- Department of Agricultural Economics, University of Nebraska-Lincoln, Lincoln, NE, United States
Introduction: Understanding drivers of individuals' food choices is critical to be able to address the public health epidemic of obesity-related health problems. A robust literature documents relationships between intertemporal preferences and body mass index (BMI). However, work on cognition differentiates resource investment in decision-making, with processes frequently categorized as habitual (low investment) vs. model-based (high investment).
Methods: We integrate variables related to cognition with intertemporal preferences to examine how discount rates and active consideration of health impacts of foods faced during choice relate to the nutritional quality of foods selected.
Results: Our results show that participants who actively consider health outcomes choose products with significantly higher nutritional quality compared to those who do not consider the health impacts of choices, even while controlling for discount rates. We also find that participants with lower discount rates selected foods with higher nutritional quality compared to high discount-rate participants, corroborating previous literature. Further, we find that participants who actively considered health during the choice process spent significantly more time making choices, while discount rates did not predict differences in decision times, suggesting a role for habitual vs. model-based decisions.
Discussion: These findings suggest that active consideration of the health consequences during food choice is an important predictor of the nutritional quality of choices even while accounting for intertemporal preferences, which may provide new opportunities to intervene in the decision-making process.
1. Introduction
Low-quality diet is a leading cause of overweight and obesity and an important contributor to recent decreases in life expectancy in the U.S. and worldwide (Murray, 2013; Preston et al., 2018; Mehta et al., 2020; Popkin and Ng, 2022). US consumers face many highly processed, energy-dense foods (Popkin, 2006; Farley et al., 2010), resulting in consumption of more calories, and subsequent weight gain (Hall et al., 2019). The most common policy response in the US to address the obesity epidemic has focused on providing nutrition information to consumers so that they can identify healthy foods, enabling improvements in dietary quality. Both packaged food products and restaurants and other retail outlets selling prepared foods that have 20 or more locations are required to carry nutrition information. However, there is little evidence that these information-focused policies have led to improved diet quality (Variyam, 2008; Elbel et al., 2009; Bleich et al., 2017; Cantu-Jungles et al., 2017).
Despite the well-established links between diet and health, many people continue to consume low-quality diets, which may reflect the fact that a low-quality diet largely imposes costs in the future. Since people discount future experiences, future costs are likely to carry less weight than the immediate pleasure of consuming preferred foods (Ericson and Laibson, 2019). However, there is also inter-individual variation in discount rates. The discount rate has been used to predict behaviors that have implications for people's long-term health and wellbeing, such as saving money for the future or health decisions like food choices and exercise (Chabris et al., 2008; Rung et al., 2018). Empirical research shows that individuals who discount the future heavily are more likely to be obese than those who discount the future little (Zhang and Rashad, 2008; Garza et al., 2013; Courtemanche et al., 2015; Dassen et al., 2015; de Oliveira et al., 2016). People who heavily discount the future are less likely to use nutrition label information and more likely to consume lower quality foods, more fast food, high sugar foods, and engage in overnight eating (Shuval et al., 2016; Bickel et al., 2021). A study of women with overweight and obesity found that those who discounted the future highly had greater energy intake when eating away-from-home and ready-to-eat foods (Appelhans et al., 2012). A systematic review of the literature shows that individuals with higher discount rates are more likely to be overweight/obese and consume unhealthy diets (Barlow et al., 2016). Models of intertemporal preferences assume that people weigh the discounted values of options occurring at different points in time. Increasing evidence, however, shows that limited attention is common in decision-making and extends to consideration of the consequences of choices. Read et al. (2017) identify asymmetric attention to opportunity costs across time, showing that people pay less attention to future opportunity costs of choices than to immediate opportunity costs. In the domain of food choices, this may mean that future health implications of alternative foods facing the individual are more likely to be overlooked than immediate opportunity costs, such as the foregone satisfaction of not eating a favored food now.
In this paper, we study the nutritional quality of food choices—and elements of the choice process including time spent making decisions, the set of products considered, and the use of nutrition information during choice—using a variable intentionally structured to differentiate between those considering health consequences of food options faced and those who do not. We examine the relationship of active consideration of health impacts and intertemporal preferences to the nutritional quality of foods chosen in a hypothetical food choice task, as well as to choice process variables that elucidate differences in behavior during the choice process. We report multiple analyses for each model, identifying the relationship of active consideration of health impacts to choice process variables and to choice outcomes; we then incorporate intertemporal preferences elicited via a standard intertemporal financial choice task to examine the robustness of the estimates of the active consideration of health impacts. Finally, we include standard demographic control variables as an additional robustness check. We are aware of only one paper that seeks to integrate intertemporal preferences with future consideration. Bartels and Urminsky (2015) investigated how awareness and discounting of the future interact to influence choices with intertemporal consequences. In a series of experiments, the authors examined the relationship that a scale-based measure of the tendency to consider future consequences (CFC), has on participants' spending habits, while also studying the influence of discount rates on spending. The findings show that the combination of awareness of future outcomes and a higher relative valuation of future outcomes (that is, less discounting of future outcomes relative to current outcomes) reduces spending. An important distinction between the approach used by Bartels and Urminsky (2015) and our work is that we use a choice instance-specific measure of future consideration rather than a scale-based measure. The scales used to capture consideration of future consequences were designed to measure a stable individual trait (Strathman et al., 1994; Toepoel, 2010). However, attention to opportunity costs of choices can be influenced by environmental factors including the presentation of information (Read et al., 2017), which creates opportunities to design interventions that target attention.
Our analyses test hypotheses about the nutritional quality of foods chosen, as well as relationships with choice process variables. We hypothesize that both actively considering health outcomes and having a lower discount rate lead to more nutritious food choices. We additionally hypothesize that actively considering health outcomes and having a lower discount rate increase the likelihood that individuals consider healthier sets of products. Based on models of cognition that differentiate between habitual (i.e., fast/simple) and model-based decision making, we hypothesize that individuals who actively consider the health impacts of the foods in the choice environment will spend longer on the choice task than individuals who do not actively consider health. However, since previous literature indicates that discount rates are related to habitual differences in choice—leading to differences in BMI status, for instance, we hypothesize that there will be no significant difference in decision time for discount rates. Similarly, we expect that active consideration of health will be related to significantly more use of nutrition information, while we hypothesize that there will not be a significant difference for discount rates. If active consideration of health—beyond stable, inter-temporal preferences—is an important contributor to the nutritional quality of food choices, it may open new pathways for promoting healthier choices, such as reminders or prompts that draw individuals' attention to health.
