- James Watt School of Engineering, University of Glasgow, Glasgow, United Kingdom
There is no doubt that the world is currently experiencing a global pandemic that is reshaping our daily lives as well as the way business activities are being conducted. With the emphasis on social distancing as an effective means of curbing the rapid spread of the infection, many individuals, institutions, and industries have had to rely on telecommunications as a means of ensuring service continuity in order to prevent complete shutdown of their operations. This has put enormous pressure on both fixed and mobile networks. Though fifth generation mobile networks (5G) is at its infancy in terms of deployment, it possesses a broad category of services including enhanced mobile broadband (eMBB), ultra-reliable low-latency communications (URLLC), and massive machine-type communications (mMTC), that can help in tackling pandemic-related challenges. Therefore, in this paper, we identify the challenges facing existing networks due to the surge in traffic demand as a result of the COVID-19 pandemic and emphasize the role of 5G empowered by artificial intelligence in tackling these problems. In addition, we also provide a brief insight on the use of artificial intelligence driven 5G networks in predicting future pandemic outbreaks, and the development a pandemic-resilient society in case of future outbreaks.
1. Introduction
Since the COVID-19 outbreak became a pandemic, there has been drastic and dramatic changes in our daily lives and the way we conduct business activities. Literally every sector of the economy (e.g., health, education, manufacturing, transportation, etc.) has been affected by the pandemic. Many companies have shut down as workers were advised to work from home, schools are closed and resorted to online learning while conferences were postponed or moved online1. Even appointments with doctors were canceled, postponed or conducted remotely in order to reduce contact with patients and curtail the spread of the infection (Singh et al., 2020; Ting et al., 2020). All these sectors now depend mainly on both wired and wireless communication networks to ensure continued operation.
As a result, there is a great increase in the use of online learning platforms, such as Zoom, Microsoft Teams, Canvas, etc., as well as an increase in the use of social media platforms, such as Facebook, Twitter, WhatsApp, etc., for social interactions. In addition, there is also a surge in the use of multimedia platforms, such as online gaming, Netflix, YouTube, etc., in order to keep people entertained during the lockdown period in effect in many countries (Elavarasan and Pugazhendhi, 2020; Ting et al., 2020).
These factors have led a surge in traffic demand on existing networks because the movement restriction policy put in place in different countries to reduce the spread of the infection has forced many workers and students to stay at home, thereby shifting normal activities in schools, hospital, and industries to online platforms. In addition, there is also a sudden shift in the traffic demand pattern from city centers to residential areas due to the stay-at-home policy. This is very challenging for existing networks as they have not been designed to accommodate this magnitude of traffic demand as well as the sudden shift in traffic demand pattern that they are currently experiencing.
Furthermore, with the shift in traffic demand to residential areas, little or no traffic demand is being placed on base stations (BSs) in city centers, resulting in energy wastage. In addition, the movement restrictions has led to a reduction in the number of maintenance workers who access BS cell sites to restore them to normal functionality in case of cell outages or service failure. Hence, network operators have had to grapple with increased capacity demands both on the radio access network (RAN) as well as the backhaul network in residential areas, how to promptly restore faulty sites to normal operations in order to prevent prolonged service failure due to cell outages, and also how to prevent energy wastage due to under-utilization of BSs in city centers, among other challenges. It is worth noting that due to existing wireless networking technologies, the impact of the pandemic have been greatly reduced, therefore, the development and deployment of more advanced wireless communication technologies, such as fifth generation mobile networks (5G) and beyond 5G (B5G), would be more effective in alleviating the negative effects of the pandemic on the various sectors.
5G networks is currently being deployed in some countries, and—compared to existing wireless networks—it would provide higher data rates, higher capacity to accommodate massive device connectivity, lower latency, higher reliability, high mobility support as well as enhanced energy efficiency (Agiwal et al., 2016; Chih-Lin et al., 2016). All these can be provided through three broad categories of services offered by 5G networks, namely: enhanced mobile broadband (eMBB), ultra-reliable low-latency communications (URLLC), and massive machine-type communications (mMTC) (Andrews et al., 2014). In addition, self-organizing networks (SON) and artificial intelligence (AI) have been implemented in 5G networks to enable the network to function in an intelligent and autonomous manner (Aliu et al., 2013; Imran et al., 2014; Klaine et al., 2017). Even though AI has only been partially included in 5G, beyond 5G (B5G) networks will experience the full implementation of AI (Letaief et al., 2019; Shafin et al., 2019). AI in 5G networks will enable intelligent and autonomous operations which is of immense importance in the current and future pandemic situations due to the drastic changes in network service demands and operational procedures resulting from movement restrictions and remote working policies put in place by government and various organizations to curtail the spread of COVID-19.
AI will enable proactive and dynamic resource allocation that will enable the network assign resources to locations that need them on a real time demand basis unlike the static resource allocation approach that is currently implemented in existing networks (Lee and Qin, 2019). This will help solve the problem of sudden shift in traffic demands from city centers to residential areas occasioned by the pandemic that is putting pressure on the RAN and backhaul of the network. In addition, AI will also help in traffic prediction and cell outage detection and enable the network to automatically reconfigure itself by switching off underutilized base stations or activate self-healing functionalities of SON (Klaine et al., 2017). This is necessary during this pandemic because of the drastic reduction in the available manpower required to attend to network challenges. Furthermore, AI will enable proactive handover optimization (Mollel et al., 2019; Mollel et al., 2020) that is needed to handle emergence health challenges during the pandemic where patients in critical conditions have to be attended to remotely via video/voice communications with medics in the hospital while in the ambulance en route hospital to prevent intermittent service disruption or failure resulting from switching connection from one base station to another.
Therefore, in this paper, we highlight the role of AI-driven 5G network in handling the challenges facing existing networks due to COVID-19 pandemics. We are focusing on networking related challenges which can be addressed by using AI in 5G. It should however be noted that since only a few AI-based solutions can be implemented in 5G, some of the AI solutions highlighted in this work would be implemented in B5G networks. Moreover, we wish to state that since a lot of review papers have been written on the application of AI and machine learning (ML) techniques in 5G and B5G networks (Mwanje et al., 2016; Li et al., 2017; Fu et al., 2018; Letaief et al., 2019; Shafin et al., 2019; Yao et al., 2019; Wang et al., 2020c; Zhang et al., 2020), as well as an in-depth analysis on the use of ML algorithms in both 5G and B5G networks (Fadlullah et al., 2017; Klaine et al., 2017; Chen et al., 2019; Morocho-Cayamcela et al., 2019; Sun et al., 2019; Wang et al., 2019, 2020; Xiong et al., 2019), we do not intent to repeat what they have already done in this work. Our goal is to identify some specific networking challenges that existing networks are facing due to COVID-19 pandemic and to highlight some AI/ML-driven solutions that can help 5G and B5G networks handle such problems.
1.1. Contributions
To the best of our knowledge, there are three major review papers (Ahmadi et al., 2020; Chamola et al., 2020; Saeed et al., 2020) that are closely related to our work. In Ahmadi et al. (2020), the authors first highlighted the role of mobile connectivity in assisting the healthcare, education and retail sectors during this pandemic after which a very brief discussion of some key 5G technologies including URLLC, mMTC, cloud, etc., that will be beneficial to various sectors was presented. In Chamola et al. (2020), the authors initially presented a review of COVID-19 outbreak including previous pandemics, various stages of the pandemic outbreak, diagnostic testing, as well as treatments and preventive measures. Then, a brief discussion on the impact of the pandemic on different sectors of the economy with relevant statistics was presented. In addition, a review of some technologies needed to reduce the impact of the pandemic including IoT, drones, robots, wearables, 5G, etc., was presented. The authors in Saeed et al. (2020) considered the role of wireless communications in fighting the pandemic by specifically considering healthcare, education, and the economy, and explored possible ways of extending network connectivity to unconnected areas via drones, balloons, and satellite. They also considered three challenges resulting from the use of wireless communication to combat COVID-19 pandemic which are privacy, security, and misinformation, as well as the role of wireless communications in ensuring the survival of the economy. In addition, some wireless network enabled technologies that found relevance during the pandemic and will remain useful afterwards were presented. Various review papers have been written on the application of AI in 5G, and B5G networks (Mwanje et al., 2016; Li et al., 2017; Fu et al., 2018; Letaief et al., 2019; Shafin et al., 2019; Yao et al., 2019; Wang et al., 2020c; Zhang et al., 2020) as well as in-depth analysis on the use of various ML algorithms in 5G and B5G network (Fadlullah et al., 2017; Klaine et al., 2017; Chen et al., 2019; Morocho-Cayamcela et al., 2019; Sun et al., 2019; Wang et al., 2019, 2020; Xiong et al., 2019).
However, in this work we adopt a methodological approach in discussing the role of AI-driven 5G networks in handling pandemic related challenges in different sectors as well as the challenges facing existing networks due to their increased use during the pandemic. We specifically focus on how the use of AI in 5G networks can handle the challenges facing existing networks due to the pandemic, strictly from a networking perspective. We also discuss the role of AI-driven 5G as an enabler for other technologies, such as IoT, Robots, autonomous vehicles, drones, etc., that are needed to combat the effects of the pandemic in various sectors. The contributions of our paper are as follows:
• We provide a comprehensive review of five key sectors that have been affected by the COVID-19 pandemic as well as the overall impact of the pandemic on the economy. Different from Chamola et al. (2020), that focused only on the economic impact of the pandemic in these sectors, we also considered the social and psychological effects of the pandemic.
• We highlight the major issues facing existing networks due to the sudden increase in traffic demand placed on them as users migrate from normal to remote operations via online platforms. Very little is mentioned about the impact on existing networks in Ahmadi et al. (2020), Chamola et al. (2020), and Saeed et al. (2020).
• We specifically focus on the role of AI driven 5G networks in reducing the negative effects of the pandemic in the key sectors identified and how it acts as an enabler for other technologies needed to combat pandemic related challenges.
• In addition, the role of AI-driven 5G in tackling the challenges facing existing networks was comprehensively discussed. Again, very little has been written about how AI-driven 5G can tackle the challenges facing existing networks in the aforementioned existing works.
• Finally, we provide a brief insight into how AI-driven 5G can act as an enabler in the prediction of future pandemics as well as its role in developing a pandemic-resilient society to proof the society against sudden disruptions due to pandemics. The lessons learnt from the pandemic that should be considered in the design of future networks are also briefly highlighted.
1.2. Organization
The remainder of this paper is organized as follows: section 2 identifies some key sectors that have been affected by the pandemic. Section 3 discusses the challenges facing existing networks in dealing with increased traffic demand from various sectors due user migration to online platforms as a result of by movement restrictions. Section 4 highlights the role of 5G alongside its technology enablers, such as SON and AI in addressing the problems facing different economic sectors due to the COVID-19 pandemic as well as in tackling the challenges facing existing networks. Section 5 briefly describes how 5G alongside other enabling technologies can be used to predict future pandemics and assist in developing a society that is resilient to pandemics. In addition, we briefly discuss how the lessons learnt from the pandemic will influence the design of future networks. Lastly, section 6 concludes the paper.
2. The Impact of the COVID-19 Pandemic on Different Sectors
The outbreak of COVID-19 and its subsequent declaration as a pandemic by the World Health Organization (WHO) has tremendously altered our life and society. The effects of its devastation is being felt all around the world. While it has affected almost all aspects of our lives and the economy, in Figure 1, we highlight a few key sectors where the impact has been particularly pronounced.
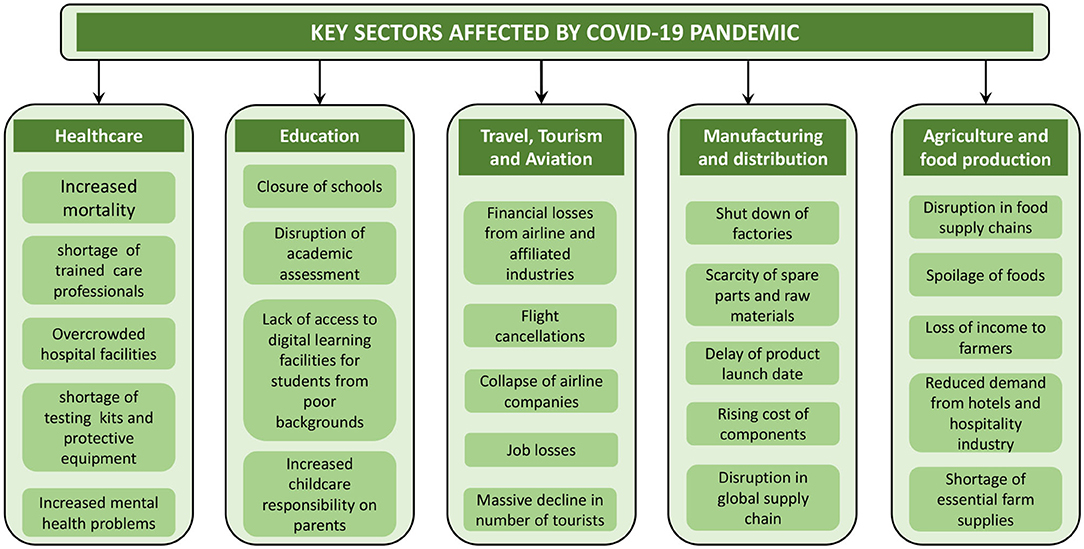
Figure 1. The figure highlights the impact of COVID-19 pandemic on the main sectors of the economy including healthcare, agriculture, travel and tourism, manufacturing and distribution, and agriculture.
2.1. Healthcare
The COVID-19 pandemic has severely impacted the healthcare sector. The magnitude of the outbreak has overwhelmed healthcare facilities, leading to a shortage of trained critical care professionals, insufficient bed spaces, severe shortage of testing kits, ventilators, personal protective equipment (PPE), etc. (Emanuel et al., 2020). A direct and immediate consequence of this is the high number of fatalities recorded daily, both directly as a result of the virus and indirectly due to other ailments, as medical care for non-COVID-19 patients have all but stopped in some countries, in some cases leading to indefinite postponement of scheduled health consultations for other ailments (Radi et al., 2020). The diversion of hospital and care facilities toward COVID-19 treatment has led to a chronic shortage in care for other ailments, leading to the so-called “excess deaths” (Leon et al., 2020) which captures the number of fatalities directly due to COVID-19 and indirectly due to inaccessibility to care that would otherwise have been available to patients but for the virus. There will likely be more maternal and child mortality arising from reduced healthcare coverage for non-COVID-19 ailments than from the disease itself in low- and middle-income countries (Fore, 2020).
To date (7th June 2020), there have been over 390 thousand deaths globally, with over a 110 thousand in the United States alone. The unprecedented surge in patient numbers have overwhelmed hospital facilities, leading to patients being treated in hospital hallways and makeshift bed spaces in many countries. Some European countries had to airlift patients to Germany to receive treatment2 while doctors from Cuba went to Italy to augment the staff shortage.
In addition, there has been a surge in mental health problems due to the pandemic (Rajkumar, 2020). For healthcare workers, this comes due to increased working hours, inadequate sleep and anxieties over contracting the virus from patients (Xiang et al., 2020). For the general public, several factors are at play, such as loss of employment or means of livelihood, confinement at home, anxiety over getting infected with the virus, etc. This leads to poor sleeping and feeding habits, weight gain, depressive behaviors and anxiety, as well as domestic violence and substance abuse.
The social care sector has also experienced severe difficulties in this period, with high fatalities and increased risk of the disease for elderly residents (Onder et al., 2020) in care homes. Shortage of PPEs and trained staff also implies that care homes are poorly equipped to deal with a pandemic of this nature. In all this, health and social care staff have been overworked and stressed, with some of them sadly losing their lives in the line of duty, leading to anxieties over their resilience, mental well-being, and the long-term implications for the health sector. Mobile communication and applications (Sait et al., 2019; Chowdhury et al., 2020), video-conferencing, and other information technology (IT) tools are helping to provide care outside hospitals during the pandemic (Chamola et al., 2020). Other systems rely on communication technologies for tracing and tracking, remote patient monitoring, COVID-19 diagnosis and telehealth services (Ding et al., 2020). We explore how 5G and other technologies can help stop COVID-19 in section 4.
