysis. Most of the series became stationary upon first differencing. That is, integrated of order 1. The co-integration analysis shows that there are several co-integration vectors, hence a long-run relationship between the series. Based on these, we specified and estimated a vector error correction model to identify the determinants of the supply response of these crops. Our results show that only the non-price factors; land, temperature, and rainfall affect the supply of both crops. While they negatively affect the supply of bananas, their effect on the supply response of pineapple is mixed. On the other hand, price factors have no influence on the supply response of these crops. A plausible explanation is that bananas and pineapples are perennial crops. Unlike arable crops, once planted, they continuously produce, making it difficult for farmers to respond to price factors. Also, their production hinges on climatic factors such as rainfall and temperature.
Our results have some policy implications. First, different policies should be pursued to boost the supply of crops. Our results suggest that the type of policy prescription depends on the nature of the crop. For horticultural crops, policy makers should focus on land, temperature, and rainfall. Farmers can be trained on efficient land management strategies to reduce the impacts of floods. To curb the effect of temperature and rainfall, timely climatic information about these factors can be provided to farmers to guide them in planning their farming activities. Training farmers on the use of climate smart agricultural technologies may also play an important role in curbing the effects of climatic factors. Further, technological innovations such as storage facilities and farm machinery may help farmers in quickly adjusting to the production of these crops. Also, road infrastructure may also be important for addressing issues of supply response, especially for farmers in the rural areas. In addition, input subsidies can improve farmers' access to improved inputs allowing for agricultural intensification. This will reduce farmers' reliance on land expansion. However, farmers should also be trained on the optimal use of such inputs to mitigate potential environmental issues associated with their use. It is worth noting that banana and pineapples have a longer gestation period and the impact of improved inputs may not be apparent in the short-run. It is worth re-iterating that our results are based only on Cameroon and could be cautiously extended to other parts of the world.
The study has several limitations. Firstly, the only non-price factors we have considered are climatic factors. In as much as they may be important in determining the supply response, other non-price factors such as infrastructure, market access, technology access, agricultural policies and subsidies may equally play important role in determining supply response. For example, access to technology, which may constantly provide farmers with climatic information, may help them to better plan their production activities. Input subsidies may also increase intensification, reducing farmers' reliance on land expansion and reducing the effects of land on supply response. Such factors may even off-set the impacts of the climatic factors. However, our study fails to capture such factors. Future studies can therefore explore the role of such factors in supply response of horticultural crops in general and banana and pineapple in particular. Secondly, our study focuses only on two horticultural crops. We cannot ascertain if similar results will be obtained with other horticultural crops. More evidence on the drivers of supply response of these crops is needed. Thirdly, our focus is only on Cameroon, hence results may be context specific. Future studies with cross-country comparisons may even be more relevant in highlighting the drivers of supply response. Future studies which address such limitations are needed.
These limitations notwithstanding, our results suggest that policy attention should be directed at non-market factors. This is even more important amidst the climate crisis, which manifests through erratic rainfall and extreme temperatures. More so, most farmers in developing countries like Cameroon bear the brunt of this crisis. Through improved climate adaptation techniques and investments in land, the supply response of such crops can be improved. A country like Cameroon holds huge potential for the cultivation of these crops. A conscious policy effort should be made to overcome such challenges in order to boost supply of bananas and pineapple.
Publicly available datasets were analyzed in this study. This data can be found at: World Development Indicators, FAOSTAT, and PINK SHEET.
SA: Conceptualization, Formal analysis, Investigation, Methodology, Software, Writing –original draft, Writing – review & editing, Supervision. EM: Conceptualization, Investigation, Methodology, Supervision, Writing –original draft, Writing –review & editing. DR: Conceptualization, Writing –original draft, Writing –review & editing. RT: Conceptualization, Writing –original draft, Writing –review & editing. RN: Conceptualization, Writing –review & editing. FN: Conceptualization, Investigation, Methodology, Writing –original draft, Writing –review & editing.