2. Methods
2.1. Data collection
We conducted an online survey with 502 US adults (≥19 years old) in August 2021. The survey was developed in Qualtrics (www.qualtrics.com) and distributed via Prolific (www.prolific.co), an online survey recruitment platform. A link to our Qualtrics survey was posted on the Prolific website so that registered Prolific participants could view the opportunity to complete the survey. Respondents had to be 19 years of age or older and a resident of the United States to complete the survey. We estimated that the survey would take ~11 min to complete, and respondents were offered $1.85 for a completed survey, which would correspond to an hourly rate of over $10/h. The average completion time was slightly over nine-and-a-half minutes.
The survey consisted of a hypothetical food choice task, questions about whether individuals used nutrition information that was available in the choice environment, as well as questions about broader issues that the respondents had considered during food choice, an intertemporal choice task, and standard demographic questions. Participation criteria were that individuals had to be at least 19 years of age and residents of the US. The study was approved by the University of Nebraska-Lincoln Institutional Review Board (protocol #20201020721EX). All participants provided informed consent before participating in the experiment and were given the option to terminate their participation at any time during the study.
The food choice task incorporated elements of real-world grocery shopping experiences, including large assortments of products and the opportunity for participants to direct their attention. That is, participants could choose to view all available products or to direct their attention to a subset of products when making their product choices; the set of products they considered was recorded as choice process data.
At the beginning of the survey, participants were exposed to a cheap talk script directing them to imagine they were making real choices that would result in spending real money. The use of cheap talk scripts has been shown to reduce hypothetical bias in a meta-analysis of techniques to diminish hypothetical bias (Penn and Hu, 2018).
In the experiment, participants viewed cereal, bread, and cracker product categories sequentially. In each product category, there were 33 products to choose from. As in real-world physical and online retail settings, participants could direct their attention to subsets of products. In this experiment, participants could choose to view subsets of the products or all the available products in each category. The products constituted a range of less healthy to healthy options and the subsets used this structure to group products into three sets of 11 items. While not displayed to participants, we use the Guiding Stars (GS) nutritional rating system (Guiding Stars, 2022), which rates products based on nutrient content from 0 (low nutritional quality) to 3 (high nutritional quality) stars, as a measure of overall nutritional quality of the choices participants made. The GS system calculates a product's score based on food attributes such as vitamins, minerals, fiber, whole grains, omega-3 fatty acids, saturated fat, trans fat, added sodium, added sugars, and artificial colors present in a product (Guiding Stars, 2022). The GS system was also used to determine the cutoff points for assigning products to the subsets. One subset had products that received 0 GS, a second had 1 GS, and the third had products with 2 or 3 GS. After choosing the set of products they wanted to view in a product category, the participant then made a product selection. Participants could also indicate that they would not choose any of the available products. The participants' choices of product sets to view in a product category constitute their consideration set, which is recorded as a choice process variable in our dataset.
The cereal, cracker, and bread products in the experiment were non-store brands that are widely available in supermarkets across the US. All the products had a selection of per-serving nutrition information that is provided on nutrition facts panels displayed below them. For a screenshot of the experimental interface, see Figure 3 in Arslain et al. (2020).
After making selections in each product category, participants answered a series of questions about choice considerations, intertemporal choice questions, and demographic questions. We take a different approach than (Bartels and Urminsky, 2015) use of the CFC to document consideration of the future because the CFC does not distinguish between habitual processes and acute, goal-oriented decision-making. Rather than use a series of questions about tendencies to consider the future, we retrospectively asked about factors that participants actively considered during the choice process after participants had completed the food choice task. Questions were asked retrospectively rather than during the choice process to avoid priming or influencing choices (Morris et al., 2021). We provided multiple check-all-that-apply response options, including the health impacts of the foods, taste preferences, trust in different brands, the impact of prices on the respondent's budget, and others.
We asked directly about factors considered because evidence shows that cognitive processes can be influenced by external forces. For instance, hunger has been found to influence food choices for both immediate and future consumption (Lozano et al., 1999), suggesting that the state of hunger affected individuals' decision processes. Further, consideration of the opportunity costs of immediate rewards can be prompted by a simple change in the presentation of the choice, making participants more likely to choose larger, delayed rewards (Read et al., 2017). The active consideration question was, “In general, which of the following did you consider when making food choices today?” A variety of options were presented, including taste and price, among others, but the considerations of interest were: “The impact the foods might have on your/your family's health in the future” and “The impact of the foods on your/your family's current health.” We chose to elicit information about what participants considered during the choice process directly after they had made all of their food choices to avoid influencing the food choice process (Morris et al., 2021). We then created a binary active consideration of health variable that captured whether a participant considered current and/or future health impacts (=1), or did not consider health impacts during choice (=0).
To create a measure of how individuals traded off immediate vs. future benefits, participants answered four intertemporal choice questions. Participants were asked to imagine choosing to receive an immediate payment of $1,000 or 1,200 in 1 month. If they chose the immediate payment of $1,000, they were then asked to choose between a payment of $1,000 today or $1,300 in a month. If they again chose $1,000 immediately, they next chose between $1,000 immediately or $1,400 in 1 month. If they still chose $1,000 immediately, the final intertemporal choice question asked them to indicate the amount they would need to receive to wait for a month rather than receive a payment of $1,000 today. In those choices, they had an option of choosing “I don't know” (data from individuals who indicated that they did not know where omitted from all analyses below). For the discount rate responses, we categorized participants based on their relative degree of patience. Participants who chose $1,200 in 1 month were classified as “low discount rate,” $1,300 as “medium-low discount rate,” $1,400 as “medium-high discount rate,” and those who required an amount >$1,400 to wait a month for a larger amount as “high discount rate.”
2.2. Data analysis
We conducted the analyses using R Studio (R Core Team, 2021). We created a panel dataset of the choices that every participant made in each of the three food categories. We analyze one choice outcome variable and three choice process variables. The choice outcome variable is the GS rating of the products that individuals chose. The three choice process variables are (1) the amount of time that the individual spent making each food choice, (2) the set of products that the individual chose to view during choice, and (3) the number of pieces of nutrition information used by each participant during food choice.
We use the GS rating as the dependent variable in a linear regression. The independent variables included were (1) active health consideration (with no health consideration constituting the omitted category), and (2) the discount level implied by the intertemporal choice questions (with “high discount rate” being the reference category). We examine the impact of the active consideration variables on GS alone and together with the discount variables and/or demographic variables (sex, age, income, and education), resulting in four sets of regressions.
