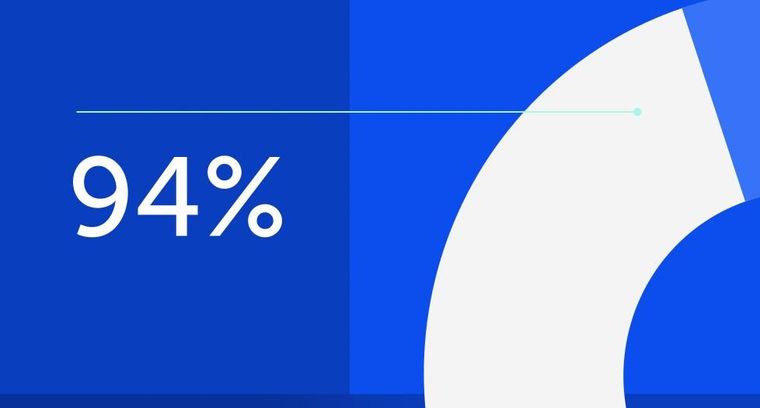
94% of researchers rate our articles as excellent or good
Learn more about the work of our research integrity team to safeguard the quality of each article we publish.
Find out more
ORIGINAL RESEARCH article
Front. Environ. Econ., 24 August 2023
Sec. Energy Economics
Volume 2 - 2023 | https://doi.org/10.3389/frevc.2023.1200703
This article is part of the Research TopicFostering the energy transition amidst geopolitical and climate risks in a post-covid worldView all 5 articles
This paper introduces a framework aimed at analyzing the water-energy-food nexus (WEF) within the context of sustainable farming practices utilizing renewable energy sources, specifically Solar PV, to optimize water management efficiency. The focus lies on investigating the interplay between the water-energy-food nexus and both technical and institutional factors. The study particularly delves into the utilization of distributed energy systems and microgrids for electricity distribution. To achieve the objectives outlined, the framework is applied to a case study involving an off-grid farm in Morocco, aligned with the country's “Green Morocco Plan” of 2008. The study employs the AnyMOD open-source modeling framework in combination with the publicly available decision support tool CropWat (Version 8.0). Through this coupling, a linear optimization model is created to assess various irrigation practices, thus evaluating the energy and water supply variations across different crop growth stages. By employing scenario analysis, the study reveals that the integration of a smart microgrid alongside storage technologies proves beneficial in terms of reducing overall system costs. This integration presents cost-effective solutions and enables the establishment of a sustainable energy supply driven by renewable energy resources. Furthermore, the investigation highlights that constraining irrigation to specific hours of the day results in increased storage requirements and higher associated costs. In conclusion, the study underscores that enhancing the water-energy-food nexus through the integration of a renewable-based microgrid is a complex task. However, it significantly contributes to the development of sustainable farming solutions. This research sheds light on the challenges and opportunities associated with aligning renewable energy, water management, and agricultural practices, ultimately facilitating the pursuit of environmentally conscious and efficient farming methodologies.
In the context of the Sustainable Development Goals (SDGs), defined by the United Nations (General, 2015), agriculture is linked directly to, i.e., zero hunger (SDG2), water (SDG 6) and energy (SDG 7). Both inputs, water, and energy, are scarce, and need to be used efficiently. This topic nowadays runs under the title of the “water-energy-food” nexus (WEF). The WEF is particularly important in arid regions, like North Africa, where some farms are completely isolated from the main grid, others are connected to the grid but still require additional energy to power their farm equipment. For both grid-connected and isolated farms, gas and diesel are the dominant alternative resources of energy. These fossil energies produce significant amounts of CO2, and affect the countries' ability to meet their climate targets, e.g., those agreed on in the Paris Agreement of the COP21 (General, 2015). Similarly, the region is threatened by water scarcity (Djaffar and Kettab, 2018). Governments and private actors often have to intervene and are trying to develop local solutions for decentralized sustainable water management systems such as water purification or desalination plants, water pumping and distribution systems, recycling units for gray water, smart agriculture projects etc., as alternative ways of coping with the situation.
While the water-energy-food nexus is a global topic, empirical evidence suggests that North Africa, i.e., the Maghreb region, is particularly affected by diminishing water resources and the need to migrate from fossil fuels to renewables. Agriculture plays an important role in these fast-growing countries. Thus, significant attention has to be paid to how the abundant renewable energy resources particularly Solar PV in the region can be sustainably developed for diverse farm production uses, with a direct impact on farm water management and opportunities for storage systems such as cooling chambers and water reservoirs. Algeria is an interesting case, that is starting to move from fossil fuels to renewables.1 Morocco is another case at hand, where despite recent technological progress, the agriculture sector is still using traditional technologies and approaches. The increasing number of small farms with complicated land title issues was one of the main limiting factors for policymakers to modernize this sector while taking into consideration the tradeoff between the economic scale and the social and rural structure of the Moroccan society (Faysse, 2015).
