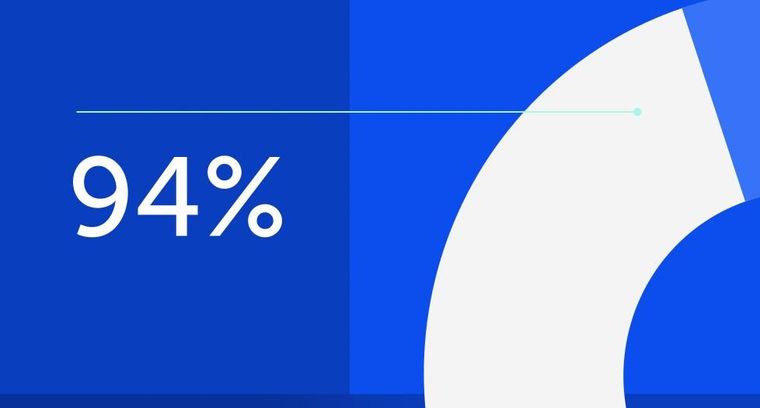
94% of researchers rate our articles as excellent or good
Learn more about the work of our research integrity team to safeguard the quality of each article we publish.
Find out more
ORIGINAL RESEARCH article
Front. Behav. Econ., 03 October 2023
Sec. Behavioral Public Policy
Volume 2 - 2023 | https://doi.org/10.3389/frbhe.2023.1205873
This article is part of the Research TopicBehavioural SpilloversView all 6 articles
Introduction: Most work on social identity, defined as one's sense of self derived from membership to social groups, focuses on a single identity and its behavioral consequences. But a central insight of social identity theory is that people belong to multiple social groups, derive self-esteem from multiple identities and care to conform to the norms for those identities. However, very little work has turned its attention to understanding when and how multiple social identities interact. We motivate hypotheses with a framework that extends a social identity model to include multiple identities.
Methods: Using a longitudinal sample (N > 600) of university students located primarily in Texas and throughout the US, we use university social identity, and the associated university norms, to characterize COVID related social distancing norms between April and October of 2020 and then unpack how another identity, the student's political identity, impacts perception of those norms.
Results: Despite incentives to do otherwise, we find that beliefs about university norms differ depending on the respondent's political identity. We interpret this as a spillover effect of attitudes from one identity to another.
Discussion: We relate our results back to a model of social identity, to the literature on spillovers where such psychological spillovers are hard to empirically identify, and to methods for future work on identity and spillovers.
Humans face significant threats to health and wellbeing that stem from complex, global, and rapidly evolving events triggered by climate change and other human activity (McMichael et al., 2006; Carlson et al., 2022). Many of these health threats unfold quickly, demand initial human behavior change prior to government policy (which often lags), and many times become highly politicized (Fuentes et al., 2020). During the wake of the COVID-19 pandemic, norms surrounding precautionary behavior, i.e., hand washing, mask wearing, and so on, quickly emerged and subsequently became highly politicized. In this study, these features of the pandemic allow us to focus on understanding when and how multiple social identities can interact and create a spillover of attitudes from one identity to another so as to impact behavior and beliefs around adoption of precautionary behavior.
Social identity is defined as one's sense of self derived from membership to social groups (Tajfel et al., 1979; Akerlof and Kranton, 2005). Central insights of Social Identity Theory are that people belong to multiple social groups, derive self-esteem from their social groups and that they care to conform to the norms for their social groups. Since the introduction of social identity into economics, work has focused on establishing the importance of social identity in being able to explain conflict between groups, human capital investment decisions, in and outgroup bias, and differences in time and risk preferences (Akerlof and Kranton, 2000, 2002; Chen and Li, 2009; Benjamin et al., 2010; Charness and Chen, 2020; Whitt et al., 2021). However, very little work has turned its attention to the consequences of having multiple social identities that may intersect in ways that impact how we understand and experience the world.1
In the present study we test for the impact of a student's political identity on their beliefs about COVID related norms for their university identity. We motivate our predictions with a framework provided by social identity theory (Akerlof and Kranton, 2000). To test these predictions we use panel data collected between April and October 2020 at three universities in Texas where COVID-19 caused students to complete their studies from their home state (locations which were distributed around the U.S.). We begin with the “behavioral” premise that precautionary behavior is both a personal decision and a social interaction. Within the social identity framework, people hold multiple identities simultaneously and, therefore, are aware of multiple group-norms (Akerlof and Kranton, 2005). Injunctive norms ascribe appropriateness to sets of actions one could take in a particular situation, are defined for each specific identity (or group), and apply to all members of the social group for that situation. The beliefs that support injunctive norms are second-order beliefs (beliefs about what others believe is appropriate or inappropriate).2 Descriptive norms ascribe expectations of frequency to sets of actions and beliefs are group specific. The beliefs that support descriptive norms are second-order beliefs (beliefs about what others believe is most commonly done).
The theory of social identity also assumes that some identities (and their norms) are more influential to the decision maker than others (Tajfel et al., 1979). Prior work suggests that political identity is likely to be among the more strongly influential identities relative to other identities.3 However, recent studies demonstrate that context, and incentives can make one identity more salient over another in a decision maker's mind (Akerlof and Kranton, 2005; Shih et al., 2006; Benjamin et al., 2010; Burks and Krupka, 2012; Chang et al., 2019). In our study we will use both incentives (in the form of cash payments to make accurate guesses) and context (in the form of evolving state mandated COVID restrictions) to increase the salience of non-political identity norms in a context where political identity may matter.
The setting for our study, the emergence of COVID-19, is particularly well-suited to this analysis for two reasons. In late December of 2019 COVID-19 emerged as a significant health threat.4 As Haushofer and Metcalf (2020) note, for most of 2020 the only approaches to reducing transmission were behavioral (hand washing, social distancing, masks, etc.). Thus, in the early months, COVID-19 could only be combated with changes in social norms and collective action on a large scale (Van Bavel et al., 2020).5 However, responses to the virus also became highly politicized (Allcott et al., 2020; Gollwitzer et al., 2020; Druckman et al., 2021; Gadarian et al., 2021; Kahane, 2021). With our data collection strategy, we use incentives to make the university identity norms for social distancing salient. We leverage the politicization of pandemic mediation efforts during our window of observation, as well as state-mandated COVID-19 restrictions, to test for the impact of an identity we do not make salient in the study, political identity, on perception of norms for our salient identity, university identity.
