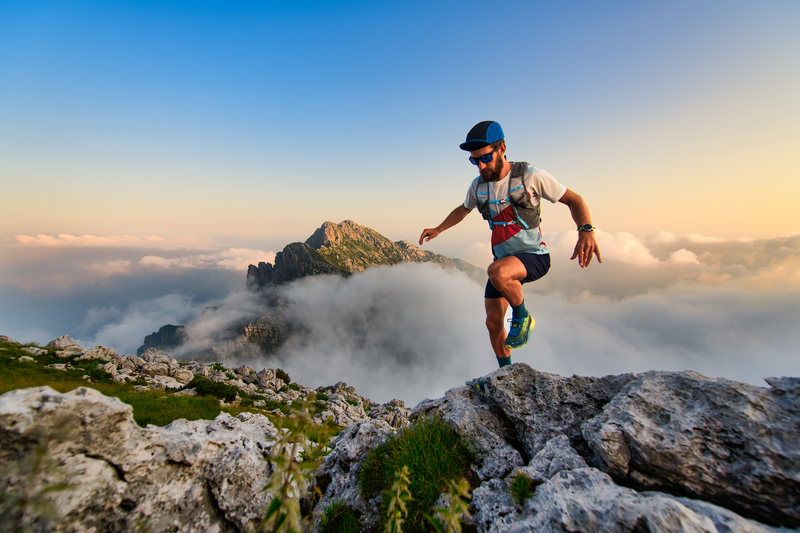
94% of researchers rate our articles as excellent or good
Learn more about the work of our research integrity team to safeguard the quality of each article we publish.
Find out more
BRIEF RESEARCH REPORT article
Front. Behav. Econ. , 28 September 2023
Sec. Culture and Ethics
Volume 2 - 2023 | https://doi.org/10.3389/frbhe.2023.1232462
This article is part of the Research Topic The Ethics and Behavioral Economics of Human-AI Interactions View all 7 articles
Mental health is an integral part of human well-being (Prince et al., 2007), but it often does not receive due attention, especially in developing economies. One hundred and fifty million people in India alone require mental health support, but those in need of support might be reluctant to seek it (Gautham et al., 2020) for several reasons, including stigma surrounding mental distress, lack of awareness, lack of access to support, or high costs (Wies et al., 2021). In addition, COVID-19 and restrictions on physical interactions imposed new challenges in providing mental health support.
Technologies such as mental health mobile apps may play a significant role in providing support to individuals in need of mental health support (Neary and Schueller, 2018). These apps can contribute to bridging the treatment gap for mental health issues (Alonso et al., 2018) and mitigate the “hidden” mental health pandemic (Bower et al., 2022) by facilitating connections between patients and available providers at a relatively low cost and with high flexibility in terms of time and location, all while maintaining privacy. Typically, the therapy itself is not automated and is performed by a qualified human provider (i.e., a therapist), as patient-doctor relations are paramount for quality patient care (Brown and Halpern, 2021; Schultz et al., 2021). Yet, some steps of the mediation and matching between the patients and therapists might be automated to allow performing at scale and keeping the cost low.
When it comes to mental health, deciding whether to automate certain processes, maintain human engagement, or adopt an in-between solution seems to be particularly challenging. The type of interaction partner matters for the behavioral responses this interaction triggers. A robust empirical finding is that hybrid interactions, i.e., interactions between humans and agents powered by technology, trigger a less emotional and social response than a human-human interaction would (Chugunova and Sele, 2022). The reduced emotional and social response can improve outcomes in interactions that benefit from increased rationality but can be harmful when emotions or social rules of conduct are crucial drivers for beneficial outcomes. In the domain of digital mental health, it means that keeping a human mediator involved may be beneficial due to a higher emotional response, but at the same time, automating an interaction and highlighting the non-human nature of the opponent may be beneficial given lower social response to such agents and stigma surrounding mental health (Bharadwaj et al., 2017). In this paper, we consider how highlighting the (non-)human nature of an interaction partner affects compliance with prompts measured by the propensity to follow them in the digital mental health setting.