The author(s) declare that no financial support was received for the research, authorship, and/or publication of this article.
The authors declare that the research was conducted in the absence of any commercial or financial relationships that could be construed as a potential conflict of interest.
The reviewer JA declared a shared affiliation with the authors to the handling editor at the time of review.
All claims expressed in this article are solely those of the authors and do not necessarily represent those of their affiliated organizations, or those of the publisher, the editors and the reviewers. Any product that may be evaluated in this article, or claim that may be made by its manufacturer, is not guaranteed or endorsed by the publisher.
The Supplementary Material for this article can be found online at: https://www.frontiersin.org/articles/10.3389/frevc.2024.1305346/full#supplementary-material
1. ^FAOSTAT: Statistical database of the Food and Agriculture Organization.
2. ^It is important to note that there is an optimal water requirement for optimal production.
Abdoussalami, A., Hu, Z., Islam, A. R. M. T., and Wu, Z. (2023). Climate change and its impacts on banana production: a systematic analysis. Environ. Dev. Sustainabil. 1–30.
Abodi, M. A., Obare, G. A., and Kariuki, I. M. (2021). Supply and demand responsiveness to maize price changes in Kenya: an application of error correction autoregressive distributed lag approach. Cogent Food Agricult. 7:1957318. doi: 10.1080/23311932.2021.1957318
AfDB (2021). “Chapter 2: feed African,” in A Resilient Continent Recovering From the Pandemic. Annual Development Effectiveness Review 2021 (Abidjan: African Development Bank),15–19.
Agnolucci, P., and De Lipsis, V. (2020). Long-run trend in agricultural yield and climatic factors in Europe. Clim. Change 159, 385–405. doi: 10.1007/s10584-019-02622-3
Akiyama, T., and Trivedi, P. K. (1987). Vintage production approach to perennial crop supply: an application to tea in major producing countries. J. Econometr. 36, 133–161. doi: 10.1016/0304-4076(87)90047-9
Anaciet, C. T. A. (2019). Modern trends in agricultural development in cameroon and ways to ensure its sustainability. Ekonomìka Ta Upravlìnnâ APK 1, 21–29. doi: 10.33245/2310-9262-2019-148-1-21-29
Anderson, K. (2023). Agriculture's globalization: endowments, technologies, tastes and policies. J. Econ. Surv. 37, 1314–1352. doi: 10.1111/joes.12529
Arora, G., Feng, H., Anderson, C. J., and Hennessy, D. A. (2020). Evidence of climate change impacts on crop comparative advantage and land use. Agricult. Econ. 51, 221–236. doi: 10.1111/agec.12551
Askari, H., and Cummings, J. T. (1977). Estimating agricultural supply response with the Nerlove model: a survey. Int. Econ. Rev. 18, 257–292. doi: 10.2307/2525749
Ayinde, O. E., and Bessler, D. A. (2014). Analysis of Supply Response and Price Risk on Rice Production in Nigeria (No. 329-2016-12951).
Bjornlund, V., Bjornlund, H., and Van Rooyen, A. F. (2020). Why agricultural production in sub-Saharan Africa remains low compared to the rest of the world-a historical perspective. Int. J. Water Resour. Dev. 36, S20–S53. doi: 10.1080/07900627.2020.1739512
Blake, D., Dawson, C., Loeillet, D., and Staver, C. (2018). Can global climate change affect prices in the world Banana market? German J. Agricult. Econ. 67, 48–64.
Boyd, C. M., and Bellemare, M. F. (2020). The microeconomics of agricultural price risk. Ann. Rev. Resour. Econ. 12, 149–169. doi: 10.1146/annurev-resource-100518-093807
Cameroon Radio Television (2018). One Billion CFAF to Boost Palm Oil, Banana, and Pineapple Production. Available online at: https://www.crtv.cm/2018/07/agriculture-one-billion-cfa-f-to-boost-palm-oil-banana-and-pineapple-production/ (accessed October 5, 2022).