The literature on partisan differences in compliance with public health recommendations and attitudes toward public health policies suggests that partisan identity will matter for compliance behaviors (Gollwitzer et al., 2020; Grossman et al., 2020; Gadarian et al., 2021; Kerr et al., 2021; Simonov et al., 2022). Prior work has also shown that attitudes toward policies that restrict behavior during the pandemic were modulated by identity (Druckman et al., 2021; Van Bavel et al., 2022). Most recently, Neville et al. (2021) argued that social norms and social identities are critical to explaining, and changing, public behavior regarding adherence to COVID-19 restrictions and they make recommendations for when and how to use these social processes. But little work has documented how multiple social identities (including partisan ones) may interact to affect attitudes or perceptions of others' behavior. Within the literature on spillovers, several recent studies have begun to center social identity as a mediator to explaining behavior spillovers (Whitmarsh and O'Neill, 2010; Lacasse, 2016; Lauren et al., 2019; Verfuerth et al., 2019; Carrico, 2021). The prevailing framework for explaining spillovers with social identity assumes a social identity is primed when one behavior is adopted and this prime, in turn, causes a spillover such that a second behavior in a different domain is also adopted. For example, Dolan and Galizzi (2015) write that a feature of the spillover literature is that the spillovers in behaviors are linked “...at a conscious or unconscious level, by some underlying motive...conceptualized as deep preferences, ‘self-defining' or ‘identity goals'...” (Dolan and Galizzi, 2015, p. 3).6 In this paper, we extend the existing work on “social identity as mediators for spillovers”. We use the identity framework to characterize a simple null hypothesis for identity spillover (that perceptions of norms for one social identity will not be affected by the norms of a second identity) and then test this hypothesis.
Our contributions are to characterize the social norms during the early period of the COVID-19 pandemic and to capture the important role that multiple identities can play in shaping beliefs. One interpretation of our findings is that social identities may impact each other, such that one identity affects an actor's ability to accurately perceive the norms of another identity even when there are incentives for accurate judgment and there are government policies which coordinate behavior. We also contribute to an important literature on spillovers. Though the term “spillover” can be applied to many different phenomena, we describe spillover effects as the transfer of emotions, attitudes, or behaviors from one context to another. While the majority of papers focusing on spillovers study behavioral spillovers, and some examine the mediating role of social identity in behavior spillover, we focus on a kind of psychological spillover from one identity to another and offer a novel methodology for further investigation of this phenomenon. Normally, such psychological spillovers are hard to empirically observe. Our method of using coordination games with incentives and distinct target groups (other university students), allows us to empirically measure beliefs about norms and to test for the presence of a spillover from one identity to another.7 We highlight how social identity theory can account for this mechanism.
To motivate our empirical approach, we adopt a framework inspired by Benjamin et al. (2010). In this framework decision makers wish to comply with the norm for their social identity and increasing salience of the identity reveals the marginal effect of increasing the strength of affiliation with that category (Benjamin et al., 2010).8 We extend this framework to a scenario where there are multiple identity considerations.9
Let x be some action choice, in our case, the level of COVID-19 precautionary behavior. Individuals belong to two social categories, university (U = {Rice, TAMU, PVAMU}) and political identity (P = {Republican, Democrat, Independent}) with strength sU ≥ 0 and sP ≥ 0. Denote action x0 as the subject's preferred action in the absence of any identity considerations. Let xU denote the action prescribed for members of the social category U and let xP denote the action prescribed for members of social category P. The individual chooses to maximize the following equation:
where 0 ≤ wU(sU) is the weight placed on the university social category U and 0 ≤ wP(sP) is the weight placed on political identity social category.10 We assume that wK(0) = 0, for K = U, P. In other words, the disutility of deviating from one's category is an increasing function of the strength of that category. We assume that sU and sP have steady-state values and . It is possible that sU and sP can be perturbed away from and by a social category prime or through increased identity salience εU and εP.11 For example the strength of the identity affiliations might follow an AR(1) process such as: and . The first-order condition provides the following optimal action:
Intuitively, the agent's optimal action depends on their ideal action, the university-level social norm and the political-affiliation social norm, for example.12 Over the three waves of data collection, we may see the agents take different levels of precautionary behavior. More specifically, for those with different political affiliations, we expect that behavioral differences will be driven by wP(sp), conditional on x0 being equal across political affiliations.
In our theoretical model, perception of an unrelated identity-specific social norm is independent from another social identity. This leads us to our main hypothesis of interest:
Hypothesis 1. Elicited university-identity social norm xU is independent of political identity considerations (P).
We provide direct incentives to coordinate on the university social norm. Thus, if political identity influences responses in the coordination game or if it influences responses in the face of state-wide imposed COVID restrictions, then this feature is not included in our model.13
The project builds on samples of students from Rice University, Prairie View A&M University (PVAMU) and Texas A&M University (TAMU) that were recruited to participate in two prior studies which began in 2016.14 The same battery of questions were asked over three waves, which were administered two months apart in time. The first wave began in early April 2020, the second wave began in late July, and the last wave began mid-October 2020. During that time, universities closed and students moved back to their home state.15 Altogether 633 respondents participated in all three waves of the study. In our preferred specification, we rely on the sub-sample of subjects who completed all three waves of the survey to avoid issues of attrition.16
The norm elicitation modules elicit beliefs about the injunctive and descriptive norms and, when aggregated, provide an empirical proxy for the respective university norms. The procedure follows the method developed in Krupka and Weber (2013); just as in their paper, respondents were incentivized to coordinate their answers with other participants from the respondent's same university. We describe a specific action (social distancing, of avoiding religious services, and of avoiding hanging out with friends), and ask subjects to coordinate on rating the appropriateness (in the case of the injunctive norm) and prevalence of the action (in the case of the descriptive norm) with another subject who is a randomly chosen participant from their university.17 See the Supplementary material for the exact phrasing of the norm elicitation questions.
Respondents play a coordination game over four possible appropriateness ratings: “very socially appropriate,” “socially appropriate,” “socially inappropriate,” and “very socially inappropriate.” This description, along with the four-category scale, follows that of Krupka and Weber (2013). In the case of eliciting beliefs about the descriptive norm, respondents play a coordination game over four possible levels of activity: “Most are not doing this (<20%)”, “some are not doing this (<50%)”, “some are doing this (>50%)”, and “most are doing this (>80%)”.18 Subjects have an incentive to anticipate and match how other participants from their university will rate an action.19
We construct an individual index for beliefs about the injunctive and descriptive norm. We build this index in each wave by taking the average of the subject's beliefs about the university norms. A respondent's belief (inj.) norm index ranges from 0 (very inappropriate) to 100 (very appropriate). The belief (desc.) norm index ranges from 1 (most are not doing this) to 100 (most are doing this).
We limit our analysis to 633 subjects who completed all three waves of the survey. We summarize the time invariant controls in Supplementary Table 2 by reporting the means (along with standard errors in parentheses) and the number of observations per university in our sample.