Next, we analyze the relationship of active consideration of health and discount rates to the choice process variables. The first analysis is a linear regression of the amount of time spent on decisions on active consideration of health and discount rates. Next, we conduct a linear regression of the pieces of nutrition information used in each food category during the choice process on active consideration of health and discount rates. Finally, we analyze the relationship of the nutrition-ranked sets of products that participants viewed during the choice process with active consideration of health and discount rates. For each analysis of choice process variables, we conduct robustness checks by adding demographic characteristics to the models as control variables. All linear regression models use cluster-robust standard errors. We consider p-values <0.05 to be statistically significant.
3. Results
Table 1 summarizes the demographic characteristics of participants. The average household income of participants was just over 74,000 dollars. Over 70% of participants identified as female and about 56% had completed bachelor's degrees. The average age of participants was 29 years old. Around 46% of participants actively considered current, future, or both current and future health outcomes and 77% exhibited patient behavior during the intertemporal monetary preferences task.
3.1. Analysis of nutritional quality of food choices
Next, we report the results of our main analyses of the impact of active consideration of health and discounting on the GS rating of products chosen in the choice task. Table 2 presents the results of four regression models examining the influence of consideration of current and future health outcomes and discounting behaviors on the nutritional quality of food choices.
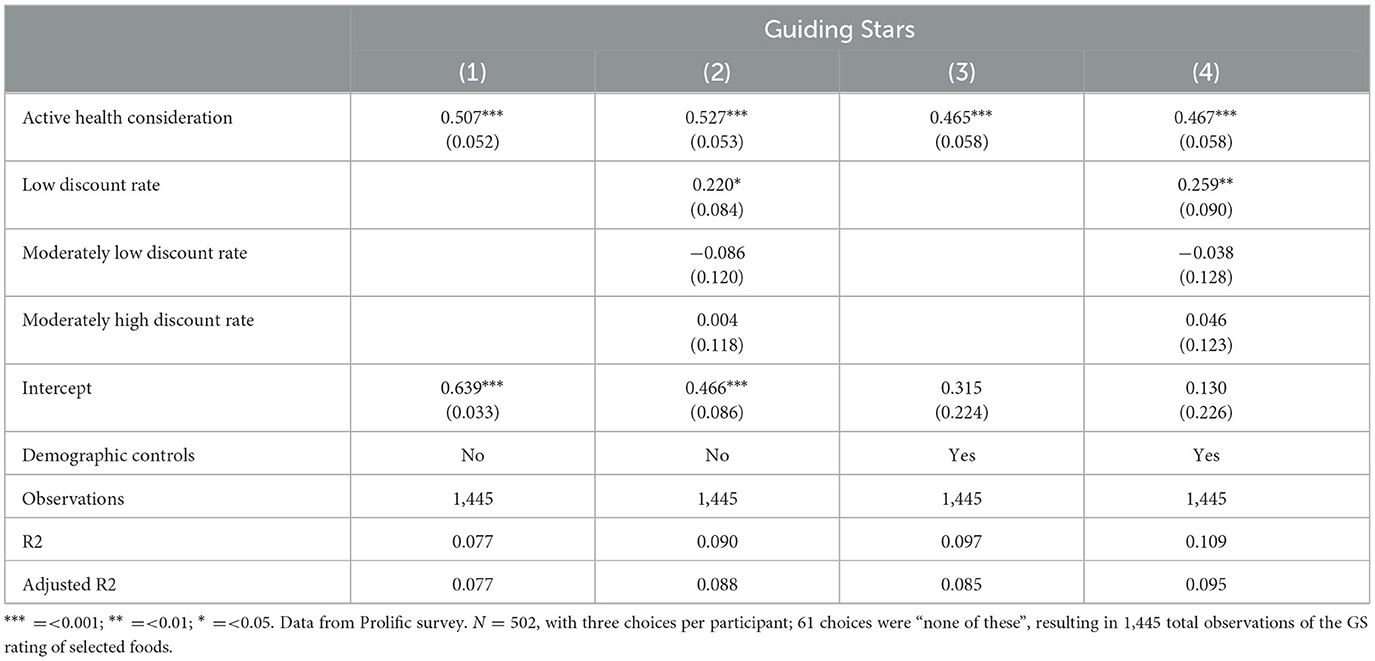
Table 2. Linear regression model of the impact of active consideration of health and intertemporal preferences on Guiding Stars (GS) with individual-clustered standard errors.
The regression reported in column 1 only includes active consideration of health. Active consideration of health is statistically significant. Participants who actively considered health outcomes selected products with ~0.5 more GS per product than those who did not consider health outcomes. That is, participants who considered health impacts chose products with one more GS than those who did not consider health impacts roughly every second choice. Supplementary Table 1 presents the distribution of products and choices across Guiding Stars ratings.
The analysis reported in column (2) incorporates both active health consideration and discounting. Individuals who actively considered health during choice and those with low discount rates chose foods with significantly more GS. Individuals who actively considered health chose over 0.5 more GS per choice and individuals with low discount rates chose around 0.2 additional GS per product than high-discount individuals.
Estimates of consideration of health outcomes and discount rates change little and remain significant when we control for demographic characteristics (columns 3 and 4). When controlling for demographic characteristics, active health consideration is statistically significant with an estimated coefficient of around 0.46 (with or without the inclusion of discount variables). Additionally, individuals with low discount rates chose products with significantly more GS (0.259). It is noteworthy that the estimated effect of active consideration of health impacts does not change importantly with the addition of variables capturing intertemporal preferences.
The estimates support our hypotheses. Participants who actively considered health—a future opportunity cost of the choice of some foods—made significantly healthier choices than those who did not actively consider health. The estimated relationship between active consideration of health and the GS rating of foods chosen was highly stable across analyses, with the highest and lowest estimated coefficients differing by only 0.06 GS. Intertemporal preferences are also significantly related to the nutritional quality of choices, with respondents with the lowest discount rates making choices with significantly higher GS than those with the highest discount rates.
3.2. Analysis of choice process variables
Next, we report the results of analyses of choice process variables. Supplementary Figure 2 displays a histogram of decision time for food choices in the experiment. First, we present the results of the analysis of decision time (Table 3). Active consideration of health during choice is significantly positively related to the amount of time spent on the food choice. Coefficient estimates of the additional amount of time spent by participants who actively considered health during food choice range from over 6 s to nearly ten additional seconds. None of the discounting variables are significantly related to the amount of time spent on choices. We report alternative specifications with the log of decision time as the dependent variable and robustness checks in Supplementary Models 1–4. The findings support our hypothesis that active consideration of health would correspond to longer decision times, but that discount rate variables would not significantly relate to decision times.