2.2. Education
The COVID-19 pandemic has led to closure of schools in 153 countries around the world as at May 2020, affecting about 1.2 billion learners3. This has caused significant disruption in academic assessment and progression for many pupils and students. It has also left millions of international students stranded in foreign countries, where the lack of social contact with classmates, mounting anxiety over getting infected, closure of universities and halls of residence, as well as isolation from families is taking a mental health toll on them (Sahu, 2020).
According to the United Nations Educational, Scientific and Cultural Organization (UNESCO), the closure of schools has many negative consequences for learners and exacerbates the inequality in education between the rich and the poor. The pandemic has disrupted opportunities for growth and learning, especially for disadvantaged learners, which has been proven to lead to loss of cognitive skills (Carlsson et al., 2015), a primary aim of education. For students in final years, the pandemic has impacted their final assessments and employability as they will be graduating at a time of major global economic recession.
The impact on children's education include high levels of post-traumatic stress compared to adults, poor dietary habits, reduced physical activity, poor sleeping habits, poor cardiorespiratory fitness (Wang et al., 2020b), as well as poorer nutrition for disadvantaged children that rely on school meals for healthy diets. In addition, children from poorer backgrounds suffer disproportionately as many of them do not have computers and other electronic gadgets required for online learning, and their parents are more unprepared for the task of continuing their education without teachers compared to their peers from wealthier and more educated homes. These effects altogether lead to spikes in risky behaviors, unhealthy peer influences and substance abuse. For parents and families, childcare responsibilities and home tutoring has forced many to stop working, which impacts the family income and further reduces the healthcare workforce. Many parents are also ill-equipped and prepared for teaching their children at home when schools are closed. There will be significant differences in children's learning according to the availability of parents and their skills and education (Burgess and Sievertsen, 2020).
The overall consequences of the closure of schools include financial loss for families forced to stop working due to childcare responsibilities, spike in risky behaviors, unhealthy peer influences and substance abuse for children and young adults, increased pressure on the healthcare system due to staff shortage4, social isolation and loneliness for learners of all age, depression, anxiety, chronic stress, and insomnia (Banerjee and Rai, 2020), spike in violence, sexual exploitation, child labor, and higher risk of dropping out due to loss of financial capability for many parents and guardians.
2.3. Travel, Tourism, and Aviation
This is one of the most adversely affected sectors of the economy. Travel restrictions have now been implemented in 100% of global destinations5, leading to a reduction in the workforce in most economic sectors (Nicola et al., 2020). The travel bans have directly or indirectly led to loss of several billions of dollars from airlines and affiliated industries (Iacus et al., 2020). Global passenger volume has dropped almost to zero as flights were canceled to contain the spread of the virus. Passenger airlines have been the most impacted in the aviation industry, leading to several airlines furloughing or laying off some of their staff. Many airlines have also collapsed or gone into administration as nations around the world shut their borders and impose travel bans. Similarly, airports have been reeling under economic losses, estimated at $76.6 billion in 2020 (Gössling et al., 2020). The International Civil Aviation Organization estimates that there will be up to 60% drop in the number of seats offered by airlines, over 80% reduction in passenger traffic and more than $400 billion loss in operating revenue for airlines (Canada, 2020), depending on how much longer the pandemic lasts, the containment measures put in place by governments and the impact of the pandemic on other economic conditions.
While cargo airlines continue to airlift vital supplies, such as PPE from manufacturing hubs like China to different countries around the world, there has also been about 25% drop in air cargo capacity compared to the same period for 2019 (Canada, 2020). With planes parked at airports, there has been a significant drop in the demand for spare parts and new aircraft, with many airlines deferring the delivery of new aircraft and many others canceling previous orders. The International Air Transport Association estimates that the airline industry requires at least $200 billion in bailout funding to restart after the crisis6.
In the same vein, the tourism industry is forecast to lose about $450 billion, which is almost a third of the entire revenue generated by the industry in 2019, with up to 50 million jobs at risk globally (Nicola et al., 2020). The World Tourism Organization has noted that the tourism industry is one of the hardest hit by the current pandemic, and estimates up to 30% drop in international tourism receipts in 2020 (Gössling et al., 2020). This comes at the backdrop of cancellations of all non-essential travel and tours as governments shut down tourist destinations and place travel bans and visa restrictions in many countries. Despite the global tourism industry having weathered major shocks, such as the 9/11 terrorist attack in the US7, SARS, MERS, etc., there are indications that the impact of COVID-19 is unprecedented (Gössling et al., 2020) and that the economic damage is huge. In Scotland for instance, up to 99% of tourism businesses have reported that they experienced cancellations and decline in bookings. Hotel bookings have plummeted due to the restrictions in travel and cancellations of major scheduled global events.
2.4. Manufacturing and Distribution
The manufacturing industry has also been hard-hit by the COVID-19 pandemic as factories were shut down across the globe to protect staff who cannot work remotely and to curtail the spread of the virus. Worse still, the countries that are hardest-hit by the virus account for the highest percentage of global manufacturing (Baldwin and Tomiura, 2020). Due to the nature of manufacturing jobs, it is often difficult to implement social distancing rules, hence, manufacturing workers are highly vulnerable to the spread of the virus. Manufacturers of automobile, aircraft, chemical and electronics have been most impacted by the disruption in the global supply chain. For instance, China manufactures 90% of global computers and 70% of mobile phones, and practically all electronics products require components made in China8, nearly causing a halt in the production of these items in other countries even before COVID-19 was declared a pandemic due to global spread. About 60% of global active pharmaceutical ingredients is also produced in China (Ozili and Arun, 2020), leading to acute shortage of essential medicines all over the world when China went into lockdown due to COVID-19. This has consequently forced many companies to postpone the launch date for their products and also led to a rise in the cost of the components due to scarcity.
In addition, due to the interconnectedness of global manufacturing and the so-called global value chains where manufactured goods are shipped around key regions of the globe several times before reaching the final consumers, thousands of factories were forced to shut down even in countries that do not yet have COVID-19 due to disruptions in global supply chain. For instance, many car plants in Europe were shut down due to lack of spare parts as early as February when the virus was raging in China (Baldwin and Tomiura, 2020). The same trend was noticed in South Korea and the US. As the pandemic spread in Europe and the US, it caused even further dislocation in the global supply chain network. According to the European Association of Automobile Manufacturers, the United Kingdom and other countries in the European Union (EU) have suffered a loss of nearly two and a half million vehicles, with an average downtime of about 1 month as at the beginning of May 20209. However, manufacturers of medical products has seen growth despite the pandemic, which is unsurprising as they must rise to meet shortage of medical equipment and increased demand from hospitals around the world. Some companies have quickly retooled to respond to the shortage of life-saving products, such as hand sanitizers, ventilators and PPE. This has enabled several companies to keep the lights on and their employees to retain their jobs. Despite stimulus funds from many governments, the extended lockdown of plants, declining demand and loss in production and revenues will force some manufacturers to shut their doors permanently.
2.5. Agriculture and Food Production
The pandemic has disrupted food supply chains and kept farmers who should be producing food locked down at home. Also, reduced demand from hotels and the hospitality industry has led to spoilage of foods that cannot be preserved and loss of income to farmers (Nicola et al., 2020). In addition, poor network facilities in rural areas where most farmers are based (Chiaraviglio et al., 2017) make it difficult or impossible for them to implement remote farming. This situation is exacerbated in poor and developing countries where food production is still highly a manual operation and where preservation facilities are still in infancy. For many countries in sub-saharan Africa, the pandemic broke out just at the beginning of farming season following the cycle of annual rainfall, thereby making it impossible for farmers to plant at the right time, which might potentially lead to a famine in the coming year when the harvests are due. Due to the lockdown measures in place in many countries, the global food supply chain has been disrupted, cutting farmers off from essential farm supplies, such as chemicals, feed, and other farm inputs. It has also cut people off from vital supplies of essential food components, such as vitamins and proteins in countries that rely on importation of these items to supplement local supply. There are also fears that countries might adopt protectionist policies post-pandemic, choosing to strengthen self-sufficiency and discourage international agricultural engagement (Kerr, 2020), which might affect sufficiency and variety of food supply in dependent nations as well as upset the income of farmers.
2.6. Overall Impact on the Economy
As at 7th June 2020, there have been more than 6.7 million confirmed cases of COVID-19 and more than 390 thousand deaths in 216 countries, areas or territories10. This is a trying time for the global economy which continues to reel from the multi-sectoral impact of the virus. The healthcare bill has risen sharply as countries struggle to equip hospitals to cope with the pandemic. In addition, there has been a general decline in economic activities due to reduced earning capacity, and the restrictions on movement and social life, leading to a fall in demand for goods and services. The global economy is predicted to shrink by up to 3% due to the coronavirus pandemic (Fernandes, 2020). This is the result of shrinkages in sectors, such as hospitality, travel and aviation; manufacturing, supply chain and distribution; transportation and energy; consumer electronics as well as financial markets, some of which have already been highlighted above. Due to disruptions in manufacturing and supply chain, global trade has continued to decline and the World Trade Organization estimates up to 32% decline (Fernandes, 2020). The drop in global travel and aviation as well as fall in manufacturing has had a knock-on effect on the oil and gas industry, causing oil prices to plummet and leading several companies to reach for force majeure provisions in their contracts as conditions have rendered contractual performance impossible. For instance, Nigeria and Angola, the two largest producers of crude oil in Africa rely almost entirely on crude oil exports to finance their economy. Other large producers like Saudi Arabia have cut their national budget for 2020 and raised VAT due to the coronavirus.
Already, Germany which is Europe's largest economy has gone into recession (Michelsen et al., 2020) after its economy contracted by 2.2% in the first quarter of 2020. This follows France, Italy and other European countries that slid into recession earlier as the pandemic took toll and sub-zero growth was recorded for many economies around the world. The above outcomes have resulted in soaring unemployment as businesses shut down or collapse and organizations struggle to remain afloat. It is estimated that job losses could be up to 30% in the US alone by the second quarter of 2020 (Jackson et al., 2020), while one in six workers in the UK could become unemployed due to the COVID-19 pandemic11. Nearly 40 million people have become unemployed in the US alone as at 14th May 2020. In Europe, over 30 million people are also relying on government support for salaries and wages in the UK, Germany, Spain, France and Italy (Jackson et al., 2020). Employment support currently costs the UK government £14 billion per month.
The COVID-19 pandemic is threatening to reverse the economic progress made in the last decade to pull millions of people in developing economies out of poverty and reduce economic disparity. The United Nations Conference on Trade and Investment (UNCTAD) predicts that foreign direct investment could drop by as much as 15%, which has dire consequences for developing economies (Barua, 2020). African countries are particularly hit due to their limited financial resources and poorly equipped health systems where many households have to spend out of pocket for healthcare products. The impact of the pandemic on some African economies has been predicted to last for several years to come. In Asia, about 29 million people are at the risk of falling into poverty due to the effects of the coronavirus. The economic damage of the pandemic will depend on how much governments and organizations are able to support businesses and economies to restart after the pandemic, and the level of adherence to public health guidance to curtail the virus. Containing similar pandemics in the future will require a holistic approach where all countries work together to strengthen health facilities in every country of the world as no country can be safe until every country is safe due to global interconnectedness (McKibbin and Fernando, 2020).
The place of wireless communications in general and 5G in particular in addressing the challenges posed by the new realities are being investigated by researchers (Ahmadi et al., 2020). In section 4, we provide insights into how the unique capabilities that 5G offer can be taken advantage of to contain the current pandemic and future-proof our society against future pandemics.
3. Impact of COVID-19 on Existing Networks
In this section, we briefly describe the challenges facing existing mobile networks because of their increased utilization by various sectors due to the disruptions to normal operations and shift to online platforms during the COVID-19 pandemic. Figure 2 summarizes the impact of COVID-19 pandemic on the existing mobile networks.
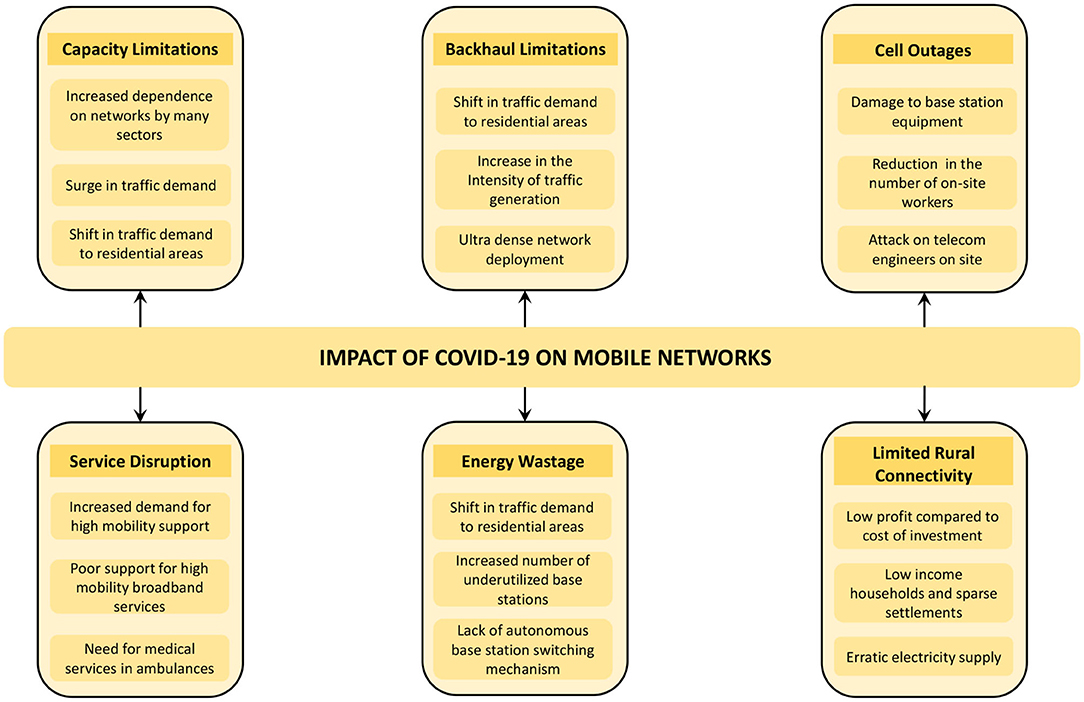
Figure 2. The figure summarizes some of the networking related challenges facing existing networks due to COVID-19 pandemic.
3.1. Capacity Limitations
The change in traffic demand because of sudden increased dependence of several sectors on both mobile and fixed networks as well as the abrupt shift in traffic demand from city centers to residential areas have taken service providers unawares. Networks in residential areas were not optimized to handle surges in traffic demand as heavy traffic demand have not been placed on them over time because most activities demanding huge network resources are mainly carried out at work places which are mainly located in city centers. However, with the shift to remote working, there is an unprecedented increase in the traffic demands, thereby putting much pressure on the limited capacity of the RANs in residential areas.
This issue might not be prevalent in developed countries as most areas have fixed broadband internet services, however, in developing countries, there would be a surge in traffic demand on the RAN, which might result in network congestion, low data rates, increased latency and poor connections, thereby making it difficult to carry out remote operations. Therefore, capacity optimization techniques (Zhang, 2019; Barakabitze et al., 2020) that will enable dynamic resource adaptation to changing traffic demands as well as other capacity-enhancing technologies (Yaacoub et al., 2016) need to be developed and optimized to improve the quality of service in these areas.
3.2. Backhaul Limitations
With the shift in traffic demand from city centers to residential areas, there would be a surge in traffic generation in residential areas due to increased use of multimedia services, such as online gaming, video streaming, video conferencing, etc., thereby putting much pressure on the limited backhaul capacity of both fixed broadband and cellular networks which are not optimized to support such drastic surges in traffic demand. For example, in UDNs where a very large number of small cells (SC) are deployed under the coverage area of a macro cell, the issue of backhaul capacity becomes even more challenging than RAN capacity, as there may not be sufficient capacity in the backhaul to successfully route the volume of traffic generated from the SCs to the macro cell as well as the core network (Gao et al., 2015; Adedoyin and Falowo, 2020). This could result in backhaul congestion, thereby leading to increased latency and poor quality of service. Hence, there is a need explore various backhaul optimization techniques (Zeydan et al., 2016) as well as other backhaul capacity boosting technologies (Jaber et al., 2016a) that will enable the reduction of traffic congestion on the backhaul.
