- 1Department of Information Engineering, Computer Science and Mathematics, University of L’Aquila, L'Aquila, Italy
- 2Department of Industrial and Information Engineering and Economics, University of L’Aquila, L'Aquila, Italy
Smart metasurfaces have recently emerged as a promising paradigm for next-generation wireless communication systems, providing a reconfigurable radio propagation environment for a wide range of services and applications. In this paper, we discuss how to include and exploit smart metasurfaces into a 6G environment employing non-orthogonal multiple access (NOMA) communications. We identify the key challenges in the characterization of NOMA systems assisted by metasurfaces, which encompass the mutual coupling between electromagnetic fields in the scattering surfaces and its impact on the signal-to-interference-plus-noise ratio at the receiver. Furthermore, we describe opportunities and limitations for fully integrated systems: in this frame we envisage and outline a “deep” cross-layer approach, that encompasses full-wave electromagnetic analysis, circuit analysis, signal processing, and communication, then providing a model with affordable computational complexity for solving challenging configuration tasks in 6G wireless scenarios.
1 Introduction and related work
6G standard definition is in its infancy, but the overall scope of the new communication infrastructure is in place Pan et al. (2021). The advent of 5G allowed mobile broadband, time-critical operations, and massive machine-type communications (MTC) to flexibly coexist in the mobile network infrastructure by instantiating network slices to meet specific Quality of Service (QoS) requirements; in 6G no strict separation is envisaged among “verticals” related to enhanced mobile broadband, purely massive MTC and purely ultra-reliable low latency communications (URLLC) in many emerging use cases (including vehicular communications, industrial-IoT, tactile Internet). This calls for research efforts towards development of new schemes and a re-design of medium access and physical layer mechanisms, exploring all the flexibility to cope with heterogeneous and time-varying requirements.
First, the 6G network will be required to support ultra-massive machine-type communication (umMTC) with a device density of up to 108 devices/km2 (versus 106 devices/km2 in 5G) with zero-energy devices having a battery lifetime of 20 years. In the umMTC context, the scarcity of communication resources according to orthogonality paradigms represents a bottleneck for the expected massive channel access Ding et al. (2020a). Therefore, Non-Orthogonal Multiple Access (NOMA) techniques represent an opportunity as they avoid the use of orthogonal physical resources, and intra-technology interference is deliberately allowed. The resulting collisions are resolved by combining channel access coordination and interference cancellation (IC) algorithms Liu et al. (2017). At the same time, diversity techniques and redundancy need to be exploited to provide a controllable environment and achieve the required reliability and latency constraints.
Once NOMA appears as a promising technology for spectrum efficient radio access, and advanced receivers are enabling interference cancellation, the component of the overall radio interface that is not yet flexibly controllable is the radio propagation environment. In this context, smart metasurfaces have recently emerged as a promising new paradigm. (IDTechEX, 2021). observes that smart metasurfaces are a key element that is missing in the 5G infrastructure and that will be instrumental for the success of 6G. The use of mmWave and THz bands will be a further incentive to an extensive adoption of smart metasurfaces to overcome the poor performance of radio waves in terms of blocking probability and propagation impairments (Pan et al., 2021).
The key idea is that metasurfaces can be installed on large flat surfaces to reflect radio frequency (RF) energy around obstacles and create a virtual line-of-sight (v-LoS) propagation path between a source and the destination. By deploying metasurfaces densely in a wireless network and coordinating their reflections, the signal propagation/wireless channels between transmitters and receivers can be flexibly configured and adapted, thus enabling an innovative approach that fundamentally exploits wireless channel fading impairment and interference issues, achieving a significant improvement in terms of reliability and system capacity Liu et al. (2021). Finally, metasurfaces can also be utilized to support sensing, localization, and wireless power transfer Di Renzo et al. (2020b). Obviously, one of the most difficult tasks for designers will be to make existing communication infrastructure and propagation environment coexist with the introduction of metasurfaces.
Several studies on metasurface-aided wireless systems have appeared in the recent literature. A detailed tutorial on smart metasurfaces as a new paradigm for future-generation wireless networks is provided by Wu and Zhang (2020). The paper examines the technology and key technical challenges for its implementation. In Basar et al. (2019), the authors summarize metasurface-enabled wireless networks, exploring theoretical performance limits of reconfigurable intelligent metasurface-assisted communication systems using mathematical techniques. Similarly, the work by Di Renzo et al. (2020b) provides a comprehensive overview of applications, benefits, primary concepts, and future research prospects, as well as reflecting design issues on smart metasurfaces, specifically channel estimation and resource allocation. In Björnson et al. (2020), the key principles around metasurfaces are presented and three specific misconceptions about their functionality and performance gains are discussed. Challenges from a signal processing perspective are highlighted in (Bjornson et al., 2022). In Di Renzo et al. (2020a), a comparison of metasurfaces and relays is done using communication performance measures.
The idea of using metasurfaces together with NOMA systems from a communication and information theory point of view is recent. The work by Sun and Jing (2022) investigates metasurface-assisted NOMA downlink transmissions with both the ideal and non-ideal settings, focusing on a special case where the direct link between the base station and one of the users is blocked, while a second unblocked user is paired with the blocked user for NOMA reception. In Tahir et al. (2021), the uplink outage performance of intelligent reflecting surface-assisted non-orthogonal multiple access (NOMA) is investigated. The authors consider the general case where all users have both direct and reflection links, and all links undergo Nakagami-m fading. The results show that under certain conditions, the presence of a metasurface might degrade the performance of users that have dominant line-of-sight (LOS) link to the base station. The role of metasurfaces is seen as an additional degree of freedom to characterize the effective channel gain between the transmitter and the receiver Ding and Vincent Poor (2020).
The analysis and optimization of metasurface-aided wireless systems call for sufficiently realistic and accurate, but also tractable, models that account for the electromagnetic (EM) properties of the scattering elements of the metasurface. To date, only a few EM-compliant models for metasurface-aided wireless systems are available (Abeywickrama et al., 2020; Williams et al., 2020; Danufane et al., 2021; Tang et al., 2021;). Although noteworthy, these works take the impact of the EM fields and of the currents impressed and induced on the radiating elements into account only approximately. A more systematic approach is proposed in (Gradoni and Di Renzo, 2021) where the Method of Moments (MoM) is adopted to model the radiating elements (antennas, passive scatterers) in the frequency domain. The use of MoM in the frequency domain has some drawbacks, so a time-domain analysis may be preferable in some case, as we will highlight in Section 4.
