- 1Autism Research Centre, Bloorview Research Institute, Holland Bloorview Kids Rehabilitation Hospital, Toronto, ON, Canada
- 2Institute of Biomedical Engineering, University of Toronto, Toronto, ON, Canada
- 3Mouse Imaging Centre, The Hospital for Sick Children, Toronto, ON, Canada
- 4Program in Neuroscience and Mental Health, Department of Medical Biophysics, The Hospital for Sick Children, University of Toronto, Toronto, ON, Canada
- 5Nuffield Department of Clinical Neurosciences, Wellcome Centre for Integrative Neuroimaging, FMRIB, University of Oxford, Oxford, United Kingdom
- 6Diagnostic Imaging, The Hospital for Sick Children, Toronto, ON, Canada
- 7Department of Medical Imaging, University of Toronto, Toronto, ON, Canada
- 8Department of Paediatrics, University of Toronto, Toronto, ON, Canada
- 9Department of Psychology, Queen's University, Kingston, ON, Canada
- 10Centre for Neuroscience Studies, Queen's University, Kingston, ON, Canada
- 11Department of Psychiatry, Queen's University, Kingston, ON, Canada
- 12Department of Psychiatry, University of Toronto, Toronto, ON, Canada
- 13Department of Psychiatry, The Hospital for Sick Children, Toronto, ON, Canada
- 14Department of Psychiatry and Behavioural Neurosciences, McMaster University, Hamilton, ON, Canada
- 15Department of Psychiatry, Western University, London, ON, Canada
Introduction: Attention-deficit/hyperactivity disorder (ADHD) and autism are multi-faceted neurodevelopmental conditions with limited biological markers. The clinical diagnoses of autism and ADHD are based on behavioural assessments and may not predict long-term outcomes or response to interventions and supports. To address this gap, data-driven methods can be used to discover groups of individuals with shared biological patterns.
Methods: In this study, we investigated measures derived from cortical/subcortical volume, surface area, cortical thickness, and structural covariance investigated of 565 participants with diagnoses of autism [n = 262, median(IQR) age = 12.2(5.9), 22% female], and ADHD [n = 171, median(IQR) age = 11.1(4.0), 21% female] as well neurotypical children [n = 132, median(IQR) age = 12.1(6.7), 43% female]. We integrated cortical thickness, surface area, and cortical/subcortical volume, with a measure of single-participant structural covariance using a graph neural network approach.
Results: Our findings suggest two large clusters, which differed in measures of adaptive functioning (χ2 = 7.8, P = 0.004), inattention (χ2 = 11.169, P < 0.001), hyperactivity (χ2 = 18.44, P < 0.001), IQ (χ2 = 9.24, P = 0.002), age (χ2 = 70.87, P < 0.001), and sex (χ2 = 105.6, P < 0.001).
Discussion: These clusters did not align with existing diagnostic labels, suggesting that brain structure is more likely to be associated with differences in adaptive functioning, IQ, and ADHD features.
Introduction
Autism spectrum disorder (hereafter autism) and attention-deficit/hyperactivity disorder (ADHD) are multifaceted neurodevelopmental conditions (1). Autism is characterized by differences in social communication and the presence of intense interests and repetitive behaviors (prevalence 1%–2%) (1); ADHD is defined by inattention and/or hyperactivity and impulsiveness (2) (prevalence 7%) (3). Currently, the diagnoses of autism and ADHD are based on observed and reported behavioral assessments (4), and no biological markers exist to inform the assignment of diagnostic labels. Despite this, the discrete labels of autism and ADHD are commonly used to inform service provision. However, these labels may not always capture the needs of neurodivergent populations (5). Within autism and ADHD, there is large variability in aetiology, neurobiology, phenotypic presentation, and profiles of strengths and disability (6, 7). At the same time, significant overlap exists between the two conditions. For example, the prevalence of co-occurring ADHD in autism is estimated to be 28% [95% CI: (25–32)] (8), while 21% [95% CI: (18–24)] of children with ADHD are reported to meet clinical thresholds for autism (9). Autism and ADHD are also reported to share genetic underpinnings (10) and neurobiological features including similarities in brain structure (11–13), function (14, 15), and connectivity (16, 17). Shared phenotypic and neurocognitive features also exist between autism and ADHD. These include differences in social communication (18), sensory processing (19), face processing (20), response inhibition and sustained attention (21). In this context, several studies have found that the labels of autism and ADHD may not be associated with unique and distinct biological and behavioural profiles (1, 12). The misalignment between these discrete labels and underlying neurobiology significantly challenges the development and provision of personalized supports that can appropriately fit the breadth of needs and strengths of neurodivergent individuals.
To address this gap, an emerging body of literature has focused on characterizing the heterogeneity in neurobiology and/or phenotypic presentation within and across neurodevelopmental conditions including autism and ADHD (10, 11–13). This approach shifts away from discrete diagnosis categories to a data-driven approach that can more closely capture profiles aligned with real-world outcomes (24). An example of this approach is the Research Domain Criteria (RDoc) framework (25). Data-driven approaches, and specifically clustering, have also received attention as they do not require any a priori assumptions of group labels; instead of examining differences between pre-defined diagnostic groupings, data-driven approaches look to the data to describe variability and discover homogeneous groupings that can map onto profiles of needs and strengths. The common finding across these studies is the lack of alignment between existing diagnostic labels and neurobiology, although the discovered subgroups vary significantly across different studies depending on the sample characteristics and data modality used [see review by Astle, Holmes, Kievit, and Cathercole (5)].
In terms of studies focused on neurobiology, measures of brain structure (12, 22), function (26), and connectivity (27) have been most commonly employed. The present work focuses on brain structure in particular. Brain structure is suggested to have utility as an intermediate phenotype that links multiple genetic variants (12, 23, 28), and gray matter volume differences have been documented in ASD and ADHD (29–32) compared to neurotypical populations. A key limitation of these studies, however, is that regional measures of brain structure are used in isolation and the relation between brain regions is often ignored in the analyses. This is a critical shortcoming as autism and ADHD are associated with pervasive differences across brain networks (33, 34). To address this limitation, the present study extends the previous literature by integrating regional associations in the form of structural covariance (35) into the clustering. Structural covariance quantifies population-level correlation among measures of gray matter morphology (36). Structural covariance networks are highly heritable, demonstrate alterations in samples of children with ASD (37) and ADHD (38, 39), have associations with cognitive ability (40), and replicate patterns of interregional functional and structural connectivity and maturational coupling in autism (41). We hypothesize that (a) data-driven clusters derived from sMRI data will not align with traditional diagnostic labels, demonstrated by low values of normalized mutual information, adjusted rand score, homogeneity, and completeness, (b) the derived clusters will transcend diagnostic boundaries and contain participants from different diagnosis groups, (c) there will be widespread cluster differences in brain structure across cortical and subcortical regions, and (d) the data-driven clusters will be associated with phenotypic differences across levels of cognition, behaviour, and function.
Materials and methods
Participants
The study used data from the Province of Ontario Neurodevelopmental Network (POND; export date: August 7, 2021). The dataset included participants with primary diagnoses of autism (n = 262) or ADHD (n = 171), as well as those who were neurotypical (TD) (n = 132) between the ages of 5–23 years (mean age: 12.2 ± 3.78; 413 males, 152 females). All participants and their parents were able to complete the testing protocols in English and had no contraindications to magnetic resonance imaging (MRI). Participants in the clinical groups met the DSM criteria for their respective diagnosis and diagnoses were supported by gold-standard assessments [autism: Autism Diagnostic Observation Schedule–2 (ADOS) (42) and Autism Diagnostic Interview–Revised (ADI-R) (20); ADHD: Parent Interview for Child Symptoms (PICS) (43)]. Participants in the TD group did not have a neurodevelopmental, psychiatric and/or neurological diagnosis or any first-degree relatives with a neurodevelopmental condition, and were born after 35 weeks gestation. Informed consent was provided by participants (when they had the capacity to consent) or their guardians, and assent was obtained from all participants as per institutional ethics board guidelines. Institutional research ethics boards approvals were received for the study.
