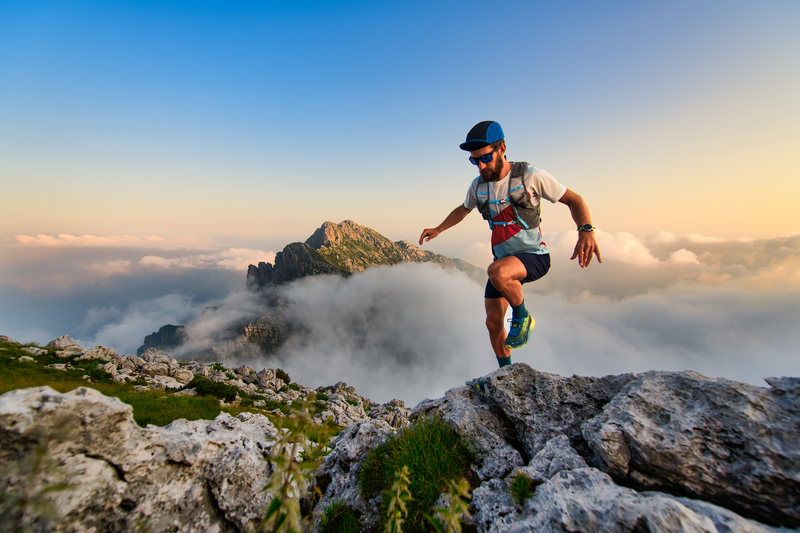
95% of researchers rate our articles as excellent or good
Learn more about the work of our research integrity team to safeguard the quality of each article we publish.
Find out more
ORIGINAL RESEARCH article
Front. Behav. Econ. , 09 December 2024
Sec. Behavioral Microfoundations
Volume 3 - 2024 | https://doi.org/10.3389/frbhe.2024.1490871
This article is part of the Research Topic Sports, Economics, and Natural Experiments: Advances and Retrospection View all 7 articles
Labor economists aspire to understand how workers' productivity impacts pay. While professional football is a well-established domain to explore this relationship, so far, research has relied on basic productivity measures. Football is now awash with advanced and granular performance metrics that can allow a deeper understanding of the pay-performance relationship. We specify a salary model considering the newly available data and use sophisticated performance measures to explain contracted salaries in the English Premier League and Italian Serie A. We make a methodological breakthrough by identifying a sample of players who are in the first year of a new contract only. This results in a much tighter relationship between pay and performance. We estimate different salary equations using both basic and advanced performance statistics. Our main findings are, first, that few of our advanced performance metrics help to explain player salary and, second, that there is misalignment between individual performance determinants of team points and player salaries.
JEL codes: J41, Z22
Over the past decade the production and use of analytics in Association Football has undergone a significant transformation. Almost all elite clubs operate dedicated performance evaluation units and employ specialists in data analysis to support player recruitment [see Adam (2022) and Biermann (2019) for examples]. Developing advanced insights into player performance has become a strategic asset as clubs seek a competitive advantage over rivals. This pursuit has been aided by technological advances, and the wider “digital revolution”; presently, it is possible for commercial data providers to record almost every match event (Gerrard, 2017a).
The increased use of performance data within the industry has been matched by a growing demand for sports analytics (Watanabe et al., 2021). Viewing audiences, fantasy sports enthusiasts, and bettors are but a few communities who take an interest in analytics. So much so, a wealth of performance measures—once the reserve of data providers—have entered the public domain. This is not however the only trend in data availability; researchers have access to improved information on player contracts. Commercial providers now compile contractual details across a range of European leagues. As such, the changes in data environment mean that the information publicly available on European football is converging toward North American sports such as Major League Baseball. That is the context where the “Moneyball1” concept originated and is one which has been well-tested (Lewis, 2004; Hakes and Sauer, 2006, 2007; Brown et al., 2017; Holmes et al., 2018).
The specific contribution of our study lies in making a first attempt to explain footballers' basic pay using advanced and unstudied performance measures, many of which are derived from sports analytics. Importantly, these data allow us to consider both individual performance and players' contributions to team success. This distinction is noteworthy; while we can attribute productivity to individuals, the sports context represents a multi-worker team production environment – outputs are a combination of individual performance inputs that aggregate to team results (see Allen, 2021 and Kempa, 2022). Achieving positive team results (wins or points) is typically (but not exclusively) an objective of managers and owners in team sports. Broadly speaking, our modeling is an advantageous empirical exercise as it offers an applied use of sports analytics, bridging on-field topics of interest in sports analytics and off-field issues relevant to sports economics (see McHale and Holmes, 2023 for a recent example). Previously, the theoretical basis and practicality of sports analytics has been subject to scrutiny (see Szymanski, 2020). We also make a methodological contribution. Our dataset was manually constructed to ensure that the players we sample are in their first years of a contract only. This allows us to estimate a tighter relationship between performance and pay and is a marked departure from past research. Typically, players sign multi-year contracts in football (Buraimo et al., 2015), so it is questionable to assume that predetermined player pay in later years of a contract is correlated with lagged performance measures in a previous season. This is an implicit assumption of the literature to date. The practicalities and significance of this novelty are detailed further in the data section.
Addressing the relationship between pay and performance via analytics is not only of academic significance but is also practically important. Having ‘actionable insight' and an ability to assist decision-makers is an important dimension of analytics (Gerrard, 2017b). Our results can inform both sides of contract negotiations. Specifically, the findings speak to clubs and agents, who act on the players behalf to negotiate pay and contract length. By detecting any performance traits that are connected to team wins but not to an individual's pay, one can point agents and organizations in the direction of any informational inefficiency in the labor market.