3.3. Cell Outages
Conspiracy theories12 and misinformation connecting 5G with the spread of COVID-19 (Ahmed et al., 2020) have led to the destruction of several BSs as well as attacks on telecoms engineers in different countries around the world. It is reported that about 77 BSs has so far been attacked in the UK alone13. Also, with the emphasis on working from home in order to reduce the spread of the infection and to ensure the safety of workers on site, fewer workers are being engaged for site operations, hence the detection and restoration to normal operations of cells in outage may be quite challenging this period. All these would result in network failure and service degradation if proactive measures are not put in place to mitigate the effect of these cell outages. Therefore, it is necessary to fully incorporate measures for the network to automatically detect and restore faulty BSs or reconfigure the network (Aliu et al., 2013; Klaine et al., 2017) in case of damaged BSs to prevent prolonged service degradation or network failure.
3.4. Service Disruptions
There is an increasing demand for high mobility support to prevent service disruptions or intermittent quality of service for highly mobile users, such as those in moving vehicles, trains, etc., especially during this pandemic where there might be need for remote medical services in case of emergency situations where video connections is required between the ambulance paramedics and experts in hospitals (Usman et al., 2019). However, with existing networks, these connections will be prone to frequent disruptions arising from insufficient support for high mobility broadband services. Improved mobility management (Imran et al., 2019a; Ozturk et al., 2019; Tabassum et al., 2019) and proactive handover (HO) optimization techniques (Ahmed and Alzahrani, 2019; Mollel et al., 2019; Mollel et al., 2020) are required for consistent quality of service along highways or speed train routes.
3.5. Energy Wastage
One of the major challenges facing mobile network operators is the need to reduce the energy consumed by BSs. This is because increased energy consumption leads to higher network operating costs (i.e., energy bills), and environmental pollution due to increase in CO2 emissions (Buzzi et al., 2016). The COVID-19 pandemic has resulted in a shift in user traffic demand from city centers to residential areas, as many have to work, continue academic activities or attend conferences, seminars and business meetings, from home. As a result, BSs in city centers are underutilized as they are constantly kept on despite the fact that very little or no traffic demand is placed on them. This amounts to energy wastage and financial loss on the part of the network operators. Hence, there is a need to develop intelligent traffic prediction and load adaptive cell switching techniques (Feng et al., 2017; Abubakar et al., 2019; Asad et al., 2019), such that the traffic demand on the network can be continually monitored to identify underutilized BSs and automatically switch them off. This will result in significant energy savings and reduction in electricity bills, particularly during the pandemic.
3.6. Limited Rural Connectivity
Rural areas are the most affected by the pandemic due to limited connectivity and poor coverage by fixed broadband and mobile networks. This is because such areas mainly comprise low income households, sparse settlements and mostly erratic electricity supply (for developing countries), coupled with the perceived low profit compared to the investment required for broadband wired or wireless connectivity on the part of network operators (Chiaraviglio et al., 2017). This makes life really difficult for rural dwellers during this period as the available networks may not be able to handle the broadband requirements for online working and learning.
In addition, incidences of panic buying14—due to insinuations regarding the possibility of food shortage as a result of movement restrictions and shutdown of many factories—has led to a drastic increase in the demand for food items during the pandemic. However, with the stay-at-home policy in place, farming activities have been affected because farmers are unable to access their farms regularly, which might result in decline in food production. In this regard, limited connectivity in rural areas has adverse effect on the productivity of farmers who would have been able to leverage internet-based technologies, such as IoT networks to facilitate smart farming activities, such as irrigation, detection of diseases in plant leaves, and collection and analysis of relevant information from their farm (Yaacoub and Alouini, 2020) in order to sustain and enhance food production. Therefore, there is a need to improve broadband penetration in rural areas through design and deployment of low cost networks (Khaturia et al., 2019) and the involvement of government in the subsidizing the network deployment cost (Chiaraviglio et al., 2017) by granting incentives to network operators to facilitate the extension of their networks to these areas.
4. How 5G Networks and Artificial Intelligence Can Help?
Wireless communication has revolutionized the way we live, play, and work. The COVID-19 pandemic has disrupted usual life and work patterns and caused severe economic losses. In Figure 3, we summarize the services required in each sector to address the challenges due to the current pandemic and the application(s) of 5G that is needed to support such service. The strength of 5G is modulated by AI, which gives networks autonomous capabilities. These capabilities allow the network to perform most functions without needing human support, such as configuring itself, adapting itself to changes in the network conditions and repairing faults when they occur.
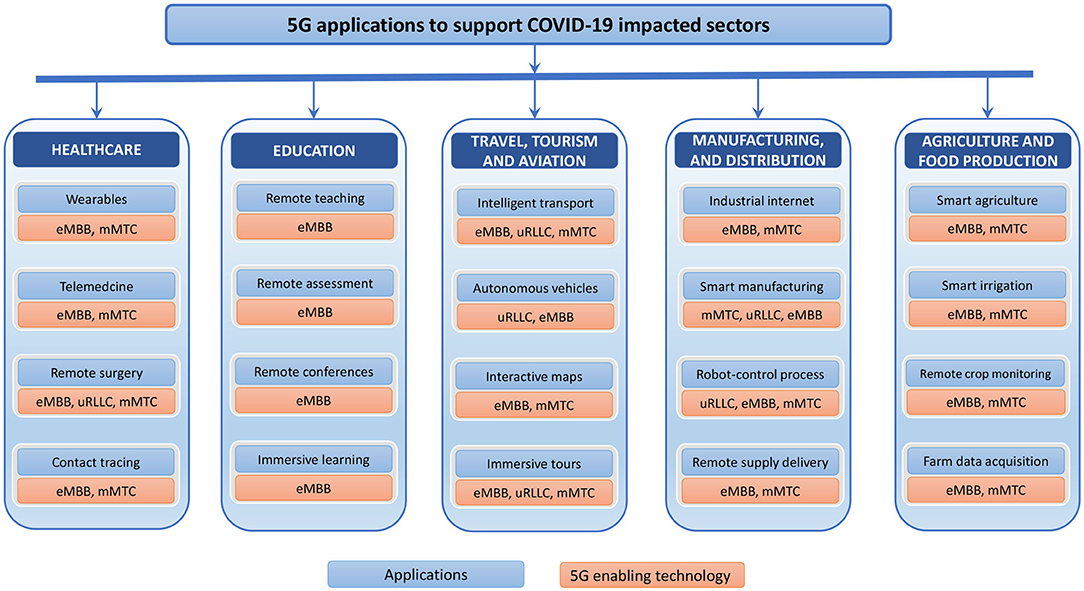
Figure 3. The role that 5G technologies (eMBB, URLLC, mMTC) play in various sectors to enable them handle the challenges caused by COVID-19 pandemic by supporting various operations or technologies in each sector.
AI techniques are usually data driven and as such the provision of support for massive device connectivity and IoT networks via mMTC services in 5G networks would make sufficient data available for the training and implementation of AI/ML models. In addition, the improvement in memory and processor technology as well as the inclusion of caching and edge computing in 5G would also assist in the deployment and utilization of AI technologies, since they are usually computationally intensive. Moreover, the software defined nature of 5G networks along with its associated architectures, such as Network function virtualization (NFV), network slicing, separation of data and control plane, etc., would also facilitate the use of AI methodologies for intelligent and dynamic network management and orchestration. More details on AI enabling technologies in 5G and B5G can be in found in Wu (2019), Xie et al. (2019), Bega et al. (2020), Challita et al. (2020), and Pham et al. (2020).
In this section, we first describe how 5G services (eMBB, URLLC, and mMTC), can be used to proffer solutions to some of the problems caused by the pandemic in various sectors followed by the role that AI/ML assisted SON would play in enabling 5G to tackle networking related challenges facing existing networks due to COVID-19 pandemic.
4.1. How 5G Networks Can Help?
Here, we highlight the characteristics of 5G as defined by 3GPP that can help in curtailing the spread of the COVID-19 pandemic and future pandemics. This covers three use-case categories, including eMBB, URLLC, and mMTC. eMBB is an evolution of the current 4G standard to support higher data rates and broadband anywhere as well as lower user-experienced latency, even at high mobility, such as in moving vehicles and trains. URLLC ensures extremely low latency, high availability, reliability, and security for mission-critical services, such as remote surgery or driverless vehicles. mMTC is concerned with providing cellular coverage for billions of low-power wide area network technologies, such as narrowband IoT devices. While 5G has a plethora of applications in virtually all areas of daily life, for the sake of brevity, we highlight only a few aspects relevant to dealing with the current COVID-19 outbreak and future pandemics.
In addition to the use-case categories highlighted below, 5G implements technologies, such as software-defined networking (Aliu et al., 2013), network function virtualization (Jaber et al., 2016a), network slicing, etc., to provide enhanced network functionalities, handle congestions, and increase backhaul capacity. For instance, network slicing makes it possible to carve out virtual networks from a common or shared physical infrastructure, which can swiftly be used to dynamically shift resources to enhance capacity in areas with increased demand without need for additional hardware or network reconfiguration (Zhang, 2019; Barakabitze et al., 2020). By using fiber optic connections between cell towers and switching centers, 5G has expanded backhaul capacity to accommodate expected increase in data traffic from different areas. In addition, software-defined networking paradigms and caching improve backhaul usage, as discussed in Jaber et al. (2016a). Dynamic BS switching can be adopted to ensure energy efficiency due to changing traffic loads as traffic shifts from city centers to residential areas (Abubakar et al., 2019). The advanced features that make 5G networks autonomous and dynamically adapt to traffic changes are fully explored in the cognitive networking discussion presented in the next subsection.
4.1.1. eMBB
5G has the capacity and tools required to meet the increased demand for data during the COVID-19 pandemic due to the rise in remote working, remote education, rising e-commerce, and increased mobile video streaming. Due to the shelter-in-place order in several countries around the globe, data demand has soared due to higher traffic through video streaming websites and applications, such as Netflix and YouTube, increase in video-conferencing, online collaboration, teaching and learning through applications, such as Zoom, Slack, Microsoft Teams, Cisco Webex, etc. However, the promise of broadband anywhere that 5G offers (Dahlman et al., 2014) can be taken advantage of to allow companies move fully to remote working even beyond the current pandemic. Distributed computing and cloud storage (Wang et al., 2012) are increasingly becoming the norm for many industries, which supports the vision of working safely from home or remote locations, which are necessary to maintain social and physical distancing as required to stop the spread of COVID-19. To ensure that people can work safely from anywhere, 5G provides the required network capacity and low latency (Parvez et al., 2018) to connect staff to their office network and ensure seamless team-working and communication. An aspect of 5G also includes a commitment by governments and operators in many countries15 to improve broadband access in remote areas (Khaturia et al., 2019), which can tackle the capacity issue in residential areas, even for those who live in rural areas.
In healthcare, 5G has many use cases defined specifically to provide next-generation care and safety (Ahad et al., 2019). A few examples include the use of wearable technologies coupled with wireless communications to remotely monitor vital signs in patients and report to experts, making it possible for them to reach more people by cutting down on consultation time and reducing hospital bed occupancy. This is an area that has seen a massive growth in recent times. In terms of the current COVID-19 pandemic, a remote contactless positioning system (Karanam et al., 2020) has already been developed for scanning patients, to remove the need for heatlhcare professionals to have contact with the patients, thereby preventing potential hospital infections. 5G provides the capacity to accommodate the increased demand due to exploding number of connected devices, as well as high reliability and low latency that may be required by some wearable applications, such as pacemakers. 5G can also be used to support tactile control communications (Sharma et al., 2020), to enable remote surgery and patient monitoring. Telemedicine is supported by 5G to provide consultations over the phone or via video, especially in remote areas where health facilities are often in inadequate supply. In the current pandemic, countries, such as China use 5G and 5G-enabled robots to provide care, disinfect surfaces, dispense drugs and measure temperatures in hospitals and public places, trace suspected cases, etc. (Chamola et al., 2020). It can also help break language barriers by providing real-time translation and transcription services to allow countries to copy tested solutions implemented by those affected early by the pandemic; this technique also makes it easy to provide public announcements simultaneously in multiple languages using drones. A common limitation of tools currently employed to combat the virus is the time-lag in accessing available data, with delays of up to 1 month or longer being common16, which makes it difficult to implement a speedy response to spatio-temporal distributions of the disease and predict areas that might be more prone to near-future spread. Again, the high speed, bandwidth, and software-defined features of 5G can bridge this time gap and ensure timely availability of data, potentially saving many lives.
Social life and interaction has also been distorted by the COVID-19 pandemic due to the shutting down of most sports and entertainment venues. Confinement at home leads to increased distress and unrest. Immersive virtual reality supported by 5G (Guo et al., 2020; Saeed et al., 2020) can bring our social life to our homes and redefine social interactions, making it possible for people to have deeper and richer social connections without physically meeting up. This can also be adapted for education and the enjoyment of sports, where immersive experiences can make teaching more real for students or provide sports ground immersive experience to sports fans at home. Virtual reality headsets are already used to provide immersive experiences in the gaming and entertainment industries. However, the volume of high resolution video and data frames required to achieve this cannot be supported by current wireless technology.
4.1.2. URLLC
This supports mission-critical services by ensuring zero perceived latency and extreme reliability communications. The COVID-19 pandemic has stretched the health systems of most countries and caused a surge in deaths arising from other ailments (Leon et al., 2020). Health facilities have been overwhelmed as overworked healthcare professionals struggled to contain the deluge of admissions, despite many countries asking citizens to stay at home and to visit the hospital only when absolutely necessary. This is an area where 5G can help to supplement hospitals and specialists' availability (Chamola et al., 2020) through telemedicine and remote consultation, remote surgery, robot-assisted drug dispensary, live-in robots and wearables. URLLC enables remote surgery, smart grids, industrial internet and autonomous transportation systems to communicate decisions and feedback with less than a few millisecond delays, as long delays could have disastrous consequences. Remote diagnosis enables monitoring and communication of patient vital signs with devices, such as electrocardiogram (ECG), blood pressure, temperature and sugar levels. Ultrafast feedback is often required if any of the vital signs triggers any alarms. Remote surgery can save lives in critical situations, such as in ambulances en route hospitals, disaster zones or developing countries with poor healthcare facilities. 5G is capable of providing high definition video and reliable voice communication to guide junior doctors to perform complicated operations. This has been used by some Doctors Without Borders team in the UK to guide colleagues through emergency surgeries in conflict regions like Syria and Yemen. Also, robotic equipment fitted with haptic feedback and control systems enable specialists to feel what the robot is feeling in realtime. Even in countries with advanced healthcare system, URLLC and enhanced broadband capacity will enable in-ambulance initial medical care by Emergency Medical Technicians (EMT) before arriving at a hospital (Usman et al., 2019).
Industrial IoT and manufacturing can be powered by 5G to ensure automated manufacturing and adherence to social distancing rules for staff who must be present on site. The integration of cyber-physical systems with wireless communications allows autonomous robots to collaborate in factories to control processes, achieve a set goal, and avoid collisions with one another and with human workers (Shi et al., 2019). By providing industrial internet based on URLLC, 5G can ensure reliable communication between factories, processes and power systems in manufacturing and distribution, using tactile feedback and control for remote operation (Cheng et al., 2018). These systems typically require <1 ms delay and extremely low block error rates, which current communications standards are incapable of satisfying. Similarly, unmanned vehicles will benefit from 5G URLLC to enable remote delivery of essential hospital supplies and goods. This is already widely used in many African countries where drones fly around programmed routes to deliver blood, medicines and even food17. The improved communication features of 5G compared to 4G will add flexibility to this pattern to exploit the full capabilities of autonomous vehicles and enhance response time for decision makers.