Behavioral measures
Participants were characterized using phenotypic measures quantifying core and co-occurring conditions. These included the Social Communication Questionnaire (SCQ; lifetime) (44) for autism-like traits (total score) and the Strengths and Weaknesses of ADHD-symptoms and Normal Behavior (SWAN) (45) for ADHD-like features (inattentive and hyperactivity subscales). Adaptive functioning was quantified using the Adaptive Behavior Assessment System-II (ABAS-II) (46) for adaptive function (general ability composite). Co-occurring emotional and behavioural symptoms were quantified using the Child Behavior Checklist (CBCL) (47). Neurophysiological assessments of participants included the Developmental Neuropsychological Assessment (NEPSY; affect recognition and memory for faces) (48) as well as the stop-signal task (response inhibition, sustained attention, and reaction time) (21). Full-scale IQ was measured using the Wechsler Abbreviated Scale of Intelligence (WASI) (49), the Wechsler Intelligence Scale for Children (WISC) (50), or the Stanford-Binet Test (51) as appropriate for age and ability level.
Imaging data
We used structural MRI (sMRI) to extract measures of surface area, cortical thickness, and cortical/subcortical regions volume. A portion of images were collected on a 3-Tesla Siemens Trio TIM at the Hospital for Sick Children (n = 280), which was upgraded to a Siemens PrismaFIT scanner (n = 395). The rest of the data were collected using 3-Tesla Siemens Trio TIM at Queen's University (n = 113) which was later updated to Siemens PrismaFit scanner (n = 5). The parameters for Imaging acquisition are provided in Supplementary Table S1. The CIVET pipeline (version 2.1.0) (52) was used to extract area, cortical thickness, and cortical volume for 76 regions of interest (ROI) according to automated anatomical labeling atlas (AAL) (53, 54) using the T1-weighted images. The pipeline includes non-uniformity image correction followed by stereotaxic registration to the Montreal Neurologic Institute (MNI ICBM) (55) template (non-linear 6th generation target) (56). Furthermore, gray matter, white matter, and cerebrospinal fluid were obtained by the process of masking, extraction, and classification. Gray matter and white matter surfaces were then created using tissue classification images (57–60). A surface diffusion kernel (44), followed by registration of regions to the AAL (53, 54) was applied. To calculate cortical thickness, the distance between two smoothed surfaces (61) was used. Surface area was generated by tissue classification images (41). Subcortical structure volumes (95 regions) were calculated based on segmentation using multiple automatically generated templates (MAGeT) (62). A list of regions included in the analysis is provided in Supplementary Table S2. The CIVET and MAGeT quality control (QC) pipelines were used. The CIVET QC pipeline excludes scans with artifacts, inaccurate segmentations, and registration errors (52). Motion artifacts are detected based on the number of surface to surface intersections per hemisphere (limit of 150 touch points per surface pair per side). MAGeT's QC pipeline removes data with missing values (63). The data were corrected for scanner effects using the ComBat Harmonization model (53), for sex (64) using linear regression, and for age using the best fitting linear, quadratic, or cubic polynomial models determined through cross validation.
Analysis
Pipeline
Structural MRI data were processed using an analytical pipeline to discover clusters based on regional volume, cortical thickness, surface area, as well as associations between pairs of regions. To this end, we first computed statistical associations among regions for each participant (“participant-level association graph”). Next, the participant association graphs were used to compute similarity among pairs of participants resulting in a participant similarity matrix. This similarity matrix was then integrated with regional structural features to generate a final similarity matrix which was used for clustering. Preprocessing and clustering were carried out in Python 3.8.0. An overview of the pipeline is described below and summarized in Figure 1. A detailed description of the pipeline is provided in the Supplementary Materials.
Compute single participant structural association graphs (participant association graphs)
Structural covariance for a pair of regions is typically computed using the entire participant pool. We extended this concept, to compute a measure of statistical association between two regions for a single participant. To this end, we computed the linear regression line for a pair of regions using the entire participant pool (as done in structural covariance computation). For each participant, we then computed a measure of deviation from this line using the Cook's distance (65). The distance was transformed into a similarity value using a Guassian kernel. This process was repeated for all region pairs, resulting in a single graph for each participant with regions as nodes and the computed similarity as edge weights. Each brain measure was treated independently, leading to four association graphs per participant (surface area, cortical thickness, cortical volume, and subcortical volume).
Compute a participant similarity graph
Participant association graphs from step 1 were used to compute the similarity between pairs of participants. This was done using the Gaussian-transformed Lambda distance (66, 67). The participant similarities were represented as a graph with participants as nodes and similarities as edge weights.
Merge similarity matrices
Step 2 generated four participant similarity matrices, one for each brain measure (cortical thickness, surface area, cortical and subcortical volume). These were merged using Similarity Network Fusion (SNF) (68), resulting in a single integrated participant similarity graph.
Integrate structural association similarity with regional brain measures
The similarity graph in step 2 was built using statistical associations between regions. Next, we integrated this matrix with information about regional measures of cortical thickness, surface area, and cortical and subcortical volume. This was accomplished using a Graph Neural Network (GNN) as described in the Supplementary Materials. K-means clustering (69) was used to cluster the compact representation of each participant derived in step 4. To reduce sensitivity of the clustering solution to choice of parameters, this step was repeated with varying the number of clusters and SNF parameters (scaling factor µ, number of neighbours K), and consensus between resultant clusters across 15,000 iterations was used to derive final clusters (27) (detailed description of methods in Supplementary Methods). The Davies Bouldin score (70), Silhouette Coefficient (71), and Calinski-Harabasz score (72) were used to find the optimal number of clusters.
Post-hoc statistical analyses
We compared the result of our clustering with the clinical diagnostic labels using normalized mutual information (73), adjusted rand score (74), homogeneity, and completeness (75). All measures provide a score between zero (low) and one (high). Phenotypic and brain characteristics were compared across clusters using the t-test or Kruskal–Wallis test for normally and non-normally distributed continuous data, respectively. For categorical data, the chi-squared test was used. Statistical analyses were performed in R 3.3.3.
Results
Participants
From a total of 661 participants, 79 failed quality control (QC) for CIVET and of the remaining participants 17 participants failed QC for MAGeT (breakdown by diagnosis and age group is provided in Supplementary Tables S3, S4). The demographic characteristics for the remaining 565 participants are shown in Table 1. As seen, the autism and ADHD groups had a significantly higher proportion of males than females (χ2 = 25.40, P < 0.0001), and a significant age difference between ADHD, autism, and TD groups was observed χ2 = 7.28, P = 0.026). Table 1 also provides the clinical characterization of the participants. A subset of the participants also had neurocognitive assessments (NEPSY and stop-signal task) available. This information is reported in Supplementary Table S5. Medication usage in the sample is reported in Supplementary Table S6.
Clusters
The Davies Bouldin score (70), Silhouette Coefficient (71), and Calinski-Harabasz score (72) all identified the 2-cluster solution as optimal; However, to enhance the generalizability of the results, we present the clustering solution for 2 to 6 clusters (Figure 2). As seen, participants were grouped into two clusters (cluster 1: n = 252, cluster 2: n = 313), although both groups had mixed representation from the diagnostic groups (TD: autism: ADHD; cluster 1: 57%, 47%, 32%; cluster 2: 43%, 53%, 68%), cluster 1 contained the majority of the TD participants (57%) whereas cluster 2 contained the majority of participants with a diagnosis of ADHD (68%). We, therefore will refer to these clusters as TD- and ADHD-enriched clusters, respectively. As we increased the number of clusters, cluster structure remained stable with the tails of the two clusters separating into new clusters (Figure 2). To examine the alignment between diagnostic labels and our cluster assignments (Supplementary Figure S1), we computed the normalized mutual information and adjusted rand scores. The scores were less than 0.02 and 0.01 consistently as the number of clusters varied from 2 to 6, indicating low agreement between the clusters and existing diagnostic labels. Furthermore, the homogeneity and completeness scores were both less than 0.02, demonstrating that obtained clusters did not represent a single diagnostic group regardless of the number of clusters used.