The next section provides a brief background to the study by discussing the development and use of analytics in football. Section 3 outlines the theory, surrounding empirical literature and our own methods. Section 4 details the dataset. The results are presented in Section 5. Section 6 concludes the paper.
We are not the first to consider the analytics theme in the context of European football. Whether or not Moneyball is portable from an atomistic sport such as baseball, based on discrete plays, to the fluid and interactive invasive team sport of football, was a question posed by Gerrard (2007). He raised concerns about making this link, including how to accurately identify player actions with and without the ball. More recently, Gerrard (2017b) emphasized other identification problems including defining suitable performance measures and how these can be weighted to offer a team-based appraisal of a player.
Addressing the concerns of Gerrard (2007) was difficult historically, not least because publicly accessible information on player performance was scarce. However, it is now possible to belie certain measurement fears. Many advanced statistics are readily available to improve upon the weaknesses of basic performance measures. First, take the standard statistic of a player's pass completion ratio. This includes short, lateral or defensive passes. Simple pass completion rate does not tell us about the nature of the passes or the quality of the passing (such as whether the ball moves closer to the opponent's goal). Furthermore, it offers no insight concerning the pressure a player is under when the pass was made. While it may capture a general “work-rate”, pass completion is an abstract measure and general pass “accuracy” is not a sharp measure of performance.
Another basic success ratio to consider is shots on target. This straightforward statistic does not offer an insight on how far or the angle from goal a shot was taken, defensive errors contributing to the chance or whether it was easily saveable. Finally, consider goals scored. While fundamental, these data fail to account for the difficulty of the opportunity—there is a great deal of variation in the type and difficulty of goals scored. As we do not know the skills needed to perform what is a rare activity in the context of a match, goals and assists can be overvalued or undervalued depending on context.
Advanced statistics offer superior insights. The type of pass and direction, (e.g., progressive-forward- pass) is now measured. There are many more examples to draw on. For example, the Goal creating Actions (GCA) measure tracks multiple attacking actions that lead to goals. GCA offers an improvement on assists as it accounts for previous contributions in the lead up to a goal. Many more event data are also available including progressive passes, progressive carries, presses, successful dribbles and final third and key passes.
The most widely documented breakthrough relates to commercial firms' construction of Expected Goals (xG) models (Brechot and Flepp, 2020). Expected goals measures are now widely used in football telecasts and match reports. The xG data offer an understanding of how many goals a player ought to have scored (i.e., taking account of a suite of circumstantial factors) and seeks to contextualize attacking threat. In particular, xG is interesting as it is an analytic which provides a normative appraisal of performance.
The canonical model of competitive labor markets implies that workers are paid according to their marginal revenue product. Although various departures exist from the competitive model exist, for example to explain why workers may be paid less than their productivity (monopsony), or the possibility of being paid more than their productivity (efficiency wage theory), the standard assumptions predict a tight relationship between pay and the underlying value of human capital. It is well-established that professional sports offer a tractable domain to test this theory. Accurate wage data is obtainable for professional athletes who function in a well-defined and observable work environment (Bar-Eli et al., 2020). There is a consensus regarding what constitutes productivity, with measurable outputs such as numerical scores attached to wins, losses, or draws. As Kahn (2000) noted, there is no other industry in the world where we know the name, face, performance, and history of every worker. This sentiment remains relevant today, given the technological advances in professional sports and the wealth of data now available beyond the measures highlighted by Kahn (2000).
Measuring player performance in sport is nothing new. Most notably in baseball, the term “Sabermetrics” has a long history. This refers to the quantification of a baseball player's within game activity.2 The use of these statistics to better inform hiring choices in sports, however, is a more recent phenomenon. Lewis' (2004) book Moneyball describes how the Oakland Athletics, led by their General Manager Billy Beane, attempted to build a successful baseball team whilst overcoming its modest revenues due to its position as a small-market team. A specific performance statistic, on-base percentage (OBP), was an undervalued performance measure from 1999 to 2003—the ability to “get on base” predicted wins, but not salaries; however as of 2004 this inefficiency was corrected with the return to OBP increasing for free agents in the years after (Hakes and Sauer, 2006; Brown et al., 2017, but see Holmes et al., 2018, for an alternative interpretation).
Because of poor wage coverage, early estimations of market inefficiencies in football relied on a “market test” approach developed by Szymanski (2000). This method involves regressing total team wage bills on team performance. Since the millennium, a growing body of salary and valuation research has built on this to understand the determinants of pay and valuation in football. Lucifora and Simmons (2003) were the first to study the correlates of pay and superstar effects using Italian (Serie A) wage data reported by the media.
