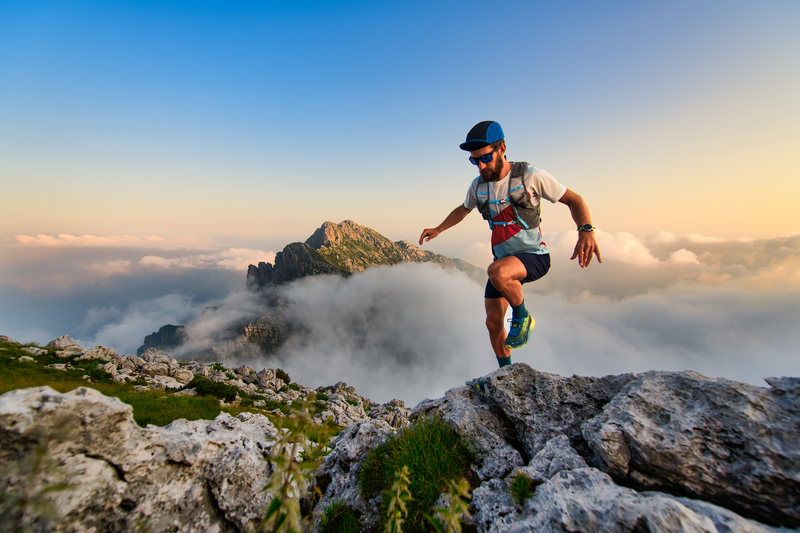
95% of researchers rate our articles as excellent or good
Learn more about the work of our research integrity team to safeguard the quality of each article we publish.
Find out more
ORIGINAL RESEARCH article
Front. Commun. Netw. , 14 June 2021
Sec. Wireless Communications
Volume 2 - 2021 | https://doi.org/10.3389/frcmn.2021.694557
This article is part of the Research Topic Index Modulation for 6G Communications View all 5 articles
In this study, a mixed massive random access scheme is considered where part of users transmit both common information and user-specific information, while others transmit only common information. In this scheme, common information is transmitted by index modulation (IM)–aided unsourced random access (URA), while user-specific information is by IM-aided sourced random access (SRA). Practically, IM-aided URA partitions channel blocks of one transmission frame into multiple groups and then employs the IM principle to activate only part of the channel blocks in each group. IM-aided SRA allocates multiple pilot sequences to each user and activates only one pilot sequence whose index carries the data information. At the receiver, the covariance-based maximum likelihood detection (CB-MLD) is employed to recover the active compressed sensing (CS) code words of URA and information of SRA jointly. To stitch the common information at different blocks of URA, a modified tree decoder is proposed to take the IM constraint into account. Furthermore, to relax the strict threshold requirement and improve the performance, an iterative CS detector and tree decoder are employed to decode the common information, where successive signal reconstruction and interference cancellation are utilized. Finally, computer simulations are given to demonstrate the performance of the proposed scheme.
Massive machine-type communication (mMTC) (Bockelmann et al., 2016) is one of the three main scenarios in the fifth-generation cellular techniques, along with enhanced mobile broadband and ultra-reliable, low-latency communications. The mMTC is motivated by the typical Internet of Things (IoT) applications (Xu et al., 2014), such as smart health care, smart homes, smart manufacturing, and smart transportation. Thus, it is expected to provide wireless connectivity to a massive number of machine-type devices. Unlike human-type communication, in MTC, the packet size of uplink data traffic is short. Therefore, the control signaling overhead becomes very significant compared to the transmit data in the conventional grant-based multiple access. Another feature of mMTC is that the traffic pattern of each device may be sporadic, that is, there are only a small fraction of devices that are active at any time and frequency. Moreover, owing to the massive number of devices, it is impossible to assign orthogonal pilot sequences to all devices (Durisi et al., 2016; Dai et al., 2015; Hasan et al., 2013; Ding et al., 2017).
Unsourced random access (URA) is a promising technique to implement the massive connection in mMTC proposed recently in (Polyanskiy, 2017). In URA, active users transmit signals to base stations (BSs) or access points randomly, and thus avoid the control signaling overhead resulted by the handshake protocol in the grant-based access. Moreover, all users employ the same codebook, and unique pilot allocation for each user required in sourced random access (SRA) can be avoided. This makes URA very suitable for the Internet of Things with a massive number of users. Correspondingly, a receiver at a BS is designed to identify the list of transmit code words, and thus, the per-user probability of error (PUPE) is introduced as a performance metric.