Darnhofer, I., Gibbon, D., and Dedieu, B. (2010). Farming Systems Research: an Approach to Inquiry. Springer Science & Business Media. Available online at: https://www.springer.com/gp/book/9789048139279 (accessd January 12, 2023).
Delgado, C. L. (1998). Africa's changing agricultural development strategies: past and present paradigms as a guide to the future. Brown J. World Aff. 5:175.
Etame Kossi, G. M., Beyegue Djonko, H., Boukong, A., and Silatsa Tedou, F. B. (2023). Extensive pineapple production constraints and land suitability in the centre region of cameroon. Agricult. Sci. 14, 240–255. doi: 10.4236/as.2023.142016
Fafchamps, M. (2000). Farmers and Price Fluctuations in Poor Countries. Oxford: University of Oxford, Department of Economics.
FAOSTAT (2022). Statistical Database of the Food and Agriculture Organisation. Available online at: https://www.fao.org/statistics/en/ (accessed October 10, 2022).
Foley, J. A., Ramankutty, N., Brauman, K. A., Cassidy, E. S., Gerber, J. S., Johnston, M., et al. (2011). Solutions for a cultivated planet. Nature 478, 337–342. doi: 10.1038/nature10452
Food and Agricultural Organization (2018). “The future of food safety,” in Food Security: The World Conference at Addis Ababa. Addis Ababa.
Food and Agriculture Organization (2019). The Future of Food and Agriculture: Alternative Pathways to 2050. Food and Agriculture Organizationof the United Nations. Available online at: http://www.fao.org/3/ca6640en/CA6640EN.pdf (accessd January 12, 2023).
French, B. C., and Matthews, J. L. (1971). A supply response model for perennial crops. Am. J. Agricult. Econ. 53, 478–490. doi: 10.2307/1238225
Gatzweiler, F. W., von Braun, J., and Dominguez-Salas, P. (2019). The role of policy and governance in the nutrition transition: the case of Chile. Glob. Food Secur. 21, 38–45.
Gelaye, Y., and Getahun, S. (2024). A review of the carbon sequestration potential of fruit trees and their implications for climate change mitigation: the case of Ethiopia. Cogent Food Agricult. 10:2294544. doi: 10.1080/23311932.2023.2294544
Ghufran, M., Aldieri, L., Pyka, A., Ali, S., Bimonte, G., Senatore, L., et al. (2024). Food security assessment in the light of sustainable development goals: a post-Paris agreement era. Environ. Dev. Sustainabil. 23, 1–29. doi: 10.1007/s10668-023-04089-w
Glenn, D. M., Kim, S. H., Ramirez-Villegas, J., and Läderach, P. (2013). Response of perennial horticultural crops to climate change. Horticult. Rev. 41, 47–130. doi: 10.1002/9781118707418.ch02
Godfray, H. C. J., Beddington, J. R., Crute, I. R., Haddad, L., Lawrence, D., Muir, J. F., et al. (2010). Food security: the challenge of feeding 9 billion people. Science 327, 812–818. doi: 10.1126/science.1185383
Gómez-Limón, J. A., Picazo-Tadeo, A. J., and Reig-Martínez, E. (2018). Determinants of technical efficiency in smallholder agriculture: an empirical analysis of family farming in Andalusia. Land Use Policy 79, 682–691.