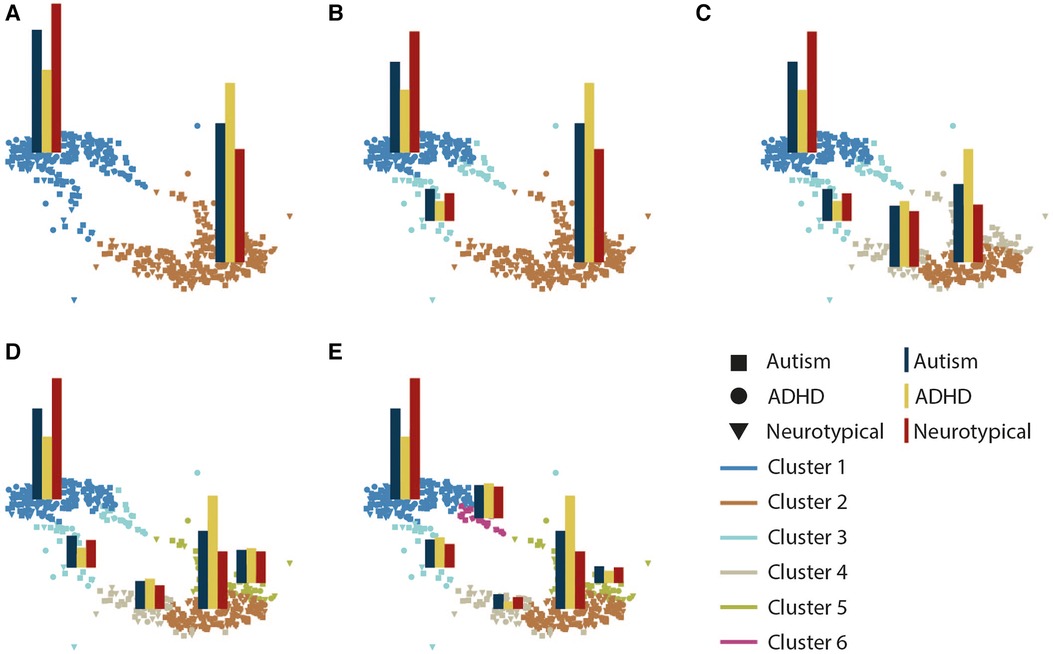
Figure 2. Graph representation of clusters as the number of clusters increases from 2 (A) to 6 (E). Each subplot illustrates a graph representation with participants as nodes. Edges are not displayed to enhance readability. Node colors distinct clusters, and superimposed bar plots show the distribution of diagnostic groups within each cluster (autism, ADHD, TD).
Brain correlates of clusters
The two clusters were compared on measures of cortical and subcortical volume, cortical thickness, and surface area. Cohen's effect size is shown for regions where significant differences were found after correction for multiple comparisons in Figure 3. As seen, the largest effect sizes were found in cortical volume (TD-enriched > ADHD-enriched) across the cortex. Volumes of subcortical regions were not significantly different between the two clusters, except in the cerebellar vermis III and VIIB (ADHD-enriched > TD-enriched).
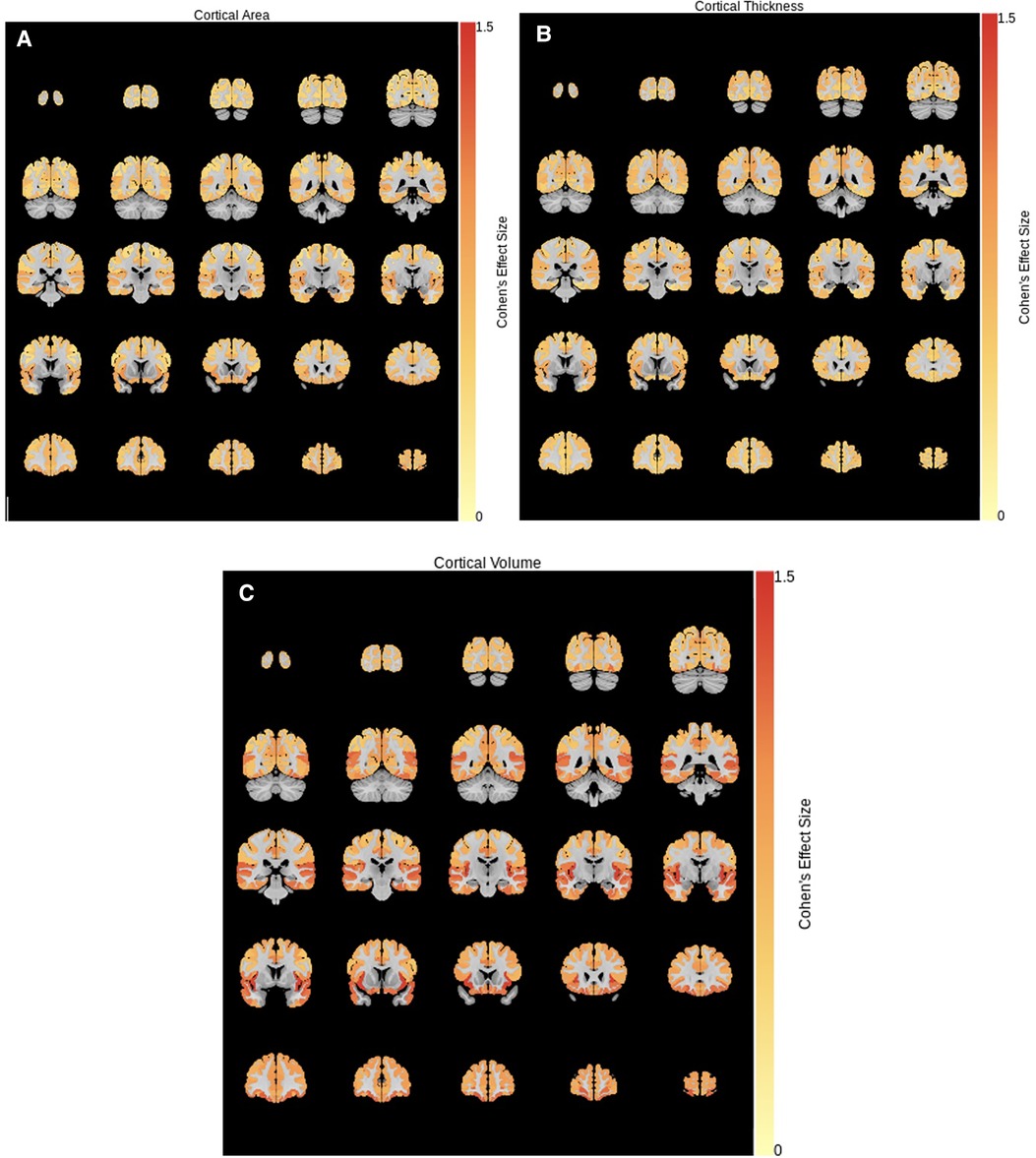
Figure 3. Cohen's effect size for cluster differences (TD-enriched—ADHD-enriched) in (A) surface area, (B) cortical thickness, and (C) cortical volume.
To further characterize cluster differences, we compared the two clusters in structural association edge weights using Kruskal–Wallis tests. After FDR correction for multiple comparisons, edge weight values were significantly different between the two clusters for cortical volume, cortical thickness, and subcortical volume for several regions, but not for surface area (Figure 4). For cortical volume, negative differences (TD-enriched < ADHD-enriched) were observed for several regions pairs, with the left middle temporal gyrus featuring most prominently (associations with the the right median cingulate and paracingulate gyri, left angular gyrus, and left inferior occipital gyrus, left insula). Association between the right cingulate gyri and various regions were also found to be significant (right median cingulate and right olfactory cortex, right insula, left gyrus rectus; right anterior cingulate and right middle temporal gyrus). Negative differences (TD-enriched < ADHD-enriched) were also found in subcortical regions, including in the associations between the left nucleus accumbens/ventral striatum and right cerebellum VI, and the right postcommissural caudate and vermal IV, suggesting closer alignment of the ADHD-enriched cluster with the sample trend.
