- School of Electronic Engineering and Computer Science, Queen Mary University of London, London, United Kingdom
The United Nations drafted an agenda for 2030 to achieve sustainable development with 17 well-defined goals which are an urgent call for action requiring collaboration and innovation across countries and organisations. The year 2023 marks the midpoint toward fulfilling the proposed agenda but the world is still behind in attaining any of the set goals. This article discusses the opportunities offered by the rise of the Internet of Things and advances in artificial intelligence in ushering these goals with tangible use cases that relate to health, energy, and cities. Moreover, the article exposes the challenges that emerge from applying these technologies in the realisation of sustainable development goals (SDG) with underlined risks related to bias, security, data privacy, and the multi-objective optimisation of often competing SDGs.
1 Introduction
In the latest report issued in 2022 by the United Nations (UN) on the progress made toward sustainable development goals (SDG) an urgent ’code red’ warning was raised in relation to global warming and climate change (United Nations, 2022a). This year, 2023, marks the midpoint between the initial drafting of the UN agenda in 2015 and the targets planned for 2030. The world is well behind in approaching the defined indicators and is struggling to recover from the setback caused by the COVID-19 pandemic. In this aftermath, practical and science-enabled solutions for SDGs are urgently needed and deemed key to accelerating the transformation1.
It follows that innovation in science and technology to usher SDG goals has taken a central role in the research community over the last decade. For instance, the potential of robotics for achieving SDGs is examined in (Mai et al., 2022). Similarly, the role of blockchain disruptive technology in the support of SDG goals is reviewed in (Jiang et al., 2022). Software is found to play a crucial role in enabling SDG technologies whilst itself being at risk of lacking sustainability (Calero et al., 2022). Within the ambit of technology innovation for SDGs, one key area is enabling data/knowledge exchange platforms that support collaboration, joint innovation, and technology transfer between countries/institutions (Zhao et al., 2022).
An overarching key component of these mentioned technologies is the intersection of the Internet of Things (IoT) and artificial intelligence (AI). The IoT is a network of connected physical objects embedded with sensors and actuators that can be used to remotely monitor and apply changes to the physical world through applications. The number of connected IoT devices today exceeds 15 billion2 that cover a plethora of devices and applications. The value of IoT is the promise of anytime-anywhere access to information from the physical world and the ability to take and implement immediate actions accordingly. The promise can be realised when IoT is married to AI, creating Intelligent IoT, with the ability to infer meaning from the collected data and to recommend actionable insights (López-Vargas et al., 2021). Based on the latest study by the UN, 95% of the world population is covered by a mobile-broadband signal including 88% with fast 4G (Fourth generation of mobile networks) (United Nations, 2022a). Such coverage is an essential baseline to allow an almost ubiquitous IoT connectivity (Wu et al., 2018); remaining coverage gaps can be serviced with alternative systems such as non-terrestrial networks (Thangavel et al., 2023) (Feltrin et al., 2021).
Leading works such as (Barakat et al., 2021) offer a comprehensive review of a specific aspect of IoT systems; in this case it is the IoT connectivity with the sixth mobile network generation (6G) as enabling technology for SDG-related use cases including transport, smart grid, and healthcare. Other works explore the role of IoT for a specific SDG target, such as (Salam, 2020), which addresses the green energy paradigm. (Vinuesa et al., 2020). investigate another aspect of IoT systems, that is AI and its applicability for realising SDG targets; the authors find that AI may indeed usher the progress of
Different from these existing studies, this work examines the complete IoT system, including connectivity and AI (see Section 3.1) that is applicable to any SDG. Emerging IoT-based SDG solutions are presented and contrasted with the generic system to underline the possibilities and threats from an SDG-perspective. To this end, we first present a system model founded on the intersection of IoT and AI that is proposed to facilitate SDGs (see full list of the 17 SDGs in Table 1). Next, we present three applications that showcase the opportunities offered by this model in transforming mobility in cities (SDG 9, 11, and 13), responsible energy consumption (SDG 7, 9, and 12), and remote health and wellbeing (SDG 3, 4, and 10). The risks and challenges that stem from this system are discussed next by examining IoT security, data privacy, and the repercussions caused by SDG-driven intelligent IoT such as delaying other SDGs.
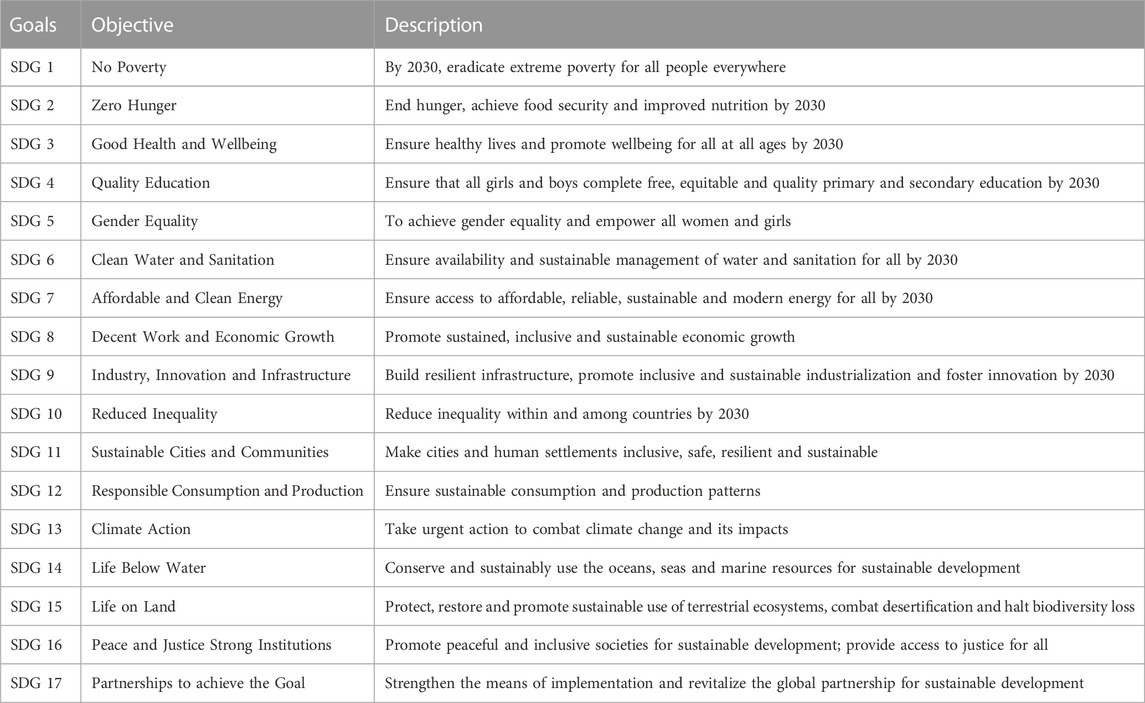
TABLE 1. 17 sustainable development goals defined by the UN in 2015 (United Nations, 2022a).
2 System model of the IoT and ML intersection
An IoT system is composed of IoT devices that connect to the cloud-based servers through gateways, as shown in Figure 1. An IoT system is essentially a network of connected devices that empower the Hyper-Connected Intelligent World (HIW)3. SDG-driven HIW use-cases include applications such as smart agriculture, smart cities, smart homes, and smart health, among many others. These share a similar architecture and connectivity protocols but use differing IoT devices that measure application-specific data to enable particular goal-driven automation. An IoT device is basically a sensor and/or an actuator embedded with a microprocessor and communication hardware that performs data collection from its environment, transmission/reception to/from the server, and that takes action based on the acquired data or instructions received from the server. The IoT gateway acts as a data aggregation point that relays the information acquired by the IoT devices to the server in the cloud for processing. In some HIW applications, the IoT gateway hosts edge intelligence and processes the data locally. Similarly, IoT devices may communicate with each other directly and act on gathered information. IoT devices are often low-cost and lightweight in that they have limited computation power, memory space, and energy source. Such characteristics allow for SDG-driven HIW applications to be affordable and deployable everywhere and anytime and enable full automation with the possibility of human intervention where and when needed. In this section, we examine the different technologies that jointly form the IoT system as shown in Figures 2, 3 (Lv, 2022).
• IoT devices: An IoT device is essentially a connected smart sensor and/or actuator. It follows that these are devices equipped with a processor and the ability for communication (wired and/or wireless). Commonly used IoT devices use sensors for scoping their environment: e.g., temperature, moisture, luminosity, motion, acoustic, chemical, water level, presence, image, gyroscope, and actuators to make a change in their environment. A feature that is common to most IoT devices is that they are designed to be robust and low-cost to enable the widespread and deep reach of SDG-driven HIW applications.