4.1.3. mMTC
By providing high capacity to connect everything that can be connected, 5G has been identified as a key technology to usher in the fourth industrial revolution (Li et al., 2018). The 5G specification (Rel 16) covers requirements for industrial, consumer and enterprise IoT, including time-sensitive networking (TSN), a service that ensures high reliability and low latency for factory automation. It is also possible to integrate current proprietary factory communication systems and other non-public networks into 5G. In addition to being a key enabler for smart manufacturing, research shows that the IoT devices and cyber-physical systems used in Industry 4.0 are being rapidly adapted for use in healthcare to provide automated homecare (Yang et al., 2020). These are all anchored on high-speed, low-latency, and high-bandwidth communication, such as provided by 5G. The International Telecommunication Union (ITU) provides requirements to allow for the massive increase in connected devices expected in the coming years, which may reach up to a million machine-type connections per square kilometer (Navarro-Ortiz et al., 2020). In the current pandemic, 5G can help to provide connectivity for collaborative robots and autonomous material movement to ensure workers keep a safe distance from their peers in factories and warehouses, as already used in China, Canada and some European countries (Chamola et al., 2020). Many mobile phones are now equipped with near field communication (NFC) capability to enable them interact with millions of wearable devices to monitor patient health in real time.
5G can be exploited to salvage the disruption to the global supply chain due to the COVID-19 pandemic by enabling smart logistics (Jangirala et al., 2019) to ensure timely delivery for both medical supplies and supplies needed for daily work and living. From factories, parts and components to delivery vehicles, sensors can be linked up to the 5G network to provide realtime status and operational health of all components in the value chain (Rao and Prasad, 2018). The 5G mMTC umbrella covers both critical applications required for industrial automation, autonomous vehicles and intelligent transport systems, energy, etc. (Navarro-Ortiz et al., 2020), as well as non-critical connections requiring low data rates and low power consumption.
Due to their communication ability, it is now possible to offload non-vital patient consultations to wearable devices and mobile applications that alert health officials when there is need for specialist intervention (Li et al., 2018; Ahad et al., 2019; Usman et al., 2019). Also, IoT devices are deployed in homes for the elderly to monitor the environment and call for help in emergencies (Pandey and Litoriya, 2020). In addition to using NFC or Bluetooth, many of the devices come equipped with internet communication protocols, enabling them to transmit their readings directly to company servers (Chamola et al., 2020), which can show maps of disease spread in real time. Examples include smart thermometers that are already used to monitor COVID-19 symptoms and track its spread as well as robots that are used to disinfect contaminated surfaces in hospitals and other public places. Others include different kinds of applications that can be used for pre-screening patients that show initial symptoms of COVID-19, similar to the application in Sait et al. (2019) used for screening for pneumonia using X-ray images; some of these applications also alert health authorities for follow up if specific symptoms are present. Massive connectivity makes it easier to track contacts with suspected cases and map regions prone to spread, as well as monitor adherence to social distancing rules. Mobile phone data (e.g., location where a particular user has been) are also used to track the movement of people and map the spread of the disease (Chamola et al., 2020; Jia et al., 2020; Xu et al., 2020).
Other applications include traffic monitoring, monitoring compliance to lockdown protocols and government instructions, smart city planning and directing traffic to enable emergency vehicles access the fastest routes to hospitals. 5G can benefit other sectors and help restart economies, in tourism, the construction industry, public transportation, etc. In the tourism industry, interactive maps can help tourists find their way to the most interesting sites. In addition, tourists who are unable to travel due to restrictions can enjoy the same effects, such as local smell, touch, language, noise of streets, and feel through immersive virtual tours, complete with the sounds of birds, streams, etc. Since a vaccine or cure is yet to be found for COVID-19, this approach could help the tourism industry with much-needed revenue and satisfy tour-goers amidst the lockdown. In public transportation, 5G capabilities can be used for contact tracing of persons who shared the same vehicle if someone in the vehicle tests positive for the virus. It can also be used to ensure that the required social distance is maintained on all public transport systems. The same applies to the construction industry where in addition to maintaining social distancing, virtual and augmented reality can be used to provide remote training to construction workers.
4.2. How Self-Organization Can Assist 5G Networks?
Thomas, et al. suggested a standard definition for cognitive networking in Thomas et al. (2005) as “a cognitive network has a cognitive process that can perceive current network conditions, and then plan, decide and act on those conditions. The network can learn from these adaptations and use them to make future decisions, all while taking into account end-to-end goals,” which matches with the cognitive loop introduced by Mitola III: observe, orient, plan, decide, act, and learn (Mitola, 2007). Therefore, it is quite self-explanatory from the definition in Thomas et al. (2005) and the cognitive circle in Mitola (2007) that a network is supposed to sense (observe/perceive) the given environment to understand the current circumstances, which is followed by an orientation via evaluating/analysing the environmental information obtained. The plan phase can be bypassed based on the urgency of the sensed information, but otherwise, alternative plans are prepared if it is regular information. Lastly, one of the alternative plans is chosen and the agent takes an action by executing the plan. The sensed information from the environment of interest also feeds the learning phase in order to make more informed decisions in the future with the help of experience.
In this regard, the idea of cognitive networking aims at bringing network automation to wireless communication systems through introducing self-control to make the systems more dynamic, agile, and efficient. In other words, since the primary goal is to minimize the human intervention in communication networks, the response time to any changes and/or faults in the systems is shortened, improving the dynamism and agility of the networks. Moreover, provided that human interventions render the management of communication systems more time-consuming and costly, reducing it would result in utilizing the resources more efficiently. In addition, this is also beneficial for cellular network operators, as their operational and capital expenditures are reduced, which in turn make their businesses more sustainable and profitable (Aliu et al., 2013; Klaine et al., 2017; Öztürk, 2020).
It is reported in Fortuna and Mohorcic (2009) that cognitive networking has characteristics of self-configuration, self-optimization, self-healing, and self-protection, the first three of which are in line with the phases of SON discussed in Aliu et al. (2013). Since self-protection could somehow be included in self-optimization and self-healing, the focus of this work will be the first three characteristics, which are common between cognitive networking (Fortuna and Mohorcic, 2009) and SON (Aliu et al., 2013) concepts. In Table 1, we summarize the networking challenges facing existing networks due to the pandemic, what is required to cope with this problems, and some the AI-driven solutions that are required to handle those challenges.
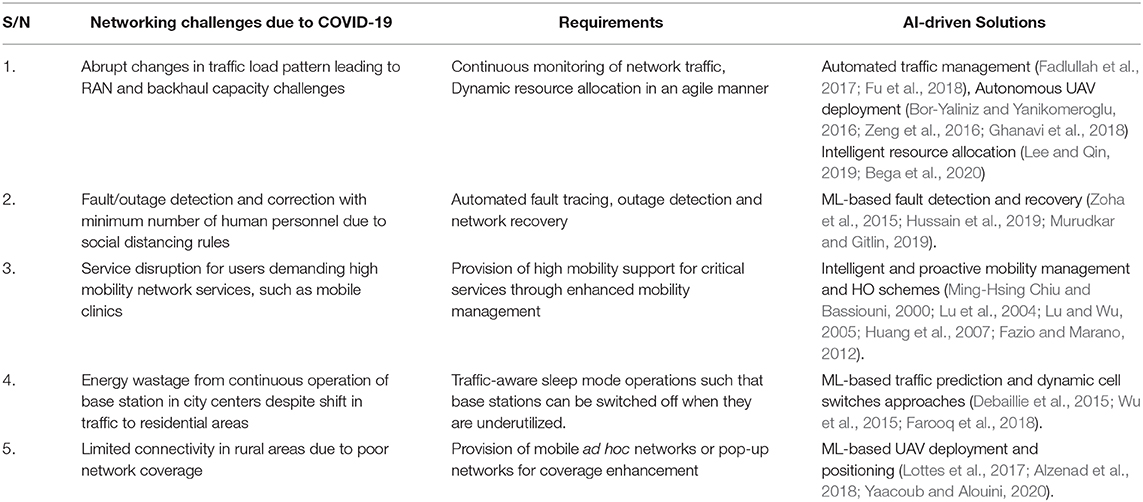
Table 1. The networking related challenges due to COVID-19, the requirements and some AI-based solutions.
4.2.1. Self-Configuration
From the network automation point of view, self-configuration is the process of configuring the initial parameters of BSs, which include IP settings, antenna radiation patterns, and neighbor lists, etc., whenever there are new BS deployments and/or changes in the network (Aliu et al., 2013). This process is crucially important when it comes to time and cost efficiency, since human intervention—which is the case in conventional networks—incurs monetary and time costs to cellular network operators. Besides, since engineers or technicians are more prone to making mistakes and errors due to the increased complexity of future cellular networks (Aliu et al., 2013), these costly configuration processes might be repeated multiple times, thereby amplifying the costs and undermining the sustainable business of operators.
4.2.2. Self-Optimization
After the initial configuration, a continuous monitoring of the system parameters is required to keep the communication system efficient and error-free. At this point, the concept of self-optimization plays a vital role by providing autonomous network optimization without—or minimal—external intervention. For example, HO management, energy efficiency, radio resource management, and load balancing are some of the domains that necessitate self-optimization (Aliu et al., 2013; Klaine et al., 2017). Given that wireless communication networks are quite dynamic due to environmental (e.g., weather, building constructions, etc.) and behavioral (e.g., different behaviors of users at different dates and times) changes, optimization is a never-ending process. However, the important point here is that while time-consuming conventional approaches would render the network optimization task highly inefficient, it could be made a lot easier and very efficient via automatic action sets.
4.2.3. Self-Healing
Self-healing—offering proactive fault detection in cellular networks alongside autonomous repair for identified issues—is a critical phase in SON. In conventional network management, the detection of failures and outages in systems mostly rely on customer complaints (Aliu et al., 2013; Asghar et al., 2018), such that once a complaint is received, engineers or technicians need to visit the corresponding site to identify and fix the problem. On the one hand, from the users' perspective, they might be left out of service or have service with reduced quality for a while, since both detection and correction take time. From the operator's point of view, on the other hand, this could harm their businesses, as cite visits by skilled human resources is costly, and unsatisfied users might switch to a different operator. Therefore, by utilizing self-healing, cellular networks become capable of autonomously detecting and fixing issues immediately after they occur, which subsequently mitigate the challenge by decreasing: (1) the dissatisfaction of users through quick and accurate actions, and (2) operating expenditure (OPEX) of the operators via eliminating human expert intervention (Aliu et al., 2013; Asghar et al., 2018).
4.3. How Can Artificial Intelligence Enable SON During the COVID-19 Outbreak?
Given that cellular networks have been gaining popularity and getting more complex from one generation to another (Gupta and Jha, 2015; Agiwal et al., 2016; Shafi et al., 2017; Busari et al., 2018; Morocho Cayamcela and Lim, 2018; Ericsson, 2019), cognition (intelligence) is required to address the ever-growing demands in more challenging scenarios.
Data is the main ingredient for intelligence, and fortunately, the amount of data generated by next generations of cellular networks will be much higher due to: (i) the prevalence of IoT devices; (ii) more BS deployments with network densification; (iii) more data transmitted by users; etc. (Xu et al., 2014; Agiwal et al., 2016; Bera et al., 2017; Lee et al., 2017; Akpakwu et al., 2018; Morocho Cayamcela and Lim, 2018). Learning, on the other hand, plays a crucial role in exploiting the huge amount of data, which subsequently enables cognitive networking. In that regard, ML—an important and promising subset of AI—has been gaining popularity in cellular network optimization.
4.3.1. Machine Learning as an Enabler
ML has a very broad range of applications in cellular networks from radio resource management to energy efficiency. This is because ML has strong optimization capabilities in addition to its adaptability in dynamic environments (Klaine et al., 2017; Imran et al., 2019b; Sun et al., 2019; Xie et al., 2019; Zhang and Dai, 2019; Zhang et al., 2019). At the system level in particular, ML application is more common due to the fact that the problems are case-specific and it is very hard to obtain generic solutions that can easily be adopted. Thus, the adaptability through learning makes ML a good candidate for optimizing these types of problems. Moreover, in cases where the optimal solutions are too complex to implement and/or not scalable, ML can help in reducing the complexity and making the solution more scalable (Xie et al., 2019; Björnson and Giselsson, 2020). However, to achieve this, the ML implementations should also be efficient and well-designed, otherwise it might contribute to the existing complexity. In addition, it is not a rule of thumb that ML implementations are always scalable; this can also be accomplished through proper and careful design. In the case of artificial neural networks, for instance, if the number of input or output neurons grows exponentially with the wireless network size, the solution cannot be said to be scalable.
The three main subcategories of ML, namely; supervised learning, unsupervised learning, and reinforcement learning, will be individually explored in the following paragraphs.
4.3.1.1. Supervised learning
This is a type of ML, where the data set consists of input and outputs—also referred to as labels. More specifically, a supervised learning algorithm is given inputs along with outputs in the training phase, and it is expected to develop a model that provides the relationship between them (Alpaydin, 2014). Then, in the testing phase the algorithm is only given new input data—which it has never seen during its training—and it predicts the associated output. The performance of the algorithm is evaluated by measuring how accurately it predicts; i.e., the actual and predicted outputs are compared18.
There are two major types of supervised learning: classification and regression. In classification, the outputs, which are given to the algorithms along with the inputs, consist of multiple classes, where each input is matched to an output class. Therefore, in the training phase, the algorithm tries to find a correlation between the input and output classes, so that when it is given new data, it can determine which class it belongs to. Object recognition is a typical example of this type of learning (Alpaydin, 2014), where the algorithm is trained with photos of different objects, such as clothes, mugs, books, etc., and their accurate labels. In contrast, in regression, the predicted output is continuous-valued instead of classes (discrete values). One good example could be determining the price of a laptop computer: while brand, processor speed, memory size, etc. are possible input features, outputs can be the prices of the computers. Hence, the algorithm can be trained with the given inputs and outputs in order to predict the price of a new computer when it is given the aforementioned input features.
4.3.1.2. Unsupervised learning
Unlike the case in supervised learning, where the data includes both input and output, the data set given to an unsupervised learning algorithm only consists of inputs. Instead, the algorithm is expected to reveal hidden patterns, regularities, or properties in a given input data (Alpaydin, 2014; Kubat, 2015). A good example for this type of learning is clustering, where the data is clustered into some groups based on their key features, and the unsupervised learning algorithm attempts to distinguish the clusters from each other with certain boundaries. However, unlike supervised learning, the identification of the clusters is not expected19 after clustering is performed.
Clustering finds broad applications in areas ranging from customer segmentation (Kansal et al., 2018) to agriculture (Mehta et al., 2015), but it also plays an important role in wireless communication networks. For instance, in wireless sensor networks (WSNs), clustering plays a vital role in detecting anomalies in a network (Zhang et al., 2010). Furthermore, unmanned aerial vehicles (UAVs) have gained significant attention in cellular networks to enhance the network capacity and to help in disaster scenarios (Klaine et al., 2018). In this regard, clustering can assist in positioning the UAVs, for example, in order to maximize the coverage or the number of users connected (Sun and Masouros, 2018).
4.3.1.3. Reinforcement learning
This is a distinctive class of ML, since it has inherent properties that distinguish it from supervised and unsupervised learning techniques. First of all, interacting with the environment-of-interest is the learning scheme adopted in reinforcement learning, whereas the learning is performed through labeled/unlabeled data in supervised/unsupervised learning (Sutton and Barto, 1998; Sun et al., 2019). In contrast to supervised and unsupervised learning, in reinforcement learning, the agent is expected to take actions by interacting with a given environment and evaluating the corresponding reward, which is aimed to be maximized (Sutton and Barto, 1998). Environment is something that produces an output for any action taken. In the case of cellular communication, for example, the environment could be the wireless network that consists of BSs and UEs with various channel conditions. In this regard, the actions that the agent takes could be a set of possible movements in the selected parameters. Taking the example of cellular networks and assuming a BS is the agent, the action set for the BS could be a range of HO margin values for the objective of load balancing. The agent goes into a state—the condition of the agent—after each action is taken, and continuing from the previous load balancing example, the agent could be in overloaded, fully-loaded, or under-loaded states based on the action taken.