Since the invention of URA, many low-complexity coding schemes have been proposed. In Ordentlich and Polyanskiy (2017), the T-fold slotted ALOHA with the compute-and-forward strategy was proposed. In Vem et al. (2017), this scheme was further studied and an improved scheme was proposed. In this improvement, the user information was first repeated and then transmitted over multiple blocks at the transmitter, and successive interference cancellation (SIC) was utilized at BS.
In Amalladinne (2020), the concatenated URA scheme consisting of inner and outer codes was proposed. In this scheme, the divide-and-conquer strategy was utilized, and the transmit frame is divided into multiple channel blocks. The common compressed sensing (CS) code is employed as an inner code in all blocks and a simple parity-check code as outer tree code across blocks. At BSs, the active code words of inner code are detected through CS-based sparse signal recovery, and the outer tree decoder is utilized to stitch the transmit information sequences in different channel blocks. In Amalladinne et al. (2020a), an enhanced decoder was proposed which performed inner CS detection and outer tree decoding in tandem. This method can reduce the search size of the inner CS detector in later channel blocks and thus reduce the complexity. In Fengler (2019), the concatenated URA scheme was extended to massive MIMO, and covariance-based maximum likelihood detection (CB-MLD) (Haghighatshoar et al., 2018; Fengler et al., 2021) was utilized to perform the inner CS detection. In Xie et al. (2020), the URA for correlated massive MIMO was further studied, and an improved coordinate descent method was proposed to reduce the iteration number. In Fengler et al. (2019), the sparse regression code (SPARC) was utilized in URA, where the approximated messing passing algorithm (AMP) (Donoho et al., 2009; Rangan, 2011) was employed to perform the inner CS detection. In Amalladinne et al. (2020b), motivated by Amalladinne et al. (2020b), an integrating AMP and belief propagation (BP) method was proposed to perform inner CS detection and outer tree decoding in tandem.
In Pradhan et al. (2019), another scheme was proposed for Gaussian channels, where the transmit data are partitioned into two parts. The former is transmitted using a CS code and also acts to determine the corresponding interleaving pattern. The latter is conveyed using low-density parity-check (LDPC)–coded interleave-division multiple access (IDMA) scheme. In Kowshik et al. (2020), this scheme was extended to fading channels, where fading coefficient estimation and LDPC decoding were implemented by the BP algorithm based on the joint factor graph. In Li et al. (2020a), the SPARC-LDPC scheme was proposed for massive MIMO systems, where the CS code in (Pradhan et al., 2019) is replaced by the SPARC. In Marshakov et al. (2019), Pradhan et al. (2020), Zheng et al. (2020), the polar code was taken to replace the LDPC code, in conjunction with the receiver framework of treat interference as noise and SIC (TIN-SIC).
In this article, we extend our earlier works in Liang and Zheng (2020), Liang and Zheng (2021), Liang et al. (2021), and the massive random access scenario where users are divided into two classes is considered. In the first class, users transmit both common and user-specific information. These users need some key devices to transmit user identification (UID)–related information, besides common information uncorrelated to UID. For example, UID-related information can be some urgent event related to its location, and the BS can know the location where urgent event happens and thus make fast response by identifying the active user. In the second class, users transmit only common information. For this scenario, an index modulation (IM) (Basar et al., 2013a; Wen et al., 2017)–aided mixed massive random access is proposed here, where a novel IM-aided URA scheme is presented to transmit common information, and IM-aided SRA (Senel and Larsson, 2018; Ni and Zheng, 2021) is utilized to transmit user-specific information. In IM, only part of resources (time/frequency/space/code sequence) is activated to transmit information by conventional modulations, and the resource activation pattern also carries information. In general, IM has attracted much attention in many aspects due to the potential advantages. For example, better error performance (Basar et al., 2013b; Zheng and Chen, 2017), stronger robustness in the rapidly time-varying channel (Basar et al., 2013c), higher spectral efficiency (Wen et al., 2016), and energy efficiency (Wen et al., 2014). Moreover, in the massive multiple access, IM has been employed in coherent grant-based NOMA (Arslan et al., 2020; Althunibat et al., 2019; Tusha et al., 2020; Li et al., 2020b) and non-coherent grant-free NOMA (Senel and Larsson, 2018; Ni and Zheng, 2021; Ni and Zheng, 2020).