In this paper, we investigate the integration of NOMA systems with metasurfaces, to give insights for a unified analytical framework, i.e., a deep cross-layer approach that accounts for multiple access to the shared radio resource, channel propagation, mutual coupling in meta-surfaces, and interference cancellation at the receiver. Moving forward from the recent literature, which considers either communication aspects of NOMA or circuital aspects of metasurfaces (Table 1), the key aspect of a deep cross-layer approach is the integration of EM characteristics and communication model. As the 6G vision is still being developed by the mobile communications industry, these insights are relevant to understand what level of abstraction from the EM and circuital analysis is needed for designers of novel communication protocols, with a comprehensive yet computationally affordable analytical description. In particular, this is relevant as a preliminary step for the definition of the key features before applying optimization techniques based on e.g., machine learning, towards the definition of intelligent and self-configuring 6G wireless systems, as envisioned in Yang et al. (2021).
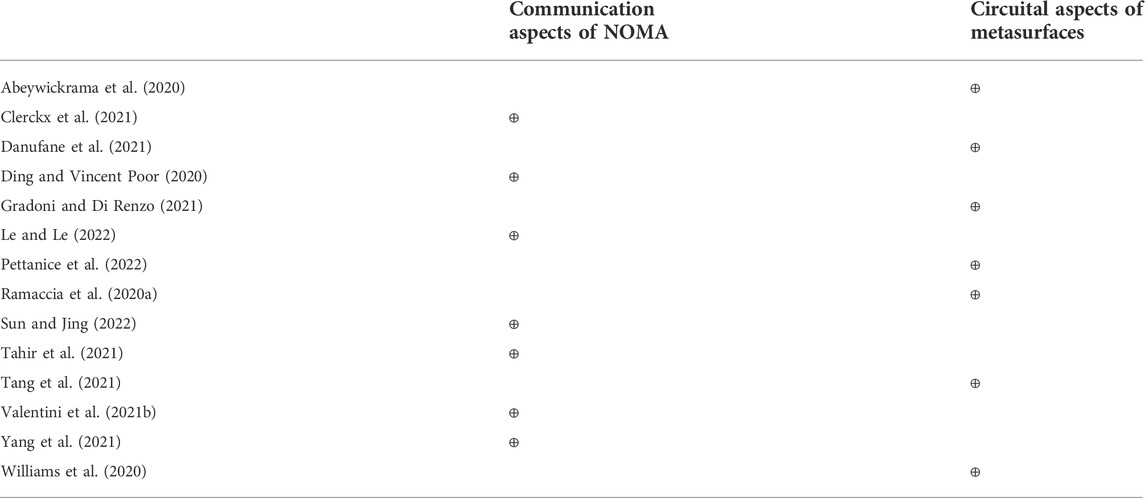
TABLE 1. Recent related literature on NOMA systems and smart metasurfaces. The works considers either communication aspects of NOMA or circuital aspects of metasurfaces.
The outline of the paper is as follows. In Section 2, we describe smart metasurfaces and potential application scenarios. Section 3 illustrates NOMA systems. Section 4 focuses on the characterization of the EM characteristics of smart metasurfaces. Section 5 discusses guidelines and challenges in analyzing in an integrated way, NOMA systems with smart metasurfaces; furthermore, the envisaged deep cross-layer approach is presented and analytically described. Then, Section 6 concludes the paper.
2 An application scenario within 6G
Ubiquitous connectivity among vehicles, infrastructure and with other road users is crucial to increase the safety of automated vehicles and their full integration into the overall transport system. In this framework, the concept of Cooperative Intelligent Transport Systems (C-ITSs) is one of the main application scenarios for future generation wireless technologies, where NOMA and metasurfaces help road users and traffic operators to share information and to coordinate their actions.
As a key implementation scenario, smart metasurfaces can be deployed in urban environments to improve the coverage by creating additional paths to favor a better connection between the users and the communication infrastructure. By operating directly at the electromagnetic level, metasurfaces process signals at the speed of light, which gives an extremely reduced power consumption and latency with respect to, e.g., a relaying solution.
Initially, smart metasurfaces have been conceived for indoor environments. In order to reduce the undesirable effects due to multiple paths, the surfaces can be applied to furniture, paintings, walls, or floors and whatever else may be revealed useful for the optimal propagation of the signal being able to exploit the advantages related to reduced weight and variable product geometry. However, smart metasurfaces have a rich set of outdoor applications. They can be applied on buildings, on the road surface or on the body of moving vehicles. A pervasive implementation of metasurface technology in future 6G scenarios inspires the visionary goal to transform building, walls, surfaces of objects, mirrors, or appliances in reconfigurable reflectors capable of re-directing wireless signals and enhance wireless connectivity.
The possibility to dynamically configure the wireless propagation environment via the elements present in the environment itself opens incredible potential to serve users with high capacity and high energy efficiency. The use of metasurfaces, in conjunction with other resources (sensors, cameras, wearable intelligent devices), could play an active role in providing concrete support for the removal of communication limits dictated by obstacles, low data rates, error rates, poor coverage and latency in an urban scenario. Besides, the technology can be exploited to provide better localization accuracy and data privacy protection when compared to existing wireless communication systems.
As an illustrative example, Figure 1 shows how metasurfaces can support connection of two users with a 6G base station in the presence of an architectural barrier. The smart metasurface is provided with a controller that takes input from the base station to dynamically adapt the reflecting properties of metasurface elements so that users that are not in line of sight (LoS) can be considered in “virtual” line of sight (v-LoS).
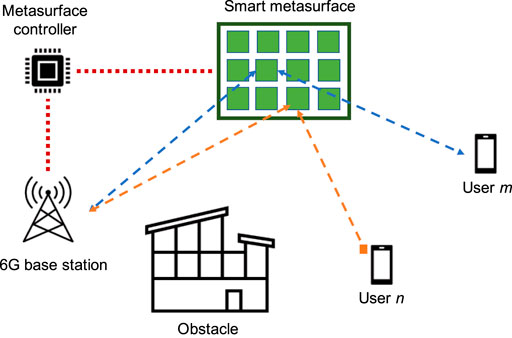
FIGURE 1. Smart metasurfaces application to an urban scenario, where the users that are not in line of sight (LoS) can be considered in “virtual” line of sight (v-LoS) through a proper configuration of the reflecting elements of the metasurface.
