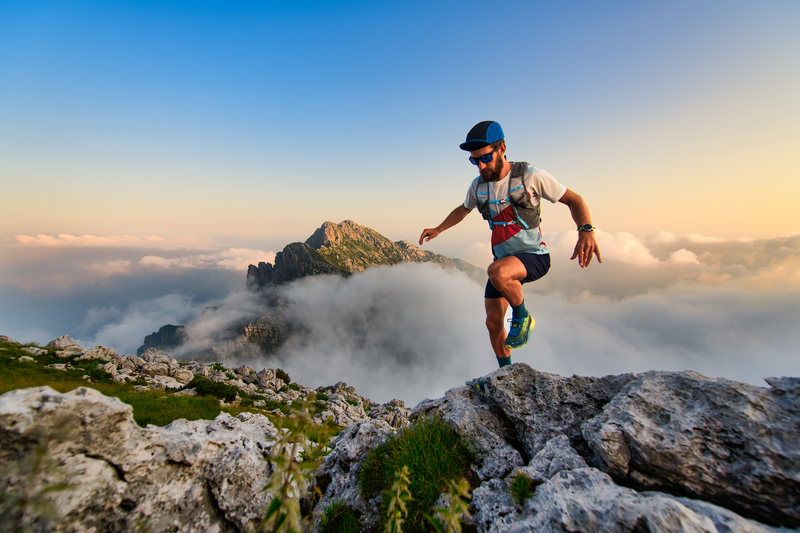
94% of researchers rate our articles as excellent or good
Learn more about the work of our research integrity team to safeguard the quality of each article we publish.
Find out more
BRIEF RESEARCH REPORT article
Front. Dement. , 29 July 2024
Sec. Aging and Risk Factors for Dementia
Volume 3 - 2024 | https://doi.org/10.3389/frdem.2024.1402091
A growing research body supports the connection between neurodegenerative disorders, including Alzheimer's disease (AD), and traffic-related air pollution (TRAP). However, the underlying mechanisms are not well understood. A deeper investigation of TRAP effects on hippocampal volume (HV), a major biomarker of neurodegeneration, may help clarify these mechanisms. Here, we explored TRAP associations with the HV in older participants of the UK Biobank (UKB), taking into account the presence of APOE e4 allele (APOE4), the strongest genetic risk factor for AD. Exposure to TRAP was approximated by the distance of the participant's main residence to the nearest major road (DNMR). The left/right HV was measured by magnetic resonance imaging (MRI) in cubic millimeters (mm3). Analysis of variance (ANOVA), Welch test, and regression were used to examine statistical significance. We found significant interactions between DNMR and APOE4 that influenced HV. Specifically, DNMR <50m (equivalent of a chronically high exposure to TRAP), and carrying APOE4 were synergistically associated with a significant (P = 0.01) reduction in the right HV by about 2.5% in women aged 60–75 years (results for men didn't reach a statistical significance). Results of our study suggest that TRAP and APOE4 jointly promote neurodegeneration in women. Living farther from major roads may help reduce the risks of neurodegenerative disorders, including AD, in female APOE4 carriers.
A growing body of research points to a connection between exposure to air pollution and neurodegenerative disorders, including Alzheimer's disease (AD), though mechanisms are not fully understood (Tham and Schikowski, 2021; Parra et al., 2022; Finch, 2023; Franz et al., 2023; Yuan et al., 2023). Various pollutants are present in the air, and some may pose risks to human health. For example, inhalable particulate matter (PM) and nitrogen dioxide (NO2) have been intensively studied in this regard (Akimoto, 2003; Craig et al., 2008; Dominski et al., 2021). A recent study of UKB (UK Biobank, 2023) data found that higher exposure to PM2.5 (median particle with diameter ≤ 2.5 μm) and NO2 was associated with multimorbidity in a dose-dependent manner (Ronaldson et al., 2022). The PM, NO2, and volatile organic compounds (VOCs) are common components of the traffic-related air pollution (TRAP). These and other types of air pollution (such as ozone, sulfur oxides, carbon monoxide, and lead), might be harmful to the central nervous system (CNS) and promote neuroinflammation and neurodegeneration (Hogan et al., 2015; Calderón-Garcidueñas et al., 2016a; Cheng et al., 2016; Spangenberg and Green, 2017; Costa et al., 2020). A review of epidemiological and experimental studies of the role of PM in neurodegeneration emphasized a link between chronic exposure to PM and onsets of cognitive deficits, dementia, and AD (You et al., 2022). A meta-analysis of 14 studies concluded that PM2.5 is a risk factor for dementia, with more limited support for nitrogen oxides, though the authors stressed that these results should be interpreted with caution (Wilker et al., 2023). Higher exposure to NO2 itself was associated with lower cortical thickness of brain regions relevant to AD (Crous-Bou et al., 2020). Another study that used the UKB data (Li et al., 2023) reported an association between residential distance to major roads and dementia that was mediated by TRAP, mainly NO2.
Exposure to environmental pollutants, including TRAP, could be especially detrimental for hippocampus, a key brain structure for learning and memory, and a primary brain region affected by AD (van der Flier and Scheltens, 2009; Rao et al., 2022). Hippocampal atrophy, manifested in reduced hippocampal volume (HV), is considered one of the major biomarkers of neurodegeneration and preclinical AD pathology (Jack et al., 2018; Grober et al., 2021). It has been associated with a decline in cognitive function and progression of mild cognitive impairment (MCI) to AD (Jack et al., 2000; Henneman et al., 2009; Qu et al., 2023). It was shown that exposure to PM can create profound metabolic disturbances in hippocampus, and adversely affect HV (Park et al., 2020; Balboni et al., 2022). A study that used brain imaging and air pollution data from the UKB found an association between higher PM2.5 concentration and smaller left HV in adult UKB participants (Hedges et al., 2019).