Gora, J. S., Verma, A. K., Singh, J., and Choudhary, D. R. (2019). Climate change and production of horticultural crops. Agricult. Impacts Clim. Change 1, 45–61. doi: 10.1201/9780429326349-3
Gouel, C. (2012). Agricultural price instability: a survey of competing explanations and remedies. J. Econ. Surv. 26, 129–156. doi: 10.1111/j.1467-6419.2010.00634.x
Granger, C. W., and Newbold, P. (1974). Spurious regressions in econometrics. J. Econometr. 2, 111–120. doi: 10.1016/0304-4076(74)90034-7
Granger, IV. C. W., Hyung, N., and Jeon, Y. (2001). Spurious regressions with stationary series. Appl. Econ. 33, 899–904. doi: 10.1080/00036840121734
Haj-Amor, Z., Araya, T., and Bouri, S. (2024). Agroforestry as a tool for climate change mitigation and agriculture sustainability: experiences from Africa. Agrofor. Carbon Ecosyst. Manag. 4, 245–256. doi: 10.1016/B978-0-323-95393-1.00015-4
Hendricks, N. P., and Peterson, J. M. (2014). Economic Damages to Agriculture From Temperature Increases: Decomposing the Effects of Heat Exposure and Water Stress. East Lansing, MI: Michigan State University.
Johansen, S. (1988). Statistical analysis of cointegration vectors. J. Econ. Dyn. Contr. 12, 231–254. doi: 10.1016/0165-1889(88)90041-3
Joshi, R. U., Singh, A. K., Singh, V. P., Rai, R., and Joshi, P. (2023). A review on adaptation of banana (Musa spp.) to cold in subtropics. Plant Breed. 2023:13088. doi: 10.1111/pbr.13088
Just, R. E., and Pope, R. D. (2001). The agricultural producer: theory and statistical measurement. Handb. Agricult. Econ. 1, 629–741. doi: 10.1016/S1574-0072(01)10015-0
Keatinge, J. D. H., Virchow, D., and Schreinemachers, P. (2016). “Horticulture for sustainable development: evidence for impact of international vegetable research and development,” in International Symposia on Tropical and Temperate Horticulture-ISTTH2016 1205, 179–190.
Kirsten, J. F., Dalheimer, B., and Brümmer, B. (2024). “Overview of the macroeconomic drivers of the region,” in Sustainability of Southern African Ecosystems under Global Change: Science for Management and Policy Interventions (Cham: Springer International Publishing), 113–130.
Kone, S., Balde, A., Zahonogo, P., and Sanfo, S. (2024). A systematic review of recent estimations of climate change impact on agriculture and adaptation strategies perspectives in Africa. Mitigat. Adapt. Strategies Glob. Change 29:18. doi: 10.1007/s11027-024-10115-7
Koohafkan, P., and Altieri, M. A. (2011). Globally Important Agricultural Heritage Systems: a Legacy for the Future (Rome: Food and Agriculture Organization of the United Nations), 1–47.
Lanfranchi, M., Giannetto, C., Rotondo, F., Ivanova, M., and Dimitrova, V. (2019). Economic and social impacts of price volatility in the markets of agricultural products. Bulgar. J. Agri. Sci. 25.
Lastochkina, O., Aliniaeifard, S., SeifiKalhor, M., Bosacchi, M., Maslennikova, D., and Lubyanova, A. (2022). Novel approaches for sustainable horticultural crop production: advances and prospects. Horticulturae 8:910. doi: 10.3390/horticulturae8100910
Li, Y., Guan, K., Schnitkey, G. D., DeLucia, E., and Peng, B. (2019). Excessive rainfall leads to maize yield loss of a comparable magnitude to extreme drought in the United States. Glob. Change Biol. 25, 2325–2337. doi: 10.1111/gcb.14628
Lobo, M. G., and Paull, R. E. (2017). Handbook of Pineapple Technology: Production, Postharvest Science, Processing and Nutrition. Hoboken, NJ: John Wiley & Sons.
Lu, W., and Horlu, G. S. A. K. (2019). Transition of small farms in Ghana: perspectives of farm heritage, employment and networks. Land Use Policy 81, 434–452. doi: 10.1016/j.landusepol.2018.10.048
Maertens, M., Minten, B., and Swinnen, J. (2012). Modern food supply chains and development: evidence from horticulture export sectors in Sub-Saharan Africa. Dev. Pol. Rev. 30, 473–497. doi: 10.1111/j.1467-7679.2012.00585.x
Maertens, M., and Swinnen, J. F. (2012). Gender and modern supply chains in developing countries. J. Dev. Stud. 48, 1412–1430. doi: 10.1080/00220388.2012.663902
Meza-Pale, P., and Yunez-Naude, A. (2015). The Effect of Rainfall Variation on Agricultural Households: Evidence From Mexico (No. 1008-2016-79827).