To examine this question, we partner with one of India's largest mental health apps, TickTalkTo. TickTalkTo acts as an intermediary and matches users to therapists who deliver qualified medical services. When interacting with the app, a user is assigned to a human mediator who serves as a contact person and guides the user toward a match with a therapist. During the first interaction, a user sees a series of messages that introduce the human mediator and prompt them to take a short psychological assessment which is used to assist the matching. Users were randomly assigned to be either in the Human condition or in the System condition. The conditions varied in how (almost) identical messages were presented: in the System condition, through message subscripts and wording, it was emphasized that the messages were sent by the System. Conversely, in the Human condition, it was emphasized that they were sent (on behalf of) the human mediator (see Figure 1 to compare the treatment conditions). These messages appeared immediately when the chat screen opened, were standard and sent automatically in both conditions on behalf of the assigned human mediator. We address our question in a field experimental setting and are mindful of seamlessly integrating it into the environment that users would normally encounter and not interfere with the regular process. To do so, we abstain from introducing additional incentives or questions. We believe that in our setting, participants are intrinsically motivated to take the interaction with the app seriously, as they actually search for mental health support and, therefore, do not require additional incentives. As we do not ask additional questions, we restrict our analysis to considering average treatment effects.
Figure 1. Communication with a user in the Human (A) and the System (B) conditions. The treatment differences are highlighted. The shade of the gray subscripts has been intensified to accommodate variations between the phone screen (participant experience) and a printed version or a computer screen (reader experience).
We find that this subtle change has a dramatic effect on compliance: Following the prompt in the Human condition, an additional 21.75% of users start the psychological assessment. Once users start the assessment, they are equally likely to complete it in both conditions. Yet we observe an additional positive effect of the Human condition: users who complete the assessment are more likely to open the results. These results are particularly relevant given the high levels of attrition to online mental health tools (Naslund et al., 2017).
Our results reinforce and extend previous findings. A recent paper by Hussein and Huang (2020) considers the use of AI in the domain of digital mental health. In a series of vignette studies, authors find that participants prefer human as compared to AI therapists due to a potential lack of warmth and, as a consequence, the lower competence of an AI therapist. Previously, Promberger and Baron (2006) also used hypothetical medical situations to establish that participants are more likely to follow a recommendation for an operation from a human physician than a computer. Our findings extend these results in the field setting. Most importantly, we document higher compliance with a human prompt, even in a task that does not require a high level of competence of the automated counterpart nor a high level of trust between the parties. Most recent advances of large language models such as ChatGPT made it possible for chatbots to answer patients' questions that are superior to doctors' answers on several metrics (Ayers et al., 2023). Our results highlight the fact that apart from the technical feasibility of automation, behavioral responses of users need to be taken into account when deciding what processes should be automated or if human involvement should be emphasized. In the context of digital mental health, it appears that retaining a human mediator and highlighting their involvement leads to more compliance.
This paper contributes to two strands of literature. First, digitization in health care has shown great potential but also challenges in adoption, and our paper advances our understanding of adoption and compliance. Prior studies find positive impacts of health information technologies on patient health through reducing mortality or morbidity among infants, patients with complex conditions, and opioid-related conditions (Miller and Tucker, 2011; McCullough et al., 2016; Wang, 2021). Digital solutions can improve mental health conditions such as depression across various patient populations (e.g., Lattie et al., 2019). The introduction of technology can affect not only the treatment delivery itself, but also a multitude of choices related to search and adherence to treatment. For instance, people were found to be responsive to (algorithmic) advice when choosing health care plans (Bundorf et al., 2019). If doctors consulted automated decision supports for making a diagnosis, they were perceived as less qualified than when they consulted human colleagues (Arkes et al., 2007; Shaffer et al., 2013). Our research brings in new field experimental evidence that can improve the adoption of digital mental health support tools.
