- University Grenoble Alpes, CNRS, UMR 5525, VetAgro Sup, Grenoble INP, CHU Grenoble Alpes, TIMC, Grenoble, France
The purpose of this review is to explore how clinical metabolomics could help physicians in the future. The recent advent of medical genomics brings new and interesting technological tools to measure genetic predispositions to a disease. But metabolomics will allow us to go even further by linking the patient’s pathological phenotype with gene expression defects and metabolic disorders. It is in this context that the clinical chemist must adapt and be a force of proposal to meet these health challenges. He must help the clinician by mastering these new innovative tools, in order to participate in the implementation of clinical studies for the discovery of biomarkers, but also to propose the assays of biomarkers called “signatures,” which can be composite biomarkers or fingerprints, which will ultimately guide the clinician. He will have to propose them as clinical chemistry tests. In the first part, we will look at some concrete examples of the use of clinical metabolomics in clinical research projects that have led to the identification of a new biomarker. We will use the example of trimethylamine N-oxide (or TMAO) and review the clinical studies that have proposed TMAO as a biomarker for cardiovascular diseases. In a second part, we will see through bibliographic studies, how the metabolomic fingerprint can be useful to build a supervised model for patient stratification. In conclusion, we will discuss the limitations currently under debate.
1 Introduction
Metabolomics, one of the recent omics techniques, has emerged thanks to the continuous development of analytical techniques and bioinformatics. In general, the metabolomics approach is based on the use of advanced analytical chemistry tools, such as nuclear magnetic resonance (NMR) and mass spectrometry (MS) coupled with different chromatographic methods [liquid-chromatography (LC-MS) or Gas-chromatography (GC-MS)] (Alseekh et al., 2021), to measure and characterize the metabolome corresponding to the molecules < 1.5 kDa. The examination of metabolites follows two strategies: 1) targeted metabolomics, driven by the measure of a specific set of metabolites and 2) untargeted metabolomics, driven by an unbiased approach in which as many as possible metabolites (including unknown metabolites) are measured and compared between samples. By the latter approach, thousands of metabolite features [i.e., peaks corresponding to individual ions with a unique mass-to-charge (m/z) ratio and retention time (RT)] can be routinely detected by LC/MS based methods. The use of either approach is to be decided based on the desired applications (Roberts et al., 2012; Schrimpe-Rutledge et al., 2016).
The metabolome can be studied both at the intracellular level, highlighting functional abnormalities at the cellular level, and at the peripheral level (for instance in blood plasma), which contains numerous metabolites (Wishart et al., 2022), reflective of organ metabolic activity and thus carrying pathophysiological information to be exploited. It is easy to understand that the study of metabolites can reveal a dysfunction of one or several organs, since the organs can be assimilated to highly specialized “metabolic engines” (for example, the liver produces bile acids and urea, the thyroid gland produces thyroxine, the adrenal glands produce cortisol and epinephrine, etc.). Some metabolites in the human blood are produced endogenously and others come from the environment (Psychogios et al., 2011). The variability observed in the metabolome is on the one hand of hereditary origin (Shin et al., 2014; Long et al., 2017; Lotta et al., 2021), and on the other hand influenced mainly by gut microbiota (Wikoff et al., 2009), by lifestyle habits such as smoking (Xu et al., 2013) or physical activity, or by diet (Playdon et al., 2016). Diet and the microbiome contribute the most to individual metabolic variability, and make each person’s metabotype unique (Bar et al., 2020). In contrast, without significant changes in health status, each individual’s metabotype is relatively stable over time. Tracking changes in metabotypes over several years would therefore allow the evolution of health status to be traced and could provide indications of disease onset (Yousri et al., 2014).