• IoT connectivity: Some IoT devices are connected through wired technology but these are likely to be less than 7% of the 27 billion predicted connected devices in 20254. The rest are wirelessly connected through a plethora of technologies that can be categorised based on their coverage range as follows (Vaezi et al., 2022):
• Short-range (
• Wide-range (
• Cellular (
• Non-terrestrial (
• Security and Privacy: IoT devices are deployed in remote places with often difficult access whilst they carry the pivotal tasks of sensing data and taking responsive action in critical applications such as e-health, autonomous driving, and industrial cases of human-robot co-working. It follows that the data exchanged may carry private information (e.g., biometrics data) and, therefore the security of IoT communication is paramount. IoT systems face various security threats and the IoT device is often seen as the weakest link due to their limited capabilities in terms of computation, storage, and power in addition to their remote and often exposed locations (Alrubayyi et al., 2021a). The IoT communication network faces different threats that include eavesdropping, private data leakage, and false data injection. It follows that, in addition to the coverage range requirements, considerations related to the security of communication play a key role in the selection of the technology for connectivity. Cellular solutions use the licensed frequency bands and incorporate 3GPP security functions including reciprocal authentication of terminal devices and networks, cryptographic algorithms, and a high level of security in creating and exchanging encryption keys owing to the SIM card. In contrast, Wide-range solutions are originally designed to operate SIM-less on unlicensed bands which may compromise the level of security and encryption.
• Data storage: The IoT data torrent demands special attention to the data retrieval process and locations for storage and processing. A three-tier architecture is often invoked in which edge, fog, and cloud are jointly employed to enable an efficient and sustainable solution for IoT data management (Aazam et al., 2022). However, given the range of SDG-driven HIW applications with differing requirements and challenges, there is no one-solution-fits-all for data management. Instead, a context-aware approach is beneficial in which a joint decision for i) the best connectivity, ii) processing unit (e.g., device, fog, and cloud), iii) the percentage of data to be offloaded is optimised for a sustainable operation that prioritises reducing energy consumption, reducing response time, improving, security, and reducing monetary cost depending on the context (Ozturk et al., 2022).
• Machine Learning: SDG-driven HIW systems that require automation rely heavily on machine learning (ML) and AI technologies to infer actionable insights from the gathered data. A plethora of AI approaches has been proposed and employed in conjunction with IoT to enable HIW systems. The selection of the winning technology depends on the application and its requirements. It may prioritise accuracy over speed, energy consumption, explain-ability, data privacy, or reproduce-ability, among many other (Bian et al., 2022).
In this work, we examine three SDG-driven HIW applications: Urban Mobility (see Section 3), Responsible Energy Consumption (see Section 4), and Intelligent IoT for wellbeing (see Section 5). In each use case, we examine the IoT system components with a focus on context-aware ML solutions. Overarching challenges are discussed in Section 6 before concluding in Section 7. These use cases are summarised in Table 2.
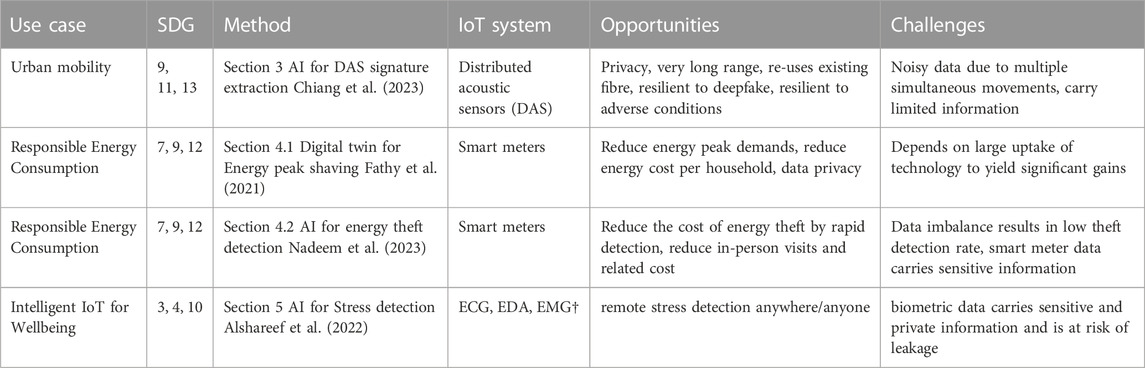
TABLE 2. Use cases discussed in this work and related challenges. †ECG = electrocardiogram, EDA = electrodermal activity, EMG = electromyogram.
3 Use case 1: urban mobility
Cities are the home of more than half of the population and are expected to increase to 70% in 2050. Cities contribute to more than 80% of the global gross domestic product on one hand and 70% of global greenhouse gas (GHG) emissions on the other. It is thus not surprising that 99% of the urban population lives in poor air quality areas as per the guidelines defined by the World Health Organisation (WHO) (United Nations, 2022a). Transportation is a major contributor to GHG emissions; in the UK transportation sector is responsible for 24% of total GHG emissions and more than 50% of these are due to cars and taxis (Department for Transport, 2022). In 37% of global areas, no alternative public transport is available which makes the realisation of the WHO air quality targets impossible without addressing the transportation problem (United Nations, 2022a). Active travel is a promising transportation solution that is defined as any mode of transport that is based on physical activity means and includes a range of modes such as walking, cycling, micro-mobility, and skateboarding. Active travel is a form of exercise that has been shown to improve physical and mental health; hence, reduces the need for medical care and increases happiness and productivity5. A 15-min city is an urban model that allows everyone to meet most of their daily needs within a 15-min journey from their home. Three levels of 15-min city concepts are defined: active travel, public transport, and private transport, as shown in Figure 4. The first strives to achieve the reach of daily needs within a 15-min active travel journey. The second extends the nucleus area to reach further destinations within 15-min journey by public transport. The last level covers a larger area within 15 min of driving in a private car or taxi. Creating 15-min cities that are connected by quality public transport and cycling infrastructure addresses the SDG challenges related to transportation and related emissions. For that, cost-effective investment in the urban transformation that could foster active travel uptake and improve public transport is of pivotal importance to reach 15-min cities and, therefore, to usher SDGs related to Sustainable Cities and Communities.
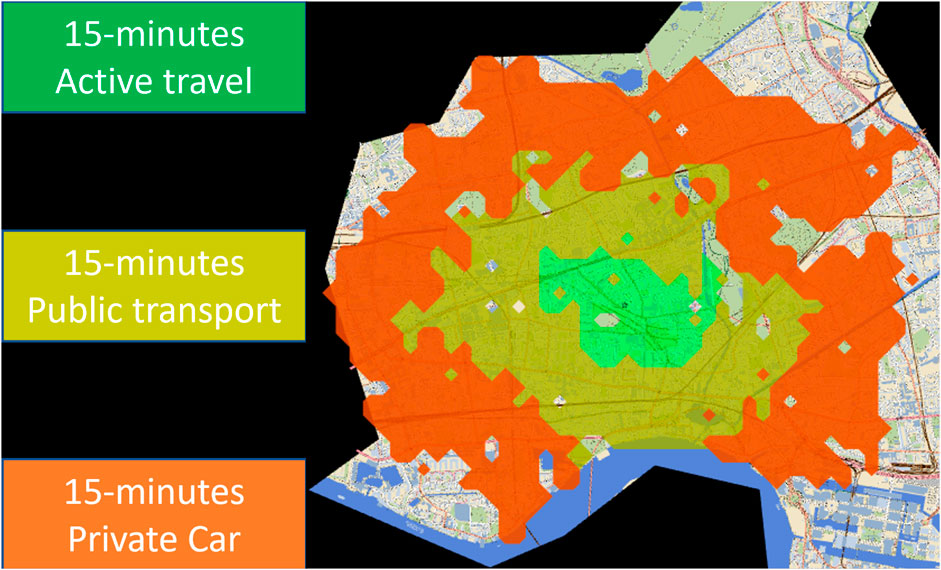
FIGURE 4. Toy example of 15-min city different levels using a-b street simulator6.