Second, this paper builds on dynamic and fast-growing literature on human interaction with technology and behavioral responses to automation. A robust finding from an extensive literature review is that interacting with automated agents triggers reduced emotional and social responses as compared to a human-human interaction (see, e.g., Chugunova and Sele, 2022, for an overview). In some contexts, the reduced emotional and social response leads to beneficial outcomes such as, for example, increased disclosure of intimate partner violence if reporting to computers (Ahmad et al., 2009; Humphreys et al., 2011). Moreover, algorithms appear to enjoy the perceived “halo” of scientific authority and objectivity (Cowgill et al., 2020). Users also tend to incorporate recommendations from an algorithm more than those from other humans (Logg et al., 2019; Sele and Chugunova, 2022). All these findings suggest that highlighting that the prompt comes from a system and de-emphasizes human involvement will increase compliance.
However, reactions to algorithms tend to be very context-specific, with people being more accepting of the algorithms in tasks that are seen as more analytical and less social (Waytz and Norton, 2014; Hertz and Wiese, 2019; Castelo et al., 2019). In the financial services domain, Luo et al. (2019) find that disclosing the non-human nature of the chatbot significantly decreases the rate of purchases compared to when users are unaware that they communicate with a chatbot. In general, several studies documented that disclosing the nature of chatbots has negative effects on both psychological and behavioral user reactions (e.g., Murgia et al., 2016). Focusing on the use of AI in the medical domain, Longoni et al. (2019) find that participants are more likely to follow medical advice when it comes from a human provider rather than an AI. The suggested mechanism is “uniqueness neglect,” i.e., the concern that an AI is unable to account for unique characteristics and circumstances. In the context of our study, the latter findings would rather suggest that informing users that the prompt is delivered by a system and de-emphasizing human engagement may reduce compliance. Therefore, based on the existing evidence, it is a priori unclear if highlighting the engagement of a system or a human will increase or decrease compliance.
To study what is the role of highlighting human or system involvement for compliance, we conduct a field experiment. All new users of the TickTalkTo app between February and March 20221 took part in the study. In the app, a user was assigned to a human mediator—a contact person who assisted in establishing a successful match with a therapist. During the first interaction with the app, users saw a picture of the assigned human mediator, and upon clicking on it, reached the chat screen. On the chat screen, they immediately saw a series of messages that introduced the assigned human mediator by name and, as a first step, asked users to fill in a psychological assessment, which could improve the match suggestion. Participants were randomly assigned to either the System or the Human condition. The difference between the conditions was subtle and manifested itself in the subscripts under the otherwise (almost) identical messages. In the System condition, it was highlighted that the messages were sent by the system (i.e., automatically), whereas in the Human condition that they were sent (on behalf of) the human mediator. Additionally, one message was adjusted to inform who enabled the psychological assessment (“The system” and “I” respectively) and one highlighted that the assessment will be used for matching either by a human or by an algorithm.2 Figure 1 depicts the difference between conditions.
Several caveats are important to note: First, all the messages appeared on the screen immediately, which might limit users' perception of how much human involvement was feasible. Second, in the second message of the System condition, the human mediator was introduced by name, albeit with a subscript indicating that the message was sent by the system. By varying the label and keeping the timing and the content of the messages comparable, we can consider the role of emphasizing the source of the messages for compliance. The prompt to take the assessment came as one of the first interactions with an app. That is, there was no previous communication with the prompt sources or experience with the app that could have affected compliance with a prompt. Completing the psychological assessment was not mandatory and users could proceed without completing it. Users who started the assessment did so typically within a minute upon receiving the prompt (median of 56.6 s with a long tail on the right side of the distribution).3 The follow-up communication in both treatments was delivered by a human mediator and clearly marked as such. Therefore, even if the delay between receiving the prompt might have affected the uptake of the psychological assessment, it would serve as a conservative estimate of our intervention.