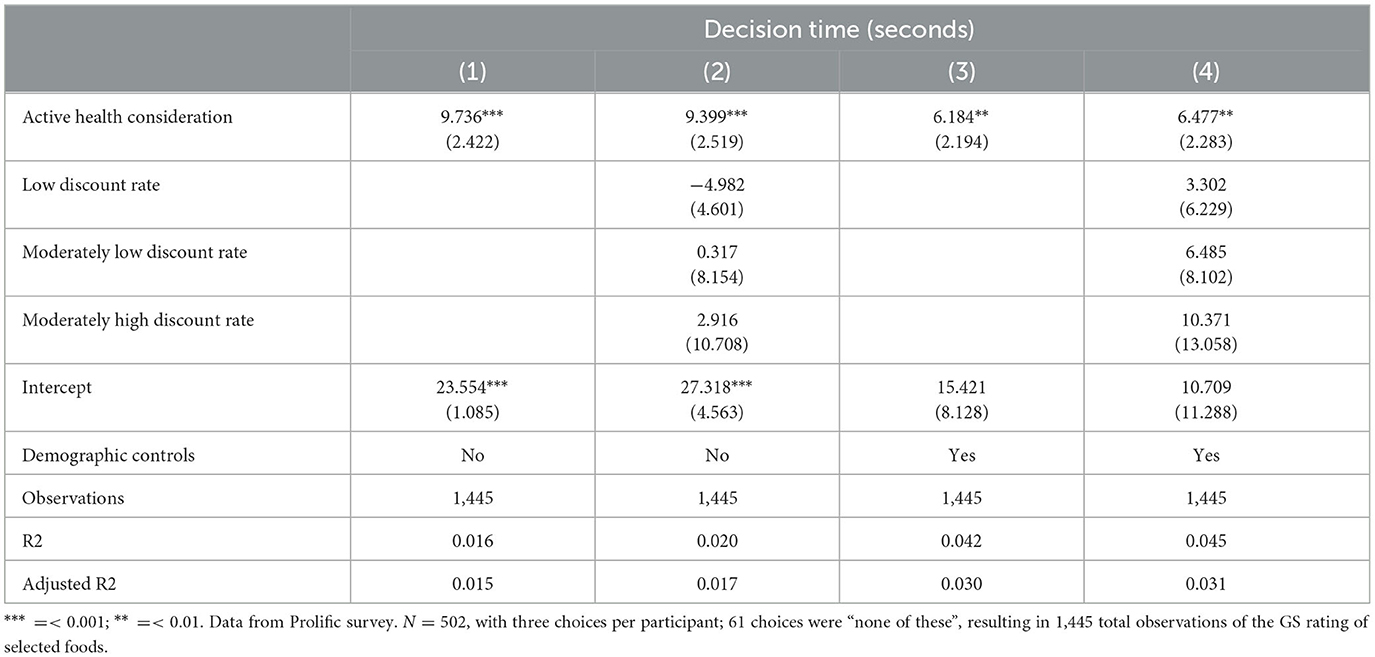
Table 3. Linear regression model of the relationship of active consideration of health and intertemporal preferences on the amount of time (in seconds) spent making food decisions with individual-clustered standard errors.
The next choice process variable we examine is the number of pieces of nutrition information used by participants during the choice process (Table 4). Supplementary Figure 1 presents a histogram of nutrition information use per product category. We find that participants who actively considered health during the choice process used significantly more pieces of nutrition information than participants who did not consider health during choice, with estimates ranging from 1.1 to 1.35 additional pieces of nutrition information considered across models. This result corresponds to our hypothesis. Discount rates are also related to the use of nutrition information, though the significance of this relationship is largely eliminated with the inclusion of demographic control variables.
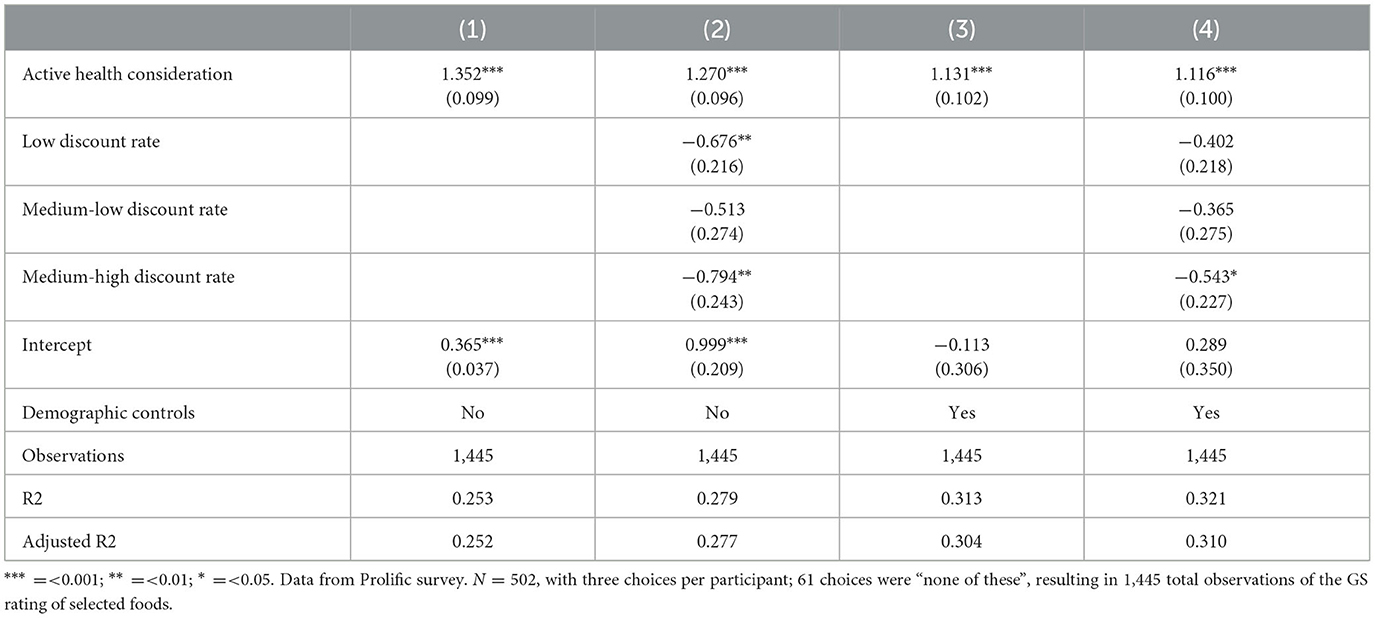
Table 4. Linear regression model of the relationship of active consideration of health and intertemporal preferences on the use of nutrition information during food choice with individual-clustered standard errors.