Metabolomics shares common features with clinical biochemistry, as the latter has historically sought to determine standard and abnormal parameters of small molecular compounds in blood and other biological fluids (e.g., blood glucose, creatinine, etc.). Among the biomarkers currently used in clinical laboratory medicine, a large number are metabolites. Hence, medical biochemists can be considered to have been practising metabolomics for a long time without knowing it (Roux et al., 2012). In any case, one of the main assets of metabolomics is to identify new biomarkers that are easily transferable to the medical laboratory. To date, we have several metabolomics normal data sets from these biological fluids (e.g., blood (Psychogios et al., 2011), urine (Bouatra et al., 2013), CSF (Wishart et al., 2008), or feces (Karu et al., 2018)). Clinical metabolomics can be considered like clinical chemistry, but it is more comprehensive, faster, and cheaper according to David Wishart (Wishart, 2019). The term clinical metabolomics appeared in the literature for the first time in 2009, although the concept was described shortly before by J.K Nicholson & J. C Lindon (Nicholson and Lindon, 2008). Indeed, Uta Ceglarek proposes the following definition: “‘Clinical metabolomics’ aims at evaluating and predicting health and disease risk in an individual by investigating metabolic signatures in body fluids or tissues, which are influenced by genetics, epigenetics, environmental exposures, diet, and behaviour” (Ceglarek et al., 2009). By definition, metabolic signatures contain a panel or a combination of affected metabolites. Although urine analysis has been used as a non-invasive and cost-effective tool for disease screening, diagnosis, and monitoring since ancient times, the first study to measure urinary metabolomic biomarkers of cancer using NMR and MS was published in 2006. Since then, these techniques have been used to detect cancers of the urologic system (kidney, prostate, and bladder) and non-urologic tumors, including those of the breast, ovary, lung, liver, gastrointestinal tract, pancreas, bone, and blood (Dinges et al., 2019). And moreover, several papers have proposed metabolomics as a method for discovering metabolic biomarkers for several other important diseases such as diabetes, neurodegenerative diseases (Chang et al., 2022), atherosclerosis, and lung diseases (Xia et al., 2013a; Wheelock et al., 2013; Wishart, 2016; Letertre et al., 2021).
The first part of this work will emphasize the role of metabolomics as a tool for the discovery of new biomarkers, taking as an example the identification of TMAO, and tracing the history of the validation of this biomarker in cardiac pathologies. The second part highlights the interest of clinical metabolomics and the identification of metabolic fingerprints to characterize the health status of patients in a hospital setting.
2 How metabolomics help to find new biomarker?
In order to understand how metabolomics can identify new clinically useful biomarkers, and what are the different steps essential to do so, we chose to trace the different stages of the discovery of the metabolite biomarker, Trimethylamine N-oxide (TMAO), in cardiovascular diseases (CVD) (Figure 1).