3.1 Modelling urban mobility for effective transformation
Identifying a cost-effective investment strategy is not trivial and it is likely to differ depending on the nature of the city and its residents, thus, requires reliable modelling. The impact of surrounding factors on the effectiveness of proposed urban mobility solutions is examined in (Tran et al., 2022) and underlines the interrelation between government policies, economic factors, transport infrastructure, urban planning, and the readiness for the uptake of proposed mobility solutions. IoT systems offer a means to collect and process relevant information from anywhere at any time and, when combined with AI (i.e., Intelligent IoT), could yield accurate modelling of different aspects of the city with high fidelity and in real-time (or right-time). Consequently, there has been a rise in research and field trials that leverage Intelligent IoT to address smart city-related challenges within SDG 11. (Bellini et al., 2022) presents a recent review of important works in this field in which the authors highlight the main trends and open challenges of adopting Intelligent IoT for the development of sustainable and efficient smart cities. In this work, eight different SDG-driven HIW are discussed, including urban mobility. Different types of traffic detectors are commonly used for traffic flow monitoring, such as remote traffic microwave sensors (Jin et al., 2019) and magnetic sensors (Fomin and Braeunig, 2019), in addition to more traditional sensors such as pneumatic road tubes (Liu et al., 2010). However, the data generated by these sensors are mostly limited in information and are suitable only for basic applications such as counting vehicles (see Table 3). A majority of mobility-related HIW solutions are based on data collection from computer vision IoT devices such as cameras. These are very rich in information and can be used for multiple urban mobility applications including optimising traffic (Worrawichaipat et al., 2021), surveillance for safety/security remote monitoring (Shambharkar and Goelcorresponding, 2023), and modelling multi-modal urban mobility (Yasir et al., 2022) (Xiao et al., 2022). Nonetheless, several challenges arise from relying on cameras for such HIW solutions (see Table 3). It is extremely challenging and costly to cover and analyse kilometres of road networks with video cameras and there are inevitable limitations due to blind areas and poor performance in adverse light and weather conditions (Liu et al., 2023). Furthermore, privacy concerns due to omnipresent cameras in urban spaces and related regulations, such as the General Data Protection Regulation (GDPR), have resulted in public reluctance to the spread of video sensors (Al-Turjman et al., 2022). The advantages and drawbacks of different IoT sensors employed in modelling urban mobility are summarised in Table 3. In this section, we present a pioneering line of research that employs distributed acoustic sensor (DAS) systems as an alternative data source for modelling urban mobility that addresses the difficulties faced by cameras (see Figure 5).
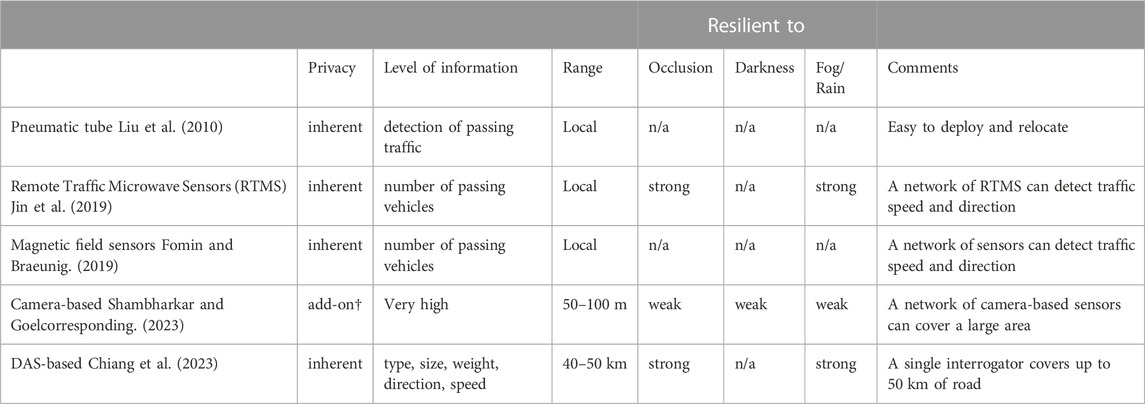
TABLE 3. IoT/sensors for monitoring traffic (n/a: not affected, †additional measures may be added to improve privacy).
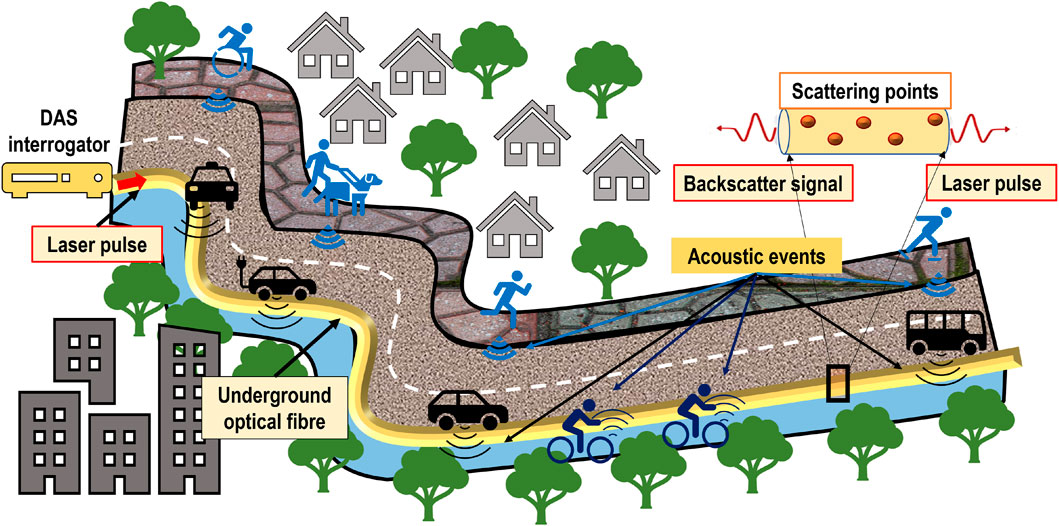
FIGURE 5. Distributed Acoustic Sensor system detecting acoustic events that may be generated by moving objects including vehicles, cyclists, and pedestrians.
3.2 Distributed acoustic sensors (DAS) system
A DAS system is an opto-electronic device sensitive to the strain distributed over an optical fibre of the length of up to 40–50 km (Hartog, 2017). The technology is rooted in Optical Time Domain Reflectometry (OTDR) where a pulse of coherent light (laser pulse in Figure 5) is periodically injected into a fibre and a fraction of the light reflected back via Rayleigh (elastic) scattering mechanism is captured by a photodetector at the launching end (see Figure 5. Each probing pulse results in a continuous time series of back-scatter intensity, commonly referred to as a fibre shot, with the time being proportional to the distance that the pulse has travelled along the fibre. In a DAS system, the intensity of the back-scatter signal (see Figure 5) is a random function of fibre position and the cumulative phase of the interference of light scattered back by the fibre within the interrogating pulse. This fibre interval giving rise to the back-scatter interference is called a resolution cell (see Figure 5). Although the back-scatter phase from each resolution cell is inherently random due to the random molecular structure of the fibreglass, it stays constant as long as the state of fibre within the corresponding resolution cell remains unchanged. If, however, a fibre is subjected to dynamic strain or acoustic event (e.g., passing vehicle or person in Figure 5), it would result in a variation of the back-scatter phase and hence back-scatter intensity. Therefore a series of back-scatter measurements at a given fibre distance carries information about the evolution of the strain applied at the corresponding fibre position.
3.3 DAS for modelling urban mobility
3.3.1 Motivation
As mentioned in Section 3.1, DAS promises to overcome the challenges posed by existing data sources for urban mobility modelling, namely, cameras. For one, it is possible to cover up to 50 km of road with a single DAS interrogator attached to an underground fibre line along the road (Hartog, 2017). It follows that DAS offers an uninterrupted source of data for up to 50 km using a single system. For instance, two DAS interrogators would be sufficient to cover the road connecting London to Oxford (about 100 km) whereas at least 1,000 surveillance cameras would be needed for the same distance (assuming 100 m separation between two consecutive cameras). Moreover, unused optical fibre cables, sometimes referred to as dark fibre, are often purposely laid along lit fibre for future use. These can then be re-used to enable a DAS system and impact positively the incurred cost of deploying a DAS solution.
Cameras and computer vision suffer from adverse weather and illumination conditions and obstructions due to moving objects such as tree branches blown by the wind. In contrast, DAS signals are much less, if at all, affected by these issues, as discussed in (Chiang et al., 2023). Given the nature of the optical fibre line underground, the illumination conditions do not impact the DAS signal at night or during the day. Interruption in the DAS signal may be caused by a fibre splice or a fibre break; however, the locations of these are well known which makes overcoming their impact almost trivial in comparison with unpredictable camera obstructions. As for the weather conditions, the authors in (Chiang et al., 2023) posit that the mitigation of potential impact from rainfall on the quality of the DAS signal is manageable using basic signal processing techniques that can block the periodic signal generated by the rain. However, this concept has not been validated yet and would require more data collection and processing to be confirmed.
HIW solutions driven by camera-based data are vulnerable to malicious deepfake attacks in which the reality is altered to misguide the application (Mitra et al., 2022). Despite research efforts to mitigate the effectiveness of deepfake attacks such as (Güera and Delp, 2018), these remain a threat and result in significant danger in HIW applications related to intelligent transportation systems (may cause fatal accidents), surveillance (may hide unlawful events from surveillance), and modelling of urban mobility. In contrast, a DAS signal representing a given moving object is extremely challenging, if at all possible, to fake. At the European Geosciences Union general assembly in 2023, authors in (Zitt et al., 2023) propose a novel approach for denoising DAS seismic data using synthetic DAS data. Nonetheless, it should be noted that faking a DAS signal to be intentionally mistaken for a specific vehicle or travel mode is a much more daunting problem than faking seismic signals, which leaves DAS-based HIW solutions for urban mobility at no risk of malicious fake attacks until such technology is developed.