Finally, we estimate the relationship between the ranked set of products considered and active consideration of health and discounting variables (Table 5). We find that active consideration of health significantly increases the nutritional ranking (where a higher ranking represents higher average nutritional quality) for each product type. Discount rates did not explain differences in the nutritional ranking of product sets that participants viewed during the choice process. The estimated results for active consideration of health are robust to the inclusion of demographic characteristics. An alternative analysis, using multinomial logistic regression to examine the specific (rather than ranked) product sets viewed is reported in an Supplementary Table 2.
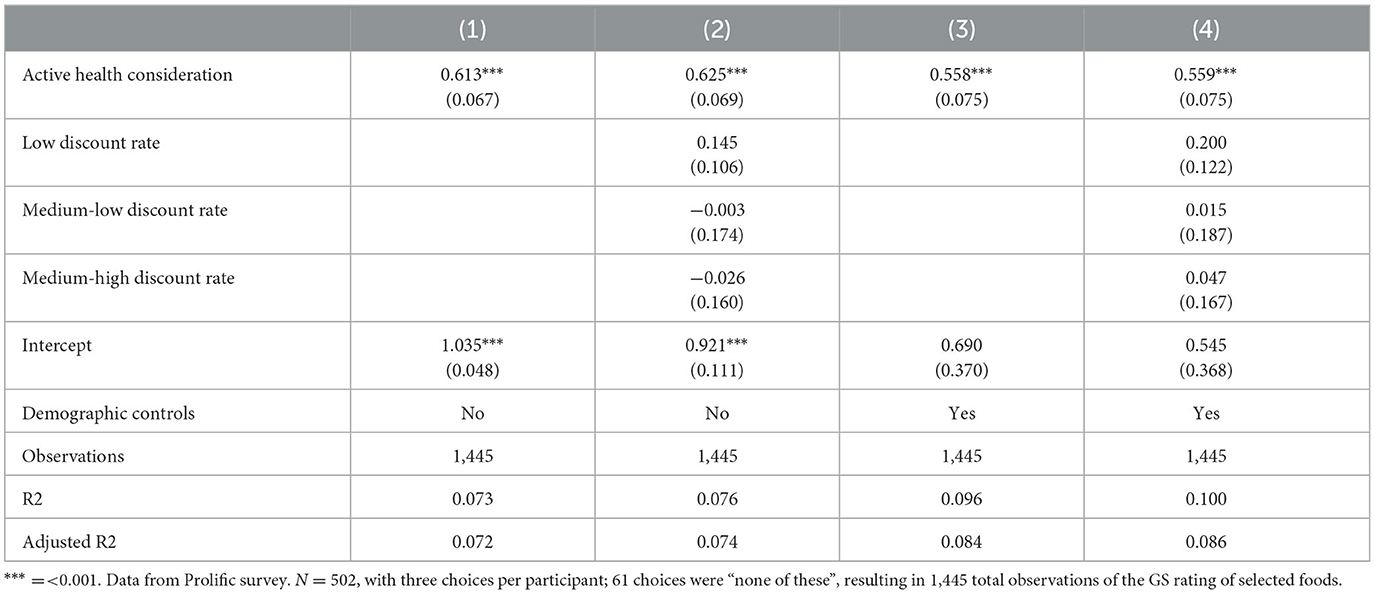
Table 5. Linear regression model of the relationship of active consideration of health and intertemporal preferences with the ranked average nutritional content of product sets considered with individual-clustered standard errors.
4. Discussion
The results of this study show that people who actively consider health implications during food choice select more nutritious foods, spend more time on decisions, use more nutrition information, and consider healthier sets of products during choice compared to those who do not, even while controlling for intertemporal preferences and demographic characteristics. These findings suggest that intertemporal preferences alone do not fully capture the moment-to-moment variation in attention to the broader, largely future, impacts of choices. In fact, not only is active consideration of health significant in explaining the nutritional quality of choices but our results also show that actively considering health outcomes explains more variation in the nutritional quality of chosen products than the discount rate derived from intertemporal choice tasks. Additionally, the stability of the estimated effect of considering health impacts on the nutritional quality of foods chosen across models that include and exclude intertemporal preference variables suggests that intertemporal preferences and active consideration of health impacts may capture different elements of the choice process.
Despite the implementation of multiple information-based policies meant to promote more nutritious food choices over the past 30 years in the US—such as the nutrition facts panel and restaurant calorie labeling, evidence suggests that these policies have had little impact on food choices on average. The availability of nutrition information on food packages and calorie labeling in restaurants has not markedly changed the average nutritional quality of foods chosen (Variyam, 2008; Elbel et al., 2009; Bleich et al., 2017), though there are individual differences in the use of nutrition information (Elbel et al., 2009; Grunert et al., 2010; Christoph et al., 2018). Both lower discount rates and active consideration of health impacts during choice have been found to correlate with higher use of nutrition information during choice in studies examining each variable in isolation (Bickel et al., 2021; Gustafson, 2022).
Since our research was cross sectional, our results do not directly identify pathways to improve food choice behaviors. While research has found that discount rates can be altered, resulting in more patient choices, lasting changes typically result from intensive educational interventions (Rung et al., 2018). The impact of episodic future thinking, one form of intervention that asks people to imagine detailed future experiences, has been shown to make people more patient in intertemporal choice tasks, for instance (Peters and Büchel, 2010). Daniel et al. (2013a) found that episodic future thinking helped reduce how much people discount their future. They analyzed decisions by 26 individuals with overweight or obesity using episodic thinking to induce consideration of future events during a delay-discounting task and an ad libitum eating task. Episodic future thinking led participants to reduce their discounting and to choose healthier foods during the experiment compared to the control group. In a second study with individuals of varying weight status, episodic future thinking reduced impulsive eating patterns, resulting in decreased calorie consumption (Daniel et al., 2013b). However, episodic future thinking may be difficult to exogenously induce in real-world decision settings.