Genetic factors may also influence HV. For example, carrying the APOE e4 allele (APOE4), the strongest genetic risk factor for AD, may accelerate hippocampal atrophy, along with cognitive decline (Abushakra et al., 2020). Several studies (Tohgi et al., 1997; Reiman et al., 1998; O'Dwyer et al., 2012; Saeed et al., 2021) reported that individuals with APOE4 have markedly smaller HV, along with increased risks of AD and other dementias, compared to those without APOE4. The APOE4 may also interact with exposure to air pollution, including TRAP, potentially modifying its effects on AD-related traits (Schikowski et al., 2015; Ma et al., 2023).
In this study, we used the UKB data to further explore the interactions between APOE4 and TRAP, to better understand how the exposure to TRAP may influence HV in older adults, who carry the strongest genetic risk factor for AD.
This study was performed using the UKB (UK Biobank, 2023), a population-based study with extensive genetic and phenotypic data for approximately 500,000 individuals from across the UK. Data for the study were obtained (November, 2019) from the UKB database. Written informed consent was obtained by the UKB from the participants in accordance with the UK national legislation and the UKB requirements. The latest (at the time of calculations) available information on participants' withdrawal in UKB was taken into account.
In our analysis, TRAP was approximated by the participant's residence distance (in meters) to the nearest major road (DNMR). The DNMR was defined based on the local road network taken from the Ordnance Survey Meridian 2 road network 2009 with scale 1:50,000 and one meter accuracy (McGarva, 2017; Environmental Exposures Metadata and Resource 2010 UK, 2023). The median value of the DNMR was 377.4 (interquartile range: [165.9, 751.9]).
Among those subjects who had both DNMR and APOE4 carrier status information, participants aged between 60 and 75 years, who attended the assessment center during the first imaging visit (starting January 1, 2014), were chosen. The APOE4 carrier status was approximated by carrying C allele of the SNP rs429358. The left and right HV were measured in cubic millimeters (mm3), and respective information was obtained from the UKB data-fields 25019 and 25020. To normalize for head size, these measurements were multiplied by the head size scaling factor obtained from the UKB data-field 25,000 (Smith et al., 2022; Supplementary material, MRI measurements).
The analytic sample (Table 1) was divided into one factor and two factor groups, as follows:
G1. One factor groups
DNMR group consists of subjects with residential proximity to the nearest major road <50m, noDNMR group consists of subjects with residential proximity to the nearest major road more than 50m, APOE4 group consists of APOE4 carriers, noAPOE4 group consists of APOE4 non-carriers.
G2. Two factor groups
DNMR_APOE4 group contains subjects from both DNMR and APOE4 groups, DNMR_noAPOE4 group contains subjects from both DNMR and noAPOE4 groups, noDNMR_APOE4 group contains subjects from both noDNMR and APOE4 groups, noDNMR_noAPOE4 group contains subjects from both noDNMR and noAPOE4 groups.
The study sample contained participants having DNMR and APOE4 carrier status data, who attended the assessment center during the first imaging visit (between January 1, 2014 and October 31, 2019) at age 60–75 years. It, thus, only included individuals, who were at risk for the late-onset but not the early onset AD.
Analysis of variance (ANOVA), the Tukey's test, and the Welch test (Welch, 1947; Chambers et al., 1992; Yandell, 1997) were utilized. We considered three sets of regression models Set1 = HV~Age,dnmr (8 models), Set2 = HV~Age,snp (8 models), and Set3 = HV~Age,snp,dnmr (64 models) (Supplementary material, Analytic approach) having HV as a response variable HV = HV (mm3) left/right and independent variables: dnmr = 1 (DNMR <50), dnmr = 0 (DNMR ≥ 50), snp = 1 (APOE4 carrier), snp = 0 (APOE4 non-carrier), and age at the time attending assessment center during the first imaging visit as the Age variable.
The regression models were evaluated using the Akaike information criterion (AIC) (Akaike, 1973). The optimal, with respect to the minimal AIC criteria, significant results for regression model were found for the regression sets described above. Here, significant regression model means that all regression coefficients were significant (P < 0.05) in a specific model, non-significance means the opposite. R standard software packages (version 3.6.3), along with glmulti package (Calcagno, 2022), were utilized.
We found significant difference in the right HV between groups DNMR and noDNMR, between groups APOE4 and noAPOE4, and between groups DNMR_APOE4 and noDNMR_noAPOE4 for females aged 60–75 years (Table 2, Figure 1). One can see that there was a 0.5% decrease in the right HV for APOE4 carriers, a 1.0% decrease in the right HV for those with DNMR <50, and a 2.5% decrease in right HV for APOE4 carriers with DNMR <50. Note that joint impact of DNMR and APOE4 is larger than separate contributions of APOE4 and DNMR (2.5% > 0.5%, 2.5% > 1.0%), or their sum (2.5% > 0.5% + 1.0%).
Figure 1. The right HV (mm3) in groups of women aged 60-75 years, by DNMR and APOE4 status. Age—age at the time attending the assessment center during the first imaging visit between January 1, 2014 and October 31, 2019. (A) UKB, HV right, DNMR < 50 m, females, aged 60–75 years. DNMR (HV: mean = 5,082, 95% CI: 5,038–5,128), noDNMR (HV: mean = 5,135, 95% CI: 5,123–5,146). (B) UKB, HV right, APOE4, females, aged 60–75 years. APOE4 (HV: mean = 5,112, 95% CI: 5,091–5,134), no APOE4 (HV: 5,139, 95% CI: 5,126–5,151). (C) UKB, HV right, DNMR < 50m and APOE4, females, age 60–75 years, DNMR_APOE4 (HV: mean = 5,012, 95% CI: 4,934–5,098), DNMR_noAPOE4 (HV: mean = 5,112, 95% CI: 5,059–5,161) noDNMR_APOE4 (HV: mean = 5,120, 95% CI: 5,098–5,140), noDNMR no APOE4 (HV: mean = 5,140, 95% CI: 5,127–5,154). For more detailed statistics, see Table 2.