The proposed IM-aided URA is constructed on the general framework of a concatenated URA scheme (Fengler et al., 2019; Amalladinne et al., 2020a) to transmit common information. The motivation is that with the implementation of IM in URA, the signal to be recovered will be sparser, which will facilitate the CB-MLD and result in better decoding performance. On the other hand, the implementation of IM will decrease the number of parity check bits in the outer code, which will increase the complexity of the tree decoder generally. However, the better CB-MLD results will reduce the false alarm probability and thus decrease the number of candidate-active code words. This will instead reduce the complexity of the tree decoder since the CB-MLD results are inputs of the tree decoder. Therefore, with proper design of IM, a better trade-off by IM-aided URA can be expected.
Concretely, in the proposed IM-aided URA, the
At the BS, the CB-MLD is first employed block by block to estimate active code words of IM-aided URA and user-specific information of SRA simultaneously. Moreover, to stitch the common information in different blocks, a modified tree decoder is proposed to take the IM signal property into account. Next, to relax the strict threshold requirement and improve the performance, an iterative CS detection and tree decoding are employed to decode the common information, where the covariance of the received signal associated with code words decided in the last iteration is reconstructed and pre-subtracted, and the resulted residual covariance is used for inner CS detection in the current iteration.
The main contributions of this study are summarized as follows:
(1) A mixed massive random access scheme is presented, where part of vital users transmit both common information and user-specific information, while other users transmit only common information. This scheme can model the random access scenario consisting of key devices whose UID is required at the BS and conventional devices whose transmit information is the only interest for the BS.
(2) An IM-aided URA technique is proposed for the common information transmission. Moreover, the corresponding modified tree decoder is presented. The proposed scheme shows better performance than conventional URA when the transmit information is short, for example, 36 bits per frame in our simulations.
(3) An iterative receiver is proposed to decode the common information. The iterative receiver can lift the performance and relax the requirement of thresholds in the inner CS detector, compared with conventional non-iterative receiver.
The rest of this article is organized as follows. In Section 2, the signal model of the proposed mixed massive random access is given. In Section 3, the CB-MLD is presented. In Section 4, a modified tree decoder is described. In Section 4, the proposed iterative receiver is presented. Finally, computer simulation results are given in Section 6, and we conclude this study in Section 7.
Notations: For a set
The uplink IM-aided URA considered here consists of K single-antenna users and one M-antennas BS, as shown in Figure 1. In the mMTC-enabled massive MIMO system, K and M are of the order of hundreds or thousands. The K users are divided into two classes. As shown in Figure 2, in the first class with
FIGURE 1. Uplink mMTC system. All users are divided into two classes with sets
The common information is transmitted by all active users. For each active user to transmit the common information, the IM-aided URA is employed. In the proposed IM-aided URA, all the
The transmitter of the proposed URA-IM scheme is shown in Figure 3. Consider the active user k. The length
The first part
The IM principle is utilized to perform the mapping from
On the other hand, the second part
Denote
where the index m is such that
where
Finally, the transmit signals of the user k in all groups are collected as
Example 1: Consider the case of
Finally, from Figure 2, for the active user k in
Remark 1: By employing the proposed IM-aided URA scheme to transmit common information, the average number of active code words in each block is
Remark 2: Compared with conventional URA (Fengler et al., 2019; Amalladinne et al., 2020a), the number of parity check bits of the proposed IM-aided URA will be reduced from
The user-specific information is also transmitted by active users in
Remark 3: Here, for simplicity, only one active pilot sequence (Senel and Larsson, 2018) is considered. This can be extended straightforwardly to the case where multiple pilot sequences are activated (Ni and Zheng, 2021).
Finally, the superimposed transmit signal of user k in the nth block of the gth group can be presented by
where
At the BS, the received signal
Here,
Assuming the first
Here,
In this section, the CB-MLD is presented. To facilitate the presentation, Eq. 5 is first represented according to the following procedure.