Our sample consists of 1,072 app users with 603 (56.25%) randomly assigned to the Human condition and 469 (43.75%) to the System condition.4 The randomization was implemented as an independent random draw at the time of downloading the app that with a 50% chance assigned a user to the Human or the System condition. We analyze the behavior of users who actually interacted with the app and therefore were exposed to one of the treatments (per-protocol analysis). Some users who downloaded the app never registered and never interacted with it. Attrition before any interaction with the app does not challenge the randomization and identification strategy. Given the field setting of the study, we do not have any additional information about the users and are not able to consider heterogeneities of the treatment effect (Rahman et al., 2022).5
Our main outcome variables are the uptake rate of the psychological assessment, the completion rates, and engagement with the assessment results (i.e., clicking on the report link). Additionally, based on the timestamps, we can see if participants took longer to proceed to the next step, depending on the treatment.
In the Human condition, 35.82% of the users started the psychological assessment after receiving the prompt. In the System condition, only 29.42% did so. Keeping in mind how subtle the intervention was, the increase in compliance of 21.75% in the Human condition is substantial and significantly different from the System condition (6.40 pp, two-sided t-test, p = 0.02). Once the psychological assessment was started, about 72% of users completed it. The attrition rates in both conditions are not significantly different (two-sided t-test, p = 0.85). In addition, apart from the increase in the initial propensity to start the assessment, introducing the prompt highlighting the human increases general engagement with the assessment. Almost all the users who completed the psychological assessment (97.6%) also opened the results report. Yet, this small share of users who did not open the report is concentrated in the System condition (two-sided t-test, p = 0.02). A median user took 3.5 min to complete the psychological assessment. There are no systematic differences in completion times by condition (t-test, p = 0.33).6 We reconfirm our results in a series of regressions (see Table 1).
We observe a significant increase in compliance and engagement when the prompt to complete a psychological assessment is marked as delivered by a human mediator, as compared to a system. Data limitations do not allow us to empirically study the mechanisms that triggered the effects. However, based on the previous literature, several mechanisms might be at play.
First, although interactions with a system are social interactions (Nass et al., 1999; Nass and Moon, 2000), there might be a stronger norm of politeness or conformity toward a human (Cormier et al., 2013) that would affect the decision to complete a psychological assessment when asked. Second, in the spirit of “uniqueness” neglect (Longoni et al., 2019), it is possible that when the prompt came from a system, it was dismissed as a standard feature (which it is). When it comes from a human, it is seen as necessary for one's particular case. However, the fact that the messages appeared on the screen all at once both in the Human and the System condition and that there was no previous communication between the parties when the prompt appeared might cast doubt onto how much “customization” was possible at that point. Finally, the likelihood of completing a psychological assessment might be related to performance expectations about an algorithm and a human in terms of the quality of the match. If users believe that a match suggested by a human would be worse than a match suggested by a system, they may be more likely to complete the assessment in the human condition to offer additional inputs for the match and thus improve human performance. Yet, for this argument to hold, we have to assume that users are interested not in the best possible match but in a match above some threshold or that users believe that the psychological assessment data would improve the match differentially by treatment.
While the effect of highlighting that the messages come from a human mediator is significant and positive, the exact magnitude of the effect (21.75%) is context specific. In particular, the initial compliance with the prompt in the System condition might have been lower due to the content of the second message. In the second message, the system might be viewed as introducing itself with the human name (message 2 in the examples: “I'm Mridula, [...]”). It might have been regarded as the system trying to hide its identity and pretend to be a human. However, as this message as well as all others were followed by the subscript that it was sent by the system, the negative effect of the mismatch between the wording and the subscript might exist, but is probably limited. Importantly, we consider an ethically uncontroversial case where a human mediator was present and engaged in the communication following the standard welcome messages. In situations where a chatbot attempts to persuade the user that it is a human, the user's reactions might drastically differ. Ishowo-Oloko et al. (2019) find that participants cooperate with bots less after they interacted with them believing that they were human. However, the authors attribute the change to the prejudice against bots and not to the false identity. In general, large literature considers the practice of not disclosing the nature of the chatbot and letting the chatbot pretend to be a human (e.g., Shi et al., 2020; Murgia et al., 2016). With that in mind, some legal regulations require that users are informed when they interact with an AI system [Article 52(1) of the EU AI Act proposal] or, specifically, a bot (California's BOT Disclosure Law).