Since Lucifora and Simmons (2003), much of the salary determinants research has depended on media sources (e.g., Kicker Magazine for the German Bundesliga and La Gazzetta Dello Sport for Italy's Serie A). These data, mostly published annually, have become key sources for testing a variety of hypotheses related to both player valuation and pay, and are broadly based on theories of pay determination. The former branch of the literature (valuation) investigates transfer market valuation, whereas we focus on the neighboring topic of explaining pay determinants using a panel of players. A wide range of theory has been tested, including moral hazard effects (Frick, 2011), returns to footedness (Bryson et al., 2013), nationality premia/penalties (Bryson et al., 2014; Farnell et al., 2024), performance consistency (Deutscher and Büschemann, 2016), assortative matching (Drut and Duhautois, 2017), age effects (Fumarco and Rossi, 2018; Scarfe et al., 2024), productivity shocks (Carrieri et al., 2020) and labor specialization (Kempa, 2022). Consistent with this analysis, these studies evaluate pay in European labor markets, where each salary is negotiated by clubs and players through their agents. It is noteworthy that this salary literature also considers wage determination for footballers in an American (MLS) context, where high quality salary data is available from the Players Union. However, these salaries are determined under different labor markets institutions; for example, salary caps exist in the US market (see Butler and Coates, 2022, and Scarfe et al., 2021, for recent MLS applications).
As suggested, much past research relies on a limited set of basic productivity measures or performance composites such as journalist ratings. While typically performance is not the primary factor under investigation in the literature, the basic measures present a challenge to modeling the determinants of pay given the drawbacks in these measures. Regarding performance composites offered by journalists, these can be subjective and potentially biased, especially if they are produced by an insufficient number of reporters.
Of late, increasingly innovative off- and on-field measures are being applied in this branch of research. For example, Carrieri et al. (2018) propose two additional factors that contribute to wages. These are player popularity (measured by the number of Google searches) and bargaining power (the total value of all players represented by the same agent). All three factors are shown to contribute positively to player salaries, with popularity being particularly important at the top end of the salary distribution. Berri et al. (2023a) also innovate by considering the role of analytics in determining pay of goalkeepers (e.g., post-shot expected goals). They find that clubs use primitive defensive statistics but also more sophisticated features of passing to outfield teammates to reward goalkeepers.
Further studies using advanced performance metrics are also beginning to emerge. For example, Weimar and Wicker (2017) examine the contribution of two measures of effort on team performance in the German Bundesliga. They found that total distance run was a strong predictor of match outcomes, but the contribution of the number of intensive runs was less clear. Zaytseva and Shaposhnikov (2022) examine the differing contributions of offensive and defensive actions to team wins. They argue that teams could find ways to win more cheaply if they reallocated portions of their wage spending away from offensive players and toward defensive players.
Our analysis is in two parts. In the first, team points per game is attributed to each player-season observation according to the team that the player appeared for prior to the first year of a new contract.3 The points per game measure is then regressed against a set of individual performance measures. In preliminary estimation, we deleted metrics with insignificant coefficients. These included several defensive measures such as blocks, clearances and interceptions. This leaves us with a set of performance covariates that explain points per game. These are shown in Table 2. We then add a set of team-level covariates, also shown in Table 2, and compare results. Our goal in this part is to assess which of the advanced performance metrics usefully predict team points per game assigned to individual players.
We then proceed to model player salary. The standard approach when specifying a salary equation in the sports economics literature is to express a Mincer type wage equation of the form;
For player i, playing for team j, in season t. The vector X includes a series of (lagged) performance and human capital control variables, and typically, a measure of a team's ability to pay—in this case team fixed effects, ηj. To account for salary inflation, salaries can either be deflated using a consumer price index or might instead be picked up by the season fixed effects, ϕt (similar to Berri et al., 2023b). For studies which include multiple leagues, league fixed effects are also appropriate to include due to institutional differences between the leagues. The inclusion of player fixed effects is rare (see Simmons, 2022), but possible provided researchers have panel data covering a long enough period for each player. Due to our focus on new contracts which results in fewer observations per player, we cannot proceed with the inclusion of player fixed effects. To complete (1), uijt is a random error term.
Note the accepted approach in the literature involves the inclusion of lagged explanatory variables to avoid issues of endogeneity created by reverse causality. This is also quite reasonable as a club's contract offer to a player will be based on their performance in the most recent (past) season. As suggested, by making a sample size trade-off and accessing new contracts only, we maintain that the assumption that past performance impacts pay is especially valid.
We proceed by modeling player salaries first using an OLS regression, with the natural logarithm of salary as the dependent variable. Salary is guaranteed and is assessed at the beginning of a given season. We begin by establishing the general relationship between performance and pay. Following this we consider the relationship between performance and pay using individual and team analytics. As demonstrated in Figure 1, salary reveals excess skewness. This is true of athletes' salaries across many sports leagues. We first attempted to address this non-normality by applying unconditional quantile regression (UQR) to uncover the effects of covariates across the salary distribution. However, initial investigations using this method produced estimates that revealed very few systematic results across quantiles, possibly due to our smaller sample size. This lack of confidence in the UQR results instead led us to apply Huber robust regressions.
Figure 1. Kernel density estimate of gross salary—Premier league and Serie A new contracts, 2018/19–2021/22.
A Huber regression is essentially a weighted least squares estimator. The procedure first performs an initial screening of the data, and based on Cook's distance greater than one eliminates gross outliers. Then, based on residuals, biweights and Huber weights are used iteratively to down-weight the influence of outliers. Naturally, if weights are set to 1, the procedure just collapses back to OLS. The resulting estimator results in standard errors that are robust to heteroskedasticity.
Our data set was merged from multiple online resources. Contract signing information was manually gathered from various websites–e.g., Wikipedia. Salary data were collected from https://www.capology.com and all individual performance statistics and biographical information were sourced from https://www.fbref.com.