As reported in Sutton and Barto (1998), there are four main elements that need to be discussed under reinforcement learning, namely policy, reward (or penalty/cost), value function, and model. Policy determines how the actions are taken by the agent, and constitutes the interface between the actions and the states (Sutton and Barto, 1998; Klaine et al., 2017). Reward function is a predefined function that is aimed to be maximized. From the optimization perspective, this can be interpreted as the objective function of the problem. According to the nature of the problem at hand, the reward function can sometimes be converted to a penalty function, in which the objective becomes minimization rather than maximization. There are two types of value function, namely state-value function and action-value function. The former indicates the expected value of visiting a state, whereas, the latter indicates the expected value of taking an action while in a particular state. Model is a representation of the environment of interest, which is utilized to comprehend how the environment reacts to actions taken by the agent (Sutton and Barto, 1998). Note that some reinforcement learning algorithms, such as Q-learning and SARSA, do not require a model, as they are executed in a model-free fashion (Sutton and Barto, 1998).
4.3.2. The Role of Machine Learning as an Enabler of SON
Well-designed ML solutions have gained considerable attention (Klaine et al., 2017; Imran et al., 2019b; Sun et al., 2019; Xie et al., 2019; Zhang and Dai, 2019; Zhang et al., 2019). Various types of ML implementations in different domains are available in the literature, and some recent examples related to the challenges due to COVID-19, as highlighted in section 3, will be discussed in the following paragraphs.
4.3.2.1. UAV assistance for capacity enhancement
UAVs have already been identified as a promising solution for wireless networking due to their multiple advantages including flexibility and mobility (Mozaffari et al., 2019). They can be used for various purposes, such as capacity enhancement—especially for UDNs—and emergency scenarios; e.g., earthquakes, fires, etc. Typically an SC is mounted on a UAV in order for it to act as a flying BS, which have multiple advantages over conventional ground SC deployments:
• They are deployed in the sky, thereby it is more likely to establish line-of-sight (LOS) with users (Zeng et al., 2016), which, by its turn, enhances the capacity of the network and provides improved data rates to the users;
• They are mobile, hence they can adjust their positions in terms of not only longitude and latitude but also altitude in order to provide better QoS in changing conditions;
• They can be deployed on demand, thus they are cost effective as they can be utilized multiple times at different locations/scenarios (Bor-Yaliniz and Yanikomeroglu, 2016; Zeng et al., 2016). In other words, rather than deploying fixed ground SCs by considering the peak traffic around the geographic area, UAVs can be deployed when needed. This provides not only reduced deployment costs for mobile network operators but also brings flexibility to cellular networks.
In that regard, the authors in Ghanavi et al. (2018) employ reinforcement learning in order to position a UAV to maintain or enhance the level of QoS offered by the ground network, such that the ground network is operating as normal in the considered scenario. Another reinforcement learning-based UAV positioning algorithm is proposed in Klaine et al. (2018). In particular, the authors design a UAV-assisted cellular network in the case of emergency, where existing cellular infrastructure may be out of service due to the experienced damage. A Q-learning based positioning of multiple UAVs, on which SCs are mounted, is aimed at increasing the number of users served by the UAV SCs. Even though their work is developed for an emergency scenario, it also proves that UAVs can supply additional capacity to cellular networks.
4.3.2.2. Backhaul optimization
Backhauling is one of the major problems for future generations of cellular networks due to the increased data rate demand as well as the dramatic inflation in the number of connected devices. For UDNs in particular where the intensity of the devices and BSs are comparatively much higher, the bottleneck is expected to appear more in the backhaul rather than the access network (Chia et al., 2009). To this end, ML is one of the important techniques used in the literature in backhaul optimization, since it offers intelligent backhauling solutions that can eventually help resolve the issues (Jaber et al., 2016a,b). Based on that, a user-centric backhaul optimization technique is presented in Jaber et al. (2016b), wherein the authors proposed to optimize the cell-range extension offset for user-cell association by considering the requirements of the users along with RAN and backhaul conditions. In particular, a Q-learning algorithm is designed to solve the given optimization problem, such that the system throughput is maximized while the user quality-of-experience is maintained.
4.3.2.3. Intelligent cell outage detection
ML is also a viable and robust tool for anomaly detection in cellular networks. Cell outages, for example, can be challenging issues to combat, since it is hard to identify the problems. Mobile network operators often rely on the client complaints (Aliu et al., 2013; Asghar et al., 2018), which delays the repair and are not always reliable. However, through ML implementations, the identification of cell outages can be done faster and more reliably (Hussain et al., 2019; Murudkar and Gitlin, 2019), which in turn helps mobile network operators in reducing their OPEX, while keeping users satisfied. The authors in Zoha et al. (2015) proposed a learning-assisted cell-outage detection and recovery approach, in which they utilize supervised and unsupervised learning algorithms to detect failures, while a fuzzy-based reinforcement learning is developed for the recovery part, which is performed by adjusting the power and antenna parameters accordingly. Moreover, a deep learning model for anomaly detection is investigated in Hussain et al. (2019), wherein real-life call detail record data set from Milan City, Italy is used. The authors adopted the mobile edge computing concept and achieved a promising detection accuracy of more than 98%.
4.3.2.4. Predictive mobility management to avoid service disruptions
In cellular communication networks, UEs perform measurements on some signal quality indicators; e.g., signal-to-interference-plus-noise ratio (SINR), received signal strength indicator (RSSI), and reference signal received power (RSRP), from both serving and neighboring BSs in an effort to keep their signal qualities above a certain level before deciding whether an HO is required. Upon a decision to HO after meeting multiple conditions, including hysteresis and time-to-trigger (TTT), certain steps are required, which can be classified under three main phases, namely; preparation, execution, and completion (Tayyab et al., 2019). Nonetheless, these phases and steps take some time and cause HO latency as well as signaling overheads (Mohamed et al., 2015; Ozturk et al., 2017b). Therefore, predictive HO schemes have been proposed in the literature to overcome these problems (Ming-Hsing Chiu and Bassiouni, 2000; Lu et al., 2004; Lu and Wu, 2005; Huang et al., 2007; Fazio and Marano, 2012; Zhang and Dai, 2019). Mobility predictions indeed have been identified as a valid and strong tool to manage resources in cellular networks in terms of radio, in-built (e.g., processing power, memory, etc.), energy, etc., as discussed in Imran et al. (2019a).
These schemes mainly try to predict future HOs of users in order to perform some of the aforementioned HO steps prior to the occurrence of the HO so that the HO signaling cost can be reduced. Even though these predictions can be provided by various methods, including statistical analysis, ML, and data mining algorithms, ML is the most commonly employed technique (Zhang and Dai, 2019). In this regard, the works in Ozturk et al. (2017b,a, 2018), and Farooq and Imran (2017) proposed predictive mobility management schemes to minimize HO signaling costs, which in turn reduces service disruptions.
4.3.2.5. Energy efficient networking
Various techniques for energy saving in cellular communication networks are outlined in Wu et al. (2015), including; hardware-based energy efficiency enhancement, component switching off, radio transmission-based energy efficiency improvement, use of renewable energy resources, and making the network more heterogeneous. Of all these techniques, while the use of renewable energy sources can be classified as an energy harvesting method, the rest would be in the category of energy conservation.
In the aforementioned energy conservation techniques, component switching off has a special place due to the following reasons;
• Even after implementing all the other techniques, such as advanced hardware and transmission efficiency, and increased network heterogeneity, there is still room for saving energy through putting components to sleep when they are under or not utilized.
• Enhancing the energy efficiency of hardware components and making the network more heterogeneous either requires new deployments or introduces new problems (Wu et al., 2015). Radio transmission optimization, for example, would require new wireless communication standards and/or concepts. However, cell switching can be implemented without extensive new replacements or communication standards, thus plugging it into the existing cellular networks is quite straightforward (Wu et al., 2015).
To this end, the underlying idea of cell switching is to deactivate components of a BS at low traffic, which is usually referred as putting the BS in a sleep mode. Various types of sleep modes have already been identified in Debaillie et al. (2015), whereby the sleep mode changes based on the depth of sleep that is correlated with the deactivation time of the component being switched off. In particular, the components in a BS are categorized according to their deactivation times in Debaillie et al. (2015), and the depth of sleep determines the components to be put to sleep; more components are put into the sleep mode with increasing depth.
An interesting idea is proposed in Farooq et al. (2018), where the HO traces of users are utilized to perform cell switching decisions. As such, rather than relying on the observations on traffic variations, the authors developed a model that predicts user HOs to determine the on/off status of a cell. More specifically, in the developed model, future traffic loads of SCs are determined through HO predictions, which are enabled by a semi-Markov process. Based on the estimated future loads, an optimization problem of minimizing the energy consumption is created, with constraints of minimum coverage and bit rate requirements.
In this regard, joint optimization of the following variables are conducted;
• optimum set of SCs to turn off/on for energy saving purposes,
• cell-specific offset for load balancing purposes, which subsequently helps maintain a good level of QoS.
4.3.2.6. Improved rural connectivity
As mentioned in section 3.6, rural connectivity has been one of the key elements during COVID-19 outbreak. In this regard, UAV assistance, as elaborated in section 4.3.2.1, can be beneficial in rural connectivity as well. UAVs can enable and facilitate smart agriculture, for example, by collecting necessary data collected by IoT sensors and transferring them to the local populace through the Internet (Yaacoub and Alouini, 2020). Moreover, UAVs can also be utilized for backhauling purposes in rural areas instead of using other wired/wireless options that require costly deployments (Alzenad et al., 2018; Yaacoub and Alouini, 2020). They are also good solutions for irregular on-demand capacity requirements, which are necessitated only by special events, such as the COVID-19 pandemic.
Based on this, the authors in Lottes et al. (2017) developed a classification problem for smart farming use-case, where camera-mounted UAVs are used to monitor the field in question and the developed ML algorithm—random forest is employed therein—classifies the plants as sugar beet crops and weeds. This is done in particular to focus on weed-dense areas in the field instead of using the required chemicals over the whole field with the same density. To that end, ML-assisted UAV deployments are discussed in Kouhdaragh et al. (2020), wherein different scenarios and challenges are elaborated for the UAV and ML-integrated connectivity solutions.
5. Preparing for Future Pandemics
The goal of this section is to give a brief insight into ways in which 5G assisted by AI can act as an enabler in predicting the outbreak of future pandemics by detecting early signs of rising cases of a particular infection in a region or country. In addition, we briefly discuss the role of 5G in making our society resilient to future pandemics through increased digitization and adoption of autonomous systems. This will result in less direct human involvement and increased support for remote operations in certain key sectors, such as health, education, government, manufacturing, and transportation, thereby making social distancing a part of our daily lives. Furthermore, we briefly highlight a few lessons learnt from COVID-19 pandemic that should be carefully considered in future network design.
5.1. Predicting/Detecting a Pandemic
Due to 5G support for IoT networks that enables the massive connectivity of different kinds of devices, such as smartphones, wearables, sensors, smart meters, etc. (Akpakwu et al., 2018; Ozturk et al., 2020), it will lead to enhancement in the acquisition of electronic records of patients in various countries which can be stored in the cloud (Yang et al., 2017). The electronic health records of these countries, those of international agencies, such as the World Health Organization (WHO), as well as other useful records of patients, such as their travel history, can be synchronized in order to obtain a more comprehensive big data. This comprehensive data can then be properly analyzed using ML models (Pham et al., 2020) to detect the onset, spread, and rise of various infections in different countries. The results of these analyses can enable prompt response and intervention to prevent outbreak and spread of infections to other regions within a country or from one country to another through proactive measures, such as movement restrictions, quarantine for those leaving affected areas and also fast-tracked treatment procedures. Such approaches were used in Taiwan to curtail the spread of COVID-19 right from the moment that the WHO raised alarm on the rising incidence of a pneumonia of an unknown source in Wuhan in December 2019 (Wang et al., 2020a).
5.2. Enhanced Digitization
There is a need for increased utilization of digital platforms, such as e-payments, e-governance, electronic IDs, electronic health records, online or distance learning, etc. (Atkinson et al., 2020), for working, transacting business, providing health services, teaching and learning. 5G will provide ubiquitous broadband services that will support both stationary and high mobility users in order to ensure seamless remote operations from any location and at any time via its enhanced mobile broadband services (Andrews et al., 2014). This will support the gradual incorporation of remote operations in various sectors so that in the case of future pandemics where a full scale migration is required, it would be much easier to adapt to such changes rather than the abrupt change in operations as is currently being experienced due to the COVID-19 pandemic.
5.3. Embracing Large-Scale Automation
5G provides integral support for mMTC and its diversity of applications, such as IoT (industrial IoT, medical IoT, etc.), device-to-device (D2D), vehicle-to-vehicle (V2V), and vehicle-to-anything (V2X) communications (Akpakwu et al., 2018; Ozturk et al., 2020). Also, AI has huge potentials for big data analysis and intelligent decision-making (Morocho-Cayamcela et al., 2019). These, alongside the advancement in the development and utilization of robots (Yang et al., 2020), can help facilitate the transformation of key sectors from conventional to smart operations. This will result in smart industries where manufacturing processes are carried out by robots and industrial IoT devices while monitoring and control is done remotely, smart transportation systems where autonomous vehicles and trains are used to move passengers from one place to another, and smart delivery systems where drones and robots are used to deliver food and drugs to various locations, etc. In addition, 5G networks will also help in providing the required connectivity that is needed to carry out remote surgeries where doctors can perform surgeries with the assistance of robots from remote locations and the use of virtual and augmented reality for immersive and more effective remote teaching and learning, conferences and business meetings (Cheng et al., 2018; Chamola et al., 2020; Elavarasan and Pugazhendhi, 2020; Saeed et al., 2020).
5.4. Lessons From Pandemic That Should Be Considered in the Design of Future Networks
The outbreak of the pandemic and the various measures that have been put in place to contain the spread of the infection as well as ensure the continuity of services via remote network-assisted operations provides a lot of lessons for us regarding the requirements of future network design. Two (2) of such requirements which we think are very crucial to the design of future networks are briefly discussed here:
• Resilience and Robustness: The future networks must be able to recover to stable functioning state after failure or network breakdown. They must also be designed in such a way that they can withstand any unseen challenges and failures (Ali-Tolppa et al., 2018). This is necessary because future networks would be required to handle ultra-reliable and mission critical services demands, such as remote surgery, driver-less vehicles, industrial control processes, etc. To achieve resilience and robustness in future networks, there would be a need for continuous monitoring of the system to detect faults, prompt response to network changes as well as immediate triggering of corrective measures to restore the network back to normal functionality. Redundancy must also be built into future networks to duplicate capacity thereby making multiple connections available such that as soon as one connection goes down or does not have enough capacity to handle a particular service demand, another connection can be activated or allocated to handle that service demands (Michalopoulos et al., 2017). AI will play a very vital role in ensuring real time network response to sudden changes in networks, fault tracing and network recovery, as well as the dynamic allocation of network resources on demand basis (Lee and Qin, 2019; Bega et al., 2020).
• Security and Privacy preservation: Future networks must be designed in such a way that they can provide adequate security against cyber-attacks. They must also be privacy preserving by ensuring that user data is properly encrypted in such a way that the user cannot be easily identified even when an unauthorized person gains access to such data (Ahmad et al., 2019; Khan et al., 2020). Since 5G and B5G networks are going to support various data intensive technologies, such as massive IoT, MTC, etc., they would be more prone to security challenges than previous generations of cellular networks. Hence, the security system of these networks have to be fully automated using AI, to ensure dynamic adjustment and adaptation to threats and security breaches (Benzaid and Taleb, 2020). In addition, the use of blockchain or distributed ledger technologies (Haddad et al., 2020) will also help to ensure the safe distribution of various information across the networks. The combination of AI and blockchain technologies would further strengthen the security of future networks and enable them to effectively handle the nature (e.g., critical, sensitive, etc.), and volume of information that will be sent across these networks (Azzaoui et al., 2020).