A potential implication of our results concerns interventions that target attention to health consequences of food choices. The most consistent differences in health-related behaviors and choice outcomes in our study were captured by attention to the health impacts of food choices. Attention can be drawn to elements of a choice via simple methods, such as primes or prompts (Papies et al., 2014), which may lead to goal activation and adherence to healthier behaviors (Marteau et al., 2012). Reminder emails have been shown to increase gym attendance, with effects extending beyond the end of the email intervention (Calzolari and Nardotto, 2017; Habla and Muller, 2021). Research in brick-and-mortar supermarkets suggests that the use of primes or simple reminder/prompt messages can promote healthier food choices in real-world settings (Papies et al., 2014; Gustafson et al., 2018). Recent work using a computer interface to document choice process variables shows that the impact of these methods acts through multiple channels: shifting attention to healthier products, increasing the use of nutrition information (Arslain et al., 2020, 2021), and—perhaps most fundamentally—increasing consideration of differences in health-related opportunity costs of foods (Gustafson, 2022). Our results—using data on choice scenario-specific consideration—and those of Gustafson (2022) suggest a promising approach that permits intervening in food choices by eliciting instantaneous health consideration.
Literature on attention-targeting interventions suggests a role for scenario-specific influences on choice. In an fMRI study, Hare et al. (2011) found that simple cues directing attention toward health during food choice changed neural activation patterns—suggesting choice scenario-specific variation in the processing of food attributes—and led to healthier decisions. Research in physical and online retail settings on prime or prompt messages delivered at the point of decision find that these interventions promote healthier choices (Papies et al., 2014; Gustafson et al., 2018; Arslain et al., 2020). Evidence suggests that these point-of-decision interventions change the sets of products that people consider and increase the likelihood that they use nutrition information during choice (Arslain et al., 2021). These studies do not directly examine whether prompts or primes increase attention to health impacts of food choices, though the increased use of nutrition information suggests it likely does (Gustafson and Rose, 2022). In a recent paper, Gustafson (2022) finds that active consideration of health impacts leads people to choose significantly healthier foods. Further, a simple health message increased the likelihood that participants considered long-term health impacts of foods, suggesting that attention can be recruited to targeted elements of a choice attributes to promote healthy decisions.
Our study has some limitations, which we plan to address in future research. First, choices in the study were hypothetical—participants did not receive what they chose and did not spend money. To mitigate concerns about hypothetical decisions, we used a cheap-talk script. The cheap-talk script has been found to minimize hypothetical bias in a meta-analysis of techniques to address hypothetical biases in consumer studies (Penn and Hu, 2018).
A second concern is that we directly asked participants whether they had actively considered various factors during the choice process—with consideration of current and future health as the elements of interest—potentially making a main independent variable subject to social desirability biases (Grimm, 2010). If perceptions of social desirability influenced responses to the question about active consideration of health, it would attenuate the estimate of the true impact of active consideration because the food choices were completed before the question was asked and so would not have been influenced. We chose to ask participants directly because while prior research has used future orientation scales to measure tendencies to consider the future, these scales are intended to be a stable measure of an individual trait. Given our desire to examine scenario-specific consideration, we chose to use retrospective reporting of consideration, which has been used in other research in which investigators needed data about consideration during the specific choice process yet also needed to avoid influencing subsequent choices (Morris et al., 2021; Gustafson and Rose, 2022). While we envisioned our approach as measuring choice-scenario specific consideration, we do not directly compare our approach with the measure we would have obtained with the CFC, preventing a comparison of the two approaches. This will be addressed in future research.
Next, as noted above, though policies intended to prompt or prime consumers to actively consider future outcomes may help consumers consider the health implications of their food choices, this study uses cross-sectional data and so does not provide evidence of the impact of recruiting attention to health. It may be that there are true drivers of healthy choices that we do not measure in our data that are correlated with active consideration of health. For instance, individuals who are more knowledgeable about health benefits of nutrients are more likely to consider those nutrients during food choice (Gustafson and Rose, 2022), making consideration of health impacts a byproduct of their nutrition knowledge. However, Gustafson (2022) found that a health education prompt increased the likelihood that participants considered future health and causally led them to make healthier choices, though that study did not measure time preferences.
Finally, the sample of participants is not representative of the general population in some important ways. Participants were younger and more highly educated on average than the U.S. population. Education, in particular, has been found to relate to multiple important health behaviors and outcomes (Din-Dzietham et al., 2000; Berrigan et al., 2003), potentially making our sample markedly more likely to consider health than a representative sample. While 56% of our participants had a college or advanced degree, only 17% had a high school degree or less. Similar to our results on dietary quality and education, large-scale analyses have found important linkages between education level and dietary quality (Popkin and Ng, 2022). Expanding the characteristics of participants to be more representative will be important given relationships between health and socio-demographic risk factors for diet-related diseases.
Our findings suggest that active consideration of health outcomes is an important pathway for choosing higher-quality diets and may provide an opportunity for targeted interventions to promote healthier consumption, even when controlling for individuals' intertemporal preferences. This result shows that there are two important elements to decisions that promote long-term wellbeing. First, individuals must value the future. Second—and more impactful in predicting differences in behavior in our results—individuals who actively considered the health implications that their choices will have in the future make healthier choices on average. Given that both intertemporal preferences and attention appear to be important determinants of the quality of choices, future work could investigate interventions that target both elements, potentially pairing a more intense, longer-term intervention incorporating methods, such as episodic future thinking, with point-of-decision interventions that recruit attention to health during the decision-process.
Data availability statement
The raw data supporting the conclusions of this article will be made available by the authors, without undue reservation.
Ethics statement
The studies involving human participants were reviewed and approved by University of Nebraska-Lincoln Institutional Review Board. The patients/participants provided their written informed consent to participate in this study.
Author contributions
CG designed the study and obtained funding, implemented the study, and edited and revised the article. OT and CG analyzed the data and drafted the article. Both authors approved the final version of the article.
Funding
This project is based on research that was supported by the University of Nebraska-Lincoln Agricultural Research Division Wheat Innovation Fund.
Conflict of interest
The authors declare that the research was conducted in the absence of any commercial or financial relationships that could be construed as a potential conflict of interest.
Publisher's note
All claims expressed in this article are solely those of the authors and do not necessarily represent those of their affiliated organizations, or those of the publisher, the editors and the reviewers. Any product that may be evaluated in this article, or claim that may be made by its manufacturer, is not guaranteed or endorsed by the publisher.
Supplementary material
The Supplementary Material for this article can be found online at: https://www.frontiersin.org/articles/10.3389/frbhe.2023.1219281/full#supplementary-material
Supplementary Figure 1. Histogram of number of pieces of nutrition information used per product category in the choice process.
Supplementary Figure 2. Histogram of decision time for food choices in the experiment.