We manually constructed a dataset of contracts awarded to 739 professional senior players over four seasons (2018/19–2021/22) from the top divisions of England (the Premier League) and Italy (Serie A). While this sample size is not large in the context of the player salary literature, the novelty is that we only include players who have recently signed a new agreement with a club (via the full range of mechanisms including renewals, loans, or transfers). Thus, we observe players only in the first year of their new contract. Several players sign multiple new contracts over this period. The salary awarded is based on past performances. The length and terms of contracts vary. A minority of players sign only a one-year or rolling contract, whereas the majority (at least in elite leagues) sign contracts lasting between three and six seasons. For example, UEFA benchmarking reports document that the average remaining length of player contracts is 33.5 months in England and 28.9 months in Italy (UEFA, 2022). Regularly, there are extension options included in contracts with these clauses at the behest of clubs and players. Due to the rewards on offer, the evidence suggests that teams generally effectively manage these contracts—there are incentives for clubs to strategically offer longer term contracts (Buraimo et al., 2015). Our analysis is based on 992 player-season observations. We omit goalkeepers, as they have different roles and performance metrics (Berri et al., 2023a). 14 contracts are also omitted because performance data were inaccessible.
The presence of multi-year contracts presents two difficulties for estimating salary equations. First, the salary in a multi-year contract will be determined at the point of signing. This may vary over the course of a contract as part of a pre-determined annual increase, while there may also be some performance-related bonuses included each year.4 On occasion, this type of detail can be substantiated when a player's contract enters the public domain.5 To express salary at any season t as a function of lagged performance variables is misleading. The salary at any season t is determined at the point of signing and by the performance before the contract was signed. Second, performance may vary over the duration of a contract for various reasons (performances of team-mates, injury, form, arrival of new coaches and players, and even strategic behavior by players—see Frick, 2011).
Taken together, these two issues show that modeling player salaries is problematic. While using the same salary awarded to a player does lend itself to a greater sample size and including player (individual level) fixed effects unique to a panel observation, this requires strong assumptions. Hence, we believe studying players in the first year of their contract only is advantageous. Naturally, a trade-off is the loss of observations from players not in the first year of their contracts.
Our new contracts dataset is matched to player salary awards sourced from http://www.capology.com. The salary data is a direct estimate of a player's base salary per-year, not via an algorithm or from crowd valuations as in Transfermarkt market values. Basic pay is the annual/seasonal salary awarded gross of tax and contains no performance-related bonuses or additional income from sponsorships and endorsements. This estimated salary is guaranteed over a year-period and is likely paid in installments. All Premier League salaries were converted to euros.
While the website does contain salary information from other major leagues, only the Premier League and Serie A have a high enough number of verified salaries to be confident in using them. A verified salary is one that is “provided directly by the club or agent, and/or confirmed by at least two sources” (Capology, 2024). At a minimum, Capology states that the figures are based on both “a network of insiders directly involved in contract negotiations as well as news publications around the world” (Capology, 2024). To check the accuracy of the salary data, we triangulated the Serie A salaries with the salary data reported by the Italian sports magazine Gazzetta dello Sport. There was an exact match between the two sources.6
As is usual in sports labor markets, salary is highly skewed; see Figure 1 for a kernel density plot of our salary data. Only a few select players (superstars such as Cristiano Ronaldo) attract exceptional rewards, while the majority of players earn relatively modest salaries in comparison. Considering kernel density plots both by league and by general position also results in similar long tails to the entire sample shown in Figure 1. Table 1 shows the distributional statistics for player salary. A summary of the seasonal-level performance statistics used in the empirical modeling is provided in Table 2.
We access a wider set of advanced variables to model player performance. These data were sourced from FBRef (http://www.fbref.com) who, at the time of data collection, published advanced metrics developed by the commercial firm StatsBomb. It is our understanding that the underlying performance statistics are provided by OPTA—this is the leading sports data provider in Europe. The range of performance metrics can be broadly grouped into categories which capture a player's goal and shot creation ability, passing ability, defensive actions, possession/game involvement, and miscellaneous individual statistics (errors, discipline etc). These can be categorized into sports analytics, where underlying data analysis has occurred (e.g., Expected Goals) and event data that consider advanced aspects of performance (e.g., progressive carries or passes into the final third).
We can also access a distinct set of statistics that evaluate a player's contribution to team outputs (e.g., points earned when a player was performing). It is noteworthy that our dataset contains both the typical basic performance statistics, such as goals, assists, shots on target etc. and advanced analytics. For example, our dataset includes non-penalty expected goals, presses, progressive passes and shot-creating actions as sophisticated performance metrics.
In line with past research modeling salary in football, we collect a range of relevant controls. These include a series of dummy variables on whether the player was a senior international (72%) which we expect to positively impact pay, along with the type of contract the player signed. In particular, whether the transfer was a loan (26%), a free transfer (6%), or a contract renewal (40%). The omitted category is thus a transfer for a fee. We control for the general position of the player [Defender (39%), Midfield (32%) or Attacker/Forward (29%)] and also include controls which capture player experience. We can adopt different measures to do this; for example, using basic measures such as total number of minutes played in senior men's football to date or using the number of elite minutes/minutes in the Big-5 leagues the player has accumulated prior to signing the contract (mean = 8,505, median = 6,586, min = 0, max = 48,061). Finally, we control for the age of the player when the contract is signed (mean = 24.8, median 25, min = 16, max = 37) and its square term. The typical turning point of salary occurs in a player's late 20′s (Frick and Winner, 2020). We also code the club, league and season of each contract to allow for fixed effects in our empirical models.