Let
where
From Eq. 6, the columns
Then, the covariance matrix
On the other hand, the empirical covariance matrix can be acquired by the following equation:
Define the log-likelihood function by the following equation:
Then, the ML estimate can be acquired by the following equation:
This problem is non-convex and can be solved efficiently by decent method (Haghighatshoar et al., 2018; Fengler et al., 2021). For autonomy, this algorithm is summarized in Algorithm 1, whose complexity is linear in
After obtaining
where the max operation is from the fact that only one
Transmit user-specific information can then be recovered by inverse IM mapping from
where
To take IM constraint into account, the modified outer tree decoder is processed group by group. Here, only the processing of the gth,
In the index bit node, for each survived path l obtained from the last node processing, the
The whole process is done for each survived path l of
FIGURE 5. Illustration of the modified outer tree decoder in the gth group with
By the CB-MLD described in Section 3, we observe a good decoding performance of user-specific information from extensive simulations. On the other hand, the decoding performance of common information is highly dependent on the threshold
Here, the iterative receiver for common information is studied. The basic ideas are two-fold. First, the SIC strategy is employed where the covariance of active code words obtained in former iteration is reconstructed and pre-subtracted from the empirical covariance matrix. This means that the signal to be estimated will be sparser in the subsequent iterations and thus can improve the performance of CB-MLD. Second, the strict threshold requirement can be relaxed since multiple iterations exist, and the threshold should decrease with iteration number. Then, in former iterations, low probability of false alarm can be guaranteed by larger threshold which also alleviates the error propagation. In subsequent iterations, the smaller threshold can reduce the probability of misdetection. In the following text, the iterative receiver is described in detail.
Noting that a good decoding performance of user-specific information is observed using CB-MLD, the covariance associated with user-specific information can be reconstructed and canceled first.
Denote the set of decided active users associated with user-specific information as follows:
Then, the
where
Therefore, the covariance is reconstructed and subtracted from the empirical covariance by the following equation:
where the approximation
The block diagram of the proposed iterative receiver for common information is shown in Figure 6. By using the SIC strategy, the processing in the tth iteration is described as below.
Denote the modified output list of inner CB-MLD in the tth iteration as follows:
where
where
Denote the message list after outer modified tree decoder as follows:
and the corresponding list in the nth block of group g as follows:
Note that one code word in some sub-frame can appear multiple times in the message list of the tree decoder output. Define the times of index r appears in
and the set of indices which appear m times,
Then, the
with
Here,
Example 2: Assume
Next, the reconstructed covariance is subtracted from the residual empirical covariance matrix by the following equation:
with initialization
where
Before sending to outer tree decoder,
This modification is to take the following case into account when
In summary, the iterative receiver is summarized in Algorithm 3.
Remark 4: In the threshold Eq. 22, four parameters
In this section, simulation results are given to validate the performance of the proposed IM-aided mixed massive random access. For common information, the per-user probabilities of misdetection and false alarm are defined as follows:
where
where
where
The system parameters for common information of the IM-aided URA scheme are
Moreover, the power allocation coefficient is set as
In Figure 7, the error probability vs. the number of active user
FIGURE 7. Error probability vs. the number of active users
In Figure 8, the performance with
FIGURE 8. Error probability vs. the number of active users
Figure 9 gives the error probability vs. channel block length L with fixed
FIGURE 9. Error probability vs. channel blocks length L. Here, the numbers of BS antennas and active users are
Figure 10 gives the error probability vs. channel block length L with fixed
FIGURE 10. Error probability vs. channel blocks length L. Here, the numbers of BS antennas and active users are
Table 3 lists the runtime of the receiver signal processing in both IM-aided URA and URA (Fengler et al., 2019). Here,
TABLE 3. Runtime of receiver of IM-aided URA and URA (Fengler et al., 2019),
The case with more common information bit transmission, that is, larger
Next, the performance of the iterative receiver is studied. In Figure 11,
FIGURE 11. Per-user error probability of misdetection for common information as a function of the iterative number for various numbers of antennas and active users.
FIGURE 12. Per-user error probability of false alarm for common information as a function of the iterative number for various numbers of antennas and active users.
FIGURE 13. Per-user error probability for common information as a function of the number of active users.
Table 4 gives the runtime of the proposed iterative receiver in the case of
In non-iterative IM-aided URA receiver, the runtime, as shown in Table 3, is 113.4s for the inner CB-MLD detector, 12.4s for the outer tree decoder, and the whole runtime is 125.8s. From Table 4, in the proposed iterative IM-aided receiver, the total runtime is 396.8s, about 3 times longer than that of the non-iterative IM-aided receiver, although there are five iterations in the iterative receiver. Recalling the superiority of the proposed iterative receiver in terms of the relaxed requirement of thresholds and better PUPE performance, this mild increased complexity is tolerable.