In this paper, we provide a framework for analyzing the microgrid approach and apply it to a concrete case study in Morocco using two models in the energy-water-food (WEF) nexus. Our hypothesis is that sustainable agriculture in the region requires both an efficient use of the scarce resource water and the conversion of the traditional, fossil-fuel based energy system to renewable resources. We combine the analysis of a real-world laboratory, a farm in Morocco, with a numerical modeling exercise to gain insights into potential development pathways for the WEF. The concept of local microgrids will be particularly useful in this context because they allow a flexible use of resources at modest cost. The next section of the paper provides elements of the techno-economic context of our paper, including distributed energy systems, the potential role of microgrids in the WEF; it also covers the setting of our case study, a farm in the Marrakesh-Safi region in Morocco. Section Methodology: models, scenarios, and data presents the modeling framework, combining an energy system model (“AnyMOD”) with a publicly available computer program of crop water requirements and irrigation (“CropWat 8.0”). We define four different scenarios that differ by assumptions on irrigation timing, and we explain the other variables. We include a scenario with an (exogenously developed) microgrid, to open the possibility of energy sector transformation. Section Results and discussion presents the results: We find a direct link between farming practices and the efficient use of water and (renewable) energy. In the longer term, the combination of a microgrid and some storage capacity, in addition so significant solar panels to generate electricity, yields the least-cost solution. We conclude in Section Conclusions and further research that the water-energy-food nexus in North Africa (and elsewhere, off course) has to be treated with care, but that sustainable development options do exist.2
This section presents some context that is needed to assess the case study of the WEF in Morocco. In particular, we review the potential role of distributed resources and microgrids, and we provide background information on the case study in Morocco.
Decentralized energy systems have been defined by small-scale units that can generate energy for local clients. Therefore, Decentralized Energy System (DES) can be stand-alone or connected and shared to the network (Vezzoli et al., 2018). Figures 1, 2 illustrate how the DES can be implemented in the local grid. Of the numerous renewable energy development alternatives available, micro-grid systems have been described as one of the most self-sufficient and stable energy systems. They also have a significant potential of meeting the operational needs of such farms, as well as generating abundant energy for larger-grid integration, or storage using batteries and other technologies for local farm and broader community use (Lefore et al., 2021). The potential of such systems on and off the farms is further enhanced if such systems are digitalized (Blasch et al., 2020). However, the proper design and implementation of these systems to derive the maximum benefits depend on the availability of high-quality data and simulated models on the expected performances of these systems.
Figure 2. Distributed energy systems. Source: (Vezzoli et al., 2018).
The microgrid concept is defined as a reliable solution to integrate distributed energy resources (DERs), load and energy storage systems. It can be seen as a box of connected units, distributing generation and the use of energy storage systems to one point that is called point of common coupling (PCC) (Olivares et al., 2014).
The deployment of microgrids is expected to reduce overall energy consumption, therefore reducing system costs. Microgrids are considered one of the features of distributed energy that can facilitate and coordinate the realization of smart grids (Vezzoli et al., 2018). Vezzoli in his paper has defined a decentralized energy system as the small-scale unit that can generate energy for local clients. Therefore, DES can be stand-alone or connected and shared to the network. Labrousse (2006) and Vezzoli et al. (2018) further elaborate on the concept and its relevance.3
According to Bloomberg NeF, the digitalization of energy systems can ensure and maintain grid stability and reliability, where the frequency can be regulated in real-time, and accurate response to the system demand will be provided. It can also provide the opportunity to monitor the grid and identify the weak point using digital technologies, optimization, and energy production forecasting tools that can help to integrate RE easily with efficient equipment. Consumers will have more control over their system and easy access to the data (energy consumption, power generation) (Cozzi et al., 2017).
The interactions between water, food, and energy are diverse, and each of such interactions counts. A significant amount of water is utilized in the production of energy, from direct energy extraction as in hydroelectric power generation to indirect contributions such as in the production of either feedstock or relevant crops for synthesizing biofuels (Raza et al., 2019). Microgrids can accommodate various renewable energies, including more traditional ones such as photovoltaics and wind, but in some cases also geothermal energy. Kaltschmitt et al. (2010) and Jacobson (2020, 2023) provide a technical-economic survey of these potentials.
Electricity is the most efficient form of energy to manage the water resources needed for farming (Jackson et al., 2010). Consequently, traditional irrigation methods that use hand-based technology such as watering cans are being replaced, particularly on large farms with electricity-powered drip irrigation systems. Therefore, the direct results of utilizing energy in attaining a dual output of boosting food production with proper water management are evident (Chen et al., 2015) provide further insights into the complexity of managing the WEF. The nexus approach has a long tradition, but reflections have been revitalized by new elements, i.e., digitization and the opportunity to apply relatively cheap renewable energies. Thus, the WEF management approach addresses multiple objectives (Bellfield, 2015). The nexus approach has been viewed in the literature as a management framework that coordinates the interactions between diverse variables across different scales and sectors. Hence its application to the study of water, energy, and food is often geared toward resource use efficiency and policy coherence (El Youssfi et al., 2020). Applying digitized microgrid technology (smart metering, sensors, block chain and IoT technologies) in crop production to ensure the effective use of water resources and boost food production using clean energy is consistent with the tenets of the Water Food Energy Nexus propositions.