Minten, B., Randrianarison, L., and Swinnen, J. F. (2009). Global retail chains and poor farmers: evidence from Madagascar. World Dev. 37, 1728–1741. doi: 10.1016/j.worlddev.2008.08.024
Mkuna, E. (2022). “Determinants of horticultural export and welfare impact of smallholder farmers: evidence from common beans (Phaseolus vulgaris L) farming in Arusha Tanzania,” in Trade and Investment in East Africa: Prospects, Challenges and Pathways to Sustainability (Singapore: Springer Nature Singapore), 267–292.
Nerlove, M. (1958). Adaptive expectations and cobweb phenomena. Quart. J. Econ. 72, 227–240. doi: 10.2307/1880597
Ngwu, E. C., Nwosumba, V. C., and Onah, V. C. (2024). Russia-Ukraine war, the leadership question and sustainable food security in Africa. Sustain. Dev. 2024:2881. doi: 10.1002/sd.2881
Nhamo, G., and Chapungu, L. (2024). Prospects for a sustainable and climate-resilient African economy post-COVID-19. Glob. Environ. Change 86:102836. doi: 10.1016/j.gloenvcha.2024.102836
Nhundu, K., Gandidzanwa, C., Chaminuka, P., Mamabolo, M., Mahlangu, S., and Makhura, M. N. (2022). Agricultural supply response for sunflower in South Africa (1947-2016): the partial Nerlovian framework approach. Afri. J. Sci. Technol. Innov. Dev. 14, 440–450. doi: 10.1080/20421338.2020.1844944
Nickell, S. (1985). Error correction, partial adjustment and all that: an expository note. Oxf. Bullet. Econ. Stat. 47, 119–129. doi: 10.1111/j.1468-0084.1985.mp47002002.x
Njukwe, E., Amah, D., Ndango, R., and Tenkouano, A. (2010). Fast-track release of black leaf streak resistant banana and plantain (Musa spp.) hybrids and related technologies to farmers in Cameroon: a strategy to enhance food security. Acta Horticult. 879, 577–584. doi: 10.17660/ActaHortic.2010.879.64
Oduniyi, O. S. (2022). Price fluctuation of agricultural products: can the theory of complex phenomena explain it better? J. Priv. Enterpr. 37.
Ogundari, K. (2018). Maize supply response to price and nonprice determinants in Nigeria: bounds testing approach. Int. Trans. Operat. Res. 25, 1537–1551. doi: 10.1111/itor.12284
Ollong, K. A. (2017). Sustainability issues in the Cameroon banana supply chain. Revista Brasileira de Estudos Africanos 1:67447. doi: 10.22456/2448-3923.67447
Ongoma, V., Epule, T. E., Brouziyne, Y., Tanarhte, M., and Chehbouni, A. (2024). COVID-19 response in Africa: impacts and lessons for environmental management and climate change adaptation. Environ. Dev. Sustainabil. 26, 5537–5559. doi: 10.1007/s10668-023-02956-0
Onono, P. A. (2018). Response of sorghum production in Kenya to prices and public investments. Sustain. Agricult. Res. 7, 19–29. doi: 10.5539/sar.v7n2p19
Onyeaka, H., Adeboye, A. S., Bamidele, O. P., Onyeoziri, I., Adebo, O. A., Adeyemi, M. M., et al. (2024). Beyond hunger: unveiling the rights to food in sub-Saharan Africa. Food Energy Secur. 13:e530. doi: 10.1002/fes3.530
Orden, D. (2002). Exchange rate effects on agricultural trade. J. Agricult. Appl. Econ. 34, 303–312. doi: 10.1017/S1074070800009056
Pretty, J., Benton, T. G., Bharucha, Z. P., Dicks, L. V., Flora, C. B., Godfray, H. C. J., et al. (2018). Global assessment of agricultural system redesign for sustainable intensification. Nat. Sustainabil. 1, 441–446. doi: 10.1038/s41893-018-0114-0
Punshi, C. P. (2020). Rethinking Error Correction Model in Macroeconometrics Analysis: a Relevant Review. MPRA Paper No. 98202.