3 Non-orthogonal multiple access systems with smart metasurfaces
A Non-orthogonal multiple access (NOMA) system is based on a multiple access technique that allows multiple users to simultaneously use the same time-and-frequency resource, abandoning the principle of orthogonality of time scheduled or frequency scheduled communications. For massive multiple access scenarios, orthogonal multiple access (OMA) requires resource-intensive scheduling strategies. Nevertheless, excessive multiple access interference is a natural result of the NOMA approach. In order to deal with the excessive interference, users allotted to the same resource have to be properly multiplexed to guarantee acceptable communication reliability Ding et al. (2020a). Based on the multiplexing strategy, we can distinguish two categories, namely power-domain NOMA and code-domain NOMA.
In power-domain NOMA, signals from multiple users are discriminated by the power level, using then advanced techniques such as successive interference cancellation (SIC) at receivers for interference cancellation. Code-domain NOMA relies on optimized design of channel codes, interleavers, and codebooks/sequences to separate multiple users, moving forward from classic code-division multiple access (CDMA) techniques to include sequences or codebooks that exhibit certain sparsity (e.g., sparse code multiple access (SCMA) Moltafet et al. (2018)). For the purpose of smart metasurface integration, we believe that power-domain NOMA is more interesting due to the possibility to control the received power levels at receivers not only from the transmitting side, but also by opportunely tuning the parameters of the smart metasurface. Fort this reason, we restrict the analysis to power-domain NOMA scenarios in the following.
3.1 Uplink vs. downlink power-domain non-orthogonal multiple acces
As a first step, it is important to characterize the different implementations of NOMA for downlink and uplink communication. Downlink NOMA transmission employs the superposition coding (SC) technique at the base station. Basically, the base station transmits to multiple users, by encoding a message for a user associated with poor channel conditions at a lower rate and power and then superimpose the signal of a user having better channel conditions on top of it. SC introduces interference, which needs to be removed by each user before decoding its own message. As such, an efficient multi-user detection technique is required to suppress interference in order to fully enjoy the benefits of SC. The SIC technique is then invoked by the users for interference cancellation, as shown in Figure 2. More precisely, the user with better channel condition, usually referred to as near user, directly decode its message by treating interference as additional noise. On the other hand, the user with worst channel conditions, referred to as far user, estimates the signal associated to the near user, then subtract the estimated signal from the superimposed received signal, and finally proceed to the detection of its intended message.
In uplink NOMA transmission, multiple users transmit their own uplink signals to the base station in the same resource, as shown in Figure 3. The base station detects all the messages of the users with the aid of SIC. This marks a first difference between downlink and uplink NOMA. The base station in downlink NOMA chooses different transmit power to help SIC at the receivers. Uplink NOMA relies on the channel conditions of each user. If the users’ channel conditions are significantly different, their received SINR can be rather different at the base station, regardless of their transmit power.
The SIC operations and interference experienced by the users in the uplink NOMA and downlink NOMA are also different. More specifically, for downlink NOMA, the strongest user decodes its message and then interference cancellation is performed by the weaker users in downlink, decontaminating the interference imposed by the strongest user. By contrast, in uplink NOMA, SIC is carried out at the base station based on the stronger-to-weaker decoding ordering scheme Ding et al. (2020a). The base station tries to detect the strongest user first by treating the other users as interference. Then, the base station re-modulates the recovered signal and subtracts the interference imposed by the strong user to detect the weaker user. The procedure is re-iterated until all the colliding packets are decoded.
The performance gain of NOMA over OMA is therefore different for downlink and uplink. The capacity region of NOMA and OMA both for downlink and uplink are illustrated in Shin et al. (2017). The capacity region of NOMA is outside OMA, which means that the use of NOMA in downlink has superior performance in terms of throughput. While in uplink, NOMA mainly has the advantages in terms of fairness, especially compared to the OMA with power control, Wei et al. (2020).
3.2 Limitations and opportunities
NOMA communication paradigm has been initially proposed as a technique for 5G. However, besides an increasing interest towards the technology, 5G standardization has not integrated NOMA solutions, Maraqa et al. (2020). The reason is mainly due to the additional complexity of the receiver, that has to perform successive interference cancellation, and issues when integrating the technology in multi-antenna multi-cell systems.
A relevant limitation to the use of NOMA is dictated by the SIC detection that in practical implementations is affected by error propagation through the detection stages. Therefore, imperfect SIC needs to be explicitly accounted when designing NOMA systems. Moreover, SIC requires channel state information (CSI) to correctly perform decoding, thus performance may also be affected by channel estimation errors, Le and Le (2022).
Another performance affecting factor for power-domain NOMA systems is the loss of symbol-level synchronization that may occur due to different propagation delays, timing errors and drifts of nodes’ local oscillator, which may affect SIC, especially in uplink NOMA scenarios. Nevertheless, recent studies have unveiled the possibility of constructively exploiting/inducing lack of synchronization to improve the rate of uplink NOMA systems, Liu et al. (2019); Li et al. (2021). In order to improve SIC efficiency and enable practical NOMA schemes, the number of interfering users in shared resources should be kept limited. To this aim, channel access coordination and pairing schemes for interfering/served users are typically used to reduce occurrence of multiple-access interference, thus maximizing the SIC efficiency, Guo et al. (2018), Guo et al. (2019).
Compared to the classic multi-user detection techniques Lee et al. (2011), power-domain NOMA usually has a single observation at each receiver. In other words, rather than using joint detection-aided multi-user detection considering all users simultaneously, the SIC technique operates iteratively, which imposes a lower hardware complexity at the receiver than the joint decoding approach.
In Clerckx et al. (2021), theoretical considerations and simulations suggest that there may be inefficiencies in applying NOMA with classic multiple antenna systems, and other multiplexing techniques such as multi-user linear precoding and rate splitting may provide higher achievable system throughput. As an alternative, a re-design of the detection ordering strategy may enable the convergence of multiple antenna systems and NOMA as proposed in Ding et al. (2020b), where a hybrid SIC scheme with adaptive decoding order is shown to provide higher flexibility and can in principle overcome the limitations deriving from multiple antenna settings.
The role of a smart metasurface as a mean to improve channel access coordination, shape coverage for multi-cell environment, and provide an alternative solution to classic multi-antenna systems can be then instrumental to overcome the current limitations of NOMA. For instance, metasurfaces can support the users’ pairing strategy and can be exploited to improve the users’ multiplexing in the power domain. Contrary to multi-antenna systems that are widely modeled and integrated in multiple access systems, smart metasurfaces exhibit different characteristics from an EM and circuital point of view that need to be accounted. A preliminary characterization of smart metasurface from an EM and circuital point of view is provided in the following.