El Youssfi et al. (2020) further describe the application of a nexus perspective in the study of water, energy, and food resources. He argues that these three variables are pillars of most economies of the world but also, he argues in favor of the need to maintain an equilibrium in the drive to achieve coherence across these three key sectors. This argument is underscored by Raza et al. (2019). The issues have become even more urgent with the necessity to combat climate change and to secure reliable and inexpensive supplies. Figure 3 illustrates some of the key factors of the water-energy-food nexus.
Figure 3. Illustration of the energy-water-food (EWF) nexus. Source: (Granit, 2022).
In the context of our case study in Morocco. The nexus highlights that water is crucial for irrigation and crop production, its availability and efficient management directly impact the food production in general. In addition, energy plays a vital role in agricultural activities, such as water pumping in our case, and machinery operation. Renewable energy technologies, such as solar photovoltaics, can provide clean and sustainable energy for the irrigation systems, reducing fossil fuels dependency and mitigating climate change. By integrating these key factors of the water-energy-food nexus, we strengthen the interconnection and enhance the management of water and energy resources for sustainable agriculture production.
Morocco is relatively advanced in its energy transformation process, and it has already specified renewables targets, too (IEA, 2019). In 2012, the Moroccan government launched the “Green Morocco Plan,” which stated a strategic plan for the agriculture sector and the development strategy till 2030 (Najib, 2012). This plan supports young entrepreneurs by mobilizing about one million hectares of collective lands of high-value agriculture while promoting human and social development.
The COVID-2019 pandemic has increased the need for an efficient use of resources across all sectors (Sridhar et al., 2022). In the agricultural sector, labor availability and farm system resilience have been outlined to be clear domains of concern (Stephens et al., 2020). In their publication, the authors raise the question of what technological measures could be adopted to reduce dependency on human labor and gain efficiency in farming. Stephens et al. also highlight that the timing of labor needs is often inflexible for seasonally produced foods. This requires an efficient management of labor to secure future growing seasons and avoid adverse consequences for future food security.
As Morocco aims to make the agricultural sector one of its main pillars for economic development (Faysse, 2015), opportunities for increasing efficiency in agricultural production need to be explored. Smart operating microgrids that coordinate water management and irrigation scheduling together with a flexible supply of renewable energy resources appear to be a promising solution to increase resilience and profitability.
The Rismar Farm (called “Abd El Hafid”) is situated in the El Kelâa des Sraghna province, Marrakech-Safi region, known for its great agricultural reputation, especially for the cultivation of olive trees.4 It occupies the first class of olive production at the national level. Particularly in the agricultural sector, olive trees remain the predominant trees in the region. They extend over more than 43,000 hectares, or almost 91% of the total area reserved for fruit farming with an average production of over 100,000 tons in a normal year, or twenty-four quintals per hectare [The Chamber of Commerce, Industry and Services of the Marrakesh–Safi Region (CCISRMS), 2022].
Recently, the Moroccan government has planned strong national investments in the agriculture sector, especially in olives. For instance, El Kelâa des Sraghna hosts the national olive exhibition. Thus, the Rismar farm could be considered like a living laboratory (or open platform) for sharing best practices in all aspects (e.g., microgrid, water, and energy).
The farmer has its own water resource using a pumping system, which operates on power-generated photovoltaic systems (15.6 kWp polycrystalline technology with no battery storage) deployed in 2014. This energy is used to pump water from the well (100 m depth). In addition, 3–4 gas bottles are used per day for additional electricity generation as shown in Figure 4. More precisely, the electricity generated from the PV system is not sufficient to power all the energy needs of the farmer; it is used also at night or on cloudy days to provide the farm with the needed electricity.
Regarding the water, no storage tank is used so far. The groundwater is directly pumped and redirected to the irrigation system, which drips slowly to the roots of the plants, from above the soil surface. It should be mentioned that a water tank (basin) as captured in Figure 5 together with its supporting system (the irrigation system including the management part) were already deployed, but not used so far because of the lack of electricity. The farm does not have access to the public utility grid, neither is any electricity storage installed.
For this study, the open-source modeling framework AnyMOD (Göke, 2021b) and the publicly available decision support tool CropWat (Smith, 1992) (Version 8.0) were used to create a linear optimization model for different irrigation habits. Using a brownfield approach, the combination of both programs allows to model different irrigation habits and to find their respective optimal capacities for power supply technologies within the already existing water infrastructure. The depicted exemplary farm cultivates wheat, potatoes, and small vegetables in equal shares.
The AnyMOD Framework uses a graph-based linear modeling approach to allow for large scale energy system models (Göke, 2021a,b). It was previously used to model the European energy system by Kendziorski et al. (2022) as well as in many other publications on energy systems, such as Zozmann et al. (2021), Eerma et al. (2022), and Göke et al. (2022). It uses a graph-based approach to allow varying levels of detail for different energy carriers and time steps to reduce the size of the model and makes it faster to compute. Other modeling frameworks do not provide this option and need a lot of computational force to achieve similar results.