We first present the models for team points per game in Table 3. In column 1, we use basic performance measures from Table 2. Goals, assists, pass completion percentage, distance carried, and the number of ball touches all contribute positively to team points. The percentage of shots on target and the number of tackles won enter insignificantly. The insignificance of tackles is interesting. Rather than showing defensive skill, many tackles can be seen as an indicator of pressure on defenses that might ultimately lead to goals conceded and concession of points to opponents. Indeed, in preliminary estimation, other defensive metrics such as blocks and interceptions had insignificant coefficients and were ultimately omitted from analysis.
In columns 2 and 3 of Table 3, we proceed to model team points per game using several advanced performance metrics. Many of these do have significant impacts on team points attributable to individual players. These significant coefficients are attached to possession (long pass completion) and offensive features (shot creating actions, progressive distance of passes though not carries). As a general defensive indicator, applicable to all outfield positions, successful presses has a significant, positive effect on points per game. Ball recoveries, however, has a negative and significant coefficient on points. This is again likely a reflection of pressure on defences resulting in fewer points in the season aggregates.
Column 3 of Table 3 introduces two team level variables, in particular the individual player's seasonal plus-minus goals and expected goals measures. Plus-minus was applied by Kharrat et al. (2020) to an analysis of player rankings in European football. Here, we show a significant, positive impact of plus-minus ratings on points per game attributable to individual players. This is not a tautology, as plus-minus is the goal difference that occurs when the individual player is on the pitch. However, we recognize that plus-minus is a consequence of team-mate contributions as well as a given player's actions. When plus-minus is introduced, some but not all of the significant coefficients from column 2 are preserved. Overall, the performance covariates in columns 2 and 3 appear to impact points per game and we proceed to apply these in or player salary models.
Estimates of corresponding salary models are reported in Tables 4–6. With regards to the individual controls, age has the predicted positive but diminishing effect on salaries. The estimated turning point, circa 26 years old, is marginally younger than previous literature suggests, likely due to exclusive focus on the first year of new contracts i.e., this is the age at which player's typically sign their “big” contract. The type of contract signed only has a limited effect on pay (compared to transfers for fees), however, contrary to previous literature (e.g., Berri et al., 2023a), players moving on free transfers appear to receive a salary penalty. This finding is more consistent with research on player movements in the major North American leagues, which suggest that free agents receive salary penalties because clubs, through releasing a player, are signaling that better options are available elsewhere (see Berri and Simmons, 2009 and Berri et al., 2023b, for example). Clubs in the Premier League and Serie A appear to value experience (i.e. minutes played) in other top European leagues than more general experience. Players who have appeared for national teams receive a strong salary premium. This could also reflect unobserved attributes (e.g., leadership, other unobserved performance metrics) that we cannot measure with our data. In line with expectations, forwards are paid more than midfielders, who in turn, are paid more than defenders.
Players are strongly rewarded for goals scored, while there are also some positive salary returns for assists, pass completion rate and touches. Interestingly, tackles are not rewarded in player salary. This could be due to prior mistakes by team-mates, poor positioning, bad team shape and other unobservables. In general, according to Table 4, the defensive statistics do not paint a good picture of defensive ability as a determinant of player pay. We found above that defensive metrics did not contribute much to team points.7 It is worth noting that the correlation between all these variables is relatively low (see Appendix Table A1).
The results from basic performance measures in Table 4 reveal many insignificant coefficients. We progress by using the advanced metrics to ask if they deliver superior insights into pay determination. This is addressed in Table 5.
Table 5 includes the same performance metrics that we showed in columns 2 and 3 of Table 3 as potential, and at times significant, determinants of team points. In the second column we add the plus-minus statistics to the covariates in column 1. The control variables perform similarly to those reported in Table 4.
In terms of how these advanced performance statistics affect player pay, it appears that only the percentage of completed long passes, and the progressive distance of carries show any significant relationship to pay. Defensive metrics are again insignificant. There is some evidence that players are rewarded for presses, though the effect is imprecisely estimated, and drops out of significance when team level variables are added in column 2. Players are also rewarded with respect to how the team performs when they are playing on the pitch (the plus-minus measure). The results hint at executives holding a consequentialist approach, seeking to extract or reward players based on being part of a successful team, regardless of how this success was achieved. This finding may also speak to executives' inability to correctly separate the ability of teammates from the productivity of a single player.
The explanatory power of the basic variables in Table 4 is virtually identical to that of the analytics measures used in Table 5. This casts doubt on the capability of analytics measures to explain player salaries. One reason is that the analytics measures feature defensive and attacking performances regardless of player position while the salary data cover players from all outfield positions. Separate salary estimations by position could offer useful insights. However, in our case the sample sizes would be too small for meaningful inference. Hence, we defer positional salary estimation to further work with a larger data set.
Table 6 models the relationship between pay and performance using Huber robust regressions to account for the potential influence of outliers. For brevity, we do not show the effect of the individual controls, though they perform similarly to those displayed in Tables 4, 5. Reassuringly, the Huber estimator produces similar estimates to those produced by OLS, with the exception of assists and expected goals plus minus. This stability increases our confidence in the validity of the results and the interpretation of our OLS estimates remains intact.