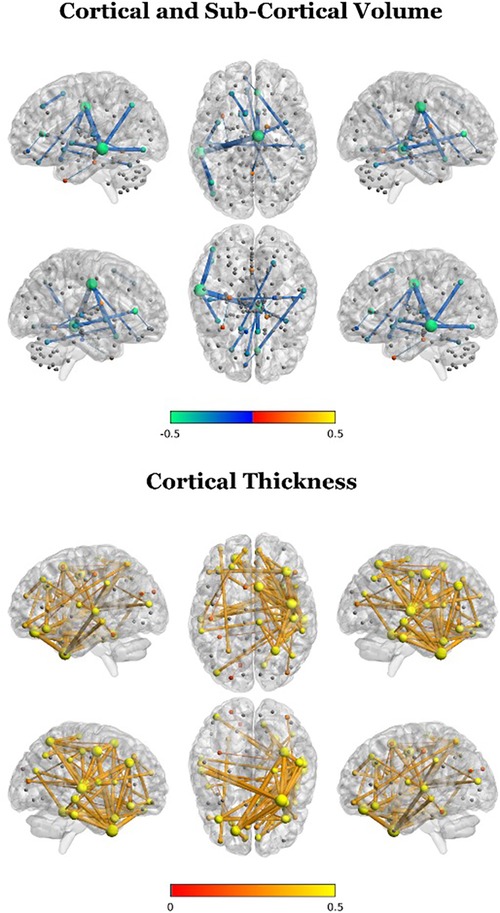
Figure 4. Cluster differences in edge weights for participant brain networks (2 clusters; TD-enriched—ADHD-enriched). A positive difference in this plot indicates that the statistical association between two given regions were more closely aligned with the overall sample structural covariance for the TD-enriched cluster compared to the ADHD-enriched cluster.
For cortical thickness, widespread positive differences (TD-enriched > ADHD-enriched) were found, mainly in the right hemisphere. Most prominently, these included associations between the right fusiform gyrus and the right temporal gyri and the left superior frontal gyrus as well as associations among frontal and temporal gyri.
Demographic/phenotypic characterization of clusters
The demographic and phenotypic differences between the two clusters are shown in Figure 5. Compared to the TD-enriched cluster, the ADHD-enriched cluster had significantly higher proportion of ADHD participants (ADHD-enriched: 53.9% ADHD, TD-enriched: 46.1% ADHD; χ2 = 16.08, P < 0.0001) and lower proportion of TD (ADHD-enriched: 15.8% ADHD, TD-enriched: 84.2% ADHD; χ2 = 9.77, P = 0.0017). The participants in the ADHD-enriched cluster were significantly younger than those in the TD-enriched group (median difference = 3.3; χ2 = 70.84, P < 0.0001). As expected, this cluster also contained a significantly higher proportion of males (ADHD-enriched: 68.5%, TD-enriched: 31.5%; χ2 = 105.60, P < 0.0001) and had significantly higher median scores on ADHD-like features [SWAN inattention: ADHD-enriched: 4.0(6.00), TD-enriched: 3.0(6.00); χ2 = 11.20, P < 0.0001; SWAN hyperactivity -ADHD-enriched: 2.0(6.00), TD-enriched: 1.0(4.00); χ2 = 18.40, P < 0.0001], lower median scores on adaptive functioning [ABAS-General Ability Composite: ADHD-enriched: 75.0(26.00), TD-enriched: 82.0(36.00), χ2 = 7.80, P = 0.0052], lower inhibitory control [Stop Task stop reaction time: ADHD-enriched: 244.8(141.82), TD-enriched:292.17(130.31), χ2 = 7.80, P = 0.0009], and lower IQ [ADHD-enriched: 100.0(22.00), TD-enriched: 106.0(21.00), χ2 = 14.06, P = 0.0024]. NEPSY memory for faces and affect recognition, SCQ, CBCL internationalizing, and CBCL externalizing scores were not significantly different between clusters.
To gain further insight into the misalignment between diagnoses and our clusters, we investigated differences among participants who had the same diagnostic label (autism, ADHD, and TD) but fell into different clusters (Figure 6). Compared to those in the ADHD-enriched cluster, TD participants in the TD-enriched cluster were significantly older [ADHD-enriched: 14.1(8.63), TD-enriched: 10.4(4.34), χ2 = 15.75, P < 0.0001], had significantly lower SCQ scores [ADHD-enriched: 2.5(3.00), TD-enriched: 1.0(2.00); χ2 = 6.91, P = 0.0086], and higher ABAS scores [ADHD-enriched: 99.0(19.00), TD-enriched: 109.0(16.50); χ2 = 15.05, P = 0.0001]. Participants with ADHD in the ADHD-enriched cluster were also younger than those in the TD-enriched cluster [ADHD-enriched: 10.4(3.59), TD-enriched: 12.2(4.98); χ2 = 7.69, P = 0.006], and had lower IQ scores [ADHD-enriched: 99.0(19.00), Mixed: 106.0(20.25); χ2 = 7.61, P = 0.006]. Autistic participants in ADHD-enriched cluster were also significantly younger [ADHD-enriched: 10.7(5.07), TD-enriched: 14.6(5.19), χ2 = 45.46, P < 0.0001], and had a significantly higher SWAN-hyperactivity score [ADHD-enriched: 4.0(5.50), TD-enriched: 2.0(5.00); χ2 = 11.03, P = 0.0009].
Discussion
Using a data-driven and diagnosis-agnostic approach, this paper characterized the variability in brain structure across neurodiverse children and youth. We introduced a novel analytics pipeline that integrated interregional couplings in addition to other measures of brain structure into the clustering algorithm. This is particularly critical as autism and ADHD are associated with differences in brain networks, and not specific regional lesions (13, 76–79).
Consistent with the previous literature (11, 13) examining measures of brain function and structure, our results revealed a mismatch between biological homogeneity and the labels of autism, ADHD, and typical development; instead, we found clusters that contained participants from different diagnostic groups. This finding lends further support to the idea that these discrete categories do not represent neurobiologically homogeneous groups. This motivates the need for an approach that characterizes neurodevelopmental diversity in a way that can identify dimensions of real-world needs (24).
Our results suggest the presence of two clusters which were neurobiologically different in brain structure. The first cluster was characterized by larger cortical volumes across the brain. This cluster was enriched for TD participants and, on average, was associated with decreased autism and ADHD traits, older age, and increased adaptive functioning and IQ. The second cluster was enriched for participants with a diagnosis of ADHD and associated with increased inattention and hyperactivity, and lower IQ and adaptive functioning. These results suggest that our clustering results are driven by features across multiple domains of cognition, behaviour, and function. In particular, our ADHD-enriched cluster was associated with decreased response inhibition [i.e., longer stop signal reaction time (SSRT)], increased ADHD symptoms (inattention and hyperactivity), and decreased adaptive functioning. Decreased response inhibition is a well-established neurocognitive feature of ADHD (80), but the findings in autism have been mixed (81). There is emerging evidence to suggest that response inhibition differences in autism may be explained by co-occurring ADHD symptoms (21), a finding that is consistent with our results. The clusters were not different in autism-like features at the cognitive (NEPSY affect recognition, memory for faces) or behavioural (SCQ) levels, suggesting specificity of the clustering to ADHD-like traits. These findings should be interpreted with caution, however, given that SSRT decreases with age and the fact that our ADHD-enriched cluster had lower average age (21). This is also supported by our finding of significant differences between participants who shared the same diagnosis label, but were assigned to different clusters. In particular, participants from the TD group who were assigned to the ADHD-cluster had higher scores on autism and inattention measures and lower scores for adaptive functioning, compared to those in the TD-enriched cluster; Participants with ADHD and ASD in the ADHD-enriched clusters had significantly higher IQ and hyperactivity, respectively, compared those in the TD-enriched cluster. These results further highlight the importance of a dimensional approach to characterization of neurodevelopmental conditions.