Another advantage of the AnyMOD framework is its transparency and ease of transfer across different applications. AnyMOD is an open source package for the Julia coding language (Bezanson et al., 2017). The user only needs to provide the necessary data files in from of csv-files (comma-separated-value-files), while AnyMOD reads in this data to write the corresponding optimization problem and all its constraints. Examples of this can be found in the AnyMOD documentation (Göke, 2021b).
In this paper, electricity generation and use were modeled on an hourly basis, while the granularity of water supply and demand varies across the different scenarios. CropWat 8.0 is a publicly available computer program by the Food and Agriculture Organization of the United Nations (FAO) for the calculation of crop water requirements and irrigation scheduling. The calculations are based on climate, soil and crop data that can be obtained using the CLIMWAT database (Smith, 1993). CropWat 8.0 uses daily input of climatic data to calculate a reference evapotranspiration (ETo) as well as effective rain and deduces net irrigation requirements. Effective rain is defined as the part of the rainfall which is effectively used by the crops and accounts for rain losses due to surface run off and deep percolation (Smith, 1993). There are four different methods to calculate effective rainfall in CropWat 8.0. In this paper, the USDA soil conservation service method was applied and for monthly values, it can be calculated as follows:
where Peff is the effective power, Ptotal is the total power, and 250 mm is a threshold of rainfall. Peff is the amount of water from precipitation that is effectively used by the crop. Ptotal is the total precipitation for crop water requirements of non-rice crops, used as a reference. Evapotranspiration (ETo) is multiplied with the respective crop coefficient (Kc) this product represents the crop evapotranspiration under optimal conditions; this must be and can be subtracted by effective rain (Peff) to calculate the irrigation requirements (Irrreq).
Crop coefficients vary throughout four phases of crop cultivation, the initial stage, crop development stage, mid-season stage and the late season stage. In this study, CropWat 8.0 was used to calculate irrigation requirements for three-time steps in every month i.e., for 36 periods for a year. The data used was extracted from the CLIMWAT 2.0 (Smith, 1993) database, which uses observed agro-climatic data of over 5,000 stations worldwide and is publicly available. Climatic data from a weather station in Marrakech (station number 2479) and crop specific values from the CropWat 8.0 database were used to provide the necessary parameters.
In every scenario, a residual load was implemented to account for cooling and security systems. The yearly electricity usage for cooling activities per capita in El Paso (Waite et al., 2017) was taken for reference. As cooling activities broadly follow outdoor temperature (IEA, 2018), an hourly time series of PV potential was used as a distribution factor to estimate an hourly demand for cooling at the farm. In addition to that, a yearly electricity consumption of a security system was taken from Ion et al. (2021) and dispatched as a constant value over the year on an hourly resolution.
Four different scenarios were created to model different irrigation habits. This was made to depict and compare different behavioral strategies of the farmer that require varying degrees of infrastructure and labor. Across all scenarios, preexisting pumping systems and a water storage with the capacity of 10,000 m3 water are installed. In order to meet the irrigation demand water needs to be pumped out of a source (a small fountain for example), into the water storage and then needs to be pumped out of the storage and onto the fields which have a uniform surface area of two hectares. Figure 6 Energy water flow, and Table 1 summarizes the scenarios.
• In the first scenario (scenario IrrFix), irrigation happens in a constant flow of water on an hourly basis, with no regard to the daytime. The sum of the water flow equals the irrigation requirements of every crop. In this scenario, all crops are irrigated at all times i.e., they are all irrigated simultaneously. This depicts a case where all fields are irrigated via water pipes that use drip irrigation.
• The second scenario (scenario Irr9-5) depicts irrigation at working hours from 9 am to 5 pm. In this scenario, irrigation is labor intensive, and crops cannot be irrigated simultaneously.5 Therefore every 10-day interval was divided into 9 working days and each crop gets its water requirement fulfilled within 3 days of irrigating from 9 am to 5 pm.
• As a third scenario (scenario IrrME), irrigation is conducted for 3 h in the early morning (5 to 8 am) and in the evening (5–8 pm) and follows the same principal as the scenario Irr9-5, i.e., irrigation occurs on three distinct working days for each crop in each 10-day interval. This scenario depicts a case where farmers avoid working in heat. The same assumption about the availability of manual labor as in scenario Irr9-5 was made.
• The last scenario (scenario IrrOpt) represents the implementation of a smart micro grid, which optimizes around the availability of renewable energy resources (Solar PV energy) in alignment with the visual representation in Figure 7. The microgrid is 100% flexible when to irrigate on an hourly basis and how much, as long as the irrigation requirements of every crop is met in every ten-day interval.
The model optimizes the energy investments and the use of storage under the assumption of full knowledge about the weather conditions (the year used for the study is 2019). The underlying assumption is that the farmer has knowledge about the weather conditions and is able to adapt the storage management accordingly. This assumption holds, when access to accurate weather forecasts is given and farmers are able to derive rational and precise decisions for their storage management based on this information. In this context, a smart microgrid that is able to take weather data as an input and then to calculate the optimal charging and discharging activities of the storages would be a significant progress and help to optimize the available renewable energy.