There was a 0.6% decrease in the left HV for APOE4 carriers in women aged 60–75 years; differences between other groups were not statistically significant (Supplementary Table 1, Supplementary Figure 1). Normal aging is associated with gradual reducing of HV even without any possible adverse factors (Harman, 2001; Fotuhi et al., 2012; López-Otín et al., 2013). In our analysis, we performed the Welch test to check a possible difference in age between groups (Supplementary Tables 2, 3). We found that on average the subjects in the group DNMR were older than the subjects in the group noDNMR, which might contribute to the reduced HV in the group DNMR compared to the group noDNMR. The subjects in the group APOE4, on average, were younger than the subjects in the group noAPOE4. Note that such age difference between APOE4 and noAPOE4 groups strengthened our results because younger subjects generally tend to have bigger HV than the older ones. The subjects in the group DNMR_APOE4, on average, were the same age as the subjects in the group noDNMR_noAPOE4.
Difference in the right HV between two groups DNMR and noDNMR in the Figure 1A could be attributed to the age only, with participants in the DNMR group older than participants in the noDNMR group (Supplementary Table 3). Based on Table 3, the decrease in the right HV became more pronounced with age in women aged 60–75 years: from 0.8% at age 60 to 0.9% at age 75 for the right HV. Difference in HV between two groups APOE4 and noAPOE4 in the Figure 1B could be attributed to the age and APOE4 carrier status, with participants being younger in the APOE4 compared those in noAPOE4 (Supplementary Table 3). Based on Table 3, the decrease in the right HV became more pronounced with age in women aged 60–75 years: from 0.6% at age 60 to 0.8% at age 75 for the right HV. When taking into account three factors (age, proximity to the nearest major road, and APOE4 carrier status), based on Table 3, difference in the right HV gradually increased from 2.4% at age 60 to 2.8% at age 75, for APOE4 carriers with proximity to the nearest major road <50m. The right HV decreased with age in women aged 60–75 years, losing about 27 mm3/year.
For males aged 60–75 years differences in the left/right HV between studied groups (Supplementary Tables 4, 5) were not statistically significant. For males aged 60–75 years, regression analysis found that for all regression sets Set1 = HV~Age,dnmr (8 models), Set2 = HV~Age,snp (8 models), Set3 = HV~Age,snp,dnmr (64 models) the best (with respect to the minimal AIC criterion) models depend only on Age variable (Supplementary Tables 6–8).
Comparison of the regression model with interactive term with the reference model, i.e., the regression with main additive effects (Table 3, model 13 in Set3: HV~Age,snp,dnmr) allows estimating deviation from the reference model, which was: deviation = 106.2 + 0.4*Age-(43.3 + 32.7) = 54.2, for Age = 60 and deviation = 60.2, for Age = 75, that is, deviation gradually increases with age. This observation reasonably supported synergy (Roell et al., 2017) in the interaction between DNMR and APOE e4 status with respect to HV decrease.
Our study, using the UKB data, found that female APOE4 carriers aged 60–75 years, who live <50 meters from a major road, had the right HV that was significantly smaller (by about 2.5%) than the HV of the same age women without these conditions. We also showed for the first time that exposure to TRAP (approximated by closeness of participant's main residence to major roads), and carrying the APOE4, synergistically affected HV in women. These findings imply that living farther from major roads may be especially beneficial to older female APOE4 carriers and could help reduce their risks of neurodegenerative disorders, including AD. In our study, the right HV also decreased with age in women aged 60–75 years, losing, on average, about 27 mm3/year. This is in agreement with an earlier report of the HV change with age by the UKB (Nobis et al., 2019).
Results of our study are broadly in line with earlier research that demonstrated that exposure to air pollution (especially to PM2.5) is associated with smaller brain/hippocampal volume (Wilker et al., 2015; Hedges et al., 2019; Balboni et al., 2022). Several studies investigated the role of air pollution in dementia and cognitive decline, including in APOE4 carriers. Chen et al. (2017) reported a modest increase in hazard ratio (1.07 [95% CI: 1.06–1.08]) of dementia in people living <50 meters from a major road. Higher PM2.5 exposure was linked to worse cognitive function in APOE4 carriers, but not in non-carriers (Franz et al., 2023). A paper found that associations of PM2.5, PM10, and NO2 with cognitive function were more pronounced in female APOE4 carriers (Schikowski et al., 2015). Female APOE4 carriers were also more at risk for air pollution-induced metabolic alterations in hippocampus and cognitive deficits (Calderón-Garcidueñas et al., 2015, 2016b). Another research that used the Women's Health Initiative Memory Study (WHIMS) data found that exposure to a high level of PM2.5 preceded onset of cognitive impairment in older women, and this relationship varied by APOE genotype, with the largest adverse effect seen in e4/e4 carriers (Cacciottolo et al., 2017). The authors suggested that exposure to PM in the air may accelerate neurodegeneration through various pathways, amyloidogenic, as well as independent of amyloid deposits. A more recent study tested the interaction between APOE genotypes and air pollution and found that the long-term exposure to ambient air pollution was associated with a more rapid cognitive decline in APOE4 carriers (Kulick et al., 2020). Some studies, however, did not find significant interactions between the air pollution and APOE4. For example, a case-control study in northern Taiwan found no differences in susceptibility to air pollution-associated dementia between APOE genotypes (Wu et al., 2015).