References
Appelhans, B. M., Waring, M. E., Schneider, K. L., Pagoto, S. L., DeBiasse, M. A., Whited, M. C., et al. (2012). Delay discounting and intake of ready-to-eat and away-from-home foods in overweight and obese women. Appetite 59, 576–584. doi: 10.1016/j.appet.2012.07.009
Arslain, K., Gustafson, C. R., and Rose, D. J. (2020). Point-of-decision prompts increase dietary fiber content of consumers' food choices in an online grocery shopping simulation. Nutrients 12, 3487. doi: 10.3390/nu12113487
Arslain, K., Gustafson, C. R., and Rose, D. J. (2021). The effect of health prompts on product consideration, attention to information, and choice in large, online product assortments: the case of fiber. Food Qual. Prefer. 94, 104329. doi: 10.1016/j.foodqual.2021.104329
Barlow, P., Reeves, A., McKee, M., Galea, G., and Stuckler, D. (2016). Unhealthy diets, obesity and time discounting: a systematic literature review and network analysis. Obes. Rev. 17, 810–819. doi: 10.1111/obr.12431
Bartels, D. M., and Urminsky, O. (2015). To know and to care: how awareness and valuation of the future jointly shape consumer spending. J. Consum. Res. 41, 1469–1485. doi: 10.1086/680670
Berrigan, D., Dodd, K., Troiano, R. P., Krebs-Smith, S. M., and Barbash, R. B. (2003). Patterns of health behavior in US adults. Prev. Med. 36, 615–623.
Bickel, W. K., Freitas-Lemos, R., Tomlinson, D. C., Craft, W. H., Keith, D. R., Athamneh, L. N., et al. (2021). Temporal discounting as a candidate behavioral marker of obesity. Neurosci. Biobehav. Rev. 129, 307–329. doi: 10.1016/j.neubiorev.2021.07.035
Bleich, S. N., Economos, C. D., Spiker, M. L., Vercammen, K. A., VanEpps, E. M., Block, J. P., et al. (2017). A systematic review of calorie labeling and modified calorie labeling interventions: impact on consumer and restaurant behavior. Obesity 25, 2018–2044. doi: 10.1002/oby.21940
Calzolari, G., and Nardotto, M. (2017). Effective reminders. Manage. Sci. 63, 2915–2932. doi: 10.1287/mnsc.2016.2499
Cantu-Jungles, T. M., McCormack, L. A., Slaven, J. E., Slebodnik, M., and Eicher-Miller, H. A. (2017). A meta-analysis to determine the impact of restaurant menu labeling on calories and nutrients (ordered or consumed) in U.S. Adults. Nutrients 9. doi: 10.3390/nu9101088
Chabris, C. F., Laibson, D., Morris, C. L., Schuldt, J. P., and Taubinsky, D. (2008). Individual laboratory-measured discount rates predict field behavior. J. Risk Uncertain. 37, 237–269. doi: 10.1007/s11166-008-9053-x
Christoph, M. J., Larson, N., Laska, M. N., and Neumark-Sztainer, D. (2018). Nutrition facts panels: who uses them, what do they use, and how does use relate to dietary intake? J. Acad. Nutr. Diet. 118, 217–228. doi: 10.1016/j.jand.2017.10.014
Courtemanche, C., Heutel, G., and McAlvanah, P. (2015). Impatience, incentives and obesity. Econ. J. 125, 1–31. doi: 10.1111/ecoj.12124
Daniel, T. O., Stanton, C. M., and Epstein, L. H. (2013a). The future is now: reducing impulsivity and energy intake using episodic future thinking. Psychol. Sci. 24, 2339–2342. doi: 10.1177/0956797613488780
Daniel, T. O., Stanton, C. M., and Epstein, L. H. (2013b). The future is now: comparing the effect of episodic future thinking on impulsivity in lean and obese individuals. Appetite 71, 120–125. doi: 10.1016/j.appet.2013.07.010
Dassen, F. C. M., Houben, K., and Jansen, A. (2015). Time orientation and eating behavior: unhealthy eaters consider immediate consequences, while healthy eaters focus on future health. Appetite 91, 13–19. doi: 10.1016/j.appet.2015.03.020
de Oliveira, A. C. M., Leonard, T. C. M., Shuval, K., Skinner, C. S., Eckel, C., and Murdoch, J. C. (2016). Economic preferences and obesity among a low-income African American community. J. Econ. Behav. Org. 131, 196–208. doi: 10.1016/j.jebo.2015.11.002
Din-Dzietham, R., Liao, D., Diez-Roux, A., Nieto, F. J., Paton, C., Howard, G., et al. (2000). Association of educational achievement with pulsatile arterial diameter change of the common crotid artery the Atherosclerosis Risk in Communities (ARIC) study, 1987–1992. Am. J. Epidemiol. 152, 617–627.
Elbel, B., Kersh, R., Brescoll, V. L., and Dixon, L. B. (2009). Calorie labeling and food choices: a first look at the effects on low-income people in New York City: calorie information on menus appears to increase awareness of calorie content, but not necessarily the number of calories people purchase. Health Aff. 28, w1110–w1121. doi: 10.1377/hlthaff.28.6.w1110
Ericson, K. M., and Laibson, D. (2019). Intertemporal choice. Handb. Behav. Econ. 2, 1–67. doi: 10.1016/bs.hesbe.2018.12.001
Farley, T. A., Baker, E. T., Futrell, L., and Rice, J. C. (2010). The ubiquity of energy-dense snack foods: a national multicity study. Am. J. Public Health 100, 306–311. doi: 10.2105/AJPH.2009.178681
Garza, K. B., Harris, C. V., and Bolding, M. S. (2013). Examination of value of the future and health beliefs to explain dietary and physical activity behaviors. Res. Soc. Administ. Pharm. 9, 851–862. doi: 10.1016/j.sapharm.2012.12.001
Grimm, P. (2010). Social Desirability Bias. Available online at: https://onlinelibrary.wiley.com/doi/10.1002/9781444316568.wiem02057 (accessed February 23, 2023).
Grunert, K. G., Wills, J. M., and Fernández-Celemín, L. (2010). Nutrition knowledge, and use and understanding of nutrition information on food labels among consumers in the UK. Appetite 55, 177–189. doi: 10.1016/j.appet.2010.05.045
Guiding Stars (2022). Summer Grilling on a Budget. Available online at: https://guidingstars.com/ (accessed May 9, 2023).