Our analytical pipeline considered measures of morphology for brain regions in isolation, as done in previous literature, but also integrated information about statistical associations among pairs of regions. This was done by using participant-level measures of deviance from regional correlations. This approach was motivated by the wide-spread consensus that autism and ADHD are associated with pervasive differences across brain networks (33, 34) instead of differences in isolated regions of the brain. Our results showed significant differences between the data-driven clusters in these association measures in regions that have been previously associated with neurodevelopmental conditions including the fronto-temporal and cingulate regions (82). For cortical volume, our pattern of findings was consistent with one of the few previous studies examining the structural covariance of gray matter volume in ADHD (83). Increased structural associations were observed in the ADHD- compared to TD-enriched group in regions including the middle temporal and cingulate gyri, regions that comprise the default mode network (84) which is frequently implicated in ADHD (85). Differences in connections involving subcortical regions were also reported between the ADHD- and TD-enriched groups; structural alterations in subcortical regions are frequently reported in the ADHD literature, potentially underlying problems in domains such as emotional regulation (86). For cortical thickness, increases in structural connectivity in the TD- compared to ADHD-enriched group were observed in fronto-temporal connections, which has been shown to be related to inhibitory control in individuals with ADHD (87).
Our results should be interpreted in the context of significant cluster differences in age and sex ratios. In particular, the TD-enriched cluster was significantly older than those in the ADHD-enriched cluster, and contained fewer males. Given that the data were corrected for age prior to analyses, the age differences between clusters may be related to differences in neurodevelopmental trajectories [e.g., differences may decrease with age (2, 32, 37)], and specifically age-related differences in presentation of ADHD-like symptoms. Longitudinal studies are needed to future investigate this issue. Sex differences in clusters are not surprising as these have been previously reported in the prevalence and expression of ASD and ADHD (88–93). These differences may also be related to increased representation of male participants in the autism and ADHD groups.
Overall, our results contribute to the emerging body of literature motivating a shift away from broad diagnostic labels for autism and ADHD towards increased precision at phenotypic, cognitive, and biological levels. Data-driven approaches, when appropriately validated and replicated, can contribute to this precision by identifying trans-diagnostic biological and cognitive features that are targets for intervention and/or markers of treatment response. Our findings, for example, when replicated, can potentially identify children who, regardless of diagnosis, may benefit from interventions focused on ADHD symptoms such as difficulties with response inhibition.
Limitations
The results of our study must be interpreted in the context of three limitations. First, our analyses focused on structural MRI data; future multi-modal analysis (e.g., including fMRI) may provide additional insights into the variability across neurodevelopmental conditions. Second, replication of the procedure on larger independent samples is also needed. Third, although the complexity of our analytic pipeline allowed for the integration of regional associations, future studies are needed to further clarify the interpretation of the findings in terms of specific neurobiological features involved.
Data availability statement
The data analyzed in this study is subject to the following licenses/restrictions: The data that support the findings of this study are available as per Ontario Brain Institute data access policy. Requests to access these datasets should be directed to https://pond-network.ca.
Ethics statement
The studies involving humans were approved by Holland Bloorview Research Ethics Board. The studies were conducted in accordance with the local legislation and institutional requirements. Written informed consent for participation in this study was provided by the participants or their legal guardians as appropriate.
Author contributions
YS: conceptualized and designed the study, analysed study data, drafted the original manuscript, interpreted the results, and revised the manuscript. RC and MM: generated manuscript figures, and critically reviewed and revised the manuscript. EA: contributed to the concept and design of the study, acquisition of study data, interpretation of results, and the critical review and revision of the manuscript. JL, MT, AI, JB, EK, MA, JC, RS, SG, and RN: contributed to the acquisition of data and the critical review and revision of the manuscript. AK: conceptualized and designed the study and analytic plan, interpreted the results, and drafted the manuscript. BS and CH: contributed to processing of the neuroimaging data and generation of figures. All authors approved the final manuscript as submitted and agree to be accountable for all aspects of the work. All authors contributed to the article and approved the submitted version.
Funding
Funding was provided by the Ontario Brain Institute (PIs: EA and JL), the New Frontiers in Research Fund (AK), the Natural Sciences and Engineering Research Council of Canada (NSERC).
Acknowledgments
We would like to thank all participants and their families for their involvement in this study. We would also like to thank POND staff at all sites for their contribution to data collection; these individuals were compensated for their time.
Conflict of interest
AK is the inventor of a software called the "Anxiety Meter". She is involved in commercialising the Anxiety Meter [patents US 9,844,332 B2 and US 16/276,208 (pending)] and will financially benefit from its sales. AK served on the board of advisors for Shaftesbury, a media company developing virtual reality products for autistic children, from February 2020—February 2021, and was compensated financially for this role. AK has received donations of hardware for her research program from Samsung Canada. AK has received personal fees from DNAstack. EA reports grants from Roche, personal fees from Roche, personal fees from Quadrant, personal fees from Wiley, book royalties from Springer, book royalties from APPI, and non-financial support from AMO Pharma outside the submitted work. In addition, EA holds a patent on the software called the “Anxiety Meter”; the Anxiety Meter is being commercialised and she will financially benefit from its sales.
The remaining authors declare that the research was conducted in the absence of any commercial or financial relationships that could be construed as a potential conflict of interest.
The author MJT declared that they were an editorial board member of Frontiers at the time of submission. This had no impact on the peer review process and the final decision.
Publisher's note
All claims expressed in this article are solely those of the authors and do not necessarily represent those of their affiliated organizations, or those of the publisher, the editors and the reviewers. Any product that may be evaluated in this article, or claim that may be made by its manufacturer, is not guaranteed or endorsed by the publisher.