One should note that DNMR, which was used as an explanatory variable in our analysis, is an indicator of aggregated exposure to various road-related pollutants, not only to those found in car exhaust fumes. Some of these pollutants may also be potentially relevant to AD pathology. E.g., the higher intensity traffic was associated with the higher concentration of airborne fungi in urban air environments. Examples include Alternaria and Cladosporium species which may cause infection and inflammation, potentially contributing to neurodegeneration (Alonso et al., 2017; Phuna and Madhavan, 2022; Muafa et al., 2024). The role of exposure to airborne fungi in AD pathology deserves separate investigation, especially in the light of our recent findings suggesting that the impact of recurrent fungal infections on AD risk can be larger than that of other types of infections, including bacterial and viral ones (Ukraintseva et al., 2023). Other road-related pollutants, such as noise (The Lancet Regional Health-Europe, 2023), light pollution (Chepesiuk, 2009; Wyse et al., 2011; Aubrecht et al., 2013), and electromagnetic fields (Ahlbom and Feychting, 2003; Kivrak et al., 2017) might also be relevant to health risks. For instance, noise is currently considered a health problem for citizens of the European Union (European Commission, 2023).
We recognize several study limitations. Since only individuals aged 60–75, who have HV measures, were included in the analysis, the sample size in this study was substantially reduced compared to the total UK Biobank sample. Also, different head-size correction (normalization) strategies might yield various volumetric results across studies (Arndt et al., 1991; Mathalon et al., 1993; Goldstein et al., 1999; Seidman et al., 1999; Sanfilipo et al., 2004; Barnes et al., 2010; O'Brien et al., 2011; Voevodskaya et al., 2014). Also, in our study we evaluated regression models using the Akaike information criterion. One should note that there is no universal procedure by which one can determine the “best model”. We applied the AIC approach calculating goodness-of-fit and model variability in order to select the most parsimonious regression model (Burnham and Anderson, 2002; Anderson, 2008; Burnham et al., 2011). Another potential limitation could be that the formal statistical association evaluated from regression analysis may not imply actual causality, which should be further studied using causal inference approaches. Finally, the UK Biobank is volunteer-based study, and so it may not represent general population, therefore, results obtained using this sample should not be extrapolated to the entire UK population, or to other populations, and need further confirmation in additional research.
In summary, this study found that the interaction between APOE4 carrier status and chronic exposure to TRAP (approximated by the closeness of a participant's main residence to a major road) is associated with a significant reduction in hippocampal volume (HV) in female participants of the UK Biobank aged 60–75 years. The results for males didn't reach statistical significance. Our findings suggest that traffic-related air pollution and genetic risk factors for AD (specifically APOE4) can synergistically promote neurodegeneration. Living farther from major roads could help reduce the risks of neurodegenerative disorders, including AD, in older female APOE4 carriers.
This study used de-identified data provided by the UK Biobank (https://www.ukbiobank.ac.uk). This data is not freely available to the public but can be accessed upon approval of a data request by the UK Biobank. Specific policies governing the process to access the UK Biobank data can be found online at https://www.ukbiobank.ac.uk/enable-your-research/apply-for-access.
The studies involving human subjects were approved by the Duke University Health System Institutional Review Board in accordance with the local legislation and institutional requirements. This publication includes only secondary analyses of existing data collected by the UK Biobank and does not include identifiable human data. Written informed consent for the UK Biobank participants was obtained by the UK Biobank (data holder) in accordance with the UK national legislation and the UK Biobank requirements. The latest (at time of calculations) available information on participants' withdrawal in the UK Biobank was taken into account.
VP: Conceptualization, Formal analysis, Investigation, Methodology, Software, Validation, Visualization, Writing – original draft, Writing – review & editing. SU: Conceptualization, Formal analysis, Investigation, Methodology, Project administration, Supervision, Writing – original draft, Writing – review & editing. HD: Data curation, Investigation, Validation, Writing – review & editing. AY: Investigation, Methodology, Writing – review & editing. KA: Investigation, Methodology, Writing – review & editing.
The author(s) declare financial support was received for the research, authorship, and/or publication of this article. This research was supported by the National Institutes of Health's National Institute on Aging (NIA/NIH) grant R01AG062623.
This research has been conducted using the UK Biobank Resource under application number 55337.
The authors declare that the research was conducted in the absence of any commercial or financial relationships that could be construed as a potential conflict of interest.
The author(s) declared that they were an editorial board member of Frontiers, at the time of submission. This had no impact on the peer review process and the final decision.
All claims expressed in this article are solely those of the authors and do not necessarily represent those of their affiliated organizations, or those of the publisher, the editors and the reviewers. Any product that may be evaluated in this article, or claim that may be made by its manufacturer, is not guaranteed or endorsed by the publisher.
This content is solely the responsibility of the authors and does not necessarily represent the official views of the NIA/NIH.
The Supplementary Material for this article can be found online at: https://www.frontiersin.org/articles/10.3389/frdem.2024.1402091/full#supplementary-material
Abushakra, S., Porsteinsson, A. P., Sabbagh, M., Bracoud, L., Schaerer, J., Power, A., et al. (2020). APOE ε4/ε4 homozygotes with early Alzheimer's disease show accelerated hippocampal atrophy and cortical thinning that correlates with cognitive decline. Alzheimer's Dement. 6:e12117. doi: 10.1002/trc2.12117
Ahlbom, A., and Feychting, M. (2003). Electromagnetic radiation: environmental pollution and health. Br. Med. Bull. 68, 157–165. doi: 10.1093/bmb/ldg030
Akaike, H. (1973). “Information theory and an extension of the maximum likelihood principle,” in 2nd International Symposium on Information Theory, eds. B. N. Petrov, F. Csáki, and T. Armenia (Budapest: Akadémiai Kiadó), 267–281
Akimoto, H. (2003). Global air quality and pollution. Science 302, 1716–1719. doi: 10.1126/science.1092666
Alonso, R., Pisa, D., Aguado, B., and Carrasco, L. (2017). Identification of fungal species in brain tissue from Alzheimer's disease by next-generation sequencing. J. Alzheimer's Dis. 58, 55–67. doi: 10.3233/JAD-170058
Anderson, D. R. (2008). Model Based Inference in the Life Sciences: A Primer on Evidence. New York: Springer.