In this article, a mixed massive random access was proposed where the considered uplink massive access system consists of users transmitting both common and user-specific information and users transmitting only common information. An IM-aided URA was proposed to transmit common information, and IM-aided non-coherent SRA was utilized to transmit user-specific information. The CB-MLD was employed to recover the user-specific information directly and the active code words associated with common information. Furthermore, the modified outer tree decoder was proposed to stitch the common information in different blocks, and an iterative receiver consisting of inner CS-based detection and outer tree decoding was employed to improve the performance. Finally, simulation results validated the superiority of the proposed mixed random access scheme.
Compared to conventional URA (Fengler et al., 2019), when there are more common information bits, that is, larger
The original contributions presented in the study are included in the article/Supplementary Material, and further inquiries can be directed to the corresponding author.
ZL and JZ conceived the work and wrote the article and ZL and JN carried out investigations.
This work was supported in part by the National Natural Science Foundation of China (grant 61671340).
The authors declare that the research was conducted in the absence of any commercial or financial relationships that could be construed as a potential conflict of interest.
Althunibat, S., Mesleh, R., and Rahman, T. F. (2019). A Novel Uplink Multiple Access Technique Based on index-modulation Concept. IEEE Trans. Commun. 67 (7), 4848–4855. doi:10.1109/TCOMM.2019.2909211
Amalladinne, V. K., Pradhan, A. K., Rush, C., Chamberland, J.-F., Narayanan, K. R., et al. (2020). Unsourced Random Access with Coded Compressed Sensing: Integrating AMP and Belief Propagation. arXiv: 2010.04364v1, [cs.IT].
Amalladinne, V. K., Chamberland, J.-F., and Narayanan, K. R. (2020a). A Coded Compressed Sensing Scheme for Unsourced Multiple Access. IEEE Trans. Inform. Theor. 66 (10), 6509–6533. doi:10.1109/TIT.2020.3012948
Amalladinne, V. K., Chamberland, J.-F., and Narayanan, K. R. (2020b). An Enhanced Decoding Algorithm for Coded Compressed sensingICASSP 2020 - 2020 IEEE International Conference on Acoustics, Speech and Signal Processing (ICASSP). Barcelona, Spain, 5270–5274. doi:10.1109/ICASSP40776.2020.9054142
Arslan, E., Dogukan, A. T., and Basar, E. (2020). Index Modulation-Based Flexible Non-orthogonal Multiple Access. IEEE Wireless Commun. Lett. 9 (11), 1942–1946. doi:10.1109/LWC.2020.3009100
Basar, E., Aygolu, U., Panayirci, E., and Poor, H. V. (2013a). Orthogonal Frequency Division Multiplexing with index Modulation. IEEE Trans. Signal. Process. 61 (22), 5536–5549. doi:10.1109/TSP.2013.2279771
Basar, E., Aygölü, U., and Panaylrcl, E. (2013b). Orthogonal Frequency Division Multiplexing with index Modulation in the Presence of High Mobility. (BlackSeaCom). Georgia: Batumi, 5536–5549. doi:10.1109/BlackSeaCom.2013.6623399
Basar, E. (2016). Index Modulation Techniques for 5G Wireless Networks. IEEE Commun. Mag. 54 (7), 168–175. doi:10.1109/MCOM.2016.7509396
Bockelmann, C., Pratas, N., Nikopour, H., Au, K., Svensson, T., Stefanovic, C., et al. (2016). Massive Machine-type Communications in 5G: Physical and MAC-Layer Solutions. IEEE Commun. Mag. 54 (9), 59–65. doi:10.1109/MCOM.2016.7565189
Dai, L., Wang, B., Yuan, Y., Han, S., Chih-lin, I., and Wang, Z. (2015). Non-orthogonal Multiple Access for 5G: Solutions, Challenges, Opportunities, and Future Research Trends. IEEE Commun. Mag. 53 (9), 74–81. doi:10.1109/MCOM.2015.7263349
Ding, Z., Lei, X., Karagiannidis, G. K., Schober, R., Yuan, J., and Bhargava, V. K. (2017). A Survey on Non-orthogonal Multiple Access for 5G Networks: Research Challenges and Future Trends. IEEE J. Select. Areas Commun. 35 (10), 2181–2195. doi:10.1109/JSAC.2017.2725519
Donoho, D. L., Maleki, A., and Montanari, A. (2009). Message-passing Algorithms for Compressed Sensing. Pnas. 106 (45), 18914–18919. doi:10.1073/pnas.0909892106
Durisi, G., Koch, T., and Popovski, P. (2016). Toward Massive, Ultrareliable, and Low-Latency Wireless Communication with Short Packets. Proc. IEEE. 104 (9), 1711–1726. doi:10.1109/JPROC.2016.2537298
Fengler, A., Caire, G., Jung, P., and Haghighatshoar, S. (2019). Massive MIMO Unsourced Random Access. arXiv: 1901.00828v1, [cs.IT].