As mentioned earlier, camera signals are rich in information and could be used to inform multiple HIW applications simultaneously. In the case of urban mobility, however, camera signals carry more information than what is required for accurate modelling. More importantly, the additional data is often private and personal such as the face of people (Doberstein et al., 2022), the colour of their skin (Heh and Wainwright, 2022) and other (Al-Turjman et al., 2022). Such intrusion into personal information and the risk of data privacy breach represent obstacles in the way of widespread HIW solutions for urban mobility in view of GDPR compliance and people’s acceptance of invasive surveillance. In contrast, DAS signals are intrinsically privacy-preserving as these do not carry personal information and cannot identify an individual in isolation.
For all these reasons, DAS-driven urban mobility is a promising emerging research direction that is cost-effective, uninterrupted, resilient to adverse conditions and deepfake, and privacy-preserving.
3.3.2 DAS for modelling motorised mobility: A pilot study
In the seminal work, (Chiang et al., 2023), present a proof of concept research in which they demonstrate that these DAS signals carry unique information about the moving object, referred to as signature, that allows identifying the type of moving vehicle with reliable accuracy. The authors formulate two different problems based on real DAS data collected in a controlled environment: 1) Identification of specific vehicle type, and 2) Categorisation of the size of a vehicle. A snapshot of the related DAS data is shown in Figure 6 where a fibre shot designates the time component (1 shot represents 1/1,000.4 s) and the fibre bin designates the location of the acoustic event along the fibre (distance between two consecutive bins is equal to 0.68 m). The colour of each pixel indicates the phase intensity of the backscatter signal in radians. In this figure, five vehicles drive in a queue at the same speed from the end of the fibre line towards the DAS interrogator.
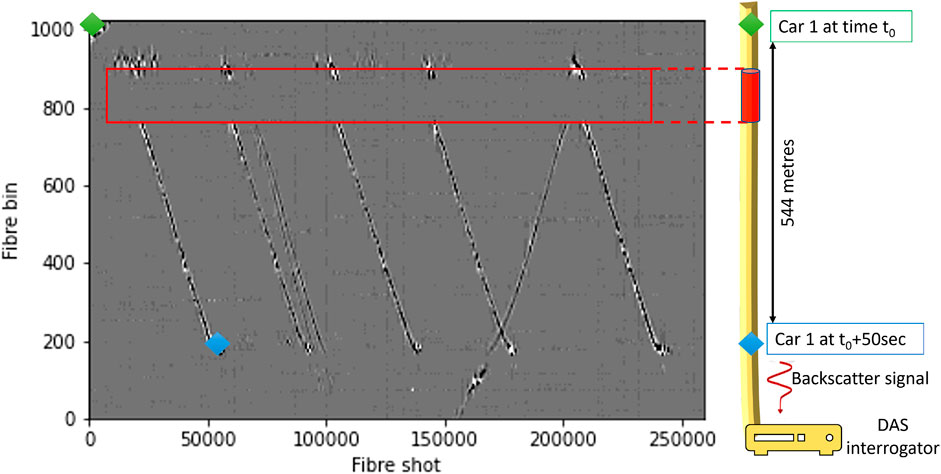
FIGURE 6. Section of DAS data collected over 600 m over 1 min. The five parallel diagonal lines indicate the tracks of 5 cars in a queue moving at a steady speed of 40 km/h. The start and stop points for the first car are shown in green and blue, respectively while it moves from the start point about 600 m away from the DAS interrogator (stop point). The colour intensity at each pixel in the figure indicates the phase of the backscatter signal. The disturbance of phase caused by the moving vehicles carries information about the vehicle. The section of the fibre line highlighted in red fails to detect the acoustic event (the tracks of the cars are invisible within the red rectangle). This may be due to an interruption in the fibre. Two other lines can be seen, these are the tracks of cars external to the experiment, one is seen driving in the same direction whereas the other drives away from the interrogator.
A deep learning approach founded on a one-dimensional convolution neural network (1D-CNN) is proposed to represent the distinctive latent features in DAS signals generated by different moving vehicles; these jointly constitute their unique signature. For the first problem, the authors reach an accuracy between 83% and 92%, depending on the type of vehicle. For the second problem, the accuracy of detecting large (or heavy) vehicles is 89% while that of detecting a small (or light) vehicle is 92%. These are two important problems in HIW applications related to intelligent transportation. The first could apply to tracking and prioritising the flow of specific vehicles (e.g., ambulances or firefighters) anywhere in the monitored area by detecting their signatures in the DAS data. The second could apply to monitoring and controlling areas in the city restricted to small and emergency vehicles. In another work (Chiang et al., 2022), a two-dimensional CNN (2D-CNN) is proposed instead of the 1D-CNN to detect the occupancy of vehicles, knowing the type of vehicle at hand, based on the analysis of the corresponding DAS signal. It is shown that categorising heavy occupancy vehicles (HOV) (more than two passengers) from light occupancy vehicles (LOV) is possible with an accuracy of
3.3.3 Remaining challenges
The results obtained in the two works presented in Section 3.3.2 are evidence that the DAS signal carries unique signatures of moving objects that can be extracted and used to distinguish between two similar events (e.g., two cars of similar size). However, more work is required to examine the applicability of DAS for informing HIW applications in realistic environments with uncontrolled traffic and with different types of roads and optical fibres. Indeed, both works rely on DAS data collected from a controlled environment where traffic was restricted to a single direction at any given time and to five pre-selected vehicles. Moreover, the experiments were scripted which facilitated the labelling of the training dataset (see Figure 6). It follows that, despite the promising results obtained in these two pioneering works, some key questions remain unanswered:
• Applicability of a model for roads and/or optical fibre conditions different from those in the training dataset. Indeed, the physical characteristics of the fibre used, the road type, and the depth of the fibre are known to impact the DAS signal. It follows that, for each deployed DAS system, the model should be re-trained to account for these physical characteristics and would yield a highly reliable method for detecting and tracking vehicles along the roads spanned by the system.
• Accuracy of the model in realistic traffic conditions with uncontrolled vehicle flow. Although the pilot study is based on a controlled experiment with limited unwanted movements, the proposed method is still successful in distinguishing the vehicle types despite external activities such as those created by other cars as shown in Figure 6. This was possible given the well-labeled data based on the a priori knowledge of the wanted car and speed. In the absence of such information, a distinct and uncontaminated DAS signature of a target car would be needed beforehand. It would then be possible to re-identify this signature in the presence of other moving vehicles. Given the length of the DAS system spanning tens of kilometres, this is a realistic assumption to have in an urban environment.
• Expanding the model to represent all vehicles on the road. The dataset studied so far is limited to five vehicle models and thus cannot be readily generalised to distinguish any vehicle model roaming the streets today. Expanding the list of vehicle models in the dataset would likely result in a more representative model but would require massive data collection and costly model training. Some applications that require identifying a specific vehicle, such as airports, manufacturing sites, energy plants, and similar confined environments entail a well-determined list of authorised vehicles. In these cases, the proposed DAS-driven approach would be effective in modelling area-specific mobility.
4 Use case 2: responsible energy consumption
Responsible energy consumption is a pillar for attaining SDG 7: Affordable and Clean Energy. Within the defined SDG 7 targets, Target 7.3 is described as doubling the global rate of improvement in energy efficiency to reach 2.6% by 2030 in comparison with values recorded in 2010. This is measured using the energy indicator Energy intensity (EI) in terms of primary energy and GDP which was 5.6 MJ/USD in 2010 and 4.7 MJ/USD in 2019 (United Nations, 2022b). Although early estimates of EI for 2020 indicate a substantial decrease, this is primarily due to the pandemic. The 2021 levels suggest an improvement of only 1.9%, well below the levels needed. It follows that an accelerated annual improvement of 3.2% is required to achieve the SDG 7.3 target. In 2022, the International Energy Agency drafted a roadmap to reach Net Zero Emissions by 2050; to meet this stringent target an even faster (and possibly disruptive) EI annual improvement of 4% is necessary (International Energy Agency, 2022).
The residential sector is responsible for nearly a third of energy consumption worldwide and is the only sector to witness decreased acceleration between 2010 and 2019 (see Figure ES10 in (United Nations, 2022b)). This drawback is a result of the growing demand for cooling, heating, and appliances in residential buildings. It follows that innovation controlling residential energy demand and consumption plays a pivotal role in improving energy efficiency at scale. In this regard, advances in smart grids as an embodiment of intelligent energy IoT systems offer a means to monitor, control, and regulate residential energy consumption (Zhang T. et al., 2022).
In this section, we present two use cases within the archetype of energy-driven HIW. The first is discussed in Section 4.1 and aims to manage the residential energy demand to flatten the collective demand and avoid peaks that are linked to energy production wastage. The second is described in Section 4.2 and addresses energy theft detection, another direct cause of energy wastage and under-par efficient energy production.