For the development of the optimization model the authors collected different types of data, including from field trips and interviews, to assemble a representative snapshot for the farm in Morocco. For the availability of Solar PV, hourly generation potentials of the year 2019 were taken from a publicly available website (Pfenninger and Staffell, 2016; Staffell and Pfenninger, 2016) and for modules with a fixed tilt of 35° and for the diesel generator, solar PV, and battery lifetime, and cost described in Table 2. To create a time series that depicts the electricity consumption of a cooling system, data of the solar generation potential was used where the tilt and azimuth are optimized to follow the path of the sun, therefore crating a flatter and longer pattern.
The cultivated crops in this study (wheat, potatoes and small vegetables) were selected based on their share of agricultural production (FAO, 2022) and their availability in the CropWat database. To calculate the irrigation requirements, data from CropWat 8.0 and CLIMWAT was used. The selected weather station in CLIMWAT has the number 2479 and is situated in Marrakech. Further cost estimates are summarized in Source: authors.
Table 2 shows the Solar PV capacities and battery storage capacities, both in kilowatts (kW). In addition, the table includes a column showing the cost differential between the lowest costs (scenario IrrOpt), as 100%, and the cost uplift of the other scenarios. Figure 7 shows the flows of electricity and water over 500 h, both demand (upper part of each panel) and supply (lower part). Across all scenarios, small battery capacities were utilized to ensure that the residual power demand could be met in hours of zero electricity generation from Solar PV. Differences in pumping activities and water storage management will also be discussed in this section, together with more general implications of the reviewed scenarios.
Investment in Solar PV capacities is quite similar throughout the scenarios. The results as shown in Table 3. However, those in battery storage capacity differ significantly. The results show that the smart microgrid (scenario IrrOpt) yielded the overall lowest system costs, around 39% below the second-best option. This is because investments in batteries are the lowest, thanks to the efficient distribution of the solar electricity generated.
When irrigating at a constant rate regardless of the time of day (scenario IrrFix), additional investments into battery capacities have to be made in order to ensure that the power demand of the irrigation can be fulfilled during the night. In order to minimize costs, efforts should be made to ensure that water that is needed for irrigation is already available and does not have to be pumped from the source during the night. This can be achieved by filling a water storage in times of high electricity generation from the Solar PV.
In this paper, the water storage is represented as a basin at ground level. However, above ground level water storages might have the potential to reduce the electricity demand for direct irrigation, by capitalizing on the potential energy of the water. This could potentially reduce the necessary battery capacities in this scenario significantly; however, it would also lead to a higher electricity demand during the day. The payoffs of above ground level water storages should be explored in further research. The overall system costs of the scenario IrrFix were 38.8% higher compared to a scenario with a microgrid that optimizes irrigation timing.
Irrigating between the traditional working hours from nine to five (scenario Irr9-5) yields a similar storage requirement as in the IrrFix scenario (+39:6 % higher costs). By irrigating at hours that are similar to working hours of other industries (i.e., from 9 am to 5 pm), most of the electricity demand occurs during the day, which is efficient for the utilization of Solar PV. However, in the early working hours (9–10 am) the generation of the installed Solar PV system has to be supplemented by batteries, due to the relatively small generation potential of solar-based technologies in this period. The battery and the water storage can then be refilled at hours of high PV availability and in the evening hours, where no direct irrigation occurs. Delaying the irrigation by just 1 h decreases the need for additional battery capacities and reduces the overall system costs by around 2%. This would make it a slightly better option, compared to the previously discussed scenario (IrrFix), but it would still be 37.6% more expensive than a scenario with smart irrigation timing by a microgrid.6
Last but not least, model results indicate that irrigating only in the early morning and in the evening (scenario IrrME) is the least efficient and most costly irrigation habit across all four scenarios. It yields around 64.4% higher system costs then a farm that is equipped with a smart irrigation system. This is because batteries have to account for the low solar potential during irrigation hours. The generation from the PV system is used to charge the battery and fill the water storage, but cannot be utilized for irrigation, which leads to inefficient energy management and high system costs. The frequent charging and discharging of the battery would also likely shorten its lifespan and lead to a more error prone production system.
Figure 7 shows the demand and the supply, respectively, of electricity flows (upper panel) and the water flows (lower panel) for a representative period of the year, between hours 2,000 and 2,500. The smart microgrid optimizes the irrigation timing around the availability of solar energy and adapts to varying weather conditions. This leads to an irrigation schedule where the amount of irrigated water is proportional to the solar availability with a refill of water storage capacities at the peak generation potential. This irrigation method allows for smaller investments into batteries, which are almost exclusively used to satisfy the residual electricity demand in the night.
Looking at the lower panel, it can be deduced that it is not optimal to have days without any irrigation, as long as there is an infeed of electricity by the installed pv-system. Also, refilling the water basin and irrigating at the same time is avoided by the model and keeping those activities separate is preferred. However, the model does assume no setup time in between irrigation at crops and refilling the water basin.7 Another interesting observation are small battery discharging activities in the evening, on days where the water storage gets refilled. In those days it is optimal to prolong the pumping activities with the use of a battery for an additional hour in the night. However, the amount of water that is being pumped in these time steps is small; no generalization can be made, whether it is best to use this additional hour for irrigation or to use it for refilling the basin.