Economic theory proposes a correspondence between player pay and productivity. If labor markets are competitive and informationally rich, workers should be paid according to their levels of human capital. The labor market we study here exhibits both traits. In particular, the football industry has undergone a “data revolution” in recent times. To date, only basic performance measures have been applied to account for skill sets or productivity in football. These measures include statistics such as goals scored, or subjective performance composites published in the press. We offer an improvement on this measurement using new player contracts only.
In alignment with economic theory on wage determination in competitive markets, there should be a tight connection between variables that predict performance and those that predict pay. Slack between this connection is the essence of Moneyball. While we do not seek to find a “golden bullet” performance metric for football (like on-base percentage in the case of baseball), the similarities and differences across our win and pay models are of interest. As suggested at the outset, consistencies and misalignments between these results offer practical implications for those negotiating salaries.
There are some variables consistent between the team performance models and the salary models, especially for the basic performance measures (Tables 3, 4), but less so for the advanced performance statistics (Tables 3, 5). In particular, goals, assists, pass completion, and touches are predictors of both salary and team points (though assists are imprecisely estimated in Table 4). Tackles are not a predictor of either team points or salaries, though we have discussed some potential reasons for this throughout. In fact, of our basic performance variables, it is only distance carried that shows any discrepancy. Distance is a strong predictor of team points, but it would appear to not be valued by teams owing to its insignificance in the salary models.
From Table 5, long pass completion is also a key predictor of both salary and team points. Progressive carries are valued by teams in that they are rewarded by higher salaries, but they do not predict wins. The opposite can be said of progressive passes. Successful presses and shot creating actions are a strong predictor of team points (though the effects are dampened when team measures are included), but there is much weaker evidence that teams reward players for these.
A natural question to ask is why clubs are not using advanced statistics more? We can only offer conjectures. It is possible that the clubs do not fully trust the measures—they are not all context-free and are observed/coded by humans based on malleable definitions decided by private firms. Moreover, as we noted earlier, our performance metrics are necessarily individual and do not properly capture teammate interactions or productivity spillovers. Investigating these would require game level analysis.
We suspect that club salary negotiators find it difficult to separate out individual contributions to team performances from teammate contributions. Further work could usefully explore teammate interactions where, for example, successful presses of teammates is entered alongside successful presses of individual players. We again defer this exercise to future work.
While the advanced statistics are sharper than clearer but basic measures, clubs may believe that they do not sufficiently cover a full player evaluation, especially a player's defensive capabilities. As highlighted, with defensive traits, optimal defensive performance is often about what is not recorded in the statistics—this issue presents future research opportunities to consider the relationship between pay and what a player does not do.
We can reach out to other explanations. Is there a possibility that the individual statistics are too informationally rich (or contain too much noise) for executives and salary negotiations? A compelling argument—that could be teased out of our results—is that executives use individual inputs to team output as a guiding metric, and do not require or ignore the glut of information on the underlying productivity drivers. For example, in Table 5 it is interesting that plus minus is significant, whilst expected goals plus minus is not. Both are important in the team points per game model. As the findings of Flepp and Franck (2021) imply, performance measured with expected goals is more informative than match outcomes. It would make sense to base salary awards on this metric, but our results seem to suggest that decision-makers are biased toward the simple information contained in match outcomes, even if those are a lot more random than expected goals. Boundedly rational decision-making models and associated behavioral heuristics may offer some guidance on why few individual analytics connect to pay determination.
Several more routes forward are worth noting. While we have access to advanced analytics, other measures are available to clubs but remain private to researchers (e.g., sprint rates, passing sequence data or activity during specific match periods). This is a limitation of this work and even more advanced metrics may offer sharper insights. Future work could also explore if the player's performance before the start of the contract can affect the pre-determined average yearly wage over the ex-ante duration of the contract or ask if longer historical statistics of players are important to new contracts.
Finally, the measures we adopt do not capture psychological factors or other intangibles—regularly managers and executives express views on the importance of player personality, resilience and response to adversity in matches; the relationship between psychological measures and player pay is another natural route forward for this literature.
Publicly available datasets were analyzed in this study. This data can be found here: https://www.capology.com/ and https://fbref.com/en/.
Ethical review and approval was not required for the study on human participants in accordance with the local legislation and institutional requirements. Written informed consent from the participants was not required to participate in this study in accordance with the national legislation and the institutional requirements.
DB: Writing – review & editing, Writing – original draft, Visualization, Validation, Software, Resources, Project administration, Methodology, Investigation, Formal analysis, Data curation, Conceptualization. AF: Writing – review & editing, Writing – original draft, Visualization, Validation, Software, Resources, Project administration, Methodology, Investigation, Formal analysis, Data curation, Conceptualization. RS: Writing – review & editing, Writing – original draft, Visualization, Validation, Software, Resources, Project administration, Methodology, Investigation, Formal analysis, Data curation, Conceptualization.
The author(s) declare that no financial support was received for the research, authorship, and/or publication of this article.
We thank conference participants at Western Economic Association International, San Diego and European Sports Economics Association, Cork for helpful comments. We also acknowledge feedback from seminars at University College Cork, University of Reading (ROSES), Lancaster University and University of Zurich.
The authors declare that the research was conducted in the absence of any commercial or financial relationships that could be construed as a potential conflict of interest.
All claims expressed in this article are solely those of the authors and do not necessarily represent those of their affiliated organizations, or those of the publisher, the editors and the reviewers. Any product that may be evaluated in this article, or claim that may be made by its manufacturer, is not guaranteed or endorsed by the publisher.