6. Conclusion
Both wired and wireless communications have played a major role in reducing the negative effects of the COVID-19 pandemic on various sectors, such as health, education, transportation, manufacturing, etc. Without these communication tools, the pandemic would have had a greater impact and might have brought many of these sectors to a halt. Therefore, in this paper, the role of 5G networks in containing the COVID-19 pandemic have been thoroughly discussed. The key sectors affected by the pandemic were first identified in order to provide an insight into the magnitude of the problems being encountered in these sectors. As these sectors now depend on telecommunication networks to support their remote operations, it has resulted in unprecedented traffic demands on existing networks. Hence, we briefly outlined some of the challenges facing existing networks in dealing with the sudden increase in service demand in order to ensure continuity of operations in these affected sectors. Most importantly, the enhanced capacity and more advanced technologies of 5G networks can tackle most of the pandemic-related challenges compared to existing networks. In this regard, we emphasized the role of 5G and its enabling technologies in solving COVID-19-related challenges as well as tackling the limitations of existing networks. Finally, to avoid being taken unawares by future pandemics, we presented a brief discussion on how 5G networks can be leveraged for predicting future pandemics as well as the development of a pandemic-resilient society through enhanced digitization and embrace of large-scale automation. A brief discussion on how the pandemic would influence the design of future networks was also presented.
Author Contributions
AA: writing, editing, and visualization. KO and MO: writing and editing. SH and MI: review and supervision. All authors contributed to the article and approved the submitted version.
Conflict of Interest
The authors declare that the research was conducted in the absence of any commercial or financial relationships that could be construed as a potential conflict of interest.
Acknowledgments
The authors declare that part of the content included in this review paper has previously appeared online, in the thesis report of one of the co-authors. We also declare that this is the only place where it has appeared. The authors acknowledge the funding support from the Tertiary Education Trust Fund (TETFund), and Petroleum Technology Development Fund (PTDF) of the Federal Republic of Nigeria as well as the support received from the Scotland 5G Centre.
Footnotes
1. ^More information can be found online at: https://www.ilo.org/global/topics/coronavirus/sectoral/lang-en/index.htm (accessed May 28, 2020).
2. ^News article available online at: https://news.sky.com/story/coronavirus-how-germany-is-helping-other-european-nations-transport-covid-19-patients-11970745 (accessed May 15, 2020).
3. ^Available online at: https://en.unesco.org/covid19/educationresponse (accessed May 13, 2020).
4. ^Some healthcare staff who have parenting responsibilities rely on schools to look after their children while they are working. With schools closed, such staff are forced to stay at home and look after their children, thereby increasing the pressure on their colleagues who must work extra hours to cover for the absentee staff members.
5. ^Available online at: https://www.unwto.org/news/covid-19-travel-restrictions (accessed May 24, 2020).
6. ^Available online at: https://www.iata.org/en/pressroom/pr/2020-03-24-01/ (accessed May 24, 2020).
7. ^The terrorist attack at the World Trade Center in the US on September 11, 2001 caused serious damage to the American economy and led to a drastic reduction in air travel in the US.
8. ^Available online at: https://www.forbes.com/sites/ibm/2020/04/29/adapt-to-survive-covid-19-is-forcing-resilience-in-the-electronics-industry/ (accessed May 30, 2020).
9. ^Available online at: https://www.acea.be/news/article/interactive-map-production-impact-of-covid-19-on-the-european-auto-industry (accessed May 13, 2020).
10. ^Available online at: https://covid19.who.int/ (accessed June 7, 2020).
11. ^News article available online at: https://www.mirror.co.uk/news/uk-news/one-six-brits-could-lose-21849752 (accessed May 27, 2020).
12. ^Available online at: https://fullfact.org/online/5g-and-coronavirus-conspiracy-theories-came/ (accessed May 29, 2020).
13. ^Available online at: https://www.rcrwireless.com/20200507/network-infrastructure/5g-covid-conspiracy-theory-claims-77-uk-phone-masts (accessed May 14, 2020).
14. ^The corresponding media coverage is available online at: https://www.bbc.com/worklife/article/20200304-coronavirus-covid-19-update-why-people-are-stockpiling (accessed May 20, 2020).
15. ^More information can be found online at: https://www.ispreview.co.uk/index.php/2020/03/operators-sign-1bn-deal-for-95-geographic-uk-4g-cover-by-2026.html (accessed May 13, 2020).
16. ^Available online at: https://www.health.org.uk/news-and-comment/blogs/covid-19-five-dimensions-of-impact (accessed May 20, 2020).
17. ^News article available online at: https://www.ft.com/content/b24bdec8-65d2-11e9-9adc-98bf1d35a056 (accessed May 25, 2020).
18. ^The prediction accuracy can be measured during both training and testing phases, but they would serve for different purposes. For example, the case of high training accuracy with low testing accuracy is a sign of over-fitting.
19. ^In supervised learning, the classes are labeled; for example, fruit, vegetable, etc. However, in unsupervised learning, there is no label for the clusters.
References
Abubakar, A. I., Ozturk, M., Hussain, S., and Imran, M. A. (2019). “Q-learning assisted energy-aware traffic offloading and cell switching in heterogeneous networks,” in 2019 IEEE 24th International Workshop on Computer Aided Modeling and Design of Communication Links and Networks (CAMAD) (Limassol), 1–6. doi: 10.1109/CAMAD.2019.8858474
Adedoyin, M. A., and Falowo, O. E. (2020). Combination of ultra-dense networks and other 5G enabling technologies: a survey. IEEE Access 8, 22893–22932. doi: 10.1109/ACCESS.2020.2969980
Agiwal, M., Roy, A., and Saxena, N. (2016). Next generation 5G wireless networks: a comprehensive survey. IEEE Commun. Surv. Tutor. 18, 1617–1655. doi: 10.1109/COMST.2016.2532458
Ahad, A., Tahir, M., and Yau, K. A. (2019). 5G-based smart healthcare network: architecture, taxonomy, challenges and future research directions. IEEE Access 7, 100747–100762. doi: 10.1109/ACCESS.2019.2930628
Ahmad, I., Shahabuddin, S., Kumar, T., Okwuibe, J., Gurtov, A., and Ylianttila, M. (2019). Security for 5G and beyond. IEEE Commun. Surv. Tutor. 21, 3682–3722. doi: 10.1109/COMST.2019.2916180
Ahmadi, H., Katzis, K., Shakir, M. Z., Arvaneh, M., and Gatherer, A. (2020). Wireless Communication and the Pandemic: The Story So Far. Available online at: https://www.comsoc.org/publications/ctn/wireless-communication-and-pandemic-story-so-far
Ahmed, A. A., and Alzahrani, A. A. (2019). A comprehensive survey on handover management for vehicular ad hoc network based on 5G mobile networks technology. Trans. Emerg. Telecommun. Technol. 30:e3546. doi: 10.1002/ett.3787
Ahmed, W., Vidal-Alaball, J., Downing, J., and López Seguí, F. (2020). COVID-19 and the 5G conspiracy theory: social network analysis of Twitter data. J. Med. Internet Res. 22:e19458. doi: 10.2196/19458
Akpakwu, G. A., Silva, B. J., Hancke, G. P., and Abu-Mahfouz, A. M. (2018). A survey on 5G networks for the internet of things: communication technologies and challenges. IEEE Access 6, 3619–3647. doi: 10.1109/ACCESS.2017.2779844
Ali-Tolppa, J., Kocsis, S., Schultz, B., Bodrog, L., and Kajo, M. (2018). “Self-healing and resilience in future 5G cognitive autonomous networks,” in 2018 ITU Kaleidoscope: Machine Learning for a 5G Future (ITU K) (Santa Fe), 1–8. doi: 10.23919/ITU-WT.2018.8598115
Aliu, O. G., Imran, A., Imran, M. A., and Evans, B. (2013). A survey of self organisation in future cellular networks. IEEE Commun. Surv. Tutor. 15, 336–361. doi: 10.1109/SURV.2012.021312.00116
Alzenad, M., Shakir, M. Z., Yanikomeroglu, H., and Alouini, M. (2018). FSO-Based vertical backhaul/fronthaul framework for 5G+ wireless networks. IEEE Commun. Mag. 56, 218–224. doi: 10.1109/MCOM.2017.1600735
Andrews, J. G., Buzzi, S., Choi, W., Hanly, S. V., Lozano, A., Soong, A. C. K., et al. (2014). What will 5G be? IEEE J. Select. Areas Commun. 32, 1065–1082. doi: 10.1109/JSAC.2014.2328098
Asad, S. M., Ozturk, M., Bin Rais, R. N., Zoha, A., Hussain, S., Abbasi, Q. H., et al. (2019). “Reinforcement learning driven energy efficient mobile communication and applications,” in 2019 IEEE International Symposium on Signal Processing and Information Technology (ISSPIT) (Ajman), 1–7. doi: 10.1109/ISSPIT47144.2019.9001888
Asghar, A., Farooq, H., and Imran, A. (2018). Self-healing in emerging cellular networks: review, challenges, and research directions. IEEE Commun. Surv. Tutor. 20, 1682–1709. doi: 10.1109/COMST.2018.2825786
Atkinson, R. D., Brake, D., Castro, D., and Ezell, S. (2020). Digital Policy for Physical Distancing: 28 Stimulus Proposals That Will Pay Long-Term Dividends. Technical report, Information Technology and Innovation Foundation.
Azzaoui, A. E., Singh, S. K., Pan, Y., and Park, J. H. (2020). Block5gintell: blockchain for ai-enabled 5G networks. IEEE Access 8, 145918–145935. doi: 10.1109/ACCESS.2020.3014356
Baldwin, R., and Tomiura, E. (2020). “Thinking ahead about the trade impact of COVID-19,” in Economics in the Time of COVID-19 (London: Centre for Economic Policy Research), 59–71.
Banerjee, D., and Rai, M. (2020). Social isolation in Covid-19: the impact of loneliness. Int. J. Soc. Psychiatry 66:0020764020922269. doi: 10.1177/0020764020922269
Barakabitze, A. A., Ahmad, A., Mijumbi, R., and Hines, A. (2020). 5G network slicing using SDN and NFV: a survey of taxonomy, architectures and future challenges. Comput. Netw. 167:106984. doi: 10.1016/j.comnet.2019.106984
Barua, S. (2020). Understanding coronanomics: the economic implications of the coronavirus (COVID-19) pandemic. SSRN Electron. J. doi: 10.2139/ssrn.3566477
Bega, D., Gramaglia, M., Garcia-Saavedra, A., Fiore, M., Banchs, A., and Costa-Perez, X. (2020). Network slicing meets artificial intelligence: an AI-based framework for slice management. IEEE Commun. Mag. 58, 32–38. doi: 10.1109/MCOM.001.1900653
Benzaid, C., and Taleb, T. (2020). AI for beyond 5G networks: a cyber-security defense or offense enabler? IEEE Netw. 1–8. doi: 10.1109/MNET.011.2000088
Bera, S., Misra, S., and Vasilakos, A. V. (2017). Software-defined networking for internet of things: a survey. IEEE Internet Things J. 4, 1994–2008. doi: 10.1109/JIOT.2017.2746186
Björnson, E., and Giselsson, P. (2020). Two applications of deep learning in the physical layer of communication systems. arXiv 2001.03350.
Bor-Yaliniz, I., and Yanikomeroglu, H. (2016). The new frontier in ran heterogeneity: multi-tier drone-cells. IEEE Commun. Mag. 54, 48–55. doi: 10.1109/MCOM.2016.1600178CM
Burgess, S., and Sievertsen, H. H. (2020). Schools, Skills, and Learning: The Impact of COVID-19 on Education. VoxEu.org.
Busari, S. A., Mumtaz, S., Al-Rubaye, S., and Rodriguez, J. (2018). 5G millimeter-wave mobile broadband: performance and challenges. IEEE Commun. Mag. 56, 137–143. doi: 10.1109/MCOM.2018.1700878
Buzzi, S., I, C., Klein, T. E., Poor, H. V., Yang, C., and Zappone, A. (2016). A survey of energy-efficient techniques for 5G networks and challenges ahead. IEEE J. Select. Areas Commun. 34, 697–709. doi: 10.1109/JSAC.2016.2550338
Canada, A. T. B. (2020). Effects of Novel Coronavirus (COVID-19) on Civil Aviation: Economic Impact Analysis. International Civil Aviation Organization (ICAO). Available online at: https://www.icao.int/sustainability/Documents/COVID-19/ICAO%20Coronavirus%202020%2004%2008%20Econ%20Impact.pdf
Carlsson, M., Dahl, G. B., Öckert, B., and Rooth, D.-O. (2015). The effect of schooling on cognitive skills. Rev. Econ. Stat. 97, 533–547. doi: 10.1162/REST_a_00501
Challita, U., Ryden, H., and Tullberg, H. (2020). When machine learning meets wireless cellular networks: deployment, challenges, and applications. IEEE Commun. Mag. 58, 12–18. doi: 10.1109/MCOM.001.1900664
Chamola, V., Hassija, V., Gupta, V., and Guizani, M. (2020). A comprehensive review of the COVID-19 pandemic and the role of IoT, drones, AI, blockchain and 5G in managing its impact. IEEE Access 8, 90225–90265. doi: 10.1109/ACCESS.2020.2992341
Chen, M., Challita, U., Saad, W., Yin, C., and Debbah, M. (2019). Artificial neural networks-based machine learning for wireless networks: a tutorial. IEEE Commun. Surv. Tutor. 21, 3039–3071. doi: 10.1109/COMST.2019.2926625
Cheng, J., Chen, W., Tao, F., and Lin, C.-L. (2018). Industrial IoT in 5G environment towards smart manufacturing. J. Ind. Inform. Integr. 10, 10–19. doi: 10.1016/j.jii.2018.04.001
Chia, S., Gasparroni, M., and Brick, P. (2009). The next challenge for cellular networks: backhaul. IEEE Micro. Mag. 10, 54–66. doi: 10.1109/MMM.2009.932832
Chiaraviglio, L., Blefari-Melazzi, N., Liu, W., Gutierrez, J. A., van de Beek, J., Birke, R., et al. (2017). Bringing 5G into rural and low-income areas: is it feasible? IEEE Commun. Standards Mag. 1, 50–57. doi: 10.1109/MCOMSTD.2017.1700023
Chih-Lin, I., Han, S., Xu, Z., Sun, Q., and Pan, Z. (2016). 5G: Rethink mobile communications for 2020+. Philos. Trans. R. Soc. A Math. Phys. Eng. Sci. 374:20140432. doi: 10.1098/rsta.2014.0432
Chowdhury, M. Z., Hossan, M. T., Shahjalal, M., Hasan, M. K., and Jang, Y. M. (2020). A new 5G ehealth architecture based on optical camera communication: an overview, prospects, and applications. IEEE Consum. Electron. Mag. 9, 23–33. doi: 10.1109/MCE.2020.2990383
Dahlman, E., Mildh, G., Parkvall, S., Peisa, J., Sachs, J., Selén, Y., et al. (2014). 5G wireless access: requirements and realization. IEEE Commun. Mag. 52, 42–47. doi: 10.1109/MCOM.2014.6979985
Debaillie, B., Desset, C., and Louagie, F. (2015). “A flexible and future-proof power model for cellular base stations,” in 2015 IEEE 81st Vehicular Technology Conference (VTC Spring) (Glasgow), 1–7. doi: 10.1109/VTCSpring.2015.7145603
Ding, X., Clifton, D., JI, N., Lovell, N. H., Bonato, P., Chen, W., et al. (2020). Wearable sensing and telehealth technology with potential applications in the coronavirus pandemic. IEEE Rev. Biomed. Eng. 1. doi: 10.1109/RBME.2020.2992838. [Epub ahead of print].