Arndt, S., Cohen, G., Alliger, R. J., Swayze, V. W., and Andreasen, N. C. (1991). Problems with ratio and proportion measures of imaged cerebral structures. Psychiatry Res. 40, 79–89. doi: 10.1016/0925-4927(91)90031-K
Aubrecht, T. G., Weil, Z. M., Magalang, U. J., and Nelson, R. J. (2013). Dim light at night interacts with intermittent hypoxia to alter cognitive and affective responses. Am. J. Physiol. Regul. Integr. Comp. Physiol. 305, R78–R86. doi: 10.1152/ajpregu.00100.2013
Balboni, E., Filippini, T., Crous-Bou, M., Guxens, M., Erickson, L. D., and Vinceti, M. (2022). The association between air pollutants and hippocampal volume from magnetic resonance imaging: a systematic review and meta-analysis. Environm. Res. 204:111976. doi: 10.1016/j.envres.2021.111976
Barnes, J., Ridgway, G. R., Bartlett, J., Henley, S. M., Lehmann, M., Hobbs, N., et al. (2010). Head size, age and gender adjustment in MRI studies: a necessary nuisance? Neuroimage 53, 1244–1255. doi: 10.1016/j.neuroimage.2010.06.025
Burnham, K. P., and Anderson, D. R. (2002). Model Selection and Multimodel Inference: A Practical Information-Theoretic Approach (2nd ed). New York, NY: Springer.
Burnham, K. P., Anderson, D. R., and Huyvaert, K. P. (2011). AIC model selection and multimodel inference in behavioral ecology: some background, observations, and comparisons. Behav. Ecol. Sociobiol. 65, 23–35. doi: 10.1007/s00265-010-1029-6
Cacciottolo, M., Wang, X., Driscoll, I., Woodward, N., Saffari, A., Reyes, J., et al. (2017). Particulate air pollutants, APOE alleles and their contributions to cognitive impairment in older women and to amyloidogenesis in experimental models. Transl. Psychiatry 7:e1022. doi: 10.1038/tp.2016.280
Calcagno, V. (2022). R Package Glmulti. Available online at: https://cran.r-project.org/web/packages/glmulti/glmulti.pdf (accessed December 11, 2023).
Calderón-Garcidueñas, L., Avila-Ramírez, J., Calderón-Garcidueñas, A., González-Heredia, T., Acuña-Ayala, H., Chao, C. K., et al. (2016a). Cerebrospinal fluid biomarkers in highly exposed PM2.5 urbanites: the risk of alzheimer's and parkinson's diseases in young Mexico City residents. J. Alzheimer's Dis. 54, 597–613. doi: 10.3233/JAD-160472
Calderón-Garcidueñas, L., Kulesza, R. J., Doty, R. L., D'Angiulli, A., and Torres-Jardón, R. (2015). Megacities air pollution problems: Mexico City Metropolitan Area critical issues on the central nervous system pediatric impact. Environ. Res. 137, 157–169. doi: 10.1016/j.envres.2014.12.012
Calderón-Garcidueñas, L., Reynoso-Robles, R., Vargas-Martínez, J., Gómez-Maqueo-Chew, A., Pérez-Guillé, B., Mukherjee, P. S., et al. (2016b). Prefrontal white matter pathology in air pollution exposed Mexico City young urbanites and their potential impact on neurovascular unit dysfunction and the development of Alzheimer's disease. Environ. Res. 146, 404–417. doi: 10.1016/j.envres.2015.12.031
Chambers, J. M., Freeny, A., and Heiberger, R. M. (1992). “Analysis of variance; designed experiments,” in Chapter 5 of Statistical Models in S, eds J. M. Chambers and T. J. Hastie (Pacific Grove: Wadsworth & Brooks/Cole).
Chen, H., Kwong, J. C., Copes, R., Tu, K., Villeneuve, P. J., van Donkelaar, A., et al. (2017). Living near major roads and the incidence of dementia, Parkinson's disease, and multiple sclerosis: a population-based cohort study. Lancet 389, 718–726. doi: 10.1016/S0140-6736(16)32399-6
Cheng, H., Davis, D. A., Hasheminassab, S., Morgan, T. E., and Finch, C. E. (2016). Urban traffic-derived nanoparticulate matter reduces neurite outgrowth via TNFα in vitro. J. Neuroinflammation 13:19. doi: 10.1186/s12974-016-0480-3
Chepesiuk, R. (2009). Missing the dark: health effects of light pollution. Environ. Health Perspect. 117, A20–A27. doi: 10.1289/ehp.117-a20
Costa, L. G., Cole, T. B., Dao, K., Chang, Y. C., Coburn, J., and Garrick, J. M. (2020). Effects of air pollution on the nervous system and its possible role in neurodevelopmental and neurodegenerative disorders. Pharmacol. Ther. 210:107523. doi: 10.1016/j.pharmthera.2020.107523
Craig, L., Brook, J. R., Chiotti, Q., Croes, B., Gower, S., Hedley, A., et al. (2008). Air pollution and public health: a guidance document for risk managers. J. Toxicol. Environ. Health Part A 71, 588–698. doi: 10.1080/15287390801997732
Crous-Bou, M., Gascon, M., Gispert, J. D., Cirach, M., Sánchez-Benavides, G., Falcon, C., et al. (2020). Impact of urban environmental exposures on cognitive performance and brain structure of healthy individuals at risk for Alzheimer's dementia. Environ. Int. 138, 105546. doi: 10.1016/j.envint.2020.105546
Dominski, F. H., Lorenzetti Branco, J. H., Buonanno, G., Stabile, L., Gameiro da Silva, M., and Andrade, A. (2021). Effects of air pollution on health: a mapping review of systematic reviews and meta-analyses. Environ. Res. 201, 111487. doi: 10.1016/j.envres.2021.111487
Environmental Exposures Metadata and Resource 2010 UK (2023). Biobank. Available online at: https://www.ukbiobank.ac.uk (accessed December 11, 2023).