Quentin, K., Mabah Tene, G. L., Ambagna, J. J., Piot-lepetit, I., and Fondo, S. (2015). The impact of food price volatility on consumer welfare in Cameroon. SSRN Electr. J. 2015:3176764. doi: 10.2139/ssrn.3176764
Resnick, D. (2024). Political economy of agriculture and food policy in Africa. Ann. Rev. Resour. Econ. 16:80332. doi: 10.1146/annurev-resource-101722-080332
Robinson, G. M. (2018). Globalization of agriculture. Ann. Rev. Resour. Econ. 10, 133–160. doi: 10.1146/annurev-resource-100517-023303
Salau, O. R., Momoh, M., Olaleye, O. A., and Owoeye, R. S. (2016). Effects of changes in temperature, rainfall and relative humidity on banana production in Ondo State, Nigeria. World Sci. News 44, 143–154.
Sarkar, T., Sengupta, S., Kanthal, S., and Kundu, S. (2024). “Climate change mitigation through agro-forestry improves natural resource and livelihood security,” in Agroforestry to Combat Global Challenges: Current Prospects and Future Challenges (Singapore: Springer Nature Singapore), 219–246.
Sharma, G., Sharma, A., Sinha, N. K., Sharma, O. P., Singh, A., Pandey, A. K., et al. (2022). Assessment of long-term climate variability and its impact on the decadal growth of horticultural crops in central India. Ecol. Process. 11, 1–28. doi: 10.1186/s13717-022-00405-w
Shoko, R. R., Belete, A., and Chaminuka, P. (2016). Estimating the supply response of maize in South Africa: a Nerlovian partial adjustment model approach. Agrekon 55, 237–253. doi: 10.1080/03031853.2016.1203802
Siegle, J., Astill, G., Plakias, Z., and Tregeagle, D. (2024). Estimating perennial crop supply response: a methodology literature review. Agricult. Econ. 55, 159–180 doi: 10.1111/agec.12812
Singh, R., Singh, R., Gehlot, A., Akram, S. V., Priyadarshi, N., and Twala, B. (2022). Horticulture 4.0: adoption of industry 4.0 technologies in horticulture for meeting sustainable farming. Appl. Sci. 12:12557. doi: 10.3390/app122412557
Smith, M. J. (2018). Getting value from artificial intelligence in agriculture. Anim. Prod. Sci. 60, 46–54. doi: 10.1071/AN18522
Sumathi, P., Parthipan, B., Amarnath, J. S., and Sivasankari, B. (2019). Supply response of maize in Dindigul District of Tamil Nadu. Ind. J. Agricult. Res. 53, 120–122. doi: 10.18805/IJARe.A-4958
Tabe-Ojong, M. P. J., Fabinin, A. N., Minkoua Nzié, J. R., Molua, E. L., and Fonkeng, E. E. (2023). Organic soil amendments and food security: evidence from Cameroon. Land Degrad. Dev. 34, 1159–1170. doi: 10.1002/ldr.4523
Tabe-Ojong, M. P. Jr., Molua, E. L., Nzie, J. R. M., and Fuh, G. L. (2020). Production and supply of tomato in Cameroon: examination of the comparative effect of price and non-price factors. Sci. Afri. 10:e00574. doi: 10.1016/j.sciaf.2020.e00574
Tadesse, G., Algieri, B., and Kalkuhl, M. (2020). The impact of food price shocks on child labor: evidence from rural Ethiopia. World Dev. 127:104748.