Elavarasan, R. M., and Pugazhendhi, R. (2020). Restructured society and environment: a review on potential technological strategies to control the COVID-19 pandemic. Sci. Total Environ. 725:138858. doi: 10.1016/j.scitotenv.2020.138858
Emanuel, E. J., Persad, G., Upshur, R., Thome, B., Parker, M., Glickman, A., et al. (2020). Fair allocation of scarce medical resources in the time of Covid-19. N. Engl. J. Med. 382, 2049–2055. doi: 10.1056/NEJMsb2005114
Ericsson (2019). Ericsson Mobility Report. Technical report. Ericsson. Available online at: https://www.ericsson.com/en/press-releases/2019/6/ericsson-mobility-report-5g-uptake-even-faster-than-expected
Fadlullah, Z. M., Tang, F., Mao, B., Kato, N., Akashi, O., Inoue, T., et al. (2017). State-of-the-art deep learning: evolving machine intelligence toward tomorrow's intelligent network traffic control systems. IEEE Commun. Surv. Tutor. 19, 2432–2455. doi: 10.1109/COMST.2017.2707140
Farooq, H., Asghar, A., and Imran, A. (2018). Mobility prediction-based autonomous proactive energy saving (AURORA) framework for emerging ultra-dense networks. IEEE Trans. Green Commun. Netw. 2, 958–971. doi: 10.1109/TGCN.2018.2858011
Farooq, H., and Imran, A. (2017). Spatiotemporal mobility prediction in proactive self-organizing cellular networks. IEEE Commun. Lett. 21, 370–373. doi: 10.1109/LCOMM.2016.2623276
Fazio, P., and Marano, S. (2012). “Mobility prediction and resource reservation in cellular networks with distributed markov chains,” in 2012 8th International Wireless Communications and Mobile Computing Conference (IWCMC) (Limassol), 878–882. doi: 10.1109/IWCMC.2012.6314320
Feng, M., Mao, S., and Jiang, T. (2017). Base station ON-OFF switching in 5G wireless networks: approaches and challenges. IEEE Wireless Commun. 24, 46–54. doi: 10.1109/MWC.2017.1600353
Fernandes, N. (2020). Economic Effects of Coronavirus Outbreak (COVID-19) on the World Economy. Available online at: https://ssrn.com/abstract=3557504
Fore, H. H. (2020). A wake-up call: COVID-19 and its impact on children's health and wellbeing. Lancet Glob. Health 8, e861–e862. doi: 10.1016/S2214-109X(20)30238-2
Fortuna, C., and Mohorcic, M. (2009). Trends in the development of communication networks: cognitive networks. Comput. Netw. 53, 1354–1376. doi: 10.1016/j.comnet.2009.01.002
Fu, Y., Wang, S., Wang, C., Hong, X., and McLaughlin, S. (2018). Artificial intelligence to manage network traffic of 5G wireless networks. IEEE Netw. 32, 58–64. doi: 10.1109/MNET.2018.1800115
Gao, Z., Dai, L., Mi, D., Wang, Z., Imran, M. A., and Shakir, M. Z. (2015). MmWave massive-MIMO-based wireless backhaul for the 5G ultra-dense network. IEEE Wireless Commun. 22, 13–21. doi: 10.1109/MWC.2015.7306533
Ghanavi, R., Kalantari, E., Sabbaghian, M., Yanikomeroglu, H., and Yongacoglu, A. (2018). “Efficient 3D aerial base station placement considering users mobility by reinforcement learning,” in 2018 IEEE Wireless Communications and Networking Conference (WCNC) (Barcelona: IEEE), 1–6. doi: 10.1109/WCNC.2018.8377340
Gössling, S., Scott, D., and Hall, C. M. (2020). Pandemics, tourism and global change: a rapid assessment of COVID-19. J. Sustain. Tourism 29, 1–20. doi: 10.1080/09669582.2020.1758708
Guo, F., Yu, R., Zhang, H., Ji, H., Leung, V. C. M., and Li, X. (2020). An adaptive wireless virtual reality framework in future wireless networks: a distributed learning approach. IEEE Trans. Vehicular Technol. 69, 8514–8528. doi: 10.1109/TVT.2020.2995877
Gupta, A., and Jha, R. K. (2015). A survey of 5G network: architecture and emerging technologies. IEEE Access 3, 1206–1232. doi: 10.1109/ACCESS.2015.2461602
Haddad, Z., Fouda, M. M., Mahmoud, M., and Abdallah, M. (2020). “Blockchain-based authentication for 5G networks,” in 2020 IEEE International Conference on Informatics, IoT, and Enabling Technologies (ICIoT) (Doha), 189–194. doi: 10.1109/ICIoT48696.2020.9089507
Huang, Q., Chan, S., and Zukerman, M. (2007). Improving handoff QoS with or without mobility prediction. Electron. Lett. 43, 534–535. doi: 10.1049/el:20070626
Hussain, B., Du, Q., Zhang, S., Imran, A., and Imran, M. A. (2019). Mobile edge computing-based data-driven deep learning framework for anomaly detection. IEEE Access 7, 137656–137667. doi: 10.1109/ACCESS.2019.2942485
Iacus, S. M., Natale, F., Santamaria, C., Spyratos, S., and Vespe, M. (2020). Estimating and projecting air passenger traffic during the COVID-19 coronavirus outbreak and its socio-economic impact. Saf. Sci. 129:104791. doi: 10.1016/j.ssci.2020.104791
Imran, A., Zoha, A., and Abu-Dayya, A. (2014). Challenges in 5G: how to empower SON with big data for enabling 5G. IEEE Netw. 28, 27–33. doi: 10.1109/MNET.2014.6963801
Imran, M., Ozturk, M., Abubakar, A. I., Klaine, P. V., Hussain, S., and Abbasi, Q. H. (2019a). Mobility prediction based resource management. Wiley 5G Ref. Essent. 5G Ref. Online 1–18. doi: 10.1002/9781119471509.w5GRef228
Imran, M. A., dos Reis, A. F., Brante, G., Klaine, P. V., and Souza, R. D. (2019b). Machine Learning in Energy Efficiency Optimization.
Jaber, M., Imran, M. A., Tafazolli, R., and Tukmanov, A. (2016a). 5G backhaul challenges and emerging research directions: a survey. IEEE Access 4, 1743–1766. doi: 10.1109/ACCESS.2016.2556011
Jaber, M., Imran, M. A., Tafazolli, R., and Tukmanov, A. (2016b). A distributed SON-based user-centric backhaul provisioning scheme. IEEE Access 4, 2314–2330. doi: 10.1109/ACCESS.2016.2566958
Jackson, J. K., Weiss, M. A., Schwarzenberg, A. B., and Nelson, R. M. Global Economic Effects of COVID-19. Congressional Research Service. Available online at: https://crsreports.congress.gov
Jangirala, S., Das, A. K., and Vasilakos, A. V. (2019). Designing secure lightweight blockchain-enabled RFID-based authentication protocol for supply chains in 5G mobile edge computing environment. IEEE Trans. Ind. Inform. 16, 7081–7093. doi: 10.1109/TII.2019.2942389
Jia, J. S., Lu, X., Yuan, Y., Xu, G., Jia, J., and Christakis, N. A. (2020). Population flow drives spatio-temporal distribution of COVID-19 in China. Nature 582, 389–394. doi: 10.1038/s41586-020-2284-y
Kansal, T., Bahuguna, S., Singh, V., and Choudhury, T. (2018). “Customer segmentation using K-means clustering,” in 2018 International Conference on Computational Techniques, Electronics and Mechanical Systems (CTEMS) (Belgaum), 135–139. doi: 10.1109/CTEMS.2018.8769171
Karanam, S., Li, R., Yang, F., Hu, W., Chen, T., and Wu, Z. (2020). Towards contactless patient positioning. IEEE Trans. Med. Imaging 39, 2701–2710. doi: 10.1109/TMI.2020.2991954
Kerr, W. A. (2020). The COVID-19 pandemic and agriculture–short and long run implications for international trade relations. Can. J. Agric. Econ. 68, 225–229. doi: 10.1111/cjag.12230
Khan, R., Kumar, P., Jayakody, D. N. K., and Liyanage, M. (2020). A survey on security and privacy of 5G technologies: potential solutions, recent advancements, and future directions. IEEE Commun. Surv. Tutor. 22, 196–248. doi: 10.1109/COMST.2019.2933899
Khaturia, M., Jha, P., and Karandikar, A. (2019). Connecting the unconnected: towards frugal 5G network architecture and standardization. arXiv 1902.01367. doi: 10.1109/MCOMSTD.001.1900006
Klaine, P. V., Imran, M. A., Onireti, O., and Souza, R. D. (2017). A survey of machine learning techniques applied to self-organizing cellular networks. IEEE Commun. Surv. Tutor. 19, 2392–2431. doi: 10.1109/COMST.2017.2727878
Klaine, P. V., Nadas, J. P. B., Souza, R. D., and Imran, M. A. (2018). Distributed drone base station positioning for emergency cellular networks using reinforcement learning. Cogn. Comput. 10, 790–804. doi: 10.1007/s12559-018-9559-8
Kouhdaragh, V., Verde, F., Gelli, G., and Abouei, J. (2020). On the application of machine learning to the design of UAV-based 5G radio access networks. Electronics 9:689. doi: 10.3390/electronics9040689
Lee, S., Bae, M., and Kim, H. (2017). Future of IoT networks: a survey. Appl. Sci. 7:1072. doi: 10.3390/app7101072
Lee, Y. L., and Qin, D. (2019). “A survey on applications of deep reinforcement learning in resource management for 5G heterogeneous networks,” in 2019 Asia-Pacific Signal and Information Processing Association Annual Summit and Conference (APSIPA ASC) (Lanzhou), 1856–1862. doi: 10.1109/APSIPAASC47483.2019.9023331
Leon, D. A., Shkolnikov, V. M., Smeeth, L., Magnus, P., Pechholdová, M., and Jarvis, C. I. (2020). COVID-19: a need for real-time monitoring of weekly excess deaths. Lancet 395:e81. doi: 10.1016/S0140-6736(20)30933-8
Letaief, K. B., Chen, W., Shi, Y., Zhang, J., and Zhang, Y. A. (2019). The roadmap to 6G: AI empowered wireless networks. IEEE Commun. Mag. 57, 84–90. doi: 10.1109/MCOM.2019.1900271
Li, R., Zhao, Z., Zhou, X., Ding, G., Chen, Y., Wang, Z., et al. (2017). Intelligent 5G: when cellular networks meet artificial intelligence. IEEE Wireless Commun. 24, 175–183. doi: 10.1109/MWC.2017.1600304WC
Li, S., Xu, L. D., and Zhao, S. (2018). 5G internet of things: a survey. J. Ind. Inform. Integr. 10, 1–9. doi: 10.1016/j.jii.2018.01.005
Lottes, P., Khanna, R., Pfeifer, J., Siegwart, R., and Stachniss, C. (2017). “UAV-based crop and weed classification for smart farming,” in 2017 IEEE International Conference on Robotics and Automation (ICRA) (Singapore), 3024–3031. doi: 10.1109/ICRA.2017.7989347
Lu, L. L., and Wu, J. C. (2005). “Handoff prediction by mobility characteristics in wireless broadband networks,” in Sixth IEEE International Symposium on a World of Wireless Mobile and Multimedia Networks (aormina-Giardini Naxos), 469–471.
Lu, L. L., Wu, J. C., and Chen, W. Y. (2004). “The study of handoff prediction schemes for resource reservation in mobile multimedia wireless networks,” in 18th International Conference on Advanced Information Networking and Applications, 2004. AINA 2004, Vol. 1 (Fukuoka), 379–384.
McKibbin, W. J., and Fernando, R. (2020). The Global Macroeconomic Impacts of COVID-19: Seven Scenarios. CAMA Working Paper No. 19/2020. doi: 10.2139/ssrn.3547729
Mehta, P., Shah, H., Kori, V., Vikani, V., Shukla, S., and Shenoy, M. (2015). “Survey of unsupervised machine learning algorithms on precision agricultural data,” in 2015 International Conference on Innovations in Information, Embedded and Communication Systems (ICIIECS) (Coimbatore), 1–8. doi: 10.1109/ICIIECS.2015.7193070
Michalopoulos, D. S., Gajic, B., Gallego-Nicasio Crespo, B., Gopalasingham, A., and Belschner, J. (2017). “Network resilience in virtualized architectures,” in Interactive Mobile Communication Technologies and Learning. IMCL 2017. Advances in Intelligent Systems and Computing, Vol. 725, eds M. Auer and T. Tsiatsos (Cham: Springer). doi: 10.1007/978-3-319-75175-7_81
Michelsen, C., Clemens, M., Hanisch, M., Junker, S., Kholodilin, K. A., and Schlaak, T. (2020). Coronavirus Plunges the German Economy Into Recession, DIW Weekly Report, Vol. 10. Berlin: Deutsches Institut für Wirtschaftsforschung (DIW), 184–190. doi: 10.18723/diw_dwr:2020-12-3
Ming-Hsing Chiu, and Bassiouni, M. A. (2000). Predictive schemes for handoff prioritization in cellular networks based on mobile positioning. IEEE J. Select. Areas Commun. 18, 510–522. doi: 10.1109/49.840208
Mitola, J. M. III. (2007). “Cognitive radio architecture,” in Cognitive Radio, Software Defined Radio, and Adaptive Wireless Systems, eds H. Arslan (Dordrecht: Springer Netherlands), 43–107.
Mohamed, A., Onireti, O., Hoseinitabatabaei, S. A., Imran, M., Imran, A., and Tafazolli, R. (2015). “Mobility prediction for handover management in cellular networks with control/data separation,” in 2015 IEEE International Conference on Communications (ICC) (London), 3939–3944. doi: 10.1109/ICC.2015.7248939
Mollel, M., Ozturk, M., Kisangiri, M., Kaijage, S., Onireti, O., Imran, M. A., et al. (2019). “Handover management in dense networks with coverage prediction from sparse networks,” in 2019 IEEE Wireless Communications and Networking Conference Workshop (WCNCW) (Marrakech), 1–6. doi: 10.1109/WCNCW.2019.8902854
Mollel, M. S., Abubakar, A. I., Ozturk, M., Kaijage, S., Kisangiri, M., Zoha, A., et al. (2020). Intelligent handover decision scheme using double deep reinforcement learning. Phys. Commun. 42:101133. doi: 10.1016/j.phycom.2020.101133
Morocho Cayamcela, M. E., and Lim, W. (2018). “Artificial intelligence in 5G technology: a survey,” in 2018 International Conference on Information and Communication Technology Convergence (ICTC) (Jeju), 860–865. doi: 10.1109/ICTC.2018.8539642
Morocho-Cayamcela, M. E., Lee, H., and Lim, W. (2019). Machine learning for 5G/B5G mobile and wireless communications: potential, limitations, and future directions. IEEE Access 7, 137184–137206. doi: 10.1109/ACCESS.2019.2942390
Mozaffari, M., Saad, W., Bennis, M., Nam, Y., and Debbah, M. (2019). A tutorial on UAVs for wireless networks: applications, challenges, and open problems. IEEE Commun. Surv. Tutor. 21, 2334–2360. doi: 10.1109/COMST.2019.2902862
Murudkar, C. V., and Gitlin, R. D. (2019). “QoE-driven anomaly detection in self-organizing mobile networks using machine learning,” in 2019 Wireless Telecommunications Symposium (WTS) (New York City, NY), 1–5. doi: 10.1109/WTS.2019.8715528
Mwanje, S., Decarreau, G., Mannweiler, C., Naseer-ul-Islam, M., and Schmelz, L. C. (2016). “Network management automation in 5G: challenges and opportunities,” in 2016 IEEE 27th Annual International Symposium on Personal, Indoor, and Mobile Radio Communications (PIMRC) (Valencia), 1–6. doi: 10.1109/PIMRC.2016.7794614
Navarro-Ortiz, J., Romero-Diaz, P., Sendra, S., Ameigeiras, P., Ramos-Munoz, J. J., and Lopez-Soler, J. M. (2020). A survey on 5G usage scenarios and traffic models. IEEE Commun. Surv. Tutor. 22, 905–929. doi: 10.1109/COMST.2020.2971781
Nicola, M., Alsafi, Z., Sohrabi, C., Kerwan, A., Al-Jabir, A., Iosifidis, C., et al. (2020). The socio-economic implications of the coronavirus pandemic (COVID-19): a review. Int. J. Surg. 78, 185–193. doi: 10.1016/j.ijsu.2020.04.018
Onder, G., Rezza, G., and Brusaferro, S. (2020). Case-fatality rate and characteristics of patients dying in relation to COVID-19 in Italy. JAMA 323, 1775–1776. doi: 10.1001/jama.2020.4683
Ozili, P. K., and Arun, T. (2020). Spillover of COVID-19: Impact on the Global Economy. Available online at: https://ssrn.com/abstract=3562570
Öztürk, M. (2020). Cognitive networking for next generation of cellular communication systems (Ph.D. thesis), University of Glasgow, Glasgow, United Kingdom.