Renewable energy, like solar panels, offers clean and reliable power for agriculture. It reduces reliance on fossil fuels, providing sustainable and cost-effective energy for farmers. This integration enhances productivity by powering irrigation systems, machinery, and other farm activities. Solar-powered pumps and drip irrigation optimize water usage, minimize costs, and improve water management, leading to more efficient agriculture. Farmers can install renewable energy systems on their land, meeting their energy needs and potentially selling surplus energy for additional income.
The efficient use of renewable energies is an important element of a sustainable energy-water-food nexus, in the Maghreb region and elsewhere. In this paper, we have provided a framework to analyze the water-energy-food nexus, and to compare capacity choices for an individual farm. We have also explained the case study chosen, i.e., a farm in Morocco, which currently still uses significant amounts of fossil fuels.
The stylized model calculations provide a comparison between different investment options to increase the use of renewable energy. We combine a model of crop water requirements (CropWat) with an energy system model (AnyMOD) to obtain patterns of electricity and water supply and demand. The model shows that the use of a smart microgrid allows the least-cost investments in Solar PV and battery storage, because it optimizes the use of solar radiation when it occurs. Other scenarios lead to higher costs. Thus, the change from a fossil-based energy-water-food nexus to a renewable energy supply is facilitated.
As always, the model is a stylized representation of reality, and can be developed and refined in various directions. For example, the combination of the water model and the energy model includes no friction losses, evaporation, and other technical details. The optimization does not take into account the costs of installing the microgrid itself. Labor input is not explicitly modeled but relies on simplifying assumptions. Further research is planned to extend the models, and to provide an even more insightful analysis of the potential role of water management and of renewable energies in the energy-water-food nexus.
The raw data supporting the conclusions of this article will be made available by the authors, without undue reservation.
RA and KS organized the data and prepared the case study in Morocco. RD and EW prepared the numerical models. CH coordinated and supervised the research. Development of scenarios was done collectively. RA and KS wrote the first draft of the manuscript. CH wrote the interpretation of results. All authors contributed to conception and design of the study, manuscript revision, read, and approved the submitted version.
This paper benefited from funding from the international research project MG Farm (Smart microgrids as an approach to electrification in agriculture), co-financed by the Federal Ministry of Education and Research (BMBF).
We thank participants of the Swarm research seminar on SDG7-economics, in particular Georg Heinemann, for comments and suggestions. The usual disclaimer applies.
The authors declare that the research was conducted in the absence of any commercial or financial relationships that could be construed as a potential conflict of interest.
All claims expressed in this article are solely those of the authors and do not necessarily represent those of their affiliated organizations, or those of the publisher, the editors and the reviewers. Any product that may be evaluated in this article, or claim that may be made by its manufacturer, is not guaranteed or endorsed by the publisher.
1. ^https://www.cerefe.gov.dz/wp-content/uploads/2022/02/Rapport_CEREFE_TE-2020-4.pdf (accessed October 14, 2022).
2. ^This paper results from the international research project MG Farm (“Smart microgrids as an approach to electrification in agriculture”), co-financed by the Federal Ministry of Education and Research (BMBF). We thank participants of the Swarm research seminar on SDG7-economics, in particular Georg Heinemann, for comments and suggestions. The usual disclaimer applies.
3. ^“When the lines between ‘supply’ and ‘demand’ blur, the energy operator does much more than sell final energy, it mainly provides services and optimizes the use of available energy resources” (Vezzoli et al., 2018).
4. ^La Région de Marrakech-Safi, Monographie Générale, Ministère de l'Intérieur, Direction Générale des Collectivités Locales, 63 pages, 2015.
5. ^This assumption was implemented to represent the availability of manual labor on a farm, that does not have the necessary infrastructure to supply varying amounts of water to different fields and has to manually irrigate one field after another.
6. ^Note that for the Irr9-5 scenario we have assumed that the farmer is not able to irrigate different crops at the same time, contrary to the scenario of the previously discussed irrigation method and the microgrid.
7. ^This would lead to the need of binary variables, which would increase the computation time of the model drastically.
Bellfield, H. (2015). Water, Energy and Food Security Nexus in Latin America and the Caribbean. Global Canopy Programme, Oxford, 61.
Bezanson, J., Edelman, A., Karpinski, S., and Shah, V. F. (2017). Julia: a fresh approach to numerical computing. SIAM Rev. 59, 65–98. doi: 10.1137/141000671
Blasch, J., van der Kroon, B., van Beukering, P., Munster, R., Fabiani, S., Nino, P., et al. (2020). Farmer preferences for adopting precision farming technologies: a case study from Italy. Eur. Rev. Agric. Econ. 49, 33–81. doi: 10.1093/erae/jbaa031
Chen, G., Maraseni, T. N., Banhazi, T., and Bundschuh, J. (2015). Benchmarking Energy Use on Farm. 15/059.