The majority of our subjects (79%) attend Rice University, with the remaining 12% and 9% attending Texas A&M and Prairie View A&M University, respectively. Our sample consists of 18% black respondents and less than half male respondents (38%).20 About 80% of all students in our sample identify themselves as Democrats, 16% as Republicans and 4% as Independents. A large majority of students from Prairie View A&M (88%) and Rice University (81%) report that they identify with the Democratic Party. In comparison, there is more heterogeneity among the students from Texas A&M University where about 70% report identifying with the Democratic Party and 26% with the Republican Party.21
In all regressions we control for COVID-19 infection data from the Center for Systems Science and Engineering (CSSE) at Johns Hopkins University (Dong et al., 2020). Seven-day moving averages of daily new cases and deaths are computed at the county-level and are merged with respondents by both location and date completed in each of the waves of the study.
Figure 1 plots the average norm index by political affiliation across each survey wave.22 Overall, we find a downward trend in the norm indices; subjects beliefs about the university injunctive norm (what one ought to do) are softening such that actions in wave 3 are viewed as less strongly prescriptive than in wave 1. We also see that the university descriptive norm (what others are doing) to prevent the spread of COVID-19 is becoming weaker such that respondents believe fewer people are taking precautionary measures in wave 3 than wave 1.
Figure 1. Injunctive and descriptive norm indices by wave and political identity. Gray areas indicate 95% confidence intervals.
When looking at the norm index level by political affiliation, the injunctive norm index is not significantly different between Democrats and Independents. For Republicans, however, they report a university injunctive norm index that is significantly lower than the university injunctive norms index reported by Democrats in wave 1 and wave 3, although not significantly lower for wave 2 (p < 0.01 for wave 1, p > 0.1 for wave 2, and p < 0.1 for wave 3; see Supplementary Table 4). In other words, Republican student respondents believed the university injunctive norm for COVID-19 precautionary behavior was lower than what Independent and Democrat students believed.
For the descriptive norm index, we see a strong ordering of beliefs: Students who identify as Democrats believe more people are engaging in precautionary behavior than students who identify as Independents, and than students who identify as Republicans. This difference in ranking is statistically significant for wave 1 and wave 2 between Democrats and Republicans but not for other waves (p < 0.05 for wave 1, p < 0.05 for wave 2, and p > 0.1 for wave 3; see Supplementary Table 5 in Supplementary material). When we look at the norm index separately for each University (Supplementary Figures 2–4 in Supplementary material) we see similar trends. Across survey waves, injunctive norms were higher than descriptive norms.
Result 1. Student respondents who are Republicans or Democrats hold different beliefs about the university injunctive norms in wave 1 (p < 0.01) and wave 3 (p < 0.10). Student respondents who are Republicans and Democrats hold different beliefs about university descriptive norms in wave 1 (p < 0.05) and wave 2 (p < 0.05).
One possibility for why political identity is correlated with university norm perception is that perhaps the incentives to use university norms as focal points in our coordination games were not salient enough to motivate subjects to disregard the political identity norms while playing the coordination game.23 We can test this critique by using the emerging COVID restrictions over our observation window. COVID restrictions should make coordination in the norms task easier, and lead to less miscoordination, since the restrictions should cause more people to take similar social distancing actions. Subjects who want to maximize earnings in the coordination game should be able to use those restrictions to inform their guesses and especially so when forming guesses around the descriptive norm; as a result, we should see lower miscoordination in the presence of restrictions.
To test the impact of restrictions on perceptions of the norms we merge restrictions data onto our data set. The restrictions data comes from the Oxford COVID-19 Government Response Tracker (OxCGRT) maintained by the University of Oxford's Blavatnik School of Government (Hale et al., 2020). Governmental restrictions are recorded at the state-level and reported daily. We utilize their reported stringency index which is composed of nine policy measures.24 Using these measures, and re-weighting based on if the restriction policy is targeted or general, the stringency index is re-scaled such that the minimum and maximum values are between 0 and 100.25
In Figure 2, we plot the stringency index (black line) along with the precautionary behavior index (gray), beliefs (desc.) norm index (light green), and the beliefs (inj.) norm index (red line) over time for wave 1. Visually, we see that the stringency index is declining over our observation window as is the descriptive and injunctive norm. Note that this figure shows movement within a wave, as data collection was in process, as well as between waves. Smooth lines connect the three waves of data collection.
Figure 2. Daily average stringency index, beliefs about norm indices, and precautionary behavior. The straight lines in the graph above correspond to the time periods between the survey waves.
Our preferred method of measuring miscoordination is the difference between individual-level second order beliefs about the norm and the weighted modal response of the respondent's respective university within each wave.26 From the inferred level of miscoordination in each of these three questions, we compute the average level of miscoordination for descriptive norms and injunctive norms. For example, if a survey taker's responses perfectly coincided with the university-level modal response, their level of miscoordination would equal 0. The summary statistics of this constructed descriptive and injunctive norm miscoordination by political affiliation is located in Supplementary Table 8 (Supplementary material).
Result 2. We fail to find that COVID restrictions reduce miscoordination among respondents. For Republican student respondents, COVID restrictions lead to an increase in descriptive norm miscoordination, such that a 1 unit increase in the stringency index increases miscoordination by 0.01 percentage points for the descriptive norm.
We use a random effects OLS model to test for correlations between changes in miscoordination and the stringency index. The results of this regression are found in Table 1. Columns (2) and (4) contain stringency index and party affiliation interaction terms which allows for heterogeneous effects of the stringency index on miscoordination.
By looking at the estimated coefficients on the party indicator variables, we see that Republicans and Democrats have the same level of miscoordination on the injunctive norm [β = 0.03, p > 0.1, (1); β=0.07, p > 0.1, (1)]. When we include party affiliation and stringency index interaction terms, we find no evidence that this model specification fits closer to the true data generating process [Vuong Statistic = −1.03, p > 0.1, (2)]. Furthermore, we find no differences in injunctive norm miscoordination by party affiliation in response to the stringency index [β = 0.00, p > 0.1, (2)].