Ozturk, M., Abubakar, A. I., Rais, R. N. B., Jaber, M., Hussain, S., and Imran, M. A. (2020). Context-aware wireless connectivity and processing unit optimization for IoT networks. arXiv 2005.00407.
Ozturk, M., Gogate, M., Onireti, O., Adeel, A., Hussain, A., and Imran, M. A. (2019). A novel deep learning driven, low-cost mobility prediction approach for 5G cellular networks: the case of the Control/Data Separation Architecture (CDSA). Neurocomputing 358, 479–489. doi: 10.1016/j.neucom.2019.01.031
Ozturk, M., Klaine, P. V., and Imran, M. A. (2017a). “3D transition matrix solution for a path dependency problem of Markov chains-based prediction in cellular networks,” in 2017 IEEE 86th Vehicular Technology Conference (VTC-Fall) (Toronto, ON), 1–5. doi: 10.1109/VTCFall.2017.8288350
Ozturk, M., Klaine, P. V., and Imran, M. A. (2017b). “Improvement on the performance of predictive handover management by setting a threshold,” in 2017 IEEE 86th Vehicular Technology Conference (VTC-Fall) (Toronto, ON), 1–5. doi: 10.1109/VTCFall.2017.8288355
Ozturk, M., Klaine, P. V., and Imran, M. A. (2018). “Introducing a novel minimum accuracy concept for predictive mobility management schemes,” in 2018 IEEE International Conference on Communications Workshops (ICC Workshops), 1–6. doi: 10.1109/ICCW.2018.8403507
Pandey, P., and Litoriya, R. (2020). Elderly care through unusual behavior detection: a disaster management approach using iot and intelligence. IBM J. Res. Dev. 64, 15:1–15:11. doi: 10.1147/JRD.2019.2947018
Parvez, I., Rahmati, A., Guvenc, I., Sarwat, A. I., and Dai, H. (2018). A survey on low latency towards 5G: RAN, core network and caching solutions. IEEE Commun. Surv. Tutor. 20, 3098–3130. doi: 10.1109/COMST.2018.2841349
Pham, Q., Fang, F., Ha, V. N., Piran, M. J., Le, M., Le, L. B., et al. (2020). A survey of multi-access edge computing in 5G and beyond: fundamentals, technology integration, and state-of-the-art. IEEE Access 8, 116974–117017. doi: 10.1109/ACCESS.2020.3001277
Pham, Q.-V., Nguyen, D. C., Hwang, W.-J., Pathirana, P. N., and Huynh-The, T. (2020). Artificial intelligence (AI) and big data for coronavirus (COVID-19) pandemic: a survey on the state-of-the-arts. Preprints 8:20202020040383. doi: 10.20944/preprints202004.0383.v1
Radi, G., Diotallevi, F., Campanati, A., and Offidani, A. (2020). Global coronavirus pandemic (2019-nCOV): implication for an Italian medium size dermatological clinic of a II level hospital. J. Eur. Acad. Dermatol. Venereol. 34, e213–e214. doi: 10.1111/jdv.16386
Rajkumar, R. P. (2020). Covid-19 and mental health: a review of the existing literature. Asian J. Psychiatry 52:102066. doi: 10.1016/j.ajp.2020.102066
Rao, S. K., and Prasad, R. (2018). Impact of 5G technologies on smart city implementation. Wireless Personal Commun. 100, 161–176. doi: 10.1007/s11277-018-5618-4
Saeed, N., Bader, A., Al-Naffouri, T. Y., and Alouini, M.-S. (2020). When wireless communication faces COVID-19: combating the pandemic and saving the economy. arXiv 2005.06637.
Sahu, P. (2020). Closure of universities due to Coronavirus Disease 2019 (COVID-19): impact on education and mental health of students and academic staff. Cureus 12:e7541. doi: 10.7759/cureus.7541
Sait, U., Shivakumar, S., K V, G. L., Kumar, T., Ravishankar, V. D., and Bhalla, K. (2019). “A mobile application for early diagnosis of pneumonia in the rural context,” in 2019 IEEE Global Humanitarian Technology Conference (GHTC) (Seattle, WA), 1–5. doi: 10.1109/GHTC46095.2019.9033048
Shafi, M., Molisch, A. F., Smith, P. J., Haustein, T., Zhu, P., De Silva, P., et al. (2017). 5G: a tutorial overview of standards, trials, challenges, deployment, and practice. IEEE J. Select. Areas Commun. 35, 1201–1221. doi: 10.1109/JSAC.2017.2692307
Shafin, R., Liu, L., Chandrasekhar, V., Chen, H., Reed, J., and Zhang, J. (2019). Artificial intelligence-enabled cellular networks: a critical path to beyond-5G and 6G. arXiv 1907.07862. doi: 10.1109/MWC.001.1900323
Sharma, S. K., Woungang, I., Anpalagan, A., and Chatzinotas, S. (2020). Toward tactile internet in beyond 5G era: recent advances, current issues, and future directions. IEEE Access 8, 56948–56991. doi: 10.1109/ACCESS.2020.2980369
Shi, Y., Han, Q., Shen, W., and Zhang, H. (2019). Potential applications of 5G communication technologies in collaborative intelligent manufacturing. IET Collab. Intell. Manuf. 1, 109–116. doi: 10.1049/iet-cim.2019.0007
Singh, R. P., Javaid, M., Kataria, R., Tyagi, M., Haleem, A., and Suman, R. (2020). Significant applications of virtual reality for covid-19 pandemic. Diabet. Metab. Syndr. Clin. Res. Revi. 14, 661–664. doi: 10.1016/j.dsx.2020.05.011
Sun, J., and Masouros, C. (2018). “Drone positioning for user coverage maximization,” in 2018 IEEE 29th Annual International Symposium on Personal, Indoor and Mobile Radio Communications (PIMRC) (Bologna), 318–322. doi: 10.1109/PIMRC.2018.8580746
Sun, Y., Peng, M., Zhou, Y., Huang, Y., and Mao, S. (2019). Application of machine learning in wireless networks: key techniques and open issues. IEEE Commun. Surv. Tutor. 21, 3072–3108. doi: 10.1109/COMST.2019.2924243
Sutton, R. S., and Barto, A. G. (1998). Reinforcement Learning: An Introduction, Vol. 1. Cambridge, MA: MIT Press.
Tabassum, H., Salehi, M., and Hossain, E. (2019). Fundamentals of mobility-aware performance characterization of cellular networks: a tutorial. IEEE Commun. Surv. Tutor. 21, 2288–2308. doi: 10.1109/COMST.2019.2907195
Tayyab, M., Gelabert, X., and Jäntti, R. (2019). A survey on handover management: from LTE to NR. IEEE Access 7, 118907–118930. doi: 10.1109/ACCESS.2019.2937405
Thomas, R. W., DaSilva, L. A., and MacKenzie, A. B. (2005). “Cognitive networks,” in First IEEE International Symposium on New Frontiers in Dynamic Spectrum Access Networks, 2005. DySPAN 2005 (Baltimore, MD), 352–360.
Ting, D. S. W., Carin, L., Dzau, V., and Wong, T. Y. (2020). Digital technology and COVID-19. Nat. Med. 26, 459–461. doi: 10.1038/s41591-020-0824-5
Usman, M. A., Philip, N. Y., and Politis, C. (2019). “5G enabled mobile healthcare for ambulances,” in 2019 IEEE Globecom Workshops (GC Wkshps) (Waikoloa, HI), 1–6. doi: 10.1109/GCWkshps45667.2019.9024584
Wang, C., Renzo, M. D., Stanczak, S., Wang, S., and Larsson, E. G. (2020c). Artificial intelligence enabled wireless networking for 5G and beyond: recent advances and future challenges. IEEE Wireless Commun. 27, 16–23. doi: 10.1109/MWC.001.1900292
Wang, C., Wang, Q., Ren, K., Cao, N., and Lou, W. (2012). Toward secure and dependable storage services in cloud computing. IEEE Trans. Serv. Comput. 5, 220–232. doi: 10.1109/TSC.2011.24
Wang, C. J., Ng, C. Y., and Brook, R. H. (2020a). Response to COVID-19 in Taiwan: big data analytics, new technology, and proactive testing. JAMA 323, 1341–1342. doi: 10.1001/jama.2020.3151
Wang, G., Zhang, Y., Zhao, J., Zhang, J., and Jiang, F. (2020b). Mitigate the effects of home confinement on children during the COVID-19 outbreak. Lancet 395, 945–947. doi: 10.1016/S0140-6736(20)30547-X
Wang, J., Jiang, C., Zhang, H., Ren, Y., Chen, K. C., and Hanzo, L. (2020). Thirty years of machine learning: the road to pareto-optimal wireless networks. IEEE Commun. Surv. Tutor. 22, 1472–1514. doi: 10.1109/COMST.2020.2965856
Wang, T., Wang, S., and Zhou, Z. (2019). Machine learning for 5G and beyond: from model-based to data-driven mobile wireless networks. China Commun. 16, 165–175.
Wu, J., Zhang, Y., Zukerman, M., and Yung, E. K. (2015). Energy-efficient base-stations sleep-mode techniques in green cellular networks: a survey. IEEE Commun. Surv. Tutor. 17, 803–826. doi: 10.1109/COMST.2015.2403395
Wu, S. (2019). “Key technology enablers of innovations in the AI and 5G era,” in 2019 IEEE International Electron Devices Meeting (IEDM) (San Francisco, CA), 36.3.1–36.3.4.
Xiang, Y.-T., Yang, Y., Li, W., Zhang, L., Zhang, Q., Cheung, T., et al. (2020). Timely mental health care for the 2019 novel coronavirus outbreak is urgently needed. Lancet Psychiatry 7, 228–229. doi: 10.1016/S2215-0366(20)30046-8
Xie, J., Yu, F. R., Huang, T., Xie, R., Liu, J., Wang, C., et al. (2019). A survey of machine learning techniques applied to software defined networking (SDN): research issues and challenges. IEEE Commun. Surv. Tutor. 21, 393–430. doi: 10.1109/COMST.2018.2866942
Xiong, Z., Zhang, Y., Niyato, D., Deng, R., Wang, P., and Wang, L. (2019). Deep reinforcement learning for mobile 5G and beyond: fundamentals, applications, and challenges. IEEE Vehicular Technol. Mag. 14, 44–52. doi: 10.1109/MVT.2019.2903655
Xu, H., Zhang, L., Onireti, O., Fang, Y., Buchanan, W. J., and Imran, M. (2020). “BeepTrace: blockchain-enabled privacy-preserving contact tracing for COVID-19 Pandemic and Beyond,” in IEEE Internet of Things Journal. doi: 10.1109/JIOT.2020.3025953
Xu, L. D., He, W., and Li, S. (2014). Internet of things in industries: a survey. IEEE Trans. Ind. Inform. 10, 2233–2243. doi: 10.1109/TII.2014.2300753
Yaacoub, E., and Alouini, M. (2020). A key 6G challenge and opportunity—connecting the base of the pyramid: a survey on rural connectivity. Proc. IEEE 108, 533–582. doi: 10.1109/JPROC.2020.2976703
Yaacoub, E., Husseini, M., and Ghaziri, H. (2016). “An overview of research topics and challenges for 5G massive MIMO antennas,” in 2016 IEEE Middle East Conference on Antennas and Propagation (MECAP) (Beirut), 1–4. doi: 10.1109/MECAP.2016.7790121
Yang, C., Huang, Q., Li, Z., Liu, K., and Hu, F. (2017). Big Data and cloud computing: innovation opportunities and challenges. Int. J. Digit. Earth 10, 13–53. doi: 10.1080/17538947.2016.1239771
Yang, G., Nelson, B., Murphy, R., Choset, H., Christensen, H., Collins, S., et al. (2020). Combating covid-19-the role of robotics in managing public health and infectious diseases. Sci. Robot. 5:eabb5589. doi: 10.1126/scirobotics.abb5589
Yang, G., Pang, Z., Deen, M. J., Dong, M., Zhang, Y., Lovell, N. H., et al. (2020). Homecare robotic systems for healthcare 4.0: visions and enabling technologies. IEEE J. Biomed. Health Inform. 24, 2535–2549. doi: 10.1109/JBHI.2020.2990529
Yao, M., Sohul, M., Marojevic, V., and Reed, J. H. (2019). Artificial intelligence defined 5G radio access networks. IEEE Commun. Mag. 57, 14–20. doi: 10.1109/MCOM.2019.1800629
Zeng, Y., Zhang, R., and Lim, T. J. (2016). Wireless communications with unmanned aerial vehicles: opportunities and challenges. IEEE Commun. Mag. 54, 36–42. doi: 10.1109/MCOM.2016.7470933
Zeydan, E., Bastug, E., Bennis, M., Kader, M. A., Karatepe, I. A., Er, A. S., et al. (2016). Big data caching for networking: moving from cloud to edge. IEEE Commun. Mag. 54, 36–42. doi: 10.1109/MCOM.2016.7565185
Zhang, C., Patras, P., and Haddadi, H. (2019). Deep learning in mobile and wireless networking: a survey. IEEE Commun. Surv. Tutor. 21, 2224–2287. doi: 10.1109/COMST.2019.2904897
Zhang, C., Ueng, Y., Studer, C., and Burg, A. (2020). Artificial intelligence for 5G and beyond 5G: implementations, algorithms, and optimizations. IEEE J. Emerg. Select. Top. Circuits Syst. 10, 149–163. doi: 10.1109/JETCAS.2020.3000103
Zhang, H., and Dai, L. (2019). Mobility prediction: a survey on state-of-the-art schemes and future applications. IEEE Access 7, 802–822. doi: 10.1109/ACCESS.2018.2885821
Zhang, S. (2019). An overview of network slicing for 5G. IEEE Wireless Commun. 26, 111–117. doi: 10.1109/MWC.2019.1800234
Zhang, Y., Meratnia, N., and Havinga, P. (2010). Outlier detection techniques for wireless sensor networks: a survey. IEEE Commun. Surv. Tutor. 12, 159–170. doi: 10.1109/SURV.2010.021510.00088
Keywords: COVID-19, coronavirus, pandemic, 5G networks, self-organizing networks, artificial intelligence, machine learning
Citation: Abubakar AI, Omeke KG, Ozturk M, Hussain S and Imran MA (2020) The Role of Artificial Intelligence Driven 5G Networks in COVID-19 Outbreak: Opportunities, Challenges, and Future Outlook. Front. Comms. Net. 1:575065. doi: 10.3389/frcmn.2020.575065
Received: 22 June 2020; Accepted: 12 October 2020;
Published: 11 November 2020.
Edited by:
Syed Ali Raza Zaidi, University of Leeds, United KingdomReviewed by:
Alexandros-Apostolos A. Boulogeorgos, University of Piraeus, GreeceAkram Alomainy, Queen Mary University of London, United Kingdom
Copyright © 2020 Abubakar, Omeke, Ozturk, Hussain and Imran. This is an open-access article distributed under the terms of the Creative Commons Attribution License (CC BY). The use, distribution or reproduction in other forums is permitted, provided the original author(s) and the copyright owner(s) are credited and that the original publication in this journal is cited, in accordance with accepted academic practice. No use, distribution or reproduction is permitted which does not comply with these terms.
*Correspondence: Attai Ibrahim Abubakar, a.abubakar.1@research.gla.ac.uk