Fengler, A., Jung, P., and Caire, G. (2019). SPARCs for Unsourced Random Access. arXiv:1901.06234v1, [cs.IT].
Fengler, A., Haghighatshoar, S., Jung, P., and Caire, G. (2021). Non-Bayesian Activity Detection, Large-Scale Fading Coefficient Estimation, and Unsourced Random Access with a Massive MIMO Receiver. IEEE Trans. Inform. Theor. 67, 2925–2951. doi:10.1109/TIT.2021.3065291
Haghighatshoar, S., Jung, P., and Caire, G. (2018). Improved Scaling Law for Activity Detection in Massive MIMO Systems. Proc IEEE ISITVail, 381–385. doi:10.1109/ISIT.2018.8437359
Hasan, M., Hossain, E., and Niyato, D. (2013). Random Access for Machine-To-Machine Communication in LTE-Advanced Networks: Issues and Approaches. IEEE Commun. Mag. 51 (6), 86–93. doi:10.1109/MCOM.2013.6525600
Kowshik, S. S., Andreev, K., Frolov, A., and Polyanskiy, Y. (2020). Energy Efficient Coded Random Access for the Wireless Uplink. IEEE Trans. Commun. 68 (8), 4694–4708. doi:10.1109/TCOMM.2020.3000635
Li, T., Wu, Y., Zheng, M., Wang, D., and Zhang, W. (2020a). SPARC-LDPC Coding for MIMO Massive Unsourced Random Access. arXiv: 2009.10912v2, [cs.IT]. doi:10.1109/GCWkshps50303.2020.9367450
Li, J., Li, Q., Dang, S., Wen, M., Jiang, X.-Q., and Peng, Y. (2020b). Low-Complexity Detection for Index Modulation Multiple Access. IEEE Wireless Commun. Lett. 9 (7), 1. doi:10.1109/LWC.2020.2974730
Liang, Z., and Zheng, J. (2021). Iterative Receiver of Uplink Massive MIMO Unsourced Random Access. Harbin, China: IEEE IWCMCIn press.
Liang, Z., and Zheng, J. (2020). Mixed Massive Random Access. IEEE ICCT, 311–316. doi:10.1109/ICCT50939.2020.9295776
Liang, Z., Zheng, J., and Ni, J. (2021). Index Modulation Aided Massive MIMO Un-sourced Random Access. Melbourne, Victoria, Austria: IEEE ISITIn press
Marshakov, E., Balitskiy, G., Andreev, K., and Frolov, A. (2019). A Polar Code Based Unsourced Random Access for the Gaussian MAC. Honolulu, HI, USA: IEEE VTC-fall. doi:10.1109/VTCFall.2019.8891583
Ni, J., and Zheng, J. (2021). Index Modulation-Based Non-coherent Transmission in grant-free Massive Access. IEEE Trans. Veh. Technol. 70 (1), 1025–1029. doi:10.1109/TVT.2020.3045448
Ni, J., and Zheng, J. (2020). Non-Coherent Grant-Free NOMA through Pilot-And Channel Block-Index Modulation. IEEE Wireless Commun. Lett. 10 (4), 1. doi:10.1109/LWC.2020.3040363
Ordentlich, O., and Polyanskiy, Y. (2017). Low Complexity Schemes for the Random Access Gaussian Channel. Proc. IEEE ISIT, 2528–2532. doi:10.1109/ISIT.2017.8006985
Polyanskiy, Y. (2017). A Perspective on Massive Random-Access. Proc. IEEE ISIT, 2523–2527. doi:10.1109/ISIT.2017.8006984
Pradhan, A., Amalladinne, V., Vem, A., Narayanan, K. R., and Chamberland, J.-F. (2019). A Joint Graph Based Coding Scheme for the Unsourced Random Access Gaussian Channel. Proc. IEEE GLOBECOM, 1–6. doi:10.1109/GLOBECOM38437.2019.9013278
Pradhan, A. K., Amalladinne, V. K., Narayanan, K. R., and Chamberland, J.-F. (2020). Polar Coding and Random Spreading for Unsourced Multiple Access. arXiv: 1911.01009v1, [cs.IT]. doi:10.1109/ICC40277.2020.9148687
Rangan, S. (2011). Generalized Approximate Message Passing for Estimation with Random Linear Mixing. Proc IEEE ISIT, 2168–2172. doi:10.1109/ISIT.2011.6033942