European Commission (2023). Environmental Noise Directive. Available online at: https://environment.ec.europa.eu/topics/noise/environmental-noise-directive_en (accessed July 17, 2024).
Finch, C. E. (2023). Air pollution, dementia, and lifespan in the socio-economic gradient of aging: perspective on human aging for planning future experimental studies. Front. Aging 4:1273303. doi: 10.3389/fragi.2023.1273303
Fotuhi, M., Do, D., and Jack, C. (2012). Modifiable factors that alter the size of the hippocampus with ageing. Nat. Rev. Neurol. 8, 189–202. doi: 10.1038/nrneurol.2012.27
Franz, C. E., Gustavson, D. E., Elman, J. A., Fennema-Notestine, C., Hagler, D. J., Baraff, A., et al. (2023). Associations between ambient air pollution and cognitive abilities from midlife to early old age: modification by APOE genotype. J. Alzheimers. Dis. 93, 193–209. doi: 10.3233/JAD-221054
Goldstein, J. M., Goodman, J. M., Seidman, L. J., Kennedy, D. N., Makris, N., Lee, H., et al. (1999). Cortical abnormalities in schizophrenia identified by structural magnetic resonance imaging. Arch. Gen. Psychiatry 56, 537–547. doi: 10.1001/archpsyc.56.6.537
Grober, E., Papp, K. V., Rentz, D. M., Sperling, R. A., Johnson, K. A., Amariglio, R. E., et al. (2021). Neuroimaging correlates of Stages of Objective Memory Impairment (SOMI) system. Alzheimer's Dement. 13:e12224. doi: 10.1002/dad2.12224
Harman, D. (2001). Aging: overview. Ann. N. Y. Acad. Sci. 928, 1–21. doi: 10.1111/j.1749-6632.2001.tb05631.x
Hedges, D. W., Erickson, L. D., Kunzelman, J., Brown, B. L., and Gale, S. D. (2019). Association between exposure to air pollution and hippocampal volume in adults in the UK Biobank. Neurotoxicology 74, 108–120. doi: 10.1016/j.neuro.2019.06.005
Henneman, W. J., Sluimer, J. D., Barnes, J., van der Flier, W. M., Sluimer, I. C., Fox, N. C., et al. (2009). Hippocampal atrophy rates in Alzheimer disease: added value over whole brain volume measures. Neurology 72, 999–1007. doi: 10.1212/01.wnl.0000344568.09360.31
Hogan, M. K., Kovalycsik, T., Sun, Q., Rajagopalan, S., and Nelson, R. J. (2015). Combined effects of exposure to dim light at night and fine particulate matter on C3H/HeNHsd mice. Behav. Brain Res. 294, 81–88. doi: 10.1016/j.bbr.2015.07.033
Jack, C. R., Bennett, D. A., Blennow, K., Carrillo, M. C., Dunn, B., Haeberlein, S. B., et al. (2018). NIA-AA Research Framework: Toward a biological definition of Alzheimer's disease. Alzheimer's Dement. 14, 535–562. doi: 10.1016/j.jalz.2018.02.018
Jack, C. R., Petersen, R. C., Xu, Y., O'Brien, P. C., Smith, G. E., Ivnik, R. J., et al. (2000). Rates of hippocampal atrophy correlate with change in clinical status in aging and AD. Neurology 55, 484–489. doi: 10.1212/WNL.55.4.484
Kivrak, E. G., Yurt, K. K., Kaplan, A. A., Alkan, I., and Altun, G. (2017). Effects of electromagnetic fields exposure on the antioxidant defense system. J. Microsc. Ultrastruct. 5, 167–176. doi: 10.1016/j.jmau.2017.07.003
Kulick, E. R., Elkind, M. S. V., Boehme, A. K., Joyce, N. R., Schupf, N., Kaufman, J. D., et al. (2020). Long-term exposure to ambient air pollution, APOE-ε4 status, and cognitive decline in a cohort of older adults in northern Manhattan. Environ. Int. 136, 105440. doi: 10.1016/j.envint.2019.105440
Li, C., Gao, D., Cai, Y. S., Liang, J., Wang, Y., Pan, Y., et al. (2023). Relationships of residential distance to major traffic roads with dementia incidence and brain structure measures: mediation role of air pollution. Health Data Sci. 3:0091. doi: 10.34133/hds.0091
López-Otín, C., Blasco, M. A., Partridge, L., Serrano, M., and Kroemer, G. (2013). The hallmarks of aging. Cell 153, 1194–1217. doi: 10.1016/j.cell.2013.05.039
Ma, H., Li, X., Zhou, T., Wang, M., Heianza, Y., and Qi, L. (2023). Long-term exposure to low-level air pollution, genetic susceptibility and risk of dementia. Int. J. Epidemiol. 52, 738–748. doi: 10.1093/ije/dyac146
Mathalon, D. H., Sullivan, E. V., Rawles, J. M., and Pfefferbaum, A. (1993). Correction for head size in brain-imaging measurements. Psychiatry Res. 50, 121–139. doi: 10.1016/0925-4927(93)90016-B
McGarva, G. (2017). GB Transportation Network (1:50 000 Meridian 2). Edinburgh: University of Edinburgh. Available online at: https://datashare.ed.ac.uk/handle/10283/2560 (accessed December 11, 2023).