Gustafson, C. R. (2022). Active consideration of future health can be prompted by simple health messages and improves nutritional quality of food choices. Front. Nutr. 9, 926643. doi: 10.3389/fnut.2022.926643
Gustafson, C. R., Kent, R., and Prate, M. R. Jr. (2018). Retail-based healthy food point-of-decision prompts (PDPs) increase healthy food choices in a rural, low-income, minority community. PLoS ONE 13, e0207792. doi: 10.1371/journal.pone.0207792
Gustafson, C. R., and Rose, D. J. (2022). US consumer identification of the health benefits of dietary fiber and consideration of fiber when making food choices. Nutrients 14, 2341. doi: 10.3390/nu14112341
Habla, W., and Muller, P. (2021). Experimental evidence of limited attention at the gym. Exp. Econ. 24, 1156–1184. doi: 10.1007/s10683-020-09693-5
Hall, K. D., Ayuketah, A., Brychta, R., Cai, H., Cassimatis, T., Chen, K. Y., et al. (2019). Ultra-processed diets cause excess calorie intake and weight gain: an inpatient randomized controlled trial of ad libitum food intake. Cell Metab. 30, 67–77. doi: 10.1016/j.cmet.2019.05.020
Hare, T. A., Malmaud, J., and Rangel, A. (2011). Focusing attention on the health aspects of foods changes value signals in vmPFC and improves dietary choice. J. Neurosci. 31, 11077–11087. doi: 10.1523/JNEUROSCI.6383-10.2011
Lozano, D. I., Crites, S. L., and Aikman, S. N. (1999). Changes in food attitudes as a function of hunger. Appetite 32, 207–218. doi: 10.1006/appe.1998.0205
Marteau, T. M., Hollands, G. J., and Fletcher, P. C. (2012). Changing human behavior to prevent disease: the importance of targeting automatic processes. Science 337, 1492–1495. doi: 10.1126/science.1226918
Mehta, N. K., Abrams, L. R., and Myrskylä, M. (2020). US life expectancy stalls due to cardiovascular disease, not drug deaths. Proc. Natl. Acad. Sci. U. S. A. 117, 6998–7000. doi: 10.1073/pnas.1920391117
Morris, A., Phillips, J., Huang, K., and Cushman, F. (2021). Generating options and choosing between them depend on distinct forms of value representation. Psychol. Sci. 32, 1731–1746. doi: 10.1177/09567976211005702
Murray, C. J. L. (2013). The state of US Health, 1990-2010: burden of diseases, injuries, and risk factors. JAMA 310, 591. doi: 10.1001/jama.2013.13805
Papies, E. K., Potjes, I., Keesman, M., Schwinghammer, S., and van Koningsbruggen, G. M. (2014). Using health primes to reduce unhealthy snack purchases among overweight consumers in a grocery store. Int. J. Obes. 38, 597–602. doi: 10.1038/ijo.2013.136
Penn, J. M., and Hu, W. (2018). Understanding hypothetical bias: an enhanced meta-analysis. Am. J. Agric. Econ. 100, 1186–1206. doi: 10.1093/ajae/aay021
Peters, J., and Büchel, C. (2010). Episodic future thinking reduces reward delay discounting through an enhancement of prefrontal-mediotemporal interactions. Neuron. 66, 138–148.
Popkin, B. M. (2006). Technology, transport, globalization and the nutrition transition food policy. Food Policy 31, 554–569. doi: 10.1016/j.foodpol.2006.02.008
Popkin, B. M., and Ng, S. W. (2022). The nutrition transition to a stage of high obesity and noncommunicable disease prevalence dominated by ultra-processed foods is not inevitable. Obes. Rev. 23. doi: 10.1111/obr.13366
Preston, S. H., Vierboom, Y. C., and Stokes, A. (2018). The role of obesity in exceptionally slow US mortality improvement. Proc. Natl. Acad. Sci. U. S. A. 115, 957–961. doi: 10.1073/pnas.1716802115
R Core Team (2021). R: A Language and Environment for Statistical Computing (version 4.1.2). Available online at: http://citeseerx.ist.psu.edu/viewdoc/download?doi=10.1.1.470.5851&rep=rep1&type=pdf (accessed January 17, 2023).
Read, D., Olivola, C. Y., and Hardisty, D. J. (2017). The value of nothing: asymmetric attention to opportunity costs drives intertemporal decision making. Manage. Sci. 63, 4277–4297. doi: 10.1287/mnsc.2016.2547
Rung, J. M., Argyle, T. M., Siri, J. L., and Madden, G. J. (2018). Choosing the right delay-discounting task: completion times and rates of nonsystematic data. Behav. Process. 151, 119–125. doi: 10.1016/j.beproc.2018.03.022
Shuval, K., Stoklosa, M., Pachucki, M., Yaroch, A. L., Drope, J., and Harding, M. (2016). Economic preferences and fast food consumption in US adults: insights from behavioral economics. Prev. Med. 93, 204–210. doi: 10.1016/j.ypmed.2016.10.016
Strathman, A., Gleicher, F., Boninger, D. S., and Edwards, C. S. (1994). The consideration of future consequences: weighing immediate and distant outcomes of behavior. J. Pers. Soc. Psychol. 66, 742–752. doi: 10.1037/0022-3514.66.4.742
Toepoel, V. (2010). Is consideration of future consequences a changeable construct? Pers. Individ. Dif. 48, 951–956. doi: 10.1016/j.paid.2010.02.029
Variyam, J. N. (2008). Do nutrition labels improve dietary outcomes? Health Econ. 17, 695–708. doi: 10.1002/hec.1287
Keywords: intertemporal preferences, attention, health, food choice, consumer behavior, discounting, choice process variables
Citation: Tuyizere O and Gustafson CR (2023) The relationship of active consideration of health outcomes and intertemporal preferences to choice process variables and nutrition: evidence from an experiment on food choice. Front. Behav. Econ. 2:1219281. doi: 10.3389/frbhe.2023.1219281
Received: 08 May 2023; Accepted: 29 June 2023;
Published: 19 July 2023.
Edited by:
Arthur E. Attema, Erasmus University Rotterdam, NetherlandsReviewed by:
Peter Moffatt, University of East Anglia, United KingdomRyota Nakamura, Hitotsubashi University, Japan
Copyright © 2023 Tuyizere and Gustafson. This is an open-access article distributed under the terms of the Creative Commons Attribution License (CC BY). The use, distribution or reproduction in other forums is permitted, provided the original author(s) and the copyright owner(s) are credited and that the original publication in this journal is cited, in accordance with accepted academic practice. No use, distribution or reproduction is permitted which does not comply with these terms.
*Correspondence: Christopher R. Gustafson, cgustafson6@unl.edu