4.1 Digital twin for residential energy demand control
Energy peak demands are a major hindrance to achieving cost-effective energy production (Rana et al., 2022). The surge of smart meters and smart grids offer an unprecedented potential for controlling/nudging energy consumption to effectively flatten the collective demand; otherwise referred to as peak shaving. There are two standard methods for peak shaving. The first relies on storing unused energy during low energy demand periods and tapping into stored energy when more is needed (Grubler et al., 2018). The second method is based on a time-of-use tariff approach designed by energy providers to motivate consumers into changing their habits toward operating their appliances during off-peak hours (Amiri-Pebdani et al., 2022). Time-of-use tariff is a billing mechanism in which the energy cost rate (i.e., cost per Joules) depends effectively on the time of the day that the energy is consumed: the low rate applies during low energy demand and the high rate during peak energy demand periods. Traditional time-of-use pricing designates a priori the time band of each energy cost rate and thus results in under-par energy shaving in view of differing collective energy consumption habits of different residential areas.
A challenge common to both described methods is the need for transmitting frequent and specific energy consumption (EC) data to the server/energy provider in order to enable effective optimisation of extra energy supply and rescheduling of energy demand. Residential EC data holds personal information about the occupants which could reveal their habits and patterns. Transmitting this data over the smart grid exposes such information to unlawful access and could be exploited for timed burglary or targeted marketing. The level of privacy risk that is associated with leaked EC data increases with a higher temporal and spatial resolution of the smart meter data. For instance, minute-based and room-based EC data could reveal the existence of children in the household, inform of their habits, and expose them to abuse (De and Métayer, 2016). In view of these risks, the uptake of smart meters is very slow; in the UK only ∼ 50% of electric meters are smart in 20227 partially due to the unwillingness to accept smart meters (Gosnell and McCoy, 2023).
Another challenge resides in the complexity of the peak shaving problem in view of the underlying multi-objective optimisation and inter-dependencies between the multi-agents involved (Su et al., 2022) (Wynn et al., 2022). It follows that solving optimisation problems related to peak shaving is computationally expensive and requires lengthy processes. As a result, such systems are unable to react timely to manage the collective energy demand on one hand and jeapordise the stability of the smart grid due to false/delayed adjustments on the other hand. To this end, a digital twin approach is adopted in (Fathy et al., 2021) which allows conducting the optimisation in a virtual replica before applying the outcomes to the actual grid. A digital twin is a virtual replica of physical assets that enables data-driven modelling of the status and behaviour of physical components in the system and of the interrelation between these. In this context, a smart meter data-driven digital twin is successfully employed to optimise the formulated complex system of peak shaving and is shown to achieve reliable and cost-effective anticipatory solutions. In (Fathy et al., 2021), a multi-layer digital twin of the energy system aims to mirror households’ actual EC in the form of a household digital twin (HDT). When linked to the energy production digital twin (EDT), HDT empowers the household-centric energy optimisation model to achieve the desired efficiency in energy use. The model intends to improve the efficiency of energy production by flattening the daily energy demand levels. This is done by collaboratively reorganising the EC patterns of residential homes to avoid peak demands whilst accommodating the residents’ needs and reducing their energy costs. Indeed, the proposed system incorporates the first HDT model to gauge the impact of various modifications on the household energy bill and, subsequently, on energy production. A distributed reinforcement learning method is proposed that runs in the virtual replica to optimise the scheduling of the household appliances before applying the end result to the physical assets. The HDT shelters all sensitive data about the household and would only escalate the aggregated information to the central controller within the Energy DT (EDT) as shown in Figure 7. The energy provider EDT comprises the central controller and multiple local transformers. The former interacts with various local transformers to obtain the aggregated energy demand of each area and returns the optimised hourly energy cost rates based on the peak-to-average energy production ratio. EDT and HDT are interlinked and equipped with machine learning algorithms to dynamically optimise the energy supply–demand from both perspectives of providers and consumers. To this end, HDT would optimise the residential energy cost based on the area-specific time-of-use energy rate determined by the EDT.
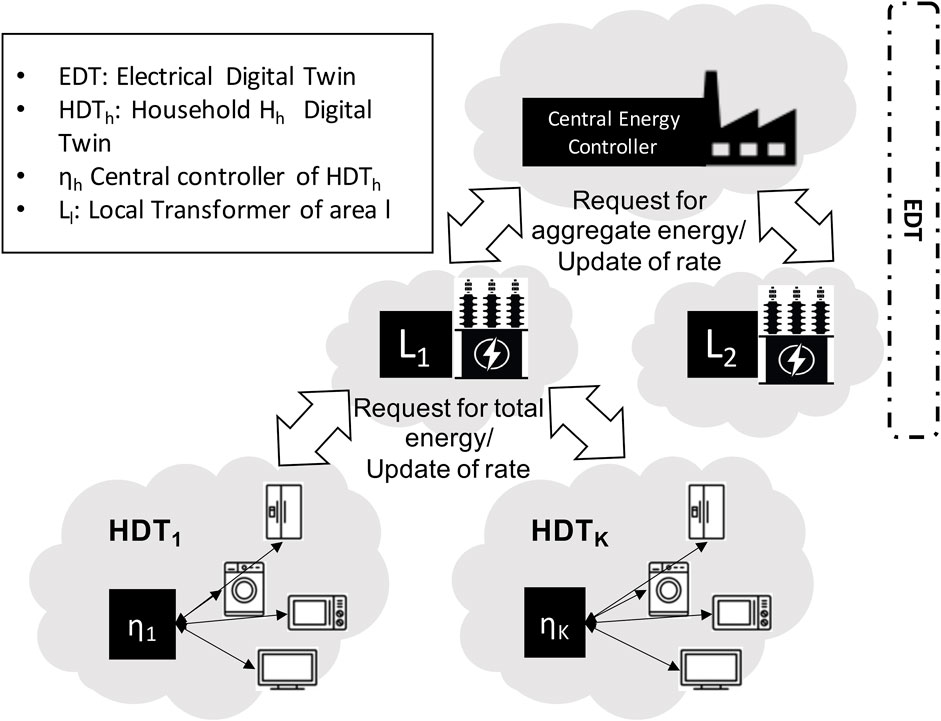
FIGURE 7. Multi-layered Digital Twin (DT) representation of the system model and data exchange. Each house has an EDT that comprises its local transformer connected to a central energy controller. Reproduced from Fathy et al. (2021), licensed under CC-BY-4.0.
The benefits of edge-distributed reinforcement learning techniques are threefold. First, HDT edge computing protects people’s privacy and hence would encourage the adoption among residential customers of such smart energy solutions. Secondly, reinforcement learning is a self-learning method that adjusts to the changing propensities of a household to use electric appliances. For instance, in the case of new tenants, new appliances, or new family members, the algorithm can self-adjust and rapidly yield optimised results. Similarly, the changing energy cost rates that the EDT may define will automatically impact the algorithm and adjust the resulting scheduling to minimise the energy cost for the household. Thirdly, the optimisation takes place in the virtual replica and would only be applied to the physical assets if the results are satisfactory; thus, there is a minimal risk of unstable behaviour or undesired outcome.
The proposed energy system is applied to a real-world IoT dataset that spans over 2 years and covers seventeen households. The conducted experiments show that the model effectively flattened the collective energy demand by 20.4%. At the same time, the average energy cost per household was reduced by 17.7%.
4.2 Energy theft detection
Energy theft exacerbates the energy crisis causing increased market volatility, revenue loss, surging costs, and risks to public safety (such as fires and electric shocks). Energy theft is primarily caused by bypassing or tampering with the electricity meter, direct tapping from feeders, injecting magnetic material to slow down metering and high line losses, and cyber-attacks for altering smart meters. Conventional methods for energy theft detection require conducting physical onsite inspections which are time-consuming, costly, labour-intensive, and prone to human error.
HIW energy applications comprising smart grids and smart meters avail EC data energy providers in near-real time and with very high granularity. EC data carries information about consumers’ trends and energy theft EC data can be seen to have distinct features that delineate it from normal EC as shown in Figure 8.
Authors in (Nadeem et al., 2023) propose a deep learning approach for detecting theft based on incoming EC data. EC data collected from real residential consumers are naturally skewed as the number of honest users outweighs the number of theft (ten to one in (Nadeem et al., 2023)). To this end, the authors define an energy-aware multi-objective metric that accounts for the incurred revenue loss from misclassified EC data. There are two forms of misclassifications: a missed theft detection and an honest sample mistaken for theft. According to the latest published report by Ofgem (Smarter Markets, 2013), energy theft could go unnoticed for 1.4 years and costs more than eight times that of investigating a falsely identified theft. The severe data bias and the competing objectives of detecting theft without causing false accusations led to an energy-aware deep model with weighted loss that outperformed all previous models in reducing the loss caused by energy theft by 30.4%.
5 Use case 3: intelligent IoT for wellbeing
Based on the latest study by the WHO, the global prevalence of anxiety and depression mostly affects young people and women (World Health Organisation, 2023). In 2019, 86 million adolescents aged 15 to 19 and 80 million adolescents aged 10 to 14 were suffering poor mental health (United Nations, 2022a). Following the pandemic, the overall number of anxiety and depression cases increased by an estimated 25% in 2020. Tragically, this increase was met with significant disruption to the services for mental conditions, which widened gaps in mental healthcare. Since 2021, minor improvements have been recorded however many people remain unable to get the care and support they need for both pre-existing and new mental health conditions.