Supplementary material
The Supplementary Material for this article can be found online at: https://www.frontiersin.org/articles/10.3389/frcha.2023.1171337/full#supplementary-material
References
1. Christensen DL, Bilder DA, Zahorodny W, Pettygrove S, Durkin MS, Fitzgerald RT, et al. Prevalence and characteristics of autism spectrum disorder among 4-year-old children in the autism and developmental disabilities monitoring network. J Dev Behav Pediatr. (2016) 37:1–8. doi: 10.1097/DBP.0000000000000235
2. Bálint S, Czobor P, Komlósi S, Mészáros A, Simon V, Bitter I. Attention deficit hyperactivity disorder (ADHD): gender- and age-related differences in neurocognition. Psychol Med. (2009) 39:1337–45. doi: 10.1017/S0033291708004236
3. Thomas R, Sanders S, Doust J, Beller E, Glasziou P. Prevalence of attention-deficit/hyperactivity disorder: a systematic review and meta-analysis. Pediatrics. (2015) 135:e994–1001. doi: 10.1542/peds.2014-3482
4. American Psychiatric Association. Diagnostic and statistical manual of mental disorders. Virginia, USA: American Psychiatric Association (2013). doi: 10.1176/appi.books.9780890425596
5. Astle DE, Holmes J, Kievit R, Gathercole SE. Annual research review: the transdiagnostic revolution in neurodevelopmental disorders. J Child Psychol Psychiatry. (2021) 63(4):397–417. doi: 10.1111/jcpp.13481
6. Eaves LC, Ho HH, Eaves DM. Subtypes of autism by cluster analysis. J Autism Dev Disord. (1994) 24, 3–22. doi: 10.1007/BF02172209
7. Zheng S, Hume KA, Able H, Bishop SL, Boyd BA. Exploring developmental and behavioral heterogeneity among preschoolers with ASD: a cluster analysis on principal components. Autism Res. (2020) 13:796–809. doi: 10.1002/aur.2263
8. Lai MC, Kassee C, Besney R, Bonato S, Hull L, Mandy W, et al. Prevalence of co-occurring mental health diagnoses in the autism population: a systematic review and meta-analysis. Lancet Psychiatry. (2019) 6:819–29. doi: 10.1016/S2215-0366(19)30289-5
9. Hollingdale J, Woodhouse E, Young S, Fridman A, Mandy W. Autistic spectrum disorder symptoms in children and adolescents with attention-deficit/hyperactivity disorder: a meta-analytical review. Psychol Med. (2020) 50:2240–53. doi: 10.1017/S0033291719002368
10. Andersson A, Tuvblad C, Chen Q, Du Rietz E, Cortese S, Kuja-Halkola R, et al. Research review: the strength of the genetic overlap between ADHD and other psychiatric symptoms—a systematic review and meta-analysis. J Child Psychol Psychiatry. (2020) 61:1173–83. doi: 10.1111/jcpp.13233
11. Baribeau DA, Dupuis A, Paton TA, Hammill C, Scherer SW, Schachar RJ, et al. Structural neuroimaging correlates of social deficits are similar in autism spectrum disorder and attention-deficit/hyperactivity disorder: analysis from the POND network. Transl Psychiatry. (2019) 9:72. doi: 10.1038/s41398-019-0382-0
12. Kushki A, Anagnostou E, Hammill C, Duez P, Brian J, Iaboni A, et al. Examining overlap and homogeneity in ASD, ADHD, and OCD: a data-driven, diagnosis-agnostic approach. Transl Psychiatry. (2019) 9:318. doi: 10.1038/s41398-019-0631-2
13. Kushki A, Cardy RE, Panahandeh S, Malihi M, Hammill C, Brian J, et al. Cross-diagnosis structural correlates of autistic-like social communication differences. Cereb Cortex. (2021) 31:5067–76. doi: 10.1093/cercor/bhab142
14. Choi EJ, Vandewouw MM, Taylor MJ, Arnold PD, Brian J, Crosbie J, et al. Beyond diagnosis: cross-diagnostic features in canonical resting-state networks in children with neurodevelopmental disorders. NeuroImage Clin. (2020) 28:102476. doi: 10.1016/j.nicl.2020.102476
15. Vandewouw MM, Brian J, Crosbie J, Schachar RJ, Iaboni A, Georgiades S, et al. Identifying replicable subgroups in neurodevelopmental conditions using resting-state functional magnetic resonance imaging data. JAMA Netw Open. (2023) 6:e232066. doi: 10.1001/jamanetworkopen.2023.2066
16. Ameis SH, Lerch JP, Taylor MJ, Lee W, Viviano JD, Pipitone J, et al. A diffusion tensor imaging study in children with ADHD, autism spectrum disorder, OCD, and matched controls: distinct and non-distinct white matter disruption and dimensional brain-behavior relationships. Am J Psychiatry. (2016) 173:1213–22. doi: 10.1176/appi.ajp.2016.15111435
17. Zhang K, Fu Z, Lai Q, Zhao Y, Liu J, Cao Q. The shared white matter developmental trajectory anomalies of attention-deficit/hyperactivity disorder and autism spectrum disorders: a meta-analysis of diffusion tensor imaging studies. Prog Neuropsychopharmacol Biol Psychiatry. (2023) 124:110731. doi: 10.1016/j.pnpbp.2023.110731
18. Baribeau DA, Doyle-Thomas KA, Dupuis A, Iaboni A, Crosbie J, McGinn H, et al. Examining and comparing social perception abilities across childhood-onset neurodevelopmental disorders. J Am Acad Child Adolesc Psychiatry. (2015) 54:479–86.e1. doi: 10.1016/j.jaac.2015.03.016
19. Scheerer NE, Pourtousi A, Yang C, Ding Z, Stojanoski B, Anagnostou E, et al. Transdiagnostic patterns of sensory processing in autism and ADHD. J Autism Dev Disord. (2022) 17:12–21. doi: 10.1007/s10803-022-05798-3
20. Vandewouw MM, Choi E, Hammill C, Arnold P, Schachar R, Lerch JP, et al. Emotional face processing across neurodevelopmental disorders: a dynamic faces study in children with autism spectrum disorder, attention deficit hyperactivity disorder and obsessive-compulsive disorder. Transl Psychiatry. (2020) 10:1–12. doi: 10.1038/s41398-020-01063-2
21. Schachar RJ, Dupuis A, Arnold PD, Anagnostou E, Kelley E, Georgiades S, et al. Autism spectrum disorder and attention-deficit/hyperactivity disorder: shared or unique neurocognitive profiles? Res Child Adolesc Psychopathol. (2023) 51:17–31. doi: 10.1007/s10802-022-00958-6
22. Aoki Y, Yoncheva YN, Chen B, Nath T, Sharp D, Lazar M, et al. Association of white matter structure with autism spectrum disorder and attention-deficit/hyperactivity disorder. JAMA Psychiatry. (2017) 74:1120. doi: 10.1001/jamapsychiatry.2017.2573
23. Ellegood J, Anagnostou E, Babineau BA, Crawley JN, Lin L, Genestine M, et al. Clustering autism: using neuroanatomical differences in 26 mouse models to gain insight into the heterogeneity. Mol Psychiatry. (2015) 20:118–25. doi: 10.1038/mp.2014.98
24. Fletcher-Watson S. Transdiagnostic research and the neurodiversity paradigm: commentary on the transdiagnostic revolution in neurodevelopmental disorders by Astle et al. J Child Psychol Psychiatry. (2022) 63:418–20. doi: 10.1111/jcpp.13589
25. Cuthbert BN. The RDoC framework: facilitating transition from ICD/DSM to dimensional approaches that integrate neuroscience and psychopathology. World Psychiatry. (2014) 13:28–35. doi: 10.1002/wps.20087
26. Cao M, Shu N, Cao Q, Wang Y, He Y. Imaging functional and structural brain connectomics in attention-deficit/hyperactivity disorder. Mol Neurobiol. (2014) 50:1111–23. doi: 10.1007/s12035-014-8685-x
27. Sidlauskaite J, Sonuga-Barke E, Roeyers H, Wiersema JR. Altered intrinsic organisation of brain networks implicated in attentional processes in adult attention-deficit/hyperactivity disorder: a resting-state study of attention, default mode and salience network connectivity. Eur Arch Psychiatry Clin Neurosci. (2016) 266:349–57. doi: 10.1007/s00406-015-0630-0
28. Panizzon MS, Fennema-Notestine C, Eyler LT, Jernigan TL, Prom-Wormley E, Neale M, et al. Distinct genetic influences on cortical surface area and cortical thickness. Cereb Cortex. (2009) 19:2728–35. doi: 10.1093/cercor/bhp026
29. Nakao T, Radua J, Rubia K, Mataix-Cols D. Gray matter volume abnormalities in ADHD: voxel-based meta-analysis exploring the effects of age and stimulant medication. Am J Psychiatry. (2011) 168:1154–63. doi: 10.1176/appi.ajp.2011.11020281
30. Lim L, Chantiluke K, Cubillo AI, Smith AB, Simmons A, Mehta MA, et al. Disorder-specific grey matter deficits in attention deficit hyperactivity disorder relative to autism spectrum disorder. Psychol Med. (2015) 45:965–76. doi: 10.1017/S0033291714001974
31. D’Mello AM, Crocetti D, Mostofsky SH, Stoodley CJ. Cerebellar gray matter and lobular volumes correlate with core autism symptoms. NeuroImage Clin. (2015) 7:631–9. doi: 10.1016/j.nicl.2015.02.007
32. Rommelse N, Buitelaar JK, Hartman CA. Structural brain imaging correlates of ASD and ADHD across the lifespan: a hypothesis-generating review on developmental ASD–ADHD subtypes. J Neural Transm. (2017) 124:259–71. doi: 10.1007/s00702-016-1651-1