4 Characterizing smart metasurfaces
A smart metasurface is a thin sheet of electromagnetic material made of many passive scattering elements that can be arranged to customize the propagation of the radio waves, depending on the input they receive from the external world. It should be noted that smart metasurfaces are given several names that are used interchangeably in the literature. They are for example referred to as, e.g., reconfigurable intelligent surfaces (RIS) and intelligent reflecting surfaces (IRS). Each of these terminologies emphasizes a different aspect of the metasurface, as it is well summarized in Zhao (2019).
Reflection of the incident EM waves in a specific direction is the main usage in communication envisioned for smart metasurfaces. However, by configuring the circuital characteristics of a metasurfaces, their element can interact with the EM wave and generate the following additional phenomena:
• Refraction: the wave that refracts from the surface is transmitted towards a specific direction, not necessarily the same as that of arrival.
• Focus: the energy of the wave is concentrated at a specific point.
• Collimation: generates the complementary phenomenon of focusing.
• Splitting: the incident wave will generate multiple reflected or refracted waves.
• Absorption: the reflected or refracted wave will undergo variations in terms of power.
• Polarization: the wave is modified in terms of the orientation of the EM field.
NTT Docomo has made a special transparent glass called smart glass, which allows diffusing a signal inside a building thanks to the refraction properties of the surface Kitayama et al. (2021). This smart glass is composed of stacked layers of metasurface with elements (meta atoms) periodically arranged at a distance from each other less than one wavelength, with dynamic control of the scattered waves over a large area in the 28-GHz band. Therefore, this enables to control the system response dynamically in three different ways: 1) full penetration, 2) partial reflection, and 3) complete reflection of incident waves. Glass does not alter the aesthetic aspect as it is transparent and through the selective blocking of radio waves will ensure high efficiency and applicability in areas where security is also concerned.
4.1 Possible realizations of smart metasurfaces
A detailed model of metasurfaces is instrumental to determine the suitable modeling abstraction for the integration in the proposed application scenario. First, metasurfaces can be categorized as passive and quasi-passive. In the first case, their properties are influenced by external factors, e.g., an impinging electrical field; in the second case, the properties of the surfaces are driven by a small voltage signal Vbias.
4.1.1 Power dependent metasurface
4.1.1.1 Strip arrays
Figure 4 shows an example of a passive metasurface that consists of an array of sub-wavelength metallic strips Ramaccia et al. (2020a). The strips are set perpendicular to the electric field of the impinging wave, and the nonlinear circuit in the gaps between two consecutive strips makes the response of the structure sensitive to the impinging power. The nonlinear circuit consists of a diode pair, therefore, when the induced voltage does not overcome the activation threshold, the metasurface exhibits its conventional capacitive behavior. Conversely, when the impinging power is high enough to turn the diodes on, the strips are short-circuited and the metasurface behaves as a perfect electric conductor.
Properly combining this power-dependent behavior, it is possible to design antenna systems with well-shaped radiation pattern by acting on the antenna input power.
4.1.1.2 Lattice of metal plates
The second type of metasurface is illustrated in Figure 5. The structure consists of a lattice of metal plates connected by horizontal diodes and a ground plane Sievenpiper (2011). The diodes are arranged so that an incoming wave will charge alternate plates to a positive or negative DC voltage. Each plate is connected to the ground by a parallel capacitor and resistor; the first one is for energy storage when the surface is hit by a high-power pulse and the second dissipates the energy between pulses.
This type of surface can be used to have an effective absorber of high-power RF surface currents, and the key to this behavior is due to the presence of the parallel capacitor and resistor vertical circuit.
4.1.1.3 Wave-form selective metasurface
The last example among the passive metasurfaces is the wave-form selective metasurface Ramaccia et al. (2020a). In Figure 6, the structure of the unit cell is reported; it consists of a meander-like inductive strip and is characterized by a surface impedance ZS that strongly depends on the meander deep. By exploiting a properly designed waveform-selective circuit, it is possible to change the metasurface ZS. The loading circuit is able to switch from an open to a short circuit condition (and vice versa) depending on the waveform of the impinging wave, and this unconventional behavior has been exploited to design a wire antenna able to automatically hide or reveal its presence depending on the waveform of the received/transmitted signal.
In Monti et al. (2016), an application of two interfering antennas, a huge and a small antenna, placed next to each other is illustrated. It is clear that the big antenna affects the small antenna’s radiation pattern, but if meander-like inductive strips are located on the big antenna, this one can become invisible to the little antenna, with a radiation pattern similar to the case of a single antenna in an empty space.
4.1.2 Time-varying reflective metasurface
Time-varying metasurfaces are driven by a small voltage signal Vbias, so they are considered quasi-passive surfaces. The cost of additional small voltage signals is paid back by a greater degree of freedom in the configuration of the metasurface. Figure 7 shows an example of time-varying reflective metasurface from Ramaccia et al. (2020a) and Ramaccia et al. (2020b).
The metasurface can be thought of as a set of unit cells, and each cell consists of four mirrored mushrooms. Each mushroom has five layers: the first and the third are two metallic copper patches connected by a via, the second is a dielectric substrate with permittivity ɛr = 4.3 and dissipation factor tan δ = 0.025 at f = 10 GHz, the fourth is a thin foam sheet and the fifth is a metallic ground plane that extends over the entire width of the metasurface enforcing the zero transmission. The four patches of the unit cell are electrically connected to each other in pairs through a biasing line, which runs at 45° with respect to the unit-cell main axes, in order to realize a chessboard electric potential. The metasurface has to exhibit the following time-domain complex reflection coefficient Γ(t) = ej(δft), where δf is the frequency shift imparted by the modulation. The time-modulation allows realizing a desired frequency shift of the reflected wave with respect to the incident one.
The modulation of the reflection coefficient is achieved by modulating in time a standard high impedance surface, which exhibits a unitary reflection and tunable phase of the reflection coefficient from − π to π.
4.2 Configuration of the metasurfaces
Configuration of smart metasurfaces is a key aspect to consider. Typically, as illustrated in Figure 1, an external controller is needed to dynamically reconfigure the propagation of radio waves based on current network dynamics. Additionally, due to the absence of signal processing units on such nearly-passive metasurfaces, channel estimation and acquisition are usually performed over the entire path from transmitter to the receiver, which may prevent an agile deployment of the system. In Albanese et al. (2022), the authors presented a self-configuring metasurfaces absorption and reflection solution, based only on CSI that can be locally estimated at the metasurface, which has power-sensing capabilities and does not require an active control channel.