Muafa, M. H. M., Quach, Z. M., Al-Shaarani, A. A. Q. A., Nafis, M. M. H., and Pecoraro, L. (2024). The influence of car traffic on airborne fungal diversity in Tianjin, China. Mycology. doi: 10.1080/21501203.2023.2300343
Nobis, L., Manohar, S. G., Smith, S. M., Alfaro-Almagro, F., Jenkinson, M., Mackay, C. E., et al. (2019). Hippocampal volume across age: nomograms derived from over 19,700 people in UK Biobank. NeuroImage. Clinical 23:101904. doi: 10.1016/j.nicl.2019.101904
O'Brien, L. M., Ziegler, D. A., Deutsch, C. K., Frazier, J. A., Herbert, M. R., and Locascio, J. J. (2011). Statistical adjustments for brain size in volumetric neuroimaging studies: some practical implications in methods. Psychiatry Res. 193, 113–122. doi: 10.1016/j.pscychresns.2011.01.007
O'Dwyer, L., Lamberton, F., Matura, S., Tanner, C., Scheibe, M., Miller, J., et al. (2012). Reduced hippocampal volume in healthy young ApoE4 carriers: an MRI study. PLoS ONE 7:e48895. doi: 10.1371/journal.pone.0048895
Park, S. J., Lee, J., Lee, S., Lim, S., Noh, J., Cho, S. Y., et al. (2020). Exposure of ultrafine particulate matter causes glutathione redox imbalance in the hippocampus: a neurometabolic susceptibility to Alzheimer's pathology. Sci. Total Environ. 718:137267. doi: 10.1016/j.scitotenv.2020.137267
Parra, K. L., Alexander, G. E., Raichlen, D. A., Klimentidis, Y. C., and Furlong, M. A. (2022). Exposure to air pollution and risk of incident dementia in the UK Biobank. Environ. Res. 209, 112895. doi: 10.1016/j.envres.2022.112895
Phuna, Z. X., and Madhavan, P. (2022). A closer look at the mycobiome in Alzheimer's disease: Fungal species, pathogenesis and transmission. Eur. J. Neurosci. 55, 1291–1321. doi: 10.1111/ejn.15599
Qu, H., Ge, H., Wang, L., Wang, W., and Hu, C. (2023). Volume changes of hippocampal and amygdala subfields in patients with mild cognitive impairment and Alzheimer's disease. Acta Neurol. Belg., 123,1381–1393. doi: 10.1007/s13760-023-02235-9
Rao, Y. L., Ganaraja, B., Murlimanju, B. V., Joy, T., Krishnamurthy, A., and Agrawal, A. (2022). Hippocampus and its involvement in Alzheimer's disease: a review. 3 Biotech 12:55. doi: 10.1007/s13205-022-03123-4
Reiman, E. M., Uecker, A., Caselli, R. J., Lewis, S., Bandy, D., de Leon, M. J., et al. (1998). Hippocampal volumes in cognitively normal persons at genetic risk for Alzheimer's disease. Ann. Neurol. 44, 288–291. doi: 10.1002/ana.410440226
Roell, K. R., Reif, D. M., and Motsinger-Reif, A. A. (2017). An introduction to terminology and methodology of chemical synergy-perspectives from across disciplines. Front. Pharmacol. 8:158. doi: 10.3389/fphar.2017.00158
Ronaldson, A., Arias de la Torre, J., Ashworth, M., Hansell, A. L., Hotopf, M., Mudway, I., et al. (2022). Associations between air pollution and multimorbidity in the UK Biobank: A cross-sectional study. Front. Public Health 10:1035415. doi: 10.3389/fpubh.2022.1035415
Saeed, U., Desmarais, P., and Masellis, M. (2021). The APOE ε4 variant and hippocampal atrophy in Alzheimer's disease and Lewy body dementia: a systematic review of magnetic resonance imaging studies and therapeutic relevance. Expert Rev. Neurother. 21, 851–870. doi: 10.1080/14737175.2021.1956904
Sanfilipo, M. P., Benedict, R. H., Zivadinov, R., and Bakshi, R. (2004). Correction for intracranial volume in analysis of whole brain atrophy in multiple sclerosis: the proportion vs. residual method. NeuroImage 22, 1732–1743. doi: 10.1016/j.neuroimage.2004.03.037
Schikowski, T., Vossoughi, M., Vierkötter, A., Schulte, T., Teichert, T., Sugiri, D., et al. (2015). Association of air pollution with cognitive functions and its modification by APOE gene variants in elderly women. Environ. Res. 142, 10–16. doi: 10.1016/j.envres.2015.06.009
Seidman, L. J., Faraone, S. V., Goldstein, J. M., Goodman, J. M., Kremen, W. S., Toomey, R., et al. (1999). Thalamic and amygdala-hippocampal volume reductions in first-degree relatives of patients with schizophrenia: an MRI-based morphometric analysis. Biol. Psychiatry 46, 941–954. doi: 10.1016/S0006-3223(99)00075-X
Smith, M. S., Alfaro-Almagro, F., and Miller, K. L. (2022). “UK Biobank brain imaging documentation, version 1.9, December 2022,” in Wellcome Centre for Integrative Neuroimaging (WIN-FMRIB) (Oxford: Oxford University). Available online at: https://biobank.ndph.ox.ac.uk (accessed December 11, 2023).