The Supplementary Material for this article can be found online at: https://www.frontiersin.org/articles/10.3389/frbhe.2024.1490871/full#supplementary-material
1. ^The idea that organisations can exploit mispricing of human capital using analytics.
2. ^In football many elite clubs operate analytics departments. However, these operations tend to be private; and their activities less publicised, though fleeting examples do exist. Notable examples include Brentford FC and Liverpool FC in the English Premier League, and FC Midtjylland in the Danish league.
3. ^In other words, if a player moves between teams, the relevant points per game we consider is the team from which the player moved from.
4. ^We do not observe any bonus salaries paid to players. While information on bonuses is available, as a researcher we do not know the structure and determinants of these bonuses.
5. ^For example, Juan Mata's contract whilst playing at Chelsea FC is freely available online. The player was paid a basic salary over five years which increases in year two of the contract. There is a flat wage profile for the remaining 4 years. A signing on fee is paid in increments over the length of the contract.
6. ^Although either Capology or Gazzetta dello Sport may have accessed each other's data (as both are public), the consistency between the estimates is encouraging.
7. ^We explored using positional interactions but given the sample size and increased complexity of the modelling we lacked confidence in the model fit and the reliability of the results.
Adam, D. (2022). Science and the World Cup: how big data is transforming football. Nature 611, 444–446. doi: 10.1038/d41586-022-03698-1
Allen, W. D. (2021). Work environment and worker performance: a view from the goal crease. J. Labor Res. 42, 418–448. doi: 10.1007/s12122-021-09323-w
Bar-Eli, M., Krumer, A., and Morgulev, E. (2020). Ask not what economics can do for sports-Ask what sports can do for economics. J. Behav. Experim. Econ. 89:101597. doi: 10.1016/j.socec.2020.101597
Berri, D., Butler, D., Rossi, G., Simmons, R., and Tordoff, C. (2023a). Salary determination in professional football: empirical evidence from goalkeepers. Eur. Sport Managem. Quart. 24, 624–640. doi: 10.1080/16184742.2023.2169319
Berri, D., Farnell, A., and Simmons, R. (2023b). The determinants of Black quarterback pay in the National Football League. Manager. Deci. Econ. 44, 1491–1503. doi: 10.1002/mde.3760
Berri, D., and Simmons, R. (2009). Race and the evaluation of signal callers in the National Football League. J. Sports Econom. 10, 23–43 doi: 10.1177/1527002508327383
Biermann, C. (2019). Football hackers: The Science and Art of a Data Revolution. London: Kings Road Publishing.
Brechot, M., and Flepp, R. (2020). Dealing with randomness in match outcomes: how to rethink performance evaluation in European club football using expected goals. J. Sports Econom. 21, 335–362. doi: 10.1177/1527002519897962
Brown, D. T., Link, C. R., and Rubin, S. L. (2017). Moneyball after 10 years: how have Major League Baseball salaries adjusted? J. Sports Econom. 18, 771–786. doi: 10.1177/1527002515609665
Bryson, A., Frick, B., and Simmons, R. (2013). The returns to scarce talent: footedness and player remuneration in European soccer. J. Sports Econom. 14, 606–628. doi: 10.1177/1527002511435118
Bryson, A., Rossi, G., and Simmons, R. (2014). The migrant wage premium in professional football: a superstar effect? Kyklos 67, 12–28. doi: 10.1111/kykl.12041
Buraimo, B., Frick, B., Hickfang, M., and Simmons, R. (2015). The economics of long-term contracts in the footballers' labour market. Scott. J. Polit. Econ. 62, 8–24. doi: 10.1111/sjpe.12064
Butler, D., and Coates, D. (2022). Position premium in major League Soccer. Int. J. Sport Finance 17, 201–214. doi: 10.32731/ijsf/174.112022.02
Capology. (2024). Features. Capology.Available at: https://www.capology.com/features/
Carrieri, V., Jones, A. M., and Principe, F. (2020). Productivity shocks and labour market outcomes for top earners: evidence from Italian Serie A. Oxf. Bull. Econ. Stat. 82, 549–576. doi: 10.1111/obes.12347
Carrieri, V., Principe, F., and Raitano, M. (2018). What makes you ‘super-rich'? New evidence from an analysis of football players' wages. Oxford Econ. Papers 70, 950–973. doi: 10.1093/oep/gpy025
Deutscher, C., and Büschemann, A. (2016). Does performance consistency pay off financially for players? Evidence from the Bundesliga. J. Sports Econ. 17, 27–43. doi: 10.1177/1527002514521428
Drut, B., and Duhautois, R. (2017). Assortative matching using soccer data: Evidence of mobility bias. J. Sports Econom. 18, 431–447. doi: 10.1177/1527002515588134
Farnell, A., Butler, D., Rossi, G., Simmons, R., Berri, D., and Bamba, E. Y. (2024). Is there a nationality wage premium in European football? Sports Econ. Rev. 7:100040. doi: 10.1016/j.serev.2024.100040
Flepp, R., and Franck, E. (2021). The performance effects of wise and unwise managerial dismissals. Econ. Inq. 59, 186–198. doi: 10.1111/ecin.12924
Frick, B. (2011). Performance, salaries, and contract length: Empirical evidence from German soccer. Int. J. Sports Finance 6, 87–118.
Frick, B., and Winner, H. (2020). “Deferred compensation when monitoring is (nearly) costless: evidence from professional football,” in Outcome Uncertainty in Sporting Events, eds. P. Rodriguez, S. Kesenne, and B. R. Humphreys (Edward Elgar Publishing), 63–74.