Senel, K., and Larsson, E. G. (2018). Grant-free Massive MTC-Enabled Massive MIMO: A Compressive Sensing Approach. IEEE Trans. Commun. 66 (12), 6164–6175. doi:10.1109/TCOMM.2018.2866559
Tusha, A., Dogan, S., and Arslan, H. (2020). A Hybrid Downlink NOMA with OFDM and OFDM-IM for beyond 5G Wireless Networks. IEEE Signal. Process. Lett. 27 (3), 491–495. doi:10.1109/LSP.2020.2979059
Vem, A., Narayanan, K. R., Cheng, J., and Chamberland, J.-F. (2017). A User-independent Serial Interference Cancellation Based Coding Scheme for the Unsourced Random Access Gaussian Channel. Proc. IEEE ITW, 121–125. doi:10.1109/ITW.2017.8278023
Wen, M., Cheng, X., Ma, M., Jiao, B., and Poor, H. V. (2016). On the Achievable Rate of OFDM with Index Modulation. IEEE Trans. Signal. Process. 64 (8), 1919–1932. doi:10.1109/TSP.2015.2500880
Wen, M., Cheng, X., and Yang, L. (2014). Optimizing the Energy Efficiency of OFDM with index Modulation. IEEE Int. Conf. Commun. Syst., 31–35. doi:10.1109/ICCS.2014.7024760
Wen, M., Cheng, X., and Yang, L. (2017). Index Modulation for 5G Wireless Communications (Wireless Networks). Berlin, Germany: Springer.
Xie, X., Wu, Y., Gao, J., and Zhang, W. (2020). Massive Unsourced Random Access for Massive MIMO Correlated Channels. arXiv: 2008.08742v2 [cs.IT]. doi:10.1109/GLOBECOM42002.2020.9347959
Xu, L. D., He, W., and Li, S. (2014). Internet of Things in Industries: A Survey. IEEE Trans. Ind. Inf. 10 (4), 2233–2243. doi:10.1109/TII.2014.2300753
Zheng, J., and Chen, R. (2017). Achieving Transmit Diversity in OFDM-IM by Utilizing Multiple Signal Constellations. IEEE Access 5, 8978–8988. doi:10.1109/ACCESS.2017.2708718
Keywords: index modulation, random access, compressed sensing, iterative receiver, non-Bayesian detection, tree decoder, massive MIMO
Citation: Liang Z, Zheng J and Ni J (2021) Index Modulation–Aided Mixed Massive Random Access. Front. Comms. Net 2:694557. doi: 10.3389/frcmn.2021.694557
Received: 13 April 2021; Accepted: 21 May 2021;
Published: 14 June 2021.
Edited by:
Shuping Dang, King Abdullah University of Science and Technology, Saudi ArabiaReviewed by:
Xuan Chen, South China University of Technology, ChinaCopyright © 2021 Liang, Zheng and Ni. This is an open-access article distributed under the terms of the Creative Commons Attribution License (CC BY). The use, distribution or reproduction in other forums is permitted, provided the original author(s) and the copyright owner(s) are credited and that the original publication in this journal is cited, in accordance with accepted academic practice. No use, distribution or reproduction is permitted which does not comply with these terms.
*Correspondence: Jianping Zheng, anB6aGVuZ0B4aWRpYW4uZWR1LmNu
Disclaimer: All claims expressed in this article are solely those of the authors and do not necessarily represent those of their affiliated organizations, or those of the publisher, the editors and the reviewers. Any product that may be evaluated in this article or claim that may be made by its manufacturer is not guaranteed or endorsed by the publisher.
Research integrity at Frontiers
Learn more about the work of our research integrity team to safeguard the quality of each article we publish.