The goal of the current study was not to establish correlations between demographic characteristics such as, for example, age, gender, and attitudes toward technology (e.g., Grzymek and Puntschuh, 2019). Due to randomization, the demographic composition of participants in both conditions is the same in expectation. While considering heterogeneities might be interesting for future studies, our main findings on the aggregate level remain valid and important for the use of automation for mental health at scale.
With the development of digital health and, in particular digital mental health, society is facing great potential and challenges in how to harness this potential. In this paper, based on the empirical evidence from a field experiment, we provide evidence that highlighting the engagement of a human mediator in sending otherwise standard messages leads to an increase in compliance in the domain of mental health. Further research is needed to understand if our findings extend to other domains, what mechanisms underlie the effect, and how digital health care providers could deliver better and more affordable care at scale.
The raw data supporting the conclusions of this article will be made available by the authors, without undue reservation.
The studies involving humans were approved by Ethic approvals of IIMA IRB 2021-26 and MPG 2021-20. The studies were conducted in accordance with the local legislation and institutional requirements. Written informed consent for participation was not required from the participants or the participants' legal guardians/next of kin because the study was conducted in the field setting and involved minimal risk to participants. When registering with the app participants agree to terms and conditions which include a clause of possible research to improve the service.
All authors meaningfully contributed to the research project that resulted in the manuscript. All authors took part in all the stages of the process from the idea to conceptualization, implementation, analysis of the data, and drafting of the manuscript. All authors contributed to the article and approved the submitted version. The author sequence is alphabetical.
MC gratefully acknowledges support by DFG CRC TRR 190 (project number 280092119).
AS is the founder of TickTalkTo. As a field partner of the research study, he was involved in the study development and design, as well as providing the data for analysis for the remaining authors. He was not involved in the analysis and interpretation of the data.
The remaining authors declare that the research was conducted in the absence of any commercial or financial relationships that could be construed as a potential conflict of interest.
The author(s) [MC, LXW] declared that they were an editorial board member of Frontiers, at the time of submission. This had no impact on the peer review process and the final decision.
All claims expressed in this article are solely those of the authors and do not necessarily represent those of their affiliated organizations, or those of the publisher, the editors and the reviewers. Any product that may be evaluated in this article, or claim that may be made by its manufacturer, is not guaranteed or endorsed by the publisher.
1. ^For technical reasons, the dataset was not received by the research team until June 2022. The pre-analysis plan AEARCTR-0009202 was uploaded to AEA RCT repository prior to any engagement with the data. The analysis reported in this paper deviates from the pre-analysis plan due to data availability.
2. ^The matching algorithm was developed by TickTalkTo without any involvement of the research team and is not a part of the study. Our outcome variable is measured before the algorithm is employed. In both conditions, the matching suggestion is based on an evaluation of the psychological assessment by an algorithm that is further reviewed by the human mediator taking into account any follow-up communication with the user (if applicable).
3. ^We detect no difference in time that spanned between receiving the prompt and starting the assessment (if the assessment was started at all) by treatment, Kolmogorov-Smirnov test for equality of distributions, p = 0.3.
4. ^We removed 15 observations (about 1% of the sample) from the initial sample of 1,087 observations. These observations had a negative difference between receiving the prompt and starting the assessment. A negative timestamp is likely due to users proceeding to the assessment from an on-screen push notification.