When we look at the descriptive norm, however, we see different levels of descriptive norm miscoordination. Republicans report lower levels of descriptive norm miscoordination [β = −0.68, p < 0.01, column (4)]. Moreover, we find that Republicans respond to changes in the stringency index are correlated with an increase in their level of descriptive norm miscoordination, such that a one unit increase in the stringency index is correlated with miscoordination by 0.01 percentage points [p < 0.01, column (4)]. Neither Independents nor Democrats respond in such a way [0.00, p > 0.1, column (4); 0.00, p > 0.1, column (4)].27
This positive coefficient on the interaction term for Republicans and the stringency index is surprising at first glance but can be explained. Intuitively, COVID-19 restrictions should result in more people doing the same thing and thus, make coordinating on prevalence of social distancing easier rather than harder. However, Republicans are the most pessimistic about the prevalence of others engaging in precautionary behavior at their university (relative to Democrats and Independents) and their beliefs remain relatively stable across waves (we see this in the means of the descriptive norms index reported in Supplementary Table 3). This implies that most of the change in miscoordination is being driven by changes in the norm rather than Republicans altering their beliefs.28 We also run specifications utilizing the unweighted norm miscoordination measures to demonstrate the robustness of our results. These regression results are contained Supplementary Table 14 in Supplementary material.29
In sum, we find that descriptive and injunctive norm miscoordination increases between waves 1 and 3 for Democrats and Independents while Republicans are mostly stable. Restrictions are loosening (per the visual evidence presented in Figure 2) and as such one might expect increased miscoordination by wave 3. However, we find that Republican beliefs regarding the descriptive norm remain largely unchanged during our observation window. Said differently, this analysis suggests that even with incentives (in the coordination game) to coordinate on university norms and with local restrictions that make behavior more uniform (affecting precisely the descriptive norm), Republicans are unable to correct for the impact of their political identity.
The rapid pace at which we face threats to our health and wellbeing stem from complex global events triggered by climate change and other human activity. These changes demand short- and long-term changes in norms and behavior. Spillover effects have the potential to both positively and negatively impact the effectiveness of interventions; as such, having a better understanding of the mechanisms for when and why we might observe them is essential. In this study we leverage a unique situation created by the COVID pandemic. Because there was no vaccine at the time of our study, the pandemic demanded rapid changes in norms. It also became highly politicized during our observation window. In this study, these features of the pandemic allow us to focus on understanding when and how multiple social identities can interact and create a spillover of attitudes from one identity to another.30
The theoretical framework provided by social identity theory allows for both heterogeneous relationships between norms and behavior (different weights, for example, on injunctive and descriptive norm conformity by reference group) and could allow for multiple identities. However, the latter aspect of the model is rarely explored. We provide evidence that joins a chorus of other findings which substantiate the claim that identity matters for behavior and that conformity to norms for identity adds additional explanatory power to organize observational data (see for example Akerlof and Kranton, 2005; Chen and Li, 2009; Benjamin et al., 2010; Chang et al., 2019). We extend this literature by providing a first empirical insight into how multiple identities interact. Akerlof and Kranton (2002) develop a theoretical framework in which people have multiple identities (they refer to them as “looks”, “jocks”, and “burnouts” in a fictional high school setting), and they focus on when someone, who could belong to multiple identities, chooses one over the other. Choice is modeled as a function of characteristic match and differential returns to identities. We show that there may be identities (to which we are more attached or which are more salient in our minds) that affect our ability to perceive the norms associated with other identities. One implication for theory is that there may be super-identities (e.g., race, gender, politics) that spillover and can be used to sufficiently predict behavior. Thus, appellations to one's identity as “a good citizen” may fall on deaf ears when political identity is a super-identity. Future work may wish to explore and integrate interactions of identities explicitly in a model.
We also contribute to literature on partisan identity and public health norms. Though numerous papers have documented how partisan identity affects compliance with public health recommendations, fewer have focused on beliefs about compliance and beliefs about public health norms which, in turn, may mediate behavior.31 Among the literature on norms, Carey et al. (2022) find that providing descriptive norm information about how many people (Americans, co-partisans, or out-partisans) report wearing masks, increases mask-wearing intentions and perceived effectiveness among Republicans and Democrats but it does not do so differently depending on whose behavior is described (Americans, co- or out-partisans).32 Rabb et al. (2022) test the role of descriptive norms on intentions to vaccinate and find the strongest relationships between vaccination intentions and social norms for respondents' friends and family (and among Republicans, co-partisans). Our paper not only introduces novel empirical data on descriptive norms for that time period, but also (and perhaps critically) adds to the less well-documented injunctive norms.
Finally, this paper is connected with the growing and urgent literature on “spillovers” as these effects can profoundly alter policy interventions aimed at encouraging behavioral change. In their recent review paper on climate change behavior and spillovers, Carrico (2021) note that recent work points to the “.., primacy of social identity processes in positive spillover.” However, this body of work tends to focus on the mediating role that social identity plays in explaining behavior spillovers. For example, Lauren et al. (2019), test how being reminded of performing “many” environmental behaviors (compared with a control condition of “few” behaviors) increases environmental intentions indirectly by changing self-perceptions; they interpret this as self-identity mediating spillovers (for a review see Carrico, 2021). We contribute to this literature by exploring spillovers between identities and providing a methodology for future work in this arena. Using an incentive compatible norm elicitation technique developed by Krupka and Weber (2013), we empirically measure beliefs about norms and test for the presence of a spillover from one identity to another. Thus, we shed light on an often difficult to observe psychological spillover that is likely correlated with behavior spillovers. In addition, we provide a methodology for future work to unpack the causal pathway from attitude spillover (rooted in social identity) to behavior or to explore whether psychological dissonance can be reduced with higher incentives in the norm elicitation task.
The datasets generated for this study can be found in the University of Michigan—Deep Blue Data repository: https://doi.org/10.7302/ghvd-xw58.
This study has received IRB approval. Texas A&M University and Texas A&M Prairie View University IRB Number: IRB2020-0379D. Rice University IRB Numbers: IRB-FY2020-278, IRB-FY2021-114. The studies were conducted in accordance with the local legislation and institutional requirements. The participants provided their written informed consent to participate in this study.
EK directed the research question and wrote the paper. HH conducted the data analysis and aided in writing the paper. CE, OO, and RW designed and administered the study and provided feedback in the writing process. RW coded, cleaned, and prepared the data for use and archiving. TR aided in creating the theoretical model. All authors contributed to the article and approved the submitted version.
This study was funded by the National Science Foundation (For Rice University, SES-1534403 and SES-2027556; for Texas A&M: SES-2027548, SES-1534411, and SES-1530796; for the University of Michigan: SES-2027513).
Thanks to Nishita Sinha, who contributed to the design of the study. Thanks to Mahmoud El-Gamal and research assistants Andy Cao, Liam Coolican, Allegra Hernandez, Carly Mayes, Sandhya Srinivas, Nanyin Yang, Sora Youn. Lab managers: Economic Research Lab, TAMU, David Cabrera; Behavioral Research Lab, Rice, Annie Pham. Portions of this manuscript have previously been made available online within the following working paper by Krupka et al. (2023).
The authors declare that the research was conducted in the absence of any commercial or financial relationships that could be construed as a potential conflict of interest.
All claims expressed in this article are solely those of the authors and do not necessarily represent those of their affiliated organizations, or those of the publisher, the editors and the reviewers. Any product that may be evaluated in this article, or claim that may be made by its manufacturer, is not guaranteed or endorsed by the publisher.