Tagang Tene, N. S. (2022). Cameroon's adaptation to climate change and sorghum productivity. Cogent Soc. Sci. 8:2140510. doi: 10.1080/23311886.2022.2140510
Thrift, N. J., Tickell, A., Woolgar, S., and Rupp, W. H. (2014). Globalization in Practice. Oxford: Oxford University Press.
Tripathi, A., and Anubhuti, R. P. (2008). Estimation of agricultural supply response by cointegraton approach. Ind. Econ. J. 57, 106–131. doi: 10.1177/0019466220090106
Van den Broeck, G., and Maertens, M. (2016). Horticultural exports and food security in developing countries. Glob. Food Secur. 10, 11–20. doi: 10.1016/j.gfs.2016.07.007
Van Kooten, G. C., Eagle, A. J., Manley, J. E., and Smolak, T. (2019). Measuring the size of the bioeconomy in Canada. Biofuels Bioprod. Biorefin. 13, 332–340.
Von Braun, J., and Diaz-Bonilla, E. (2008). Globalization of agriculture and food: causes, consequences and policy implications. Global. Food Agricult. Poor 2008, 1–45.
Wamuci, S. (2022). Cameroon Pineapples. Available online at: https://www.selinawamucii.com/produce/fruits-and-vegetables/cameroon-bananas/ (accessed October 5, 2022).
Wang, E., Martre, P., Zhao, Z., Ewert, F., Maiorano, A., Rötter, R. P., et al. (2017). The uncertainty of crop yield projections is reduced by improved temperature response functions. Nat. Plants 3, 1–13. doi: 10.1038/nplants.2017.125
Wani, M. A., Din, A., Nazki, I. T., Rehman, T. U., Al-Khayri, J. M., Jain, S. M., et al. (2023). Navigating the future: exploring technological advancements and emerging trends in the sustainable ornamental industry. Front. Environ. Sci. 11:1188643. doi: 10.3389/fenvs.2023.1188643
Wani, M. H., Sehar, H., Paul, R. K., Kuruvila, A., and Hussain, I. (2015). Supply response of horticultural crops: the case of apple and pear in Jammu & Kashmir. Agricult. Econ. Res. Rev. 28, 83–89. doi: 10.5958/0974-0279.2015.00006.3
Keywords: horticulture, price, supply response, Nerlovian expectation model, co-integration, error-correction model
Citation: Awa ST, Molua E, Raoul Fani DC, Tabetando R, Nkendah R and Ndip FE (2024) Exploring non-price factors shaping supply response: insights from Cameroon's banana and pineapple horticultural industries. Front. Environ. Econ. 3:1305346. doi: 10.3389/frevc.2024.1305346
Received: 01 October 2023; Accepted: 10 June 2024;
Published: 02 July 2024.
Edited by:
Gideon Danso-Abbeam, University for Development Studies, GhanaReviewed by:
Niraj Prakash Joshi, Hiroshima University, JapanCopyright © 2024 Awa, Molua, Raoul Fani, Tabetando, Nkendah and Ndip. This is an open-access article distributed under the terms of the Creative Commons Attribution License (CC BY). The use, distribution or reproduction in other forums is permitted, provided the original author(s) and the copyright owner(s) are credited and that the original publication in this journal is cited, in accordance with accepted academic practice. No use, distribution or reproduction is permitted which does not comply with these terms.
*Correspondence: Francis E. Ndip, ZWJhaWZyYW5jaXMxQGdtYWlsLmNvbQ==
†These authors have contributed equally to this work
Disclaimer: All claims expressed in this article are solely those of the authors and do not necessarily represent those of their affiliated organizations, or those of the publisher, the editors and the reviewers. Any product that may be evaluated in this article or claim that may be made by its manufacturer is not guaranteed or endorsed by the publisher.
Research integrity at Frontiers
Learn more about the work of our research integrity team to safeguard the quality of each article we publish.