5. ^We have information on some basic demographic characteristics of only 24% of the sample who chose to fill these fields in their user profiles. Due to this very limited coverage, we are not able to use this data systematically in the analysis.
6. ^Seven participants took over 60 h to complete the assessment. The results remain unchanged if these observations are excluded. Table 1 reports the regression coefficients excluding these observations because these outliers strongly affect the magnitudes of coefficient estimates (but do not affect statistical significance).
Ahmad, F., Hogg-Johnson, S., Stewart, D. E., Skinner, H. A., Glazier, R. H., and Levinson, W. (2009). Computer-assisted screening for intimate partner violence and control: a randomized trial. Ann. Intern. Med. 151, 93–102. doi: 10.7326/0003-4819-151-2-200907210-00124
Alonso, J., Liu, Z., Evans-Lacko, S., Sadikova, E., Sampson, N., Chatterji, S., et al. (2018). Treatment gap for anxiety disorders is global: results of the world mental health surveys in 21 countries. Depress. Anxiety 35, 195–208. doi: 10.1002/da.22711
Arkes, H. R., Shaffer, V. A., and Medow, M. A. (2007). Patients derogate physicians who use a computer-assisted diagnostic aid. Med. Decis. Mak. 27, 189–202. doi: 10.1177/0272989X06297391
Ayers, J. W., Poliak, A., Dredze, M., Leas, E. C., Zhu, Z., Kelley, J. B., et al. (2023). Comparing physician and artificial intelligence chatbot responses to patient questions posted to a public social media forum. JAMA Intern. Med. 183, 589–596. doi: 10.1001/jamainternmed.2023.1838
Bharadwaj, P., Pai, M. M., and Suziedelyte, A. (2017). Mental health stigma. Econ. Lett. 159, 57–60. doi: 10.1016/j.econlet.2017.06.028
Bower, M., Smout, S., Donohoe-Bales, A., Teesson, L., Lauria, E., Boyle, J., et al. (2022). A hidden pandemic? An umbrella review of global evidence on mental health in the time of COVID-19. Front. Psychiatry 14:1107560. doi: 10.31234/osf.io/bzpvw
Brown, J. E., and Halpern, J. (2021). Ai chatbots cannot replace human interactions in the pursuit of more inclusive mental healthcare. SSM-Ment. Health 1:100017. doi: 10.1016/j.ssmmh.2021.100017
Bundorf, M. K., Polyakova, M., and Tai-Seale, M. (2019). How Do Humans Interact With Algorithms? Experimental Evidence From Health Insurance (No. w25976). National Bureau of Economic Research.
Castelo, N., Bos, M. W., and Lehmann, D. R. (2019). Task-dependent algorithm aversion. J. Market. Res. 56, 809–825. doi: 10.1177/0022243719851788
Chugunova, M., and Sele, D. (2022). An interdisciplinary review of the experimental evidence on how humans interact with machines. J. Behav. Exp. Econ. 2022:101897. doi: 10.1016/j.socec.2022.101897
Cormier, D., Newman, G., Nakane, M., Young, J. E., and Durocher, S. (2013). “Would you do as a robot commands? An obedience study for human-robot interaction,” in International Conference on Human-Agent Interaction, 13. Available online at: https://hci.cs.umanitoba.ca/assets/publication_files/2013-would-you-do-as-a-robot-commands.pdf
Cowgill, B., Dell'Acqua, F., and Matz, S. (2020). “The managerial effects of algorithmic fairness activism,” in AEA Papers and Proceedings, Vol. 110 (Nashville, TN: American Economic Association), 85–90.