Fumarco, L., and Rossi, G. (2018). The relative age effect on labour market outcomes–evidence from Italian football. Eur. Sport Manage. Quart. 18, 501–516. doi: 10.1080/16184742.2018.1424225
Gerrard, B. (2007). Is the Moneyball approach transferable to complex invasion team sports? Int. J. Sports Finance 2, 214–225.
Gerrard, B. (2017a). “Analytics, technology and high-performance sport,” in Critical Issues in Global Sport Management, eds. N. Schulenkorf, and S. Frawley (Oxfordshire: Routledge), 205–219.
Gerrard, B. (2017b). “The role of analytics in assessing playing talent,” in The Handbook of Talent Identification and Development in Sport. Routledge International Handbooks, eds. Baker, J, Cobley, S, Schorer, J and Wattie, N (London: Routledge).
Hakes, J. K., and Sauer, R. D. (2006). An economic evaluation of the Moneyball hypothesis. J. Econ. Persp. 20, 173–185. doi: 10.1257/jep.20.3.173
Hakes, J. K., and Sauer, R. D. (2007). The Moneyball anomaly and payroll efficiency. Int. J. Sports Finance 2, 177–189.
Holmes, P. M., Simmons, R., and Berri, D. J. (2018). Moneyball and the baseball players' labor market. Int. J. Sport Finan. 13, 141–155.
Kahn, L. M. (2000). The sports business as a labor market laboratory. J. Econ. Perspect. 14, 75–94. doi: 10.1257/jep.14.3.75
Kempa, K. (2022). Task-specific human capital and returns to specialization: evidence from association football. Oxf. Econ. Pap. 74, 136–154. doi: 10.1093/oep/gpab006
Kharrat, T., McHale, I. G., and Peña, J. L. (2020). Plus–minus player ratings for soccer. Eur. J. Oper. Res. 283, 726–736. doi: 10.1016/j.ejor.2019.11.026
Lucifora, C., and Simmons, R. (2003). Superstar effects in sport: Evidence from Italian soccer. J. Sports Econom. 4, 35–55. doi: 10.1177/1527002502239657
McHale, I. G., and Holmes, B. (2023). Estimating transfer fees of professional footballers using advanced performance metrics and machine learning. Eur. J. Oper. Res. 306, 389–399. doi: 10.1016/j.ejor.2022.06.033
Scarfe, R., Singleton, C., Sunmoni, A., and Telemo, P. (2024). The age-wage-productivity puzzle: Evidence from the careers of top earners. Econ. Inq. 62, 584–606. doi: 10.1111/ecin.13191
Scarfe, R., Singleton, C., and Telemo, P. (2021). Extreme wages, performance, and superstars in a market for footballers. Ind. Relat.: J. Econ. Soc. 60, 84–118. doi: 10.1111/irel.12270
Simmons, R. (2022). Professional labor markets in the Journal of Sports Economics. J. Sports Econom. 23, 728–748. doi: 10.1177/15270025211051062
Szymanski, S. (2000). A market test for discrimination in the English professional soccer leagues. J. Polit. Econ. 108, 590–603. doi: 10.1086/262130
Szymanski, S. (2020). Sport analytics: science or alchemy? Kinesiol. Rev. 9, 57–63. doi: 10.1123/kr.2019-0066
UEFA (2022). The European Club Footballing Landscape: Club Licensing Benchmarking Report Financial Year 2022. Available at: https://editorial.uefa.com/resources/027e-174740f39cc6-d205dd2e86bf-1000/ecfl_bm_report_2022_high_resolution_.pdf (accessed May 20, 2023).
Watanabe, N. M., Shapiro, S., and Drayer, J. (2021). Big data and analytics in sport management. J. Sport. Manage. 35, 197–202. doi: 10.1123/jsm.2021-0067
Weimar, D., and Wicker, P. (2017). Moneyball revisited: Effort and team performance in professional soccer. J. Sports Econom. 18, 140–161. doi: 10.1177/1527002514561789
Keywords: salary, sports analytics, football, soccer, contracts
Citation: Butler D, Farnell A and Simmons R (2024) Do sports analytics affect footballer pay? Front. Behav. Econ. 3:1490871. doi: 10.3389/frbhe.2024.1490871
Received: 03 September 2024; Accepted: 14 October 2024;
Published: 09 December 2024.
Edited by:
Raphael Flepp, University of Zurich, SwitzerlandReviewed by:
Kaori Narita, University of Liverpool, United KingdomCopyright © 2024 Butler, Farnell and Simmons. This is an open-access article distributed under the terms of the Creative Commons Attribution License (CC BY). The use, distribution or reproduction in other forums is permitted, provided the original author(s) and the copyright owner(s) are credited and that the original publication in this journal is cited, in accordance with accepted academic practice. No use, distribution or reproduction is permitted which does not comply with these terms.
*Correspondence: David Butler, RGF2aWQuYnV0bGVyQHVjYy5pZQ==
Disclaimer: All claims expressed in this article are solely those of the authors and do not necessarily represent those of their affiliated organizations, or those of the publisher, the editors and the reviewers. Any product that may be evaluated in this article or claim that may be made by its manufacturer is not guaranteed or endorsed by the publisher.
Research integrity at Frontiers
Learn more about the work of our research integrity team to safeguard the quality of each article we publish.