Cozzi, L., Turk, D., Abergel, T. (2017). Digitalization and Energy. Paris: International Energy Agency.
Djaffar, S., and Kettab, A. (2018). Water Management in Algeria: What Policies, What Strategies, What Future? Research Gate 4.
Eerma, M. H., Manning, D., kland, G. L., del Angel, C., Rodriguez, P. E., Seifert, J., et al. (2022). The potential of behavioral changes to achieve a fully renewable energy system - a case study for Germany. Renew. Sustain. Energ. Transit. 2:100028. doi: 10.1016/j.rset.2022.100028
El Youssfi, L., Doorsamy, W., Aghzar, A., Cherkaoui, S. I., Elouadi, I., Faundez, A. G., et al. (2020). “Review of water energy food nexus in africa: morocco and south africa as case studies,” in E3S Web of Conferences, eds L. El Youssfi, S. I. Cherkaoui, A. Aghzar, M. Farissi, A. Godoy Faundez, D. Rivera Salazar, et al., 02002. doi: 10.1051/e3sconf/202018302002
FAO. (2022). Production of Agricultural Crops in Morocco in 2020, by Product (in Metric Tons). Available online at: https://www.statista.com/statistics/1302570/agricultural-production-in-morocco-by-product/
Faysse, N. (2015). The rationale of the green morocco plan: missing links between goals and implementation. J. North Afr. Stud. 20, 622–34. doi: 10.1080/13629387.2015.1053112
General, A. (2015). Transforming Our World: The 2030 Agenda for Sustainable Development. General Assembly. Available online at: https://documents-dds-ny.un.org/doc/UNDOC/GEN/N15/291/89/PDF/N1529189.pdf?
Göke, L. (2021a). A graph-based formulation for modeling macro-energy systems. Appl. Energy 301:117377. doi: 10.1016/j.apenergy.2021.117377
Göke, L. (2021b). AnyMOD.Jl: a julia package for creating energy system models. SoftwareX 16:100871. doi: 10.1016/j.softx.2021.100871
Göke, L., Kendziorski, M., Kemfert, C., and von Hirschhausen, C. (2022). Accounting for spatiality of renewables and storage in transmission planning. Energy Econ. 113:106190. doi: 10.1016/j.eneco.2022.106190
Granit, I. (2022). Microgrids through the energy-water-food security nexus in la Guajira, Colombia: increasing water and food security or jeopardizing groundwater levels? Energy Res. Soc. Sci. 93:102814. doi: 10.1016/j.erss.2022.102814
IEA (2018). The Future of Cooling. Available online at: https://www.iea.org/reports/the-future-of-cooling
IEA (2019). Energy Policies beyond IEA Countries: Morocco 2019. Paris. Available online at: https://www.iea.org/reports/energy-policies-beyond-iea-countries-morocco-2019
IEA (2023). Technology Cost Trends and Key Material Prices for Lithium-Ion Batteries. Technology Cost Trends and Key Material Prices for Lithium-Ion Batteries, 2017-2022. Available online at: https://www.iea.org/data-and-statistics/charts/technology-cost-trends-and-key-material-prices-for-lithium-ion-batteries-2017-2022
Ion, V., Andrei, H., Diaconu, E., Constantin Puchianu, D., and Cosmin Gheorghe, A. (2021). “Cost and energy consumption analysis of residential security systems,” in 2021 7th International Symposium on Electrical and Electronics Engineering (ISEEE) (Galati, Romania: IEEE), 1–4. doi: 10.1109/ISEEE53383.2021.9628613
Jackson, T. M., Khan, S., and Hafeez, M. (2010). A comparative analysis of water application and energy consumption at the irrigated field level. Agric. Water Manag. 97, 1477–85. doi: 10.1016/j.agwat.2010.04.013
Jacobson, M. (2020). 100% Clean, Renewble Energy and Storage for Everything. Cambridge: Cambridge University Press. doi: 10.1017/9781108786713
Jacobson, M. Z. (2023). No Miracles Needed: How Today's Technology Can Save Our Climate and Clean Our Air. Cambridge, New York, NY: Cambridge University Press. doi: 10.1017/9781009249553
Kaltschmitt, M., Streicher, W., and Wiese, A. (2010). Renewable Energy: Technology, Economics and Environment. Softcover version of original hardcover edition 2007. Berlin Heidelberg: Springer. doi: 10.1007/3-540-70949-5
Kendziorski, M., Göke, L., von Hirschhausen, C., Kemfert, C., and Zozmann, E. (2022). Centralized and decentral approaches to succeed the 100% energiewende in germany in the european context–a model-based analysis of generation, network, and storage investments. Energy Policy 167:113039. doi: 10.1016/j.enpol.2022.113039
Labrousse, M. (2006). L'énergie Répartie et La Production Décentralisée d'énergie. Les Cahiers de Global Chance 21, 7–33.