Daniela, S., and Chugunova, M. (2022). “Putting a human in the loop: increasing uptake, but decreasing accuracy of automated decision-making,” in Max Planck Institute for Innovation & Competition Research Paper No. 22-20. Available online at: https://ssrn.com/abstract=4285645
Gautham, M. S., Gururaj, G., Varghese, M., Benegal, V., Rao, G. N., Kokane, A., et al. (2020). The national mental health survey of India, Prevalence, socio-demographic correlates and treatment gap of mental morbidity. Int. J. Soc. Psychiatry 66, 361–372. doi: 10.1177/0020764020907941
Grzymek, V., and Puntschuh, M. (2019). What Europe Knows and Thinks About Algorithms Results of a Representative Survey. Bertelsmann Stiftung.
Hertz, N., and Wiese, E. (2019). Good advice is beyond all price, but what if it comes from a machine? J. Exp. Psychol. Appl. 25, 386–395. doi: 10.1037/xap0000205
Humphreys, J., Tsoh, J. Y., Kohn, M. A., and Gerbert, B. (2011). Increasing discussions of intimate partner violence in prenatal care using video doctor plus provider cueing: a randomized, controlled trial. Women's Health Issues 21, 136–144. doi: 10.1016/j.whi.2010.09.006
Hussein, M. A., and Huang, S. (2020).“Stress, addiction, and artificial intelligence,” in NA - Advances in Consumer Research, Vol. 48, eds J. Argo, T. M. Lowrey, and H. J. Schau (Duluth, MN: Association for Consumer Research), 11551159. Available online at: https://www.acrwebsite.org/volumes/2657172/volumes/v47/NA-48
Ishowo-Oloko, F., Bonnefon, J.-F., Soroye, Z., Crandall, J., Rahwan, I., and Rahwan, T. (2019). Behavioural evidence for a transparency-efficiency tradeoff in human-machine cooperation. Nat. Mach. Intell. 1, 517–521. doi: 10.1038/s42256-019-0113-5
Lattie, E. G., Adkins, E. C., Winquist, N., Stiles-Shields, C., Wafford, Q. E., and Graham, A. K. (2019). Digital mental health interventions for depression, anxiety, and enhancement of psychological well-being among college students: systematic review. J. Med. Intern. Res. 21:e12869. doi: 10.2196/12869
Logg, J. M., Minson, J. A., and Moore, D. A. (2019). Algorithm appreciation: people prefer algorithmic to human judgment. Organ. Behav. Hum. Decis. Process. 151, 90–103. doi: 10.1016/j.obhdp.2018.12.005
Longoni, C., Bonezzi, A., and Morewedge, C. K. (2019). Resistance to medical artificial intelligence. J. Consum. Res. 46, 629–650. doi: 10.1093/jcr/ucz013
Luo, X., Tong, S., Fang, Z., and Qu, Z. (2019). Frontiers: Machines vs. humans: the impact of artificial intelligence chatbot disclosure on customer purchases. Mark. Sci. 38, 937–947. doi: 10.1287/mksc.2019.1192
McCullough, J. S., Parente, S. T., and Town, R. (2016). Health information technology and patient outcomes: the role of information and labor coordination. RAND J. Econ. 47, 207–236. doi: 10.1111/1756-2171.12124
Miller, A. R., and Tucker, C. E. (2011). Can health care information technology save babies? J. Polit. Econ. 119, 289–324. doi: 10.1086/660083
Murgia, A., Janssens, D., Demeyer, S., and Vasilescu, B. (2016). “Among the machines: Human-bot interaction on social q&a websites,” in Proceedings of the 2016 CHI Conference Extended Abstracts on Human Factors in Computing Systems, 1272–1279. doi: 10.1145/2851581.2892311
Naslund, J. A., Aschbrenner, K. A., Araya, R., Marsch, L. A., Unützer, J., Patel, V., et al. (2017). Digital technology for treating and preventing mental disorders in low-income and middle-income countries: a narrative review of the literature. Lancet Psychiatry 4, 486–500. doi: 10.1016/S2215-0366(17)30096-2