A key innovative aspect is the possibility to use learning mechanism to support the configuration of the metasurfaces. Convolutional neural networks at the metasurface controller have been applied to estimate interfering devices from the incident signals at the metasurfaces Yang et al. (2021) and learning models have been applied to the problems of metasurface elements allocation and phase shifts configuration Cao et al. (2021). However, before applying optimization techniques and autonomous configuration algorithms, it is instrumental to derive the key electromagnetic features of the metasurfaces that affect the communication performance of the system.
5 A deep cross-layer approach for metasurface-aided non-orthogonal multiple access systems
The electromagnetic characterization of the metasurface plays a key role in understanding the achievable performance of practical implementations. As an example, the dielectric properties and the absorption dictated by the implementation aspect of metasurfaces introduce latencies that need to be opportunely considered when modeling the combined signals at the receiver. At the same time, by tuning the phase shifts of the metasurface, the signals reradiated from the metasurface add constructively or destructively with the signals from other paths and users, and channel estimation plays a key role in the performance of the receiver, thus requiring a signal processing view on the problem.
Most of the existing works have considered quasi passive designs and channel estimation schemes in systems with a single metasurface. In some scenarios, however, it may be convenient to enable the transmission of signals through reflections from multiple surfaces to route the signals and bypass the blocking objects in a smart manner. Smart metasurfaces in NOMA scenarios call for a system-level analysis of the end-to-end performance. Therefore, the study of metasurfaces-assisted NOMA systems requires a methodological approach that encompasses full-wave electromagnetic analysis, circuit analysis, signal processing, and communication, providing an abstract model that captures the complex relation among the main components of the system.
The proposed approach for the analysis of metasurface-aided NOMA systems is therefore denoted as deep cross-layer. The idea is to extend the classic cross-layer approach, where the interactions among channel access strategies, physical layer, and channel propagation characteristics are considered, to include the electromagnetic characterization of the circuital elements of the system.
An illustrative representation of the proposed approach and the deep cross-layer interactions is presented in Figure 8. The NOMA scheduler takes as an input the system model including the number and users and their estimated relative distances to allocate transmitter/receiver pairs. At the circuital layer, the partial element equivalent circuit (PEEC) method Ruehli et al. (2017) is used to compute the impulse response of the system in terms of s-parameters choosing as inputs one of the circuital model of the metasurfaces described in Section 4. Then, a convolution solver is used, taking as the input the impulse response of the system, the selected transmitter/receiver terminations, and the configuration parameters of the metasurface. The convolution solver produces output port signals accounting for the mutual coupling among the radiating elements of the metasurface. These output signals are fed into a block that calculates the signal-to-interference-plus-noise ratio (SINR) to describe the impact of multiple access interference, taking as an input also the channel model that includes the propagation characteristics. Then SIC is applied at the receiver to determine the overall performance metrics of the system in terms of e.g., successful reception probability or system capacity. The performance metrics can be optionally used as inputs to optimization algorithms for the NOMA scheduler or for the metasurface configuration controller, as illustrated by dotted arrows in Figure 8.
5.1 Time vs. frequency analysis for the electromagnetic characterization
To date, electromagnetic characterization of metasurface-aided systems is done using the frequency-domain approach based on the Method of Moments (Gradoni and Di Renzo, 2021). The use of the Method of Moments in the frequency domain has two main drawbacks:
• it may lead to large linear systems of equations to be solved over the frequency range of interest.
• it prevents to incorporate directly non-linear circuits to reconfigure the metasurface or connected to the receiver.
In our scenario, we suggest to focus on a time-domain approach, which has some promising beneficial aspects:
• nonlinear terminations can be directly included in the analysis;
• a single run allows characterizing the channel for assigned antenna terminations, thus avoiding the repeated runs over the frequency range of interest.
Time-domain solvers for Maxwell’s equations are available but, however, the metasurface context poses major challenges:
• Transmitters, metasurface elements and receivers are far apart making almost impossible the use of differential methods like finite-difference time-domain (FDTD) or time-domain finite-element method (TDFEM).
• Transmitters, metasurface elements and receivers can be driven or loaded by complex circuits that often exhibit non linearities.
• The EM modeling must be compliant with the time-varying scenario due to the time-varying relative positions of transmitters, metasurface elements and receivers.
These three challenges require the adoption of time-domain integral equation-based methods. For this purpose, the partial element equivalent circuit (PEEC) method Ruehli et al. (2017) can be used to compute the impulse response of the system in terms of s-parameters.
The study of this type of systems can be traced back to that of a multiport system, whose ports are those of the system consisting of the transmitter, the M elements of the metasurface, and the receiver. The M + 2 multiport system is characterized in the time domain using a PEEC solver in terms of its scattering parameters s(t), allowing to use the convolution to obtain the reflected waves b(t) from the incident ones a(t) as:
Once the multiport system is characterized in the time domain, the resulting model can be connected to arbitrary port terminations described by
where
In Pettanice et al. (2022), the PEEC method has been used to compute the impulse response of this multiport system in terms of s-parameters. Then, a convolution-based solver is adopted to study the system with arbitrary sources and terminations. The convolution-based solver is found to be accurate compared to other well-established and known methods based on both frequency and time domain analysis. Furthermore, the convolution-based solver is found to be always stable, differently from traditional time-step methods, e.g., backward differentiation formulas Ruehli et al. (2017). Pettanice et al. (2022) assumes linear terminations, however, the time-domain solver could be used to provide the port voltages and currents also for arbitrary nonlinear terminations. Time-varying scenarios can also be considered by resorting to advanced interpolation techniques which allows taking the time-varying relative positions of transmitters, metasurface elements, and receivers into account. To efficiently integrate it with the communication model, an equivalent compressed model, which accounts for the relevant electromagnetic parameters, is needed.
The outcome of the analysis is an abstraction of the transfer function of the metasurface system, accounting for the coupling among the radiating elements of large surfaces. This guarantees a model that is EM-compliant, mutual coupling and unit cell aware.