Lefore, N., Closas, A., and Schmitter, P. (2021). Solar for all: a framework to deliver inclusive and environmentally sustainable solar irrigation for smallholder agriculture. Energy Policy 154:112313. doi: 10.1016/j.enpol.2021.112313
Olivares, D. E., Mehrizi-Sani, A., Etemadi, A. H., Cañizares, A. A., Iravani, R., Kazerani, M., et al. (2014). Trends in microgrid control. IEEE Trans. Smart Grid 5, 1905–1919. doi: 10.1109/TSG.2013.2295514
Pfenninger, S., and Staffell, I. (2016). Long-term patterns of European PV output using 30 years of validated hourly reanalysis and satellite data. Energy 114, 1251–1265. doi: 10.1016/j.energy.2016.08.060
Raza, A., Razzaq, A., Mehmood, S., Zou, X., Zhang, X., Lv, Y., et al. (2019). Impact of climate change on crops adaptation and strategies to tackle its outcome: a review. Plants 8:34. doi: 10.3390/plants8020034
Smith, A. M. (1993). CLIMWAT for CROPWAT. A Climatic Database for Irrigation Planning and Management. Rome: FAO. Available online at: http://books.google.com/books?id=SCEtAQAAMAAJ
Smith, M. (1992). CROPWAT: A Computer Program for Irrigation Planning and Management. Electronic resource. Ver. 5.7. FAO Irrigation and Drainage Paper 46. Rome: Food and Agriculture Organization of the United Nations.
Sridhar, A., Balakrishnan, A., Jacob, M. M., Sillanpää, M., and Dayanandan, N. (2022). Global impact of COVID-19 on agriculture: role of sustainable agriculture and digital farming. Environ. Sci. Pollut. Res. 30, 42509–42525. doi: 10.1007/s11356-022-19358-w
Staffell, I., and Pfenninger, S. (2016). Using bias-corrected reanalysis to simulate current and future wind power output. Energy 114, 1224–39. doi: 10.1016/j.energy.2016.08.068
Stephens, E. C., Martin, G., van Wijk, M., Timsina, J., and Snow, V. (2020). Editorial: impacts of COVID-19 on agricultural and food systems worldwide and on progress to the sustainable development goals. Agric. Syst. 183:102873. doi: 10.1016/j.agsy.2020.102873
Stiftung, H. B. (2017). Comparison of Costs of Electricity Generation in Nigeria. Available online at: https://www.africanpowerplatform.org/resources/reports/west-africa/nigeria/412-comparison-of-costs-of-electricity-generation-in-nigeria.html
The Chamber of Commerce, Industry Services of the Marrakesh–Safi Region (CCISRMS). (2022). The Agriculture Sector. Available online at: https://www.ccisrms.ma/en/agriculture-2/
Timilsina, G. R. (2020). Demystifying the Costs of Electricity Generation Technologies. Working Paper. Washington, DC: World Bank. doi: 10.1596/1813-9450-9303
Vezzoli, C., Ceschin, F., Osanjo, L., M'Rithaa, M. K., Moalosi, R., Nakazibwe, V., et al. (2018). “Distributed/decentralised renewable energy systems,” in Designing Sustainable Energy for All, ed C. Vezzoli (Springer), 23–39. doi: 10.1007/978-3-319-70223-0_2
Waite, M., Cohen, E., Torbey, H., Piccirilli, M., Tian, Y., and Modi, V. (2017). Global trends in urban electricity demands for cooling and heating. Energy 127, 786–802. doi: 10.1016/j.energy.2017.03.095
Keywords: water-energy-food nexus, microgrids, renewable energy, agriculture, linear optimization, Maghreb region, Morocco
Citation: Agadi R, Sakhraoui K, Dupke RKM, Wiebrow E and von Hirschhausen C (2023) Integration of renewable energy resources into the water-energy-food nexus–Modeling a demand side management approach and application to a microgrid farm in Morocco. Front. Environ. Econ. 2:1200703. doi: 10.3389/frevc.2023.1200703
Received: 05 April 2023; Accepted: 31 July 2023;
Published: 24 August 2023.
Edited by:
Paola D'Orazio, Chemnitz University of Technology, GermanyReviewed by:
Fabien Muhirwa, Chinese Academy of Sciences (CAS), ChinaCopyright © 2023 Agadi, Sakhraoui, Dupke, Wiebrow and von Hirschhausen. This is an open-access article distributed under the terms of the Creative Commons Attribution License (CC BY). The use, distribution or reproduction in other forums is permitted, provided the original author(s) and the copyright owner(s) are credited and that the original publication in this journal is cited, in accordance with accepted academic practice. No use, distribution or reproduction is permitted which does not comply with these terms.
*Correspondence: Redha Agadi, cmVhQHdpcC50dS1iZXJsaW4uZGU=
Disclaimer: All claims expressed in this article are solely those of the authors and do not necessarily represent those of their affiliated organizations, or those of the publisher, the editors and the reviewers. Any product that may be evaluated in this article or claim that may be made by its manufacturer is not guaranteed or endorsed by the publisher.
Research integrity at Frontiers
Learn more about the work of our research integrity team to safeguard the quality of each article we publish.