Spangenberg, E. E., and Green, K. N. (2017). Inflammation in Alzheimer's disease: lessons learned from microglia-depletion models. Brain Behav. Immun. 61, 1–11. doi: 10.1016/j.bbi.2016.07.003
Tham, R., and Schikowski, T. (2021). The role of traffic-related air pollution on neurodegenerative diseases in older people: an epidemiological perspective. J. Alzheimer's Dis. 79, 949–959. doi: 10.3233/JAD-200813
The Lancet Regional Health-Europe (2023). Noise pollution: more attention is needed. The Lancet regional health. Europe 24:100577. doi: 10.1016/j.lanepe.2022.100577
Tohgi, H., Takahashi, S., Kato, E., Homma, A., Niina, R., Sasaki, K., et al. (1997). Reduced size of right hippocampus in 39- to 80-year-old normal subjects carrying the apolipoprotein E epsilon4 allele. Neurosci. Lett. 236, 21–24. doi: 10.1016/S0304-3940(97)00743-X
UK Biobank (2023). Available online at: https://www.ukbiobank.ac.uk (accessed December 11, 2023).
Ukraintseva, S., Yashkin, A. P., Akushevich, I., Arbeev, K., Duan, H., Gorbunova, G., et al. (2023). Associations of infections and vaccines with Alzheimer's disease point to a major role of compromised immunity rather than specific pathogen in AD. medRxiv [preprint] medRxiv 2023.12.04.23299092. doi: 10.1101/2023.12.04.23299092
van der Flier, W. M., and Scheltens, P. (2009). Alzheimer disease: hippocampal volume loss and Alzheimer disease progression. Nat. Rev. Neurol. 5, 361–362. doi: 10.1038/nrneurol.2009.94
Voevodskaya, O., Simmons, A., Nordenskjöld, R., Kullberg, J., Ahlström, H., Lind, L., et al. (2014). The effects of intracranial volume adjustment approaches on multiple regional MRI volumes in healthy aging and Alzheimer's disease. Front. Aging Neurosci. 6:264. doi: 10.3389/fnagi.2014.00264
Welch, B. L. (1947). The generalisation of student's problems when several different population variances are involved. Biometrika 34, 28–35. doi: 10.1093/biomet/34.1-2.28
Wilker, E. H., Osman, M., and Weisskopf, M. G. (2023). Ambient air pollution and clinical dementia: systematic review and meta-analysis. BMJ 381:e071620. doi: 10.1136/bmj-2022-071620
Wilker, E. H., Preis, S. R., Beiser, A. S., Wolf, P. A., Au, R., Kloog, I., et al. (2015). Long-term exposure to fine particulate matter, residential proximity to major roads and measures of brain structure. Stroke 46, 1161–1166. doi: 10.1161/STROKEAHA.114.008348
Wu, Y. C., Lin, Y. C., Yu, H. L., Chen, J. H., Chen, T. F., Sun, Y., et al. (2015). Association between air pollutants and dementia risk in the elderly. Alzheimer's Dement. 1, 220–228. doi: 10.1016/j.dadm.2014.11.015
Wyse, C. A., Selman, C., Page, M. M., Coogan, A. N., and Hazlerigg, D. G. (2011). Circadian desynchrony and metabolic dysfunction; did light pollution make us fat?. Med. Hypotheses 77, 1139–1144. doi: 10.1016/j.mehy.2011.09.023
Yandell, B. S. (1997). Practical Data Analysis for Designed Experiments. Boca Raton, FL: Chapman & Hall. doi: 10.1007/978-1-4899-3035-4
You, R., Ho, Y. S., and Chang, R. C. (2022). The pathogenic effects of particulate matter on neurodegeneration: a review. J. Biomed. Sci. 29:15. doi: 10.1186/s12929-022-00799-x
Yuan, S., Huang, X., Zhang, L., Ling, Y., Tan, S., Peng, M., et al. (2023). Associations of air pollution with all-cause dementia, Alzheimer's disease, and vascular dementia: a prospective cohort study based on 437,932 participants from the UK biobank. Front. Neurosci. 17:1216686. doi: 10.3389/fnins.2023.1216686
Keywords: hippocampal volume, neurodegeneration, air pollution, TRAP, major road, APOE, aging, Alzheimer's disease
Citation: Popov VA, Ukraintseva SV, Duan H, Yashin AI and Arbeev KG (2024) Traffic-related air pollution and APOE4 can synergistically affect hippocampal volume in older women: new findings from UK Biobank. Front. Dement. 3:1402091. doi: 10.3389/frdem.2024.1402091
Received: 16 March 2024; Accepted: 10 July 2024;
Published: 29 July 2024.
Edited by:
Irena Maria Nalepa, Polish Academy of Sciences, PolandReviewed by:
Ciro Gaona, Alzheimer's Foundation of Venezuela, VenezuelaCopyright © 2024 Popov, Ukraintseva, Duan, Yashin and Arbeev. This is an open-access article distributed under the terms of the Creative Commons Attribution License (CC BY). The use, distribution or reproduction in other forums is permitted, provided the original author(s) and the copyright owner(s) are credited and that the original publication in this journal is cited, in accordance with accepted academic practice. No use, distribution or reproduction is permitted which does not comply with these terms.
*Correspondence: Vladimir A. Popov, dnAyM0BkdWtlLmVkdQ==; Svetlana V. Ukraintseva, c3ZvQGR1a2UuZWR1
†These authors have contributed equally to this work and share first authorship
Disclaimer: All claims expressed in this article are solely those of the authors and do not necessarily represent those of their affiliated organizations, or those of the publisher, the editors and the reviewers. Any product that may be evaluated in this article or claim that may be made by its manufacturer is not guaranteed or endorsed by the publisher.
Research integrity at Frontiers
Learn more about the work of our research integrity team to safeguard the quality of each article we publish.