33. Spronk M, Keane BP, Ito T, Kulkarni K, Ji JL, Anticevic A, et al. A whole-brain and cross-diagnostic perspective on functional brain network dysfunction. Cereb Cortex. (2021) 31:547–61. doi: 10.1093/cercor/bhaa242
34. Morgan SE, White SR, Bullmore ET, Vértes PE. A network neuroscience approach to typical and atypical brain development. Biol Psychiatry Cogn Neurosci Neuroimaging. (2018) 3:754–66. doi: 10.1016/j.bpsc.2018.03.003
35. Vijayakumar N, Ball G, Seal ML, Mundy L, Whittle S, Silk T. The development of structural covariance networks during the transition from childhood to adolescence. Sci Rep. (2021) 11:9451. doi: 10.1038/s41598-021-88918-w
36. Evans AC. Networks of anatomical covariance. NeuroImage. (2013) 80:489–504. doi: 10.1016/j.neuroimage.2013.05.054
37. Duan X, Wang R, Xiao J, Li Y, Huang X, Guo X, et al. Subcortical structural covariance in young children with autism spectrum disorder. Prog Neuropsychopharmacol Biol Psychiatry. (2020) 99:109874. doi: 10.1016/j.pnpbp.2020.109874
38. Bethlehem RAI, Romero-Garcia R, Mak E, Bullmore ET, Baron-Cohen S. Structural covariance networks in children with autism or ADHD. Cereb Cortex. (2017) 27:4267–76. doi: 10.1093/cercor/bhx135
39. Griffiths KR, Grieve SM, Kohn MR, Clarke S, Williams LM, Korgaonkar MS. Altered gray matter organization in children and adolescents with ADHD: a structural covariance connectome study. Transl Psychiatry. (2016) 6:e947. doi: 10.1038/tp.2016.219
40. Sharda M, Khundrakpam BS, Evans AC, Singh NC. Disruption of structural covariance networks for language in autism is modulated by verbal ability. Brain Struct Funct. (2016) 221:1017–32. doi: 10.1007/s00429-014-0953-z
41. Lerch JP, van der Kouwe AJ, Raznahan A, Paus T, Johansen-Berg H, Miller KL, et al. Studying neuroanatomy using MRI. Nat Neurosci. (2017) 20:314–26. doi: 10.1038/nn.4501
42. Hus V, Lord C. The autism diagnostic observation schedule, module 4: revised algorithm and standardized severity scores. J Autism Dev Disord. (2014) 44:1996–2012. doi: 10.1007/s10803-014-2080-3
43. Ickowicz A, Schachar RJ, Sugarman R, Chen SX, Millette C, Cook L. The parent interview for child symptoms: a situation-specific clinical research interview for attention-deficit hyperactivity and related disorders. Can J Psychiatry. (2006) 51:325–8. doi: 10.1177/070674370605100508
44. Snow A. Social communication questionnaire. In: Volkmar FR, editor. Encyclopedia of autism spectrum disorders. New York: Springer (2013). p. 2893–5. doi: 10.1007/978-1-4419-1698-3_1651
45. Fine AH, Kotkin RA. Therapist’s guide to learning and attention disorders. Cambridge, MA: Academic Press (2003).
46. Oakland T, Harrison PL. Adaptive behavior assessment system-II: Clinical use and interpretation. Cambridge, MA: Elsevier, Academic Press (2008).
47. Achenbach TM, Edelbrock CS. Manual for the child behavior checklist and revised child behavior profile. Pediatr Rev. (2000) 21(8):265–71. doi: 10.1542/pir.21-8-265
48. Luriia AR. The working brain: An introduction to neuropsychology. London, United Kingdom: Basic Books (1973).
49. Wechsler D. Wechsler abbreviated scale of intelligence. Washington, DC: American Psychological Association (2012). doi: 10.1037/t15170-000
50. Powel J. Wechsler memory scale-revised David A. Wechsler. New York: the psychological corporation. Harcourt Brace Jovanovich, Inc, 1987. 150 pp. Arch Clin Neuropsychol. (1988) 3:397–403. doi: 10.1016/0887-6177(88)90053-4
51. Parish L, Terman LM, Merrill MA. Stanford-binet intelligence scale. Third revision. Form LM. Br J Educ Stud. 11:88 (1962). doi: 10.2307/3118634
52. Lepage C, Wagstyl K, Jung B, Seidlitz J, Sponheim C, Ungerleider L, et al. CIVET-macaque: an automated pipeline for MRI-based cortical surface generation and cortical thickness in macaques. NeuroImage. (2021) 227:117622. doi: 10.1016/j.neuroimage.2020.117622
53. Lyttelton O, Boucher M, Robbins S, Evans A. An unbiased iterative group registration template for cortical surface analysis. NeuroImage. (2007) 34:1535–44. doi: 10.1016/j.neuroimage.2006.10.041
54. Boucher M, Whitesides S, Evans A. Depth potential function for folding pattern representation, registration and analysis. Med Image Anal. (2009) 13:203–14. doi: 10.1016/j.media.2008.09.001
55. Sled JG, Zijdenbos AP, Evans AC. A nonparametric method for automatic correction of intensity nonuniformity in MRI data. IEEE Trans Med Imaging. (1998) 17:87–97. doi: 10.1109/42.668698
56. Grabner G, Janke AL, Budge MM, Smith D, Pruessner J, Collins DL. Symmetric atlasing and model based segmentation: an application to the hippocampus in older adults. In: Larsen R, Nielsen M, Sporring J, editors. Medical Image Computing and Computer-Assisted Intervention -MICCAI 2006: 9th International Conference, Copenhagen, Denmark, October 1-6, 2006. Proceedings, Part II 9. Springer Berlin Heidelberg (2006). p. 58–6.
57. Smith SM. Fast robust automated brain extraction. Hum Brain Mapp. (2002) 17:143–55. doi: 10.1002/hbm.10062
58. Tohka J, Zijdenbos A, Evans A. Fast and robust parameter estimation for statistical partial volume models in brain MRI. NeuroImage. (2004) 23:84–97. doi: 10.1016/j.neuroimage.2004.05.007
59. Zijdenbos A, Forghani R, Evans A. Automatic quantification of MS lesions in 3D MRI brain data sets: validation of INSECT. In: International Conference on medical image computing and computer-assisted intervention. Berlin, Heidelberg: Springer Berlin Heidelberg (1998). p. 439–48.
60. Kim JS, Singh V, Lee JK, Lerch J, Ad-Dab'bagh Y, MacDonald D, et al. Automated 3-D extraction and evaluation of the inner and outer cortical surfaces using a Laplacian map and partial volume effect classification. NeuroImage. (2005) 27:210–21. doi: 10.1016/j.neuroimage.2005.03.036
61. Lerch JP, Evans AC. Cortical thickness analysis examined through power analysis and a population simulation. NeuroImage. (2005) 24:163–73. doi: 10.1016/j.neuroimage.2004.07.045
62. Pipitone J, Park MT, Winterburn J, Lett TA, Lerch JP, Pruessner JC, et al. Multi-atlas segmentation of the whole hippocampus and subfields using multiple automatically generated templates. NeuroImage. (2014) 101:494–512. doi: 10.1016/j.neuroimage.2014.04.054
63. Park MT, Pipitone J, Baer LH, Winterburn JL, Shah Y, Chavez S, et al. Derivation of high-resolution MRI atlases of the human cerebellum at 3T and segmentation using multiple automatically generated templates. NeuroImage. (2014) 95:217–31. doi: 10.1016/j.neuroimage.2014.03.037
64. Fortin JP, Cullen N, Sheline YI, Taylor WD, Aselcioglu I, Cook PA, et al. Harmonization of cortical thickness measurements across scanners and sites. NeuroImage. (2018) 167:104–20. doi: 10.1016/j.neuroimage.2017.11.024
65. Cook RD. Detection of influential observation in linear regression. Technometrics. (1977) 19:15–8. doi: 10.2307/1268249
66. Van Mieghem P. Graph spectra for complex networks. Cambridge, United Kingdom: Cambridge University Press (2010). doi: 10.1017/CBO9780511921681
67. Sanfeliu A, Fu K-S. A distance measure between attributed relational graphs for pattern recognition. IEEE Trans Syst Man Cybern. (1983) SMC-13:353–62. doi: 10.1109/TSMC.1983.6313167
68. Wang B, Mezlini AM, Demir F, Fiume M, Tu Z, Brudno M, et al. Similarity network fusion for aggregating data types on a genomic scale. Nat Methods. (2014) 11:333–7. doi: 10.1038/nmeth.2810
69. Likas A, Vlassis NJ, Verbeek J. The global k-means clustering algorithm. Pattern Recognit. (2003) 36:451–61. doi: 10.1016/S0031-3203(02)00060-2
70. Davies DL, Bouldin DW. A cluster separation measure. IEEE Trans Pattern Anal Mach Intell. (1979) 1:224–7. doi: 10.1109/TPAMI.1979.4766909
71. Rousseeuw PJ. Silhouettes: a graphical aid to the interpretation and validation of cluster analysis. J Comput Appl Math. (1987) 20:53–65. doi: 10.1016/0377-0427(87)90125-7
72. Calinski T, Harabasz J. A dendrite method for cluster analysis. Commun Stat Theory Methods. (1974) 3:1–27. doi: 10.1080/03610927408827101
73. Gregory S. Finding overlapping communities using disjoint community detection algorithms. In: Complex networks: results of the 2009 international workshop on complex networks (complenet 2009). Berlin, Heidelberg: Springer Berlin Heidelberg (2009). p. 47–61.