The Global Action Plan for Healthy Lives and Wellbeing for All (SDG3 GAP) is a set of commitments that play significant roles in health, development and humanitarian responses to help countries accelerate progress on the health-related targets. These are championed but different accelerator working groups who collaborate towards meeting these commitments. Among these, the Data and Digital Health accelerator working group has contributed significantly to successes reported in World Health Organisation (2023).
In this context, Intelligent IoT for pervasive mental health support falls within the ambit of the Data and Digital Health efforts and promises to bridge the gap by reaching those that have been failed by existing mental health services. Intelligent stress monitoring assistant is examined in (Alshareef et al., 2022) in which affective computing is employed to detect stress levels based on biological signals. The authors formulate two different problems. The first aims to detect stress in a predefined and well-known group of people; this may be useful for monitoring the wellbeing of a team providing a critical service such as air control or medical staff. The second targets the wider population with the objective of detecting stress in any unseen person; such a model could support understaffed service providers to reach school children and other affected groups effectively. The authors use an open-source dataset and train an attention-based transformer network to extract the stress-specific features with an encouraging accuracy of 96%.
Detecting stress is a problem of critical importance when three-quarters of people in our society feel unable to cope due to stress (Foundation, 2018). Despite the promising benefits of developing Intelligent IoT systems for remote and pervasive stress detection and the dire need for support in view of the rising conditions of mental health, some issues need to be addressed before deploying such solutions. The use of HIW in healthcare raises concerns regarding the security of sensitive patient data, which when transferred and stored could be exposed to unauthorised access and misuse. Furthermore, training a model on sensitive data, such as (Alshareef et al., 2022), involves significant privacy risks. In this work, multiple sensors are employed including electrocardiogram (ECG), electrodermal activity (EDA), and electromyogram (EMG). It follows that the model might potentially memorise or learn certain characteristics of individual patients, allowing for re-identification or inference of sensitive information (Nadas et al., 2020). As a result, it is critical to integrate strong security and privacy measures throughout the development and deployment of machine learning models for healthcare applications.
6 Challenges of meeting multi-objective SDGs
In each of the use cases discussed in Sections 3, 4, and 5, we’ve highlighted the governing challenges that are specific to the application. There are two overarching concerns that affect all SDG-driven HIW applications: 1) Security and data privacy and 2) the interconnectivity and interdependence of different SDGs. Overarching open challenges and promising research directions are listed in Table 4. Data privacy is discussed in the context of each of the use cases and is directly related to security threats that may expose private data (mobility, energy, or health) to unlawful access. Malware attacks target vulnerable IoT devices or gateways (see Figure 1) and may be used to leak personal data and cause a privacy breach. There are multiple methods to combat/detect malware attacks but these are often too complex to run on lightweight IoT devices (Alrubayyi et al., 2021a). An artificial immune system method for malware detection is proposed in (Alrubayyi et al., 2021b) which is highly effective and suitable for Intelligent IoT systems as it has low complexity and the ability to detect unseen malware. However, malware detection does not address the risk of man-in-the-middle, a security breach that targets the communication network for eavesdropping or altering the transmitted data; in this context, private/personal data. There are various methods in the literature for mitigating this risk; these involve avoiding data transmission, protecting transmitted data, or both. The multi-layer digital twin with distributed reinforcement learning in (Fathy et al., 2021) is an example in which the personal data is kept at the edge (or client), thus protecting it from man-in-the-middle attacks. There are various other methods for avoiding private data transmission over the IoT, these involve edge computing and include replacing real data with synthetic data that carries the required latent features, or federated and distributed learning. Given the risk of inferring real data from synthetic data or model parameters, these methods are often combined with data protection methods such as (Issa et al., 2023) which employs blockchain in conjunction with federated learning for intelligent IoT systems. Other methods for data protection against man-in-the-middle attacks include data anonymisation (Yang et al., 2023), differential privacy (Wu et al., 2022), and encryption (Zhang L. et al., 2022).
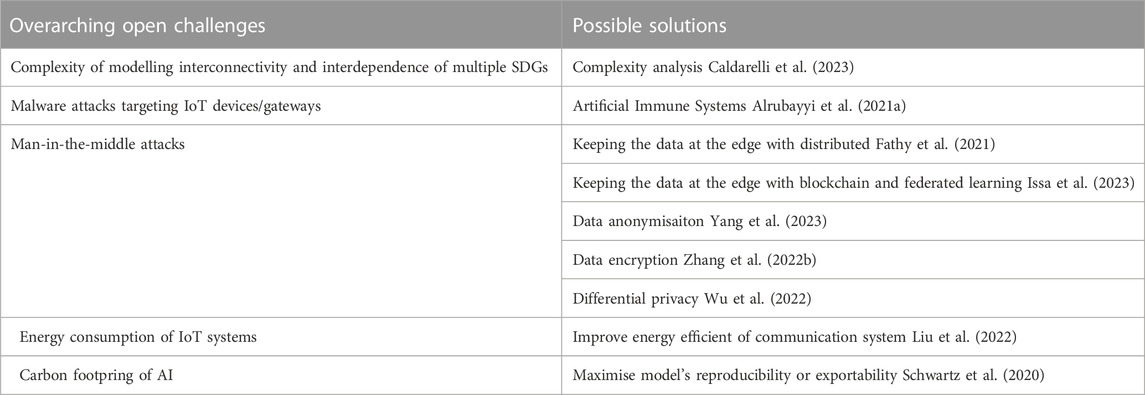
TABLE 4. Overarching challenges delaying the progress of IoT-based solutions for SDG-driven HIW applications.
The other overarching challenge stems from the interconnectivity and interdependency between different SDGs. To this end, (Caldarelli et al., 2023), argue that the theories and methods drawn from complexity science are urgently needed to guide the development and use of digital technologies, digital twins in this case, for sustainable development of cities. The most common pitfall when using intelligent IoT for ushering an SDG such as SDG 11 or SDG 3 is that these incur energy consumption in the process and thus risk delaying SDG 7. For instance provisioning an effective communication system for IoT systems, an essential component in all HIW applications, is a major contributor to energy consumption. Nevertheless, communication provisioning is often geared by energy-unaware objectives such as latency, data rate, and resilience. As a result, recent attention to designing future wireless technologies prioritises energy efficiency. Authors in (Liu et al., 2022), for instance, use reinforcement learning to maximise energy efficiency in the user-beamform selection mechanism in non-orthogonal multiple access systems. Similarly, other works propose a reinforcement learning approach for enabling context-aware connectivity with multi-objectives, including energy efficiency (Ozturk et al., 2022).
AI, which includes reinforcement learning and deep learning, has witnessed great success in multiple areas, not the least in enabling Intelligent IoT. However, this was achieved at a very high price in terms of computation time and related emissions footprint. In their seminal work, (Strubell et al., 2019), demonstrated that training a single model for natural language processing can emit as much carbon as five cars in their lifetimes. A surge of research has followed in which new stringent efficiency requirements are demanded from AI models, in addition to the importance of accuracy measures. Efficiency is measured in terms of model training lead-time and related carbon footprint and the model’s reproducibility or exportability (Schwartz et al., 2020). Such requirements would firstly ensure that the utility of a model is maximised at the minimum energy cost. Moreover, reducing the computational cost of AI directly impacts the monetary cost and renders the technology available to a wider population.
7 Conclusion
This work examines pioneering research that leverages the Internet of Things (IoT) systems and harnesses advances in artificial intelligence to create intelligent IoT systems within the hyper-connected intelligent world to usher in sustainable development goals (SDG). To this end, key components of the IoT system are first discussed. Next, three critical SDGs are studied and emerging related state-of-the-art Intelligent IoT solutions are summarised. The remaining challenges in each of the use cases are exposed in addition to overarching obstacles affecting all SDG-driven Intelligent IoT solutions.
This study demonstrates that Intelligent IoT systems are paramount for any hope of reaching the set SDGs in 2030, specifically following the slow progress achieved since 2015. Despite that, Intelligent IoT systems can be crippled with security and data privacy risks. It follows that mitigating these risks is an essential milestone in paving the way toward effective SDG solutions. Moreover, the interdependencies between different SDGs complicate the design of Intelligent IoT systems. In particular, the connectivity of IoT systems and the carbon footprint of artificial intelligence model training may cause negative repercussions toward the SDG target of responsible energy consumption.
Author contributions
MJ contributed to the conception and design of the study and the writeup of the manuscript. The author confirms being the sole contributor of this work and has approved it for publication.
Funding
Research grant EP/X01262X/1 Project title: Distributed Acoustic Sensor system for Modelling Active Travel DASMATE Funder: Engineering and Physical Sciences Research Council (EPSRC)- UK Research and Innovation (UKRI).