Nass, C., Carney, P., and Moon, Y. (1999). Are people polite to computers? Responses to computer-based interviewing systems 1. J. Appl. Soc. Psychol. 29, 1093–1109. doi: 10.1111/j.1559-1816.1999.tb00142.x
Nass, C., and Moon, Y. (2000). Machines and mindlessness: social responses to computers. J. Soc. Issues 56, 81–103. doi: 10.1111/0022-4537.00153
Neary, M., and Schueller, S. M. (2018). State of the field of mental health apps. Cogn. Behav. Pract. 25, 531–537. doi: 10.1016/j.cbpra.2018.01.002
Prince, M., Patel, V., Saxena, S., Maj, M., Maselko, J., Phillips, M. R., et al. (2007). No health without mental health. Lancet 370, 859–877. doi: 10.1016/S0140-6736(07)61238-0
Promberger, M., and Baron, J. (2006). Do patients trust computers? J. Behav. Decis. Mak. 19, 455–468. doi: 10.1002/bdm.542
Rahman, S., Amit, S., and Kafy, A.-A. (2022). Gender disparity in telehealth usage in Bangladesh during COVID-19. SSM-Ment. Health 2:100054. doi: 10.1016/j.ssmmh.2021.100054
Schultz, K., Sandhu, S., and Kealy, D. (2021). Primary care and suicidality among psychiatric outpatients: the patient-doctor relationship matters. Int. J. Psychiatry Med. 56, 166–176. doi: 10.1177/0091217420977994
Shaffer, V. A., Probst, C. A., Merkle, E. C., Arkes, H. R., and Medow, M. A. (2013). Why do patients derogate physicians who use a computer-based diagnostic support system? Med. Decis. Mak. 33, 108–118. doi: 10.1177/0272989X12453501
Shi, W., Wang, X., Oh, Y. J., Zhang, J., Sahay, S., and Yu, Z. (2020). “Effects of persuasive dialogues: testing bot identities and inquiry strategies,” in Proceedings of the 2020 CHI Conference on Human Factors in Computing Systems, 113.
Wang, L. X. (2021). The complementarity of drug monitoring programs and health it for reducing opioid-related mortality and morbidity. Health Econ. 30, 2026–2046. doi: 10.1002/hec.4360
Waytz, A., and Norton, M. I. (2014). Botsourcing and outsourcing: robot, British, Chinese, and German workers are for thinking “not feeling” jobs. Emotion 14:434. doi: 10.1037/a0036054
Keywords: mental health, compliance, digital technology, experimental evidence, India
Citation: Chatterjee C, Chugunova M, Ghosh M, Singhal A and Wang LX (2023) Human mediation leads to higher compliance in digital mental health: field evidence from India. Front. Behav. Econ. 2:1232462. doi: 10.3389/frbhe.2023.1232462
Received: 31 May 2023; Accepted: 07 September 2023;
Published: 28 September 2023.
Edited by:
Francesco Fallucchi, University of Bergamo, ItalyReviewed by:
Tatiana Celadin, Ca' Foscari University of Venice, ItalyCopyright © 2023 Chatterjee, Chugunova, Ghosh, Singhal and Wang. This is an open-access article distributed under the terms of the Creative Commons Attribution License (CC BY). The use, distribution or reproduction in other forums is permitted, provided the original author(s) and the copyright owner(s) are credited and that the original publication in this journal is cited, in accordance with accepted academic practice. No use, distribution or reproduction is permitted which does not comply with these terms.
*Correspondence: Marina Chugunova, bWFyaW5hLmNodWd1bm92YUBpcC5tcGcuZGU=
†These authors have contributed equally to this work
Disclaimer: All claims expressed in this article are solely those of the authors and do not necessarily represent those of their affiliated organizations, or those of the publisher, the editors and the reviewers. Any product that may be evaluated in this article or claim that may be made by its manufacturer is not guaranteed or endorsed by the publisher.
Research integrity at Frontiers
Learn more about the work of our research integrity team to safeguard the quality of each article we publish.