The Supplementary Material for this article can be found online at: https://www.frontiersin.org/articles/10.3389/frbhe.2023.1205873/full#supplementary-material
1. ^Ben-Ner et al. (2009) explores the difference in the relative strength of various identities (e.g., body type, political views, nationality, religion, etc.) among several experimental measures. These measures include willingness to give money to, share an office with, and commute with an in-group vs. out-group member. They find that the in-group/out-group difference in behavior was largest for familial identities (brother, father, in-laws) followed by political views, sports-team loyalty, and religion. A related stream of work has explored how multiple identities interact with one another in the context of in-group or out-group favoritism. Kumar et al. (2021) tested behavior in the canonical dictator and prisoner's dilemma game where subjects were made aware of the recipients nationality (Indian or US American) and gender (male or female) and found that subjects cooperated more with one's in-group nationality in comparison to one's in-group gender.
2. ^These are different from first-order beliefs of appropriateness, which instead are beliefs about what the individual personally considers appropriate or inappropriate. This distinction is important and discussed in Nosenzo and Görges (2020).
3. ^Though studies vary in terms of sampling strategy (population or specialized samples) and methodology (individual-level surveys, mobility data by locality, as well as county or state level compliance or mortality data), political affiliation remains an important correlate of behavior and policy preferences in the US. Democrats are more likely to comply with COVID restrictions and more likely to support policies designed to limit the spread of the virus or mitigate its impact (Allcott et al., 2020; Gollwitzer et al., 2020; Milosh et al., 2020; Pickup et al., 2020; Druckman et al., 2021; Gadarian et al., 2021; Kahane, 2021). Responses to Governors' recommendations are similarly partisan (Grossman et al., 2020). The disparities are magnified as the two groups express greater dislike for one another (Druckman et al., 2021), and trusted news sources and political messaging may have exacerbated differences (Zhao et al., 2020; Pennycook et al., 2021).
4. ^In the space of little over a year, the virus infected and killed over half a million people in the US. In addition, COVID-19 contributed to the most rapid change in the unemployment rate in modern American history (Chetty et al., 2020).
5. ^Social change is often supported by social norms that are grounded in community values and articulated around collective objectives (Sherif, 1988; Ostrom, 2000; Hardin, 2015).
6. ^See also Galizzi and Whitmarsh (2019).
7. ^In this paper, we cannot test for any correlated behavior spillovers that might stem from the psychological spillover because we don't measure behavior in 2 domains. We empirically document a normally difficult and invisible psychological process of spillovers here and future work should explore this topic.
8. ^The idea that actors wish to comply with identity-dependent social norms has been advanced in multiple papers elsewhere (Akerlof and Kranton, 2005; d'Adda et al., 2020). For example, Akerlof and Kranton (2005) note that “…much of utility depends not only on what economists normally think of as tastes, but also on norms as to how people think that they and others should behave ….” (p. 12).
9. ^We take a reduced-form approach to model norm compliance. We start with the assumption that individuals care about behaving in a manner consistent with norms rather than developing a theory of norm compliance based on underlying preferences and refer to Andreoni and Bernheim (2009) and Bénabou and Tirole (2011) for micro-foundations. Though a discussion of the origins of identity is extremely interesting, it is also not something we can do justice to in the paper. However, we note that Falk et al. (2016) have a very nice review paper and Charness and Chen (2020) follow up in 2020 with a more recent synthesis of developments in this area (see also Shayo, 2009; Carvalho, 2013; Atkin et al., 2021).
10. ^Given this formulation, it is possible for agents to have wU(SU) = 0, in which case behavior will not be influenced by xU. We also note that a linear weighted utility function is an approach taken in other literatures that look at polarization. For example, Rao and Steckel (1991) develop a model of group preferences that incorporates empirically documented group-polarization and estimate a group preference evaluation that is a weighted linear model of individual preferences with the addition of an intercept term. Kranton et al. (2020) model individual preferences using a weighted utility framework as well.
11. ^We use the assumption of steady-state identity saliently and the process of being temporarily perturbed as described in Benjamin et al. (2010).
12. ^For ease of interpretation, one may include a normalizing constant of in the utility function. This common factor, which is 1 over the sum of the three weights, ensures that the weights determine the relative rather than the absolute importance of each norm. Because utility functions are invariant to linear transformations the inclusion of this constant does not change the optimal solution in (2). We include this footnote as it may be more intuitive for some readers.
13. ^Though there are multiple reasons why individuals might report inaccurate beliefs of the university-level social norm - they may hold inaccurate beliefs due to motivated beliefs (Mobius et al., 2011; Thaler, 2021) or they have a biased representation of the university social norm due to the belief formation process (Ross et al., 1977; Prentice and Miller, 1993; Pronin et al., 2004)—finding that norm perception is influenced by an other identity (here political identity) would be an important contribution for how we model social identity.
14. ^Rice University is a private research university in Houston, Texas; Texas A&M is a large public land-grant research university in College Station, Texas and the flagship institution of the Texas A&M University system; and PVAMU is a historically black university also in the Texas A&M University System. See the Supplementary material for additional information on these prior studies.
15. ^We discuss this and further study details in Supplementary Figure 1 (Supplementary material).
16. ^Supplementary Table 1 in Supplementary material reports the differences in mean demographic variables of those who complete all three waves and those who did not complete all three waves.
17. ^We chose this set of three descriptive and injunctive norm questions as they were elicited throughout all three waves.
18. ^Krupka and Weber (2013) provide evidence that collectively-recognized social norms create focal points in these matching game (see also Schelling, 1980; Mehta et al., 1994; Sugden, 1995; Goerg and Walkowitz, 2010).
19. ^Details of the experimental design can be found in the Supplementary material.
20. ^For Prairie View A&M University, only 8% of the respondents were men. When we loosen the inclusion restriction of our sample and allow for individuals which do not have all three wave observations, we see that 19% of the Prairie View A&M University respondents are male, in comparison to 46% for Rice University and 39% for Texas A&M University. This gender difference for Prairie View, however, may be attributable to the fact that 60.9% of the incoming freshmen in 2016 were females.
21. ^Supplementary Table 1 in Supplementary material shows that the in-sample and out-sample group statistically differ across university and race, but not among political-identity.
22. ^The numbers used in the figure can be found in Supplementary Table 3 (Supplementary material) which reports the injunctive norm and descriptive norm index by political identity across all three waves.
23. ^Veselỳ (2015) finds that people provide virtually the same responses in incentivized and non-incentivized versions of the Krupka-Weber game. A working paper by König-Kersting (2021) elicits dictator game norms and systematically changes the salience of incentives by explaining the incentive once (at the beginning) or at each choice during the experiment, removing the financial incentive and either asking for first order or second order beliefs. The author finds no differences in ratings across treatments for the dictator game. However, in the above two papers, subjects may not have been experiencing any significant or differential cognitive distress associated with the task. As one referee points out, in the current situation, subjects might require more compensation to give up keeping a certain consistent self-image compared to the utility from the extra money of guessing the correct norm.