Conflict of interest
The author declares that the research was conducted in the absence of any commercial or financial relationships that could be construed as a potential conflict of interest.
Publisher’s note
All claims expressed in this article are solely those of the authors and do not necessarily represent those of their affiliated organizations, or those of the publisher, the editors and the reviewers. Any product that may be evaluated in this article, or claim that may be made by its manufacturer, is not guaranteed or endorsed by the publisher.
Footnotes
1https://sdgs.un.org/gsdr/gsdr2023
2https://www.statista.com/statistics/1183457/iot-connected-devices-worldwide/
3https://www.forbes.com/sites/bernardmarr/2022/09/26/the-5-biggest-technology-trends-in-2023-everyone-must-get-ready-for-now/
4https://iot-analytics.com/number-connected-iot-devices/
5Department of Health and Social Care, “Physical activity guidelines: infographics,” Sep. 2019.
6https://a-b-street.github.io/docs/software/abstreet.html
7https://assets.publishing.service.gov.uk/government/uploads/system/uploads/attachment_data/file/1099629/Q2_2022_Smart_Meters_Statistics_Report.pdf
References
Aazam, M., Islam, S. U., Lone, S. T., and Abbas, A. (2022). Cloud of things (cot): Cloud-fog-iot task offloading for sustainable internet of things. IEEE Trans. Sustain. Comput.7, 87–98. doi:10.1109/TSUSC.2020.3028615
Al-Turjman, F., Zahmatkesh, H., and Shahroze, R. (2022). An overview of security and privacy in smart cities’ iot communications. Trans. Emerg. Telecommun. Technol.33, e3677. doi:10.1002/ett.3677
Alrubayyi, H., Goteng, G., Jaber, M., and Kelly, J. (2021b). “A novel negative and positive selection algorithm to detect unknown malware in the iot,” in IEEE INFOCOM 2021 - IEEE Conference on Computer Communications Workshops (INFOCOM WKSHPS), Vancouver, BC, Canada (IEEE), 1–6. doi:10.1109/INFOCOMWKSHPS51825.2021.9484483
Alrubayyi, H., Goteng, G., Jaber, M., and Kelly, J. (2021a). Challenges of malware detection in the iot and a review of artificial immune system approaches. J. Sens. Actuator Netw.10, 61. doi:10.3390/jsan10040061
Alshareef, M. S., Alturki, B., and Jaber, M. (2022). “A transformer-based model for effective and exportable iomt-based stress detection,” in GLOBECOM 2022 - 2022 IEEE Global Communications Conference, Rio de Janeiro, Brazil (IEEE), 1158–1163. doi:10.1109/GLOBECOM48099.2022.10001083
Amiri-Pebdani, S., Alinaghian, M., and Safarzadeh, S. (2022). Time-of-use pricing in an energy sustainable supply chain with government interventions: A game theory approach. Energy255, 124380. doi:10.1016/j.energy.2022.124380
Barakat, B., Taha, A., Samson, R., Steponenaite, A., Ansari, S., Langdon, P. M., et al. (2021). 6g opportunities arising from internet of things use cases: A review paper. Future Internet13, 159. doi:10.3390/fi13060159
Bellini, P., Nesi, P., and Pantaleo, G. (2022). Iot-enabled smart cities: A review of concepts, frameworks and key technologies. Appl. Sci.12, 1607. doi:10.3390/app12031607
Bian, J., Arafat, A. A., Xiong, H., Li, J., Li, L., Chen, H., et al. (2022). Machine learning in real-time internet of things (iot) systems: A survey. IEEE Internet Things J.9, 8364–8386. doi:10.1109/JIOT.2022.3161050
Caldarelli, G., Arcaute, E., Barthelemy, M., Batty, M., Gershenson, C., Helbing, D., et al. (2023). The role of complexity for digital twins of cities. Nat. Comput. Sci.3, 374–381. doi:10.1038/s43588-023-00431-4
Calero, C., Ángeles Moraga, M., and García, F. (2022). Software, sustainability, and un sustainable development goals. IT Prof.24, 41–48. doi:10.1109/MITP.2021.3117344
Chiang, C. Y., Jaber, M., Chai, K. K., and Loo, J. (2023). Distributed acoustic sensor systems for vehicle detection and classification. IEEE Access11, 31293–31303. doi:10.1109/ACCESS.2023.3260780
Chiang, C. Y., Jaber, M., and Hayward, P. (2022). A distributed acoustic sensor system for intelligent transportation using deep learning. doi:10.48550/ARXIV.2209.05978
De, S. J., and Métayer, D. L. (2016). “Privacy harm analysis: A case study on smart grids,” in 2016 IEEE Security and Privacy Workshops (SPW), San Jose, CA, USA (IEEE), 58–65. doi:10.1109/SPW.2016.21
Diana, M., and Ceccato, R. (2022). A multimodal perspective in the study of car sharing switching intentions. Transp. Lett.14, 317–323. doi:10.1080/19427867.2019.1707351
Doberstein, C., Charbonneau, É., Morin, G., and Despatie, S. (2022). Measuring the acceptability of facial recognition-enabled work surveillance cameras in the public and private sector. Public Perform. Manag. Rev.45, 198–227. doi:10.1080/15309576.2021.1931374
Fathy, Y., Jaber, M., and Nadeem, Z. (2021). Digital twin-driven decision making and planning for energy consumption. J. Sens. Actuator Netw.10, 37. doi:10.3390/jsan10020037
Feltrin, L., Jaldén, N., Trojer, E., and Wikström, G. (2021). Potential for deep rural broadband coverage with terrestrial and non-terrestrial radio networks. Front. Commun. Netw.2. doi:10.3389/frcmn.2021.691625
Fomin, A., and Braeunig, S. (2019). “A wireless sensor system for traffic flow detection based on measurement of earth’s magnetic field changes,” in 2019 8th Mediterranean Conference on Embedded Computing (MECO), Budva, Montenegro (IEEE), 1–4. doi:10.1109/MECO.2019.8760134
Gosnell, G., and McCoy, D. (2023). Market failures and willingness to accept smart meters: Experimental evidence from the UK. J. Environ. Econ. Manag.118, 102756. doi:10.1016/j.jeem.2022.102756
Grubler, A., Wilson, C., Bento, N., Boza-Kiss, B., Krey, V., McCollum, D. L., et al. (2018). A low energy demand scenario for meeting the 1.5°c target and sustainable development goals without negative emission technologies. Nat. Energy3, 515–527. doi:10.1038/s41560-018-0172-6
Güera, D., and Delp, E. J. (2018). “Deepfake video detection using recurrent neural networks,” in 2018 15th IEEE International Conference on Advanced Video and Signal Based Surveillance (AVSS), Auckland, New Zealand (IEEE), 1–6. doi:10.1109/AVSS.2018.8639163
Hartog, A. (2017). An introduction to distributed optical fibre sensors. Boca Raton, FL: CRC Press. doi:10.1201/9781315119014
Heh, E., and Wainwright, J. (2022). No privacy, no peace: Urban surveillance and the movement for black lives. J. Race, Ethn. City3, 121–141. doi:10.1080/26884674.2022.2061392
Issa, W., Moustafa, N., Turnbull, B., Sohrabi, N., and Tari, Z. (2023). Blockchain-based federated learning for securing internet of things: A comprehensive survey. ACM Comput. Surv.55, 1–43. doi:10.1145/3560816
Jiang, S., Jakobsen, K., Bueie, J., Li, J., and Haro, P. H. (2022). A tertiary review on blockchain and sustainability with focus on sustainable development goals. IEEE Access10, 114975–115006. doi:10.1109/ACCESS.2022.3217683
Jin, Y., Jia, Z., Wang, P., Sun, Z., Wen, K., and Wang, J. (2019). Quantitative assessment on truck-related road risk for the safety control via truck flow estimation of various types. IEEE Access7, 88799–88810. doi:10.1109/ACCESS.2019.2924699
Liu, B., Li, Q., Chen, D., and Sun, H. (2010). “Pattern recognition of vehicle types and reliability analysis of pneumatic tube test data under mixed traffic condition,” in 2010 2nd International Asia Conference on Informatics in Control, Automation and Robotics (CAR 2010), Wuhan, China (IEEE), 44–47. doi:10.1109/CAR.2010.5456723
Liu, R. W., Guo, Y., Lu, Y., Chui, K. T., and Gupta, B. B. (2023). Deep network-enabled haze visibility enhancement for visual iot-driven intelligent transportation systems. IEEE Trans. Industrial Inf.19, 1581–1591. doi:10.1109/TII.2022.3170594
Liu, Y., Zhong, R., and Jaber, M. (2022). “A reinforcement learning approach for energy efficient beamforming in noma systems,” in GLOBECOM 2022 - 2022 IEEE Global Communications Conference, Rio de Janeiro, Brazil (IEEE), 3827–3832. doi:10.1109/GLOBECOM48099.2022.10000828
López-Vargas, A., Ledezma, A., Bott, J., and Sanchis, A. (2021). Iot for global development to achieve the united nations sustainable development goals: The new scenario after the Covid-19 pandemic. IEEE Access9, 124711–124726. doi:10.1109/ACCESS.2021.3109338
Lotze, C., Marszal, P., Schröder, M., and Timme, M. (2022). Dynamic stop pooling for flexible and sustainable ride sharing. New J. Phys.24, 023034. doi:10.1088/1367-2630/ac47c9
Lv, Z. (2022). Practical application of internet of things in the creation of intelligent services and environments. Front. Internet Things1. doi:10.3389/friot.2022.912388
Mai, V., Vanderborght, B., Haidegger, T., Khamis, A., Bhargava, N., Boesl, D. B., et al. (2022). The role of robotics in achieving the united nations sustainable development goals—The experts’ meeting at the 2021 ieee/rsj iros workshop [industry activities]. IEEE Robot. Autom. Mag.29, 92–107. doi:10.1109/MRA.2022.3143409
Mitra, A., Bigioi, D., Mohanty, S. P., Corcoran, P., and Kougianos, E. (2022). Iface 1.1: A proof-of-concept of a facial authentication based digital id for smart cities. IEEE Access10, 71791–71804. doi:10.1109/ACCESS.2022.3187686
Mordor Intelligence (2023). Satellite iot communication market - growth, trends, Covid-19 impact, and forecasts (2023 - 2028). Tech. Rep.