74. Steinley D. Properties of the Hubert-Arable adjusted rand index. Psychol Methods. (2004) 9:386–96. doi: 10.1037/1082-989X.9.3.386
75. Jablonski W, Schwaiger J. Stability of the homogeneity and completeness. Verlag der Österreichischen Akademie der Wissenschaften (2006). Vol. 2005, p. SII111–32.
76. Gibbard CR, Ren J, Skuse DH, Clayden JD, Clark CA. Structural connectivity of the amygdala in young adults with autism spectrum disorder. Hum Brain Mapp. (2018) 39:1270–82. doi: 10.1002/hbm.23915
77. Qian L, Li Y, Wang Y, Wang Y, Cheng X, Li C, et al. Shared and distinct topologically structural connectivity patterns in autism spectrum disorder and attention-deficit/hyperactivity disorder. Front Neurosci. (2021) 15:664363. doi: 10.3389/fnins.2021.664363
78. Bralten J, Greven CU, Franke B, Mennes M, Zwiers MP, Rommelse NN, et al. Voxel-based morphometry analysis reveals frontal brain differences in participants with ADHD and their unaffected siblings. J Psychiatry Neurosci. (2016) 41:272–9. doi: 10.1503/jpn.140377
79. Konrad K, Eickhoff SB. Is the ADHD brain wired differently? A review on structural and functional connectivity in attention deficit hyperactivity disorder. Hum Brain Mapp. (2010) 31:904–16. doi: 10.1002/hbm.21058
80. Pievsky MA, McGrath RE. The neurocognitive profile of attention-deficit/hyperactivity disorder: a review of meta-analyses. Arch Clin Neuropsychol. (2018) 33:143–57. doi: 10.1093/arclin/acx055
81. Sáenz AA, Septier M, Van Schuerbeek P, Baijot S, Deconinck N, Defresne P, et al. ADHD and ASD: distinct brain patterns of inhibition-related activation? Transl Psychiatry. (2020) 10:1–10. doi: 10.1038/s41398-020-0707-z
82. Ecker C, Pretzsch CM, Bletsch A, Mann C, Schaefer T, Ambrosino S, et al. Interindividual differences in cortical thickness and their genomic underpinnings in autism spectrum disorder. Am J Psychiatry. (2022) 179:242–54. doi: 10.1176/appi.ajp.2021.20050630
83. Li X, Cao Q, Pu F, Li D, Fan Y, An L, et al. Abnormalities of structural covariance networks in drug-naïve boys with attention deficit hyperactivity disorder. Psychiatry Res Neuroimaging. (2015) 231:273–8. doi: 10.1016/j.pscychresns.2015.01.006
84. Laird AR, Eickhoff SB, Li K, Robin DA, Glahn DC, Fox PT. Investigating the functional heterogeneity of the default mode network using coordinate-based meta-analytic modeling. J Neurosci. (2009) 29:14496–505. doi: 10.1523/JNEUROSCI.4004-09.2009
85. Norman LJ, Sudre G, Price J, Shastri GG, Shaw P. Evidence from “big data” for the default-mode hypothesis of ADHD: a mega-analysis of multiple large samples. Neuropsychopharmacology. (2023) 48:281–9. doi: 10.1038/s41386-022-01408-z
86. Hoogman M, Bralten J, Hibar DP, Mennes M, Zwiers MP, Schweren LSJ, et al. Subcortical brain volume differences in participants with attention deficit hyperactivity disorder in children and adults: a cross-sectional mega-analysis. Lancet Psychiatry. (2017) 4:310–9. doi: 10.1016/S2215-0366(17)30049-4
87. Tremblay LK, Hammill C, Ameis SH, Bhaijiwala M, Mabbott DJ, Anagnostou E, et al. Tracking inhibitory control in youth with ADHD: a multi-modal neuroimaging approach. Front Psychiatry. (2020) 11:4–6. doi: 10.3389/fpsyt.2020.00831
88. Schmithorst VJ, Holland SK. Sex differences in the development of neuroanatomical functional connectivity underlying intelligence found using Bayesian connectivity analysis. NeuroImage. (2007) 35:406–19. doi: 10.1016/j.neuroimage.2006.11.046
89. Greven CU, Richards JS, Buitelaar JK. Sex differences in ADHD, vol. 1. Oxford, United Kingdom: Oxford University Press (2018).
90. van Rooij D, Boedhoe P, Hoogman M. Structural brain differences between and across the ENIGMA OCD, ADHD and ASD cohorts. Biol Psychiatry. (2020) 87:S87. doi: 10.1016/j.biopsych.2020.02.245
91. Mostofsky SH, Cooper KL, Kates WR, Denckla MB, Kaufmann WE. Smaller prefrontal and premotor volumes in boys with attention-deficit/hyperactivity disorder. Biol Psychiatry. (2002) 52:785–94. doi: 10.1016/S0006-3223(02)01412-9
92. Cauvet É, Van't Westeinde A, Toro R, Kuja-Halkola R, Neufeld J, Mevel K, et al. Sex differences along the autism continuum: a twin study of brain structure. Cereb Cortex. (2019) 29:1342–50. doi: 10.1093/cercor/bhy303
Keywords: neurodevelopmental conditions, autism, ADHD, clustering, brain structure, data driven
Citation: Sadat-Nejad Y, Vandewouw MM, Cardy R, Lerch J, Taylor MJ, Iaboni A, Hammill C, Syed B, Brian JA, Kelley E, Ayub M, Crosbie J, Schachar R, Georgiades S, Nicolson R, Anagnostou E and Kushki A (2023) Investigating heterogeneity across autism, ADHD, and typical development using measures of cortical thickness, surface area, cortical/subcortical volume, and structural covariance. Front. Child Adolesc. Psychiatry 2:1171337. doi: 10.3389/frcha.2023.1171337
Received: 22 February 2023; Accepted: 30 August 2023;
Published: 27 September 2023.
Edited by:
Roberto Canitano, Siena University Hospital, ItalyReviewed by:
Alessandra Maria Passarotti, University of Illinois Chicago, United StatesSteve Lukito, King's College London, United Kingdom
© 2023 Sadat-Nejad, Vandewouw, Cardy, Lerch, Taylor, Iaboni, Hammill, Syed, Brian, Kelley, Ayub, Crosbie, Schachar, Georgiades, Nicolson, Anagnostou and Kushki. This is an open-access article distributed under the terms of the Creative Commons Attribution License (CC BY). The use, distribution or reproduction in other forums is permitted, provided the original author(s) and the copyright owner(s) are credited and that the original publication in this journal is cited, in accordance with accepted academic practice. No use, distribution or reproduction is permitted which does not comply with these terms.
*Correspondence: Azadeh Kushki YWt1c2hraUBob2xsYW5kYmxvb3J2aWV3LmNh