24. ^These include school closing, workplace closing, canceling of public events, restrictions on gathering size, closure of public transport, restrictions on internal movement, restrictions on international travel, and public information campaigns.
25. ^Several papers have used other sources for policy restrictions, such as data provided by the National Association of Counties (NACo)—County Explorer (Amuedo-Dorantes et al., 2021; Brodeur et al., 2021). We opt to use the Oxford COVID-19 Response Tracker as policies are reported throughout our entire time period of interest whereas the NACo data was last updated on April 15 2020.
26. ^For Texas A&M, the observed modal response does not correspond to the university-level modal response, as the survey intentionally over-sampled Black students from a previous study. To correct for this over-sampling, we calculate survey weights by iterative proportional fitting (raking) and use the race distribution of each university in Fall of 2020. The sum of the weights, as opposed to the sum of the observations, is used to determine the modal response of each norm elicitation task. Our results are robust to the specification were we use the observed modal response to calculate miscoordination instead of the weighted modal response.
27. ^We also find that controlling for the heterogeneous response to the stringency index by political affiliation is influential to the model fit, given the Vuong statistic of −2.37 [p < 0.05, column (4)].
28. ^This can also be seen in Supplementary Table 8 (Supplementary material). As a robustness check, we run an alternative regression with an multiordinal logit with mixed effects. The results are quantitatively similar and can be found in Supplementary Table 13.
29. ^In the Supplementary material, we also show that this ‘clouding' of perception of university norms does not happen when the context is a-political. A description of the robustness check and the results of these tests are presented in Supplementary Table 11.
30. ^However, as one referee points out, the current study context does not feature a counterfactual case. The pandemic and political party support we identify here are a particular scenario, because being a Republican and not perceiving the rules in a pandemic as norms correlates with other preferences.
31. ^COVID offered the opportunity to investigate the critical role that partisan identity can play in co-determining compliance to public health policies (Druckman et al., 2020; Gollwitzer et al., 2020; Grossman et al., 2020; Gadarian et al., 2021; Kerr et al., 2021; Simonov et al., 2022; Dayton and Dragojevic, 2023).
32. ^Shin et al. (2022) use a social identity framework to study how political identity impacts precautionary behavior promotion. They find that having a Republican identity, and in particular, if the respondent expressed increased loyalty to the Trump leadership, it was associated with a significantly lower level of mask promotion.
Akerlof, G. A., and Kranton, R. E. (2000). Economics and identity. Q. J. Econ. 115, 715–753. doi: 10.1162/003355300554881
Akerlof, G. A., and Kranton, R. E. (2002). Identity and schooling: some lessons for the economics of education. J. Econ. Lit. 40, 1167–1201. doi: 10.1257/.40.4.1167
Akerlof, G. A., and Kranton, R. E. (2005). Identity and the economics of organizations. J. Econ. Perspect. 19, 9–32. doi: 10.1257/0895330053147930
Allcott, H., Boxell, L., Conway, J., Gentzkow, M., Thaler, M., and Yang, D. (2020). Polarization and public health: Partisan differences in social distancing during the coronavirus pandemic. J. Public Econ. 191, 104254. doi: 10.1016/j.jpubeco.2020.104254
Amuedo-Dorantes, C., Kaushal, N., and Muchow, A. N. (2021). Timing of social distancing policies and COVID-19 mortality: county-level evidence from the US. J. Popul. Econ. 34, 1445–1472. doi: 10.1007/s00148-021-00845-2
Andreoni, J., and Bernheim, B. D. (2009). Social image and the 50–50 norm: a theoretical and experimental analysis of audience effects. Econometrica 77, 1607–1636. doi: 10.3982/ECTA7384
Atkin, D., Colson-Sihra, E., and Shayo, M. (2021). How do we choose our identity? A revealed preference approach using food consumption. J. Polit. Econ. 129, 1193–1251. doi: 10.1086/712761
Bénabou, R., and Tirole, J. (2011). Identity, morals, and taboos: beliefs as assets. Q. J. Econ. 126, 805–855. doi: 10.1093/qje/qjr002
Benjamin, D. J., Choi, J. J., and Strickland, A. J. (2010). Social identity and preferences. Am. Econ. Rev. 100, 1913–1928. doi: 10.1257/aer.100.4.1913
Ben-Ner, A., McCall, B. P., Stephane, M., and Wang, H. (2009). Identity and in-group/out-group differentiation in work and giving behaviors: experimental evidence. J. Econ. Behav. Organ. 72, 153–170. doi: 10.1016/j.jebo.2009.05.007
Brodeur, A., Grigoryeva, I., and Kattan, L. (2021). Stay-at-home orders, social distancing, and trust. J. Popul. Econ. 34, 13021–1354. doi: 10.1007/s00148-021-00848-z
Burks, S. V., and Krupka, E. (2012). A multimethod approach to identifying norms and normative expectations within a corporate hierarchy: evidence from the financial services industry. Manage. Sci. 58, 203–217. doi: 10.1287/mnsc.1110.1478
Carey, J., Nyhan, B., Phillips, J. B., and Reifler, J. (2022). Partisanship unmasked? The role of politics and social norms in covid-19 mask-wearing behavior. J. Exp. Polit. Sci. 1–14. doi: 10.1017/XPS.2022.20
Carlson, C. J., Albery, G. F., Merow, C., Trisos, C. H., Zipfel, C. M., Eskew, E. A., et al. (2022). Climate change increases cross-species viral transmission risk. Nature 607, 555–562. doi: 10.1038/s41586-022-04788-w
Carrico, A. R. (2021). Climate change, behavior, and the possibility of spillover effects: recent advances and future directions. Curr. Opin. Behav. Sci. 42, 76–82. doi: 10.1016/j.cobeha.2021.03.025
Chang, D., Chen, R., and Krupka, E. (2019). Rhetoric matters: a social norms explanation for the anomaly of framing. Games Econ. Behav. 116, 158–178. doi: 10.1016/j.geb.2019.04.011
Charness, G., and Chen, Y. (2020). Social identity, group behavior, and teams. Annu. Rev. Econ. 12, 691–713. doi: 10.1146/annurev-economics-091619-032800
Chen, Y., and Li, S. X. (2009). Group identity and social preferences. Am. Econ. Rev. 99, 431–57. doi: 10.1257/aer.99.1.431
Chetty, R., Friedman, J. N., Hendren, N., Stepner, M., et al. (2020). How Did Covid-19 and Stabilization Policies Affect Spending and Employment? A New Real-Time Economic Tracker Based on Private Sector Data. Technical report, National Bureau of Economic Research.