5.2 Signal-to-interference-plus-noise ratio characterization
When considering explicitly the NOMA communication, the impulse response of the system with metasurfaces need to be integrated with a model of the multiple access interference in the channel and the SIC procedures at the receiver. At this level of abstraction, such a system could be treated as a multiple access system with active backscattering elements. An analytical framework has been advocated in (Valentini et al., 2020; Valentini et al., 2021a) for systems with backscattering elements, including the impact of the multiple access protocol, the propagation characteristics, and the behavior of the receiver. Then, the convergence of power-domain NOMA protocols and backscattering communications has been studied in (Valentini et al., 2021b).
A key component of the analytical model in Valentini et al. (2021b) is the use of moment matching approximation for the characterization of the signal-to-interference-plus-noise ratio (SINR) to describe the multiple access interference in propagation links and to approximate the capture probabilities at a receiver. In this context, a relevant approach is the use of capture diversity, which exploits the macroscopic diversity offered by multi-static settings with dislocated users. Capture diversity enables SIC during resource units with simultaneous transmissions and, consequently, yields substantial performance improvement in terms of throughput. We believe that this approach has enormous potential for the characterization of radio environments with combination of NOMA and metasurface, as it can be used to predict the system behavior and therefore to optimize the configuration of the metasurface parameters, which can be associated to the reflection coefficients of the backscattering tags.
Let us consider uplink power-domain NOMA for exemplification purposes. The base-band equivalent received signal at the base station can be expressed as
where N is the number of scheduled users, and θn = f(hf,n, hr, vn) is the composite complex gain, which is a function of the channels
We remark that the signal model in Eq. 3 is valid under the assumptions of quasi-static frequency-flat fading channel and single-antenna base station and users. Nevertheless, more general dyadic channel models, which include temporal dispersion and time selectivity, such as the one described in Griffin and Durgin (2008), can be adopted in the proposed deep cross-layer methodology.
Starting from the model of the received signal, signal detection of each user is accomplished by means of SIC. Specifically, by assuming CSI knowledge at the base station, the stronger scheduled user is decoded first and the estimated symbol is exploited to cancel the relative interference contribution from the received signal y. Then, the procedure is re-iterated until all the scheduled users are decoded. The receiver operates on a symbol-by-symbol basis and produces an estimate for each symbol of the nth user as
where
As a distinguishing aspect, the geometric size of the metasurface is typically an order of magnitude larger than the wavelength, so that it should be actually treated as a specular reflector. Backscatter tags are instead more representative of diffusive rather than specular reflectors, since antenna elements’ size is smaller than or comparable with the wavelength Basar et al. (2019). This is a competitive advantage for metasurface-assisted systems compared to e.g., systems with passive backscattering tags. Indeed, the geometric size of the metasurfaces, when appropriately optimized, may yield remarkable link budget gains, which is not achievable with passive backscattering tags. The range of power levels for power-domain discrimination between the near user and the far user in the NOMA systems is increased proportionally with the link budget gain introduced by the metasurface.
Complexity is a fundamental aspect to investigate towards practical implementations of metasurface-aided NOMA systems. The introduction of smart metasurfaces has a cost in terms of additional infrastructure, but this cost is expected to be lower than installing new base stations to increase the coverage. The additional complexity of the algorithms is distributed within the infrastructural elements, including the metasurfaces, which are typically installed in fixed locations with power supply and redundant connectivity options. Power-constrained devices do not need complex operations, besides the SIC operations in downlink. However, the presence of additional elements (i.e., the metasurfaces) to control the propagation environment may simplify the way channel state information is obtained at the power constrained node. In the context of latency-sensitive scenarios, smart metasurfaces provide additional paths from a source to a destination with the additional processing done directly on the radio signal, thus introducing low delays compared to classic amplify and forward systems.
To address the complexity of the system model, a semi-analytical approach that combines the derivation of the electromagnetic characteristics of metasurfaces and Monte-Carlo simulations to describe channel access and scheduling in NOMA is envisioned. As a key challenge in the integration, we believe mobility is the one that poses major challenges to the ability to configure the metasurface-aided NOMA system properly. Indeed, mobility is critical when trying to obtain an accurate estimation of the CSI at the receiver, which is used in NOMA systems to enable interference cancellation.
6 Conclusion
In this paper, we presented a methodological approach for the integration of smart metasurfaces into an application scenario employing non-orthogonal multiple access (NOMA) communications. We reviewed the most recent literature, highlighting challenges and opportunities in the analytical characterization of NOMA systems assisted by metasurfaces. The key idea is a deep cross-layer approach that includes the mutual coupling between electromagnetic fields in the scattering surfaces and the SINR at the receiver. Our future work includes a technical evaluation of the impact of metasurfaces in NOMA systems with a computationally affordable model.
Data availability statement
The original contributions presented in the study are included in the article/supplementary material, further inquiries can be directed to the corresponding author.
Author contributions
All authors contributed to the conception of the study. PD organized the contribution and wrote the first draft of the manuscript. RV and FS contributed mainly to aspects related to NOMA communication. GP and GA contributed mainly to the part concerning smart metasurfaces. All authors contributed to manuscript revision, read, and approved the submitted version.
Conflict of interest
The authors declare that the research was conducted in the absence of any commercial or financial relationships that could be construed as a potential conflict of interest.
Publisher’s note
All claims expressed in this article are solely those of the authors and do not necessarily represent those of their affiliated organizations, or those of the publisher, the editors and the reviewers. Any product that may be evaluated in this article, or claim that may be made by its manufacturer, is not guaranteed or endorsed by the publisher.
References
Abeywickrama, S., Zhang, R., Wu, Q., and Yuen, C. (2020). Intelligent reflecting surface: Practical phase shift model and beamforming optimization. IEEE Trans. Commun. 68, 5849–5863. doi:10.1109/TCOMM.2020.3001125
Albanese, A., Devoti, F., Sciancalepore, V., Di Renzo, M., and Costa-Perez, X. (2022). “Marisa: A self-configuring metasurfaces absorption and reflection solution towards 6g,” in IEEE INFOCOM 2022 - IEEE Conference on Computer Communications, London, United Kingdom (IEEE), 250–259.