Nadas, J., Jaber, M., van Berghe, S. D., and Imran, M. A. (2020). “Towards continuous subject identification using wearable devices and deep cnns,” in ICC 2020 - 2020 IEEE International Conference on Communications (ICC), Dublin, Ireland (IEEE), 1–6. doi:10.1109/ICC40277.2020.9149260
Nadeem, Z., Aslam, Z., Jaber, M., Qayyum, A., and Qadir, J. (2023). “Energy-aware theft detection based on iot energy consumption data,” in 2023 IEEE 97th Vehicular Technology Conference: (VTC2023-Spring), 1–6.
Ozturk, M., Abubakar, A. I., Rais, R. N. B., Jaber, M., Hussain, S., and Imran, M. A. (2022). Context-aware wireless connectivity and processing unit optimization for iot networks. IEEE Internet Things J.9, 16028–16043. doi:10.1109/JIOT.2022.3152381
Rana, M. M., Atef, M., Sarkar, M. R., Uddin, M., and Shafiullah, G. (2022). A review on peak load shaving in microgrid—potential benefits, challenges, and future trend. Energies15, 2278. doi:10.3390/en15062278
Salam, A. (2020). Internet of Things in sustainable energy systems. Cham: Springer International Publishing, 183–216. doi:10.1007/978-3-030-35291-2_6
Schwartz, R., Dodge, J., Smith, N. A., and Etzioni, O. (2020). Green ai. Commun. ACM63, 54–63. doi:10.1145/3381831
Shambharkar, P. G., and Goelcorresponding, R. (2023). From video summarization to real time video summarization in smart cities and beyond: A survey. Frontier5, 1106776. doi:10.3389/fdata.2022.1106776
Smarter Markets (2013). Smarter markets. Tech. Rep. Available at: http://thznetwork.net/index.php/thz-images (Date last accessed July 15, 2014).
Strubell, E., Ganesh, A., and McCallum, A. (2019). Energy and policy considerations for deep learning in nlp.
Su, H., Feng, D., Zhao, Y., Zhou, Y., Zhou, Q., Fang, C., et al. (2022). Optimization of customer-side battery storage for multiple service provision: Arbitrage, peak shaving, and regulation. IEEE Trans. Industry Appl.58, 2559–2573. doi:10.1109/TIA.2022.3145330
Tavory, S. S., Trop, T., and Shiftan, Y. (2023). Sustainable self-organized ridesharing initiatives as learning opportunities. Transp. Res. Procedia69, 211–216. doi:10.1016/j.trpro.2023.02.164
Thangavel, K., Spiller, D., Sabatini, R., Marzocca, P., and Esposito, M. (2023). Near real-time wildfire management using distributed satellite system. IEEE Geoscience Remote Sens. Lett.20, 1–5. doi:10.1109/LGRS.2022.3229173
Tran, C. N. N., Tat, T. T. H., Tam, V. W. Y., and Tran, D. H. (2022). Factors affecting intelligent transport systems towards a smart city: A critical review. Int. J. Constr. Manag.0, 1982–1998. doi:10.1080/15623599.2022.2029680
Vaezi, M., Azari, A., Khosravirad, S. R., Shirvanimoghaddam, M., Azari, M. M., Chasaki, D., et al. (2022). Cellular, wide-area, and non-terrestrial iot: A survey on 5g advances and the road toward 6g. IEEE Commun. Surv. Tutorials24, 1117–1174. doi:10.1109/COMST.2022.3151028
Vinuesa, R., Azizpour, H., Leite, I., Balaam, M., Dignum, V., Domisch, S., et al. (2020). The role of artificial intelligence in achieving the sustainable development goals. Nat. Commun.11, 233. doi:10.1038/s41467-019-14108-y
World Health Organisation (2023). What worked? What didn’t? What’s next? 2023 progress report on the global action plan for healthy lives and well-being for all. Tech Report (SDG3).
Worrawichaipat, P., Gerding, E., Kaparias, I., and Ramchurn, S. (2021). Resilient intersection management with multi-vehicle collision avoidance. Front. Sustain. Cities3. doi:10.3389/frsc.2021.670454
Wu, J., Guo, S., Huang, H., Liu, W., and Xiang, Y. (2018). Information and communications technologies for sustainable development goals: State-of-the-art, needs and perspectives. IEEE Commun. Surv. Tutorials20, 2389–2406. doi:10.1109/COMST.2018.2812301
Wu, X., Zhang, Y., Shi, M., Li, P., Li, R., and Xiong, N. N. (2022). An adaptive federated learning scheme with differential privacy preserving. Future Gener. Comput. Syst.127, 362–372. doi:10.1016/j.future.2021.09.015
Wynn, S. L. L., Pinthurat, W., and Marungsri, B. (2022). Multi-objective optimization for peak shaving with demand response under renewable generation uncertainty. Energies15, 8989. doi:10.3390/en15238989
Xiao, Y., Chen, S., Miao, S., and Yu, Y. (2022). Exploring the mediating effect of physical activities on built environment and obesity for elderly people: Evidence from shanghai, China. Front. Public Health10, 853292. doi:10.3389/fpubh.2022.853292
Yang, T., Cang, L. S., Iqbal, M., and Almakhles, D. (2023). “Attack risk analysis in data anonymization in internet of things,” in IEEE transactions on computational social systems, 1–8. doi:10.1109/TCSS.2023.3243089
Yasir, A., Hu, X., Ahmad, M., Alvarado, R., Anser, M. K., Işık, C., et al. (2022). Factors affecting electric bike adoption: Seeking an energy-efficient solution for the post-covid era. Front. Energy Res.9. doi:10.3389/fenrg.2021.817107
Zhang, L., Xu, J., Vijayakumar, P., Sharma, P. K., and Ghosh, U. (2022b). “Homomorphic encryption-based privacy-preserving federated learning in iot-enabled healthcare system,” in IEEE transactions on network science and engineering, 1–17. doi:10.1109/TNSE.2022.3185327
Zhang, T., Li, H. Z., and Xie, B. C. (2022a). Have renewables and market-oriented reforms constrained the technical efficiency improvement of China’s electric grid utilities?Energy Econ.114, 106237. doi:10.1016/j.eneco.2022.106237
Zhao, W., Yin, C., Hua, T., Meadows, M. E., Li, Y., Liu, Y., et al. (2022). Achieving the sustainable development goals in the post-pandemic era. Humanit. Soc. Sci. Commun.9, 258. doi:10.1057/s41599-022-01283-5
Keywords: data bias, e-health, IoT, ML, data privacy, SDG, security
Citation: Jaber M (2023) IoT and machine learning for enabling sustainable development goals. Front. Comms. Net 4:1219047. doi: 10.3389/frcmn.2023.1219047
Received: 08 May 2023; Accepted: 13 June 2023;
Published: 03 July 2023.
Edited by:
Muhammad Ali Imran, University of Glasgow, United KingdomReviewed by:
Muhammad Ilyas, Altınbaş University, TürkiyeShuja Shahid Ansari, University of Glasgow, United Kingdom
Copyright © 2023 Jaber. This is an open-access article distributed under the terms of the Creative Commons Attribution License (CC BY). The use, distribution or reproduction in other forums is permitted, provided the original author(s) and the copyright owner(s) are credited and that the original publication in this journal is cited, in accordance with accepted academic practice. No use, distribution or reproduction is permitted which does not comply with these terms.
*Correspondence: Mona Jaber, bS5qYWJlckBxbXVsLmFjLnVr