d'Adda, G., Dufwenberg, M., Passarelli, F., and Tabellini, G. (2020). Social norms with private values: theory and experiments. Games Econ. Behav. 124, 288–304. doi: 10.1016/j.geb.2020.08.012
Dayton, Z. A., and Dragojevic, M. (2023). When wearing a mask is (not) the norm: political partisanship and persuasion in the context of COVID-19. Commun. Rep. 36, 1–14. doi: 10.1080/08934215.2022.2081710
Dolan, P., and Galizzi, M. M. (2015). Like ripples on a pond: behavioral spillovers and their implications for research and policy. J. Econ. Psychol. 47, 1–16. doi: 10.1016/j.joep.2014.12.003
Dong, E., Du, H., and Gardner, L. (2020). An interactive web-based dashboard to track COVID-19 in real time. Lancet Infect. Dis. 20, 533–534. doi: 10.1016/S1473-3099(20)30120-1
Druckman, J., Klar, S., Kkrupnikov, Y., Levendusky, M., and Ryan, J. B. (2020). The political impact of affective polarization: how Partisan animus shapes COVID-19 attitudes. doi: 10.31234/osf.io/ztgpn
Druckman, J. N., Klar, S., Krupnikov, Y., Levendusky, M., and Ryan, J. B. (2021). Affective polarization, local contexts and public opinion in America. Nat. Hum. Behav. 5, 28–38. doi: 10.1038/s41562-020-01012-5
Falk, A., Becker, A., Dohmen, T., Huffman, D. B., and Sunde, U. (2016). The Preference Survey Module: A Validated Instrument for Measuring Risk, Time, and Social Preferences. Technical report, IZA Discussion Papers.
Fuentes, R., Galeotti, M., Lanza, A., and Manzano, B. (2020). COVID-19 and climate change: a tale of two global problems. Sustainability 12, 8560. doi: 10.3390/su12208560
Gadarian, S. K., Goodman, S. W., and Pepinsky, T. B. (2021). Partisanship, health behavior, and policy attitudes in the early stages of the COVID-19 pandemic. PLoS ONE 16, e0249596. doi: 10.1371/journal.pone.0249596
Galizzi, M. M., and Whitmarsh, L. (2019). How to measure behavioral spillovers: a methodological review and checklist. Front. Psychol. 10, 342. doi: 10.3389/fpsyg.2019.00342
Goerg, S. J., and Walkowitz, G. (2010). On the prevalence of framing effects across subject-pools in a two-person cooperation game. J. Econ. Psychol. 31, 849–859. doi: 10.1016/j.joep.2010.06.001
Gollwitzer, A., Martel, C., Brady, W. J., Pärnamets, P., Freedman, I. G., Knowles, E. D., et al. (2020). Partisan differences in physical distancing are linked to health outcomes during the COVID-19 pandemic. Nat. Hum. Behav. 4, 1186–1197. doi: 10.1038/s41562-020-00977-7
Grossman, G., Kim, S., Rexer, J. M., and Thirumurthy, H. (2020). Political partisanship influences behavioral responses to governors' recommendations for COVID-19 prevention in the United States. Proc. Natl. Acad. Sci. U.S.A. 117, 24144–24153. doi: 10.1073/pnas.2007835117
Hale, T., Webster, S., Petherick, A., Phillips, T., and Kira, B. (2020). Oxford COVID-19 Government Response Tracker. Data Use Policy: Creative Commons Attribution CC BY Standard.
Haushofer, J., and Metcalf, J. (2020). Combining Behavioral Economics and Infectious Disease Epidemiology to Mitigate the COVID-19 Outbreak. Princeton University.
Kahane, L. H. (2021). Politicizing the mask: political, economic and demographic factors affecting mask wearing behavior in the USA. East. Econ. J. 47, 163–183. doi: 10.1057/s41302-020-00186-0
Kerr, J., Panagopoulos, C., and Van Der Linden, S. (2021). Political polarization on COVID-19 pandemic response in the united states. Pers. Individ. Diff. 179, 110892. doi: 10.1016/j.paid.2021.110892
König-Kersting, C. (2021). On the Robustness of Social Norm Elicitation. Technical report, Working Papers in Economics and Statistics.
Kranton, R., Pease, M., Sanders, S., and Huettel, S. (2020). Deconstructing bias in social preferences reveals groupy and not-groupy behavior. Proc. Natl. Acad. Sci. U.S.A. 117, 21185–21193. doi: 10.1073/pnas.1918952117
Krupka, E., Hoover, H., Eckel, C., Rosenblat, T., Ojumu, O., and Wilson, R. (2023). “Multiple social identities cloud norm perception: responses to covid-19 among university aged republicans and democrats,” in Working Paper.
Krupka, E., and Weber, R. (2013). Identifying social norms using coordination games: why does dictator game sharing vary? J. Eur. Econ. Assoc. 11, 495–524. doi: 10.1111/jeea.12006
Kumar, M. M., Tsoi, L., Lee, M. S., Cone, J., and McAuliffe, K. (2021). Nationality dominates gender in decision-making in the dictator and prisoner's dilemma games. PLoS ONE 16, e0244568. doi: 10.1371/journal.pone.0244568
Lacasse, K. (2016). Don't be satisfied, identify! strengthening positive spillover by connecting pro-environmental behaviors to an “environmentalist” label. J. Environ. Psychol. 48, 149–158. doi: 10.1016/j.jenvp.2016.09.006
Lauren, N., Smith, L. D., Louis, W. R., and Dean, A. J. (2019). Promoting spillover: how past behaviors increase environmental intentions by cueing self-perceptions. Environ. Behav. 51, 235–258. doi: 10.1177/0013916517740408
McMichael, A. J., Woodruff, R. E., and Hales, S. (2006). Climate change and human health: present and future risks. Lancet 367, 859–869. doi: 10.1016/S0140-6736(06)68079-3
Mehta, J., Starmer, C., and Sugden, R. (1994). The nature of salience: an experimental investigation of pure coordination games. Am. Econ. Rev. 84, 658–673.
Milosh, M., Painter, M., Van Dijcke, D., and Wright, A. L. (2020). Unmasking Partisanship: How Polarization Influences Public Responses to Collective Risk. University of Chicago; Becker Friedman Institute for Economics Working Paper, 102.
Mobius, M., Niederle, M., Niehaus, P., and Rosenblat, T. (2011). Managing Self-Confidence: Theory and Experimental Evidence. Technical report, National Bureau of Economic Research.