Basar, E., Di Renzo, M., De Rosny, J., Debbah, M., Alouini, M.-S., and Zhang, R. (2019). Wireless communications through reconfigurable intelligent surfaces. IEEE Access 7, 116753–116773. doi:10.1109/ACCESS.2019.2935192
Björnson, E., Özdogan, O., and Larsson, E. G. (2020). Reconfigurable intelligent surfaces: Three myths and two critical questions. IEEE Commun. Mag. 58, 90–96. doi:10.1109/MCOM.001.2000407
Bjornson, E., Wymeersch, H., Matthiesen, B., Popovski, P., Sanguinetti, L., and de Carvalho, E. (2022). Reconfigurable intelligent surfaces: A signal processing perspective with wireless applications. IEEE Signal Process. Mag. 39, 135–158. doi:10.1109/MSP.2021.3130549
Cao, X., Yang, B., Huang, C., Yuen, C., Renzo, M. D., Niyato, D., et al. (2021). Reconfigurable intelligent surface-assisted aerial-terrestrial communications via multi-task learning. IEEE J. Sel. Areas Commun. 39, 3035–3050. doi:10.1109/JSAC.2021.3088634
Clerckx, B., Mao, Y., Schober, R., Jorswieck, E. A., Love, D. J., Yuan, J., et al. (2021). Is noma efficient in multi-antenna networks? A critical look at next generation multiple access techniques. IEEE Open J. Commun. Soc. 2, 1310–1343. doi:10.1109/OJCOMS.2021.3084799
Danufane, F. H., Renzo, M. D., de Rosny, J., and Tretyakov, S. (2021). On the path-loss of reconfigurable intelligent surfaces: An approach based on green’s theorem applied to vector fields. IEEE Trans. Commun. 69, 5573–5592. doi:10.1109/TCOMM.2021.3081452
Di Renzo, M., Ntontin, K., Song, J., Danufane, F. H., Qian, X., Lazarakis, F., et al. (2020a). Reconfigurable intelligent surfaces vs. relaying: Differences, similarities, and performance comparison. IEEE Open J. Commun. Soc. 1, 798–807. doi:10.1109/OJCOMS.2020.3002955
Di Renzo, M., Zappone, A., Debbah, M., Alouini, M.-S., Yuen, C., de Rosny, J., et al. (2020b). Smart radio environments empowered by reconfigurable intelligent surfaces: How it works, state of research, and the road ahead. IEEE J. Sel. Areas Commun. 38, 2450–2525. doi:10.1109/JSAC.2020.3007211
Ding, Z., Schober, R., and Poor, H. V. (2020a). Unveiling the importance of SIC in NOMA systems—Part 1: State of the art and recent findings. IEEE Commun. Lett. 24, 2373–2377. doi:10.1109/LCOMM.2020.3012604
Ding, Z., Schober, R., and Poor, H. V. (2020b). Unveiling the importance of sic in noma systems—Part ii: New results and future directions. IEEE Commun. Lett. 24, 2378–2382. doi:10.1109/LCOMM.2020.3012601
Ding, Z., and Vincent Poor, H. (2020). A simple design of irs-noma transmission. IEEE Commun. Lett. 24, 1119–1123. doi:10.1109/LCOMM.2020.2974196
Gradoni, G., and Di Renzo, M. (2021). End-to-end mutual coupling aware communication model for reconfigurable intelligent surfaces: An electromagnetic-compliant approach based on mutual impedances. IEEE Wirel. Commun. Lett. 10, 938–942. doi:10.1109/LWC.2021.3050826
Griffin, J. D., and Durgin, G. D. (2008). Gains for rf tags using multiple antennas. IEEE Trans. Antennas Propag. 56, 563–570. doi:10.1109/TAP.2007.915423
Guo, J., Durrani, S., and Zhou, X. (2019). Monostatic backscatter system with multi-tag to reader communication. IEEE Trans. Veh. Technol. 68, 10320–10324. doi:10.1109/TVT.2019.2937439
Guo, J., Zhou, X., Durrani, S., and Yanikomeroglu, H. (2018). Design of non-orthogonal multiple access enhanced backscatter communication. IEEE Trans. Wirel. Commun. 17, 6837–6852. doi:10.1109/TWC.2018.2864741
IDTechEX (2021). Report: 6g communications market devices materials. Cambridge, United Kingdom: IDTechEx.
Kitayama, D., Hama, Y., Goto, K., Miyachi, K., Motegi, T., and Kagaya, O. (2021). Transparent dynamic metasurface for a visually unaffected reconfigurable intelligent surface: Controlling transmission/reflection and making a window into an rf lens. Opt. Express 29, 29292–29307. doi:10.1364/oe.435648
Le, N. P., and Le, K. N. (2022). Uplink noma short-packet communications with residual hardware impairments and channel estimation errors. IEEE Trans. Veh. Technol. 71, 4057–4072. doi:10.1109/TVT.2022.3148124
Lee, J., Toumpakaris, D., and Yu, W. (2011). Interference mitigation via joint detection. IEEE J. Sel. Areas Commun. 29, 1172–1184. doi:10.1109/JSAC.2011.110606
Li, S., Wei, Z., Yuan, W., Yuan, J., Bai, B., and Ng, D. W. K. (2021). “On the achievable rates of uplink noma with asynchronized transmission,” in 2021 IEEE Wireless Communications and Networking Conference (WCNC), Nanjing, China (IEEE), 1–7. doi:10.1109/WCNC49053.2021.9417538
Liu, J., Li, Y., Song, G., and Sun, Y. (2019). Detection and analysis of symbol-asynchronous uplink noma with equal transmission power. IEEE Wirel. Commun. Lett. 8, 1069–1072. doi:10.1109/LWC.2019.2906184
Liu, Y., Liu, X., Mu, X., Hou, T., Xu, J., Di Renzo, M., et al. (2021). Reconfigurable intelligent surfaces: Principles and opportunities. IEEE Commun. Surv. Tutorials 23, 1546–1577. doi:10.1109/COMST.2021.3077737
Liu, Y., Qin, Z., Elkashlan, M., Ding, Z., Nallanathan, A., and Hanzo, L. (2017). Nonorthogonal multiple access for 5g and beyond. Proc. IEEE 105, 2347–2381. doi:10.1109/JPROC.2017.2768666
Maraqa, O., Rajasekaran, A. S., Al-Ahmadi, S., Yanikomeroglu, H., and Sait, S. M. (2020). A survey of rate-optimal power domain noma with enabling technologies of future wireless networks. IEEE Commun. Surv. Tutorials 22, 2192–2235. doi:10.1109/COMST.2020.3013514
Moltafet, M., Yamchi, N. M., Javan, M. R., and Azmi, P. (2018). Comparison study between pd-noma and scma. IEEE Trans. Veh. Technol. 67, 1830–1834. doi:10.1109/TVT.2017.2759910
Monti, A., Soric, J., Barbuto, M., Ramaccia, D., Vellucci, S., Trotta, F., et al. (2016). Mantle cloaking for co-site radio-frequency antennas. Appl. Phys. Lett. 108, 113502. doi:10.1063/1.4944042
Pan, C., Ren, H., Wang, K., Kolb, J. F., Elkashlan, M., Chen, M., et al. (2021). Reconfigurable intelligent surfaces for 6g systems: Principles, applications, and research directions. IEEE Commun. Mag. 59, 14–20. doi:10.1109/MCOM.001.2001076
Pettanice, G., Loreto, F., Romano, D., Santucci, F., Di Marco, P., Antonini, G., et al. (2022). “Time-domain characterization of reconfigurable intelligent surfaces for wireless communications,” in Proc. of International Symposium and Exhibition on Electromagnetic Compatibility (EMC Europe), Goteborg, Sweden (AMC society), 1–6.