Neville, F. G., Templeton, A., Smith, J. R., and Louis, W. R. (2021). Social norms, social identities and the COVID-19 pandemic: theory and recommendations. Soc. Pers. Psychol. Compass 15, e12596. doi: 10.1111/spc3.12596
Nosenzo, D., and Görges, L. (2020). Measuring social norms in economics: why it is important and how it is done. Analyse Kritik 42, 285–312. doi: 10.1515/auk-2020-0012
Ostrom, E. (2000). Collective action and the evolution of social norms. J. Econ. Perspect. 14, 137–158. doi: 10.1257/jep.14.3.137
Pennycook, G., McPhetres, J., Bago, B., and Rand, D. G. (2021). Beliefs about COVID-19 in Canada, the UK, and the USA: a novel test of political polarization and motivated reasoning. Pers. Soc. Psychol. Bull. 48, 750–765. doi: 10.1177/01461672211023652
Pickup, M., Stecula, D., and Van Der Linden, C. (2020). Novel coronavirus, old partisanship: COVID-19 attitudes and behaviours in the United States and Canada. Can. J. Polit. Sci. 53, 357–364. doi: 10.1017/S0008423920000463
Prentice, D. A., and Miller, D. T. (1993). Pluralistic ignorance and alcohol use on campus: some consequences of misperceiving the social norm. J. Pers. Soc. Psychol. 64, 243. doi: 10.1037/0022-3514.64.2.243
Pronin, E., Gilovich, T., and Ross, L. (2004). Objectivity in the eye of the beholder: divergent perceptions of bias in self versus others. Psychol. Rev. 111, 781. doi: 10.1037/0033-295X.111.3.781
Rabb, N., Bowers, J., Glick, D., Wilson, K. H., and Yokum, D. (2022). The influence of social norms varies with “others” groups: evidence from covid-19 vaccination intentions. Proc. Natl. Acad. Sci. U.S.A. 119, e2118770119. doi: 10.1073/pnas.2118770119
Rao, V. R., and Steckel, J. H. (1991). A polarization model for describing group preferences. J. Cons. Res. 18, 108–118. doi: 10.1086/209245
Ross, L., Greene, D., and House, P. (1977). The “false consensus effect”: an egocentric bias in social perception and attribution processes. J. Exp. Soc. Psychol. 13, 279–301.
Shayo, M. (2009). A model of social identity with an application to political economy: nation, class, and redistribution. Am. Polit. Sci. Rev. 103, 147–174. doi: 10.1017/S0003055409090194
Sherif, M. (1988). The Robbers Cave Experiment: Intergroup Conflict and Cooperation [Orig. pub. as Intergroup conflict and group relations]. Middletown, CT: Wesleyan University Press.
Shih, M., Pittinsky, T. L., and Trahan, A. (2006). Domain-specific effects of stereotypes on performance. Self Identity 5, 1–14. doi: 10.1080/15298860500338534
Shin, J., Yang, A., Liu, W., Min Kim, H., Zhou, A., and Sun, J. (2022). Mask-wearing as a Partisan issue: social identity and communication of party norms on social media among political elites. Soc. Media Soc. 8, 20563051221086233. doi: 10.1177/20563051221086233
Simonov, A., Sacher, S., Dubé, J.-P., and Biswas, S. (2022). Frontiers: the persuasive effect of fox news: noncompliance with social distancing during the COVID-19 pandemic. Market. Sci. 41, 230–242. doi: 10.1287/mksc.2021.1328
Tajfel, H., Turner, J. C., Austin, W. G., and Worchel, S. (1979). An integrative theory of intergroup conflict. Organ. Ident. Reader 56–65.
Thaler, M. (2021). The supply of motivated beliefs. arXiv preprint arXiv:2111.06062. doi: 10.48550/arXiv.2111.06062
Van Bavel, J. J., Baicker, K., Boggio, P. S., Capraro, V., Cichocka, A., Cikara, M., et al. (2020). Using social and behavioural science to support covid-19 pandemic response. Nat. Hum. Behav. 4, 460–471. doi: 10.1038/s41562-020-0884-z
Van Bavel, J. J., Cichocka, A., Capraro, V., Sjåstad, H., Nezlek, J. B., Pavlović, T., et al. (2022). National identity predicts public health support during a global pandemic. Nat. Commun. 13, 517. doi: 10.1038/s41467-021-27668-9
Verfuerth, C., Jones, C. R., Gregory-Smith, D., and Oates, C. (2019). Understanding contextual spillover: using identity process theory as a lens for analyzing behavioral responses to a workplace dietary choice intervention. Front. Psychol. 10, 345. doi: 10.3389/fpsyg.2019.00345
Veselỳ, Š. (2015). Elicitation of normative and fairness judgments: do incentives matter? Judgment Decis. Mak. 10, 191–197. doi: 10.1017/S1930297500003958
Whitmarsh, L., and O'Neill, S. (2010). Green identity, green living? the role of pro-environmental self-identity in determining consistency across diverse pro-environmental behaviours. J. Environ. Psychol. 30, 305–314. doi: 10.1016/j.jenvp.2010.01.003
Whitt, S., Wilson, R. K., and Mironova, V. (2021). Inter-group contact and out-group altruism after violence. J. Econ. Psychol. 86, 102420. doi: 10.1016/j.joep.2021.102420
Keywords: COVID-19, norms, preferences, social identity, norm miscoordination, spillovers
Citation: Krupka E, Hoover H, Eckel C, Rosenblat T, Ojumu O and Wilson RK (2023) Multiple social identities cloud norm perception: responses to COVID-19 among university aged Republicans and Democrats. Front. Behav. Econ. 2:1205873. doi: 10.3389/frbhe.2023.1205873
Received: 14 April 2023; Accepted: 04 September 2023;
Published: 03 October 2023.
Edited by:
Filippos Exadaktylos, Loyola Andalusia University, SpainReviewed by:
Ennio Bilancini, IMT School for Advanced Studies Lucca, ItalyCopyright © 2023 Krupka, Hoover, Eckel, Rosenblat, Ojumu and Wilson. This is an open-access article distributed under the terms of the Creative Commons Attribution License (CC BY). The use, distribution or reproduction in other forums is permitted, provided the original author(s) and the copyright owner(s) are credited and that the original publication in this journal is cited, in accordance with accepted academic practice. No use, distribution or reproduction is permitted which does not comply with these terms.
*Correspondence: Erin Krupka, ZWtydXBrYUB1bWljaC5lZHU=
Disclaimer: All claims expressed in this article are solely those of the authors and do not necessarily represent those of their affiliated organizations, or those of the publisher, the editors and the reviewers. Any product that may be evaluated in this article or claim that may be made by its manufacturer is not guaranteed or endorsed by the publisher.
Research integrity at Frontiers
Learn more about the work of our research integrity team to safeguard the quality of each article we publish.