Ramaccia, D., Barbuto, M., Monti, A., Vellucci, S., Marini, A., Toscano, A., et al. (2020a). “Latest developments on non-linear and time-varying metasurfaces and topological antennas,” in 2020 14th European Conference on Antennas and Propagation (EuCAP), Copenhagen, Denmark (IEEE), 1–2. doi:10.23919/EuCAP48036.2020.9135967
Ramaccia, D., Sounas, D. L., Alù, A., Toscano, A., and Bilotti, F. (2020b). Phase-induced frequency conversion and Doppler effect with time-modulated metasurfaces. IEEE Trans. Antennas Propag. 68, 1607–1617. doi:10.1109/TAP.2019.2952469
Ruehli, A. E., Antonini, G., and Jiang, L. (2017). Circuit oriented electromagnetic modeling using the PEEC techniques. Hoboken, NJ: Wiley-IEEE Press.
Shin, W., Vaezi, M., Lee, B., Love, D. J., Lee, J., and Poor, H. V. (2017). Non-orthogonal multiple access in multi-cell networks: Theory, performance, and practical challenges. IEEE Commun. Mag. 55, 176–183. doi:10.1109/MCOM.2017.1601065
Sievenpiper, D. F. (2011). Nonlinear grounded metasurfaces for suppression of high-power pulsed rf currents. IEEE Antennas Wirel. Propag. Lett. 10, 1516–1519. doi:10.1109/LAWP.2011.2182593
Sun, Z., and Jing, Y. (2022). On the performance of multi-antenna irs-assisted noma networks with continuous and discrete irs phase shifting. IEEE Trans. Wirel. Commun. 21, 3012–3023. doi:10.1109/TWC.2021.3117494
Tahir, B., Schwarz, S., and Rupp, M. (2021). Analysis of uplink irs-assisted noma under nakagami-m fading via moments matching. IEEE Wirel. Commun. Lett. 10, 624–628. doi:10.1109/LWC.2020.3043810
Tang, W., Chen, M. Z., Chen, X., Dai, J. Y., Han, Y., Di Renzo, M., et al. (2021). Wireless communications with reconfigurable intelligent surface: Path loss modeling and experimental measurement. IEEE Trans. Wirel. Commun. 20, 421–439. doi:10.1109/TWC.2020.3024887
Valentini, R., Di Marco, P., Alesii, R., and Santucci, F. (2021a). Cross-layer analysis of multi-static rfid systems exploiting capture diversity. IEEE Trans. Commun. 69, 6620–6632. doi:10.1109/TCOMM.2021.3096541
Valentini, R., Di Marco, P., Alesii, R., and Santucci, F. (2021b). Exploiting capture diversity for performance enhancement of aloha-based multi-static backscattering systems in the 6g perspective. Sensors 21, 5070. doi:10.3390/s21155070
Valentini, R., Marco, P. D., Alesii, R., and Santucci, F. (2020). “Cross-layer analysis of distributed passive rfid systems over faded backscattering links,” in 2020 IEEE Wireless Communications and Networking Conference (WCNC), Seoul, South Korea (IEEE), 1–6. doi:10.1109/WCNC45663.2020.9120771
Wei, Z., Yang, L., Ng, D. W. K., Yuan, J., and Hanzo, L. (2020). On the performance gain of noma over oma in uplink communication systems. IEEE Trans. Commun. 68, 536–568. doi:10.1109/TCOMM.2019.2948343
Williams, R. J., de Carvalho, E., and Marzetta, T. L. (2020). “A communication model for large intelligent surfaces,” in 2020 IEEE International Conference on Communications Workshops (ICC Workshops), (IEEE, virtual conference), 1–6. doi:10.1109/ICCWorkshops49005.2020.9145091
Wu, Q., and Zhang, R. (2020). Towards smart and reconfigurable environment: Intelligent reflecting surface aided wireless network. IEEE Commun. Mag. 58, 106–112. doi:10.1109/MCOM.001.1900107
Yang, B., Cao, X., Huang, C., Yuen, C., Qian, L., and Renzo, M. D. (2021). Intelligent spectrum learning for wireless networks with reconfigurable intelligent surfaces. IEEE Trans. Veh. Technol. 70, 3920–3925. doi:10.1109/TVT.2021.3064042
Keywords: smart metasurfaces, time-domain analysis, non-orthogonal multiple access, cross-layer, signal-to- interference-plus-noise ratio (SINR)
Citation: Di Marco P, Valentini R, Santucci F, Pettanice G and Antonini G (2022) Boosting NOMA systems through smart metasurfaces. Front. Comms. Net 3:995135. doi: 10.3389/frcmn.2022.995135
Received: 15 July 2022; Accepted: 23 September 2022;
Published: 07 October 2022.
Edited by:
Marco Di Renzo, UMR8506 Laboratoire des Signaux et Systèmes (L2S), FranceReviewed by:
Xuelin Cao, Singapore University of Technology and Design, SingaporeQiang Li, Jinan University, China
Copyright © 2022 Di Marco, Valentini , Santucci , Pettanice and Antonini. This is an open-access article distributed under the terms of the Creative Commons Attribution License (CC BY). The use, distribution or reproduction in other forums is permitted, provided the original author(s) and the copyright owner(s) are credited and that the original publication in this journal is cited, in accordance with accepted academic practice. No use, distribution or reproduction is permitted which does not comply with these terms.
*Correspondence: Piergiuseppe Di Marco, cGllcmdpdXNlcHBlLmRpbWFyY29AdW5pdmFxLml0