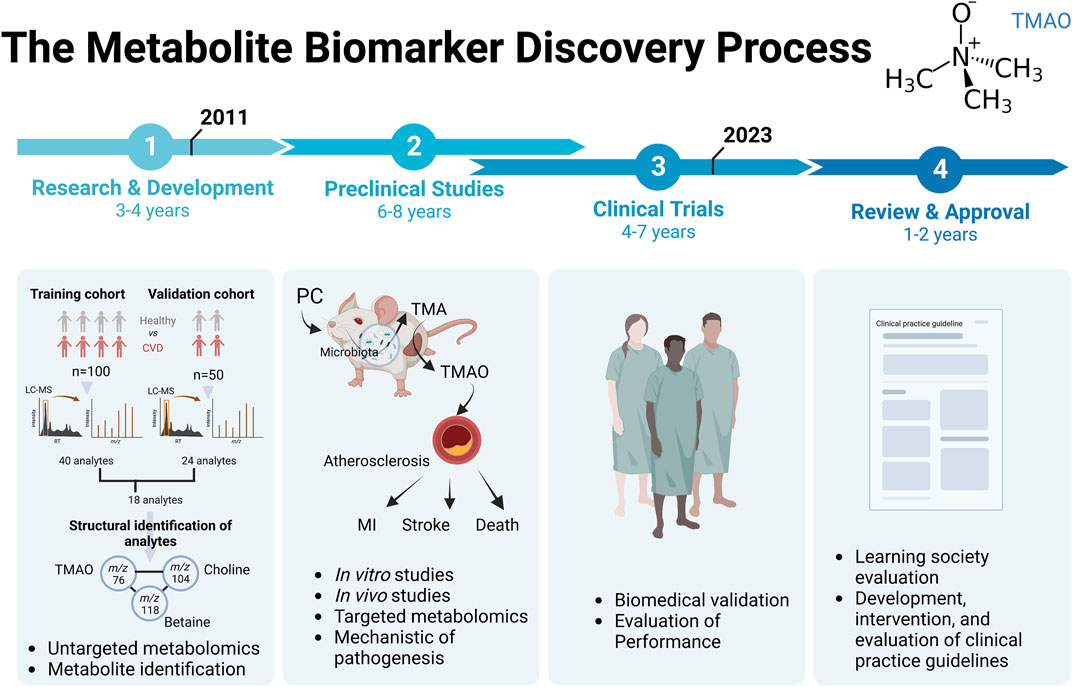
FIGURE 1. The metabolite biomarker discovery process for Trimethylamine N-Oxyde (TMAO) in evaluation of risk of CVD. Abbreviation: PC, phosphatidylcholine; MI, myocardial infarction.
The story of the discovery of this potential biomarker begins with the publication in 2011, when researchers published the first demonstration of the use of untargeted metabolomics to identify metabolites’ biomarkers, predictive of CVD development (Wang et al., 2011). A first part of the study used LC-MS metabolomics on a “training” cohort. This cohort of 100 plasma from stable patients undergoing cardiac evaluation had been sampled, before half of them suffered a myocardial infarction (MI), stroke, or death during the subsequent 3-year period. Control subjects were age- and sex-matched subjects who had not experienced a cardiovascular event during the period. The analysis identified a list of 40 analytes associated with increased cardiovascular risk. The 40 analytes selected in this step were defined according to their RT and m/z ratio detected in the LC-MS analysis. The second step consisted in using an independent “validation” cohort of 50 new patients, and allowed to confirm 18 analytes, which met the criteria of acceptability in the training and validation cohorts. Then, the structural identification of the 18 small molecules present in the plasma had been carried out, in particular by using NMR, MSn, LC/MS/MS and GC/MS/MS and experiments carried out in animals with orally administered deuterated molecules. Among the 18 analytes, the metabolite with m/z 76 was identified as the TMAO. Moreover, in these first clinical studies, a significant correlation was found between the analytes of m/z 76, 104 and 118 (p < 0.001) suggesting a common metabolic pathway. This was later confirmed, the m/z 104 molecule was choline, and the m/z 118 molecule was betaine. At that time, it was known that lipid-rich foods of the phosphatidylcholine (PC, also known as lecithin) family, including mainly eggs, milk, liver, red meat, poultry, shellfish and fish, were considered to be the main dietary sources of choline, and thus of TMAO production (Zeisel et al., 2003).
Experiments on animals, notably via oral gavage with choline, had been decisive in identifying betaine (m/z 118) but also in understanding the pathophysiological mechanism and the role of the microbiota in this mechanism. First, it was found that oral administration of choline in a controlled animal experiment is absolutely necessary to observe the accumulation of TMAO in the blood of the gavaged animals. When d9-PC and/or d9-choline were administered orally, pretreatment of the mice with a broad-spectrum antibiotic treatment for 3 weeks affecting the intestinal microbiota completely suppressed the appearance of d9-TMAO in the plasma. These results were reversible when the flora was restored before administering the oral treatment, confirming the need for the presence of a gut microbiota to recover plasma TMAO from PC in the diet.
Subsequently, to confirm that plasma levels of TMAO, choline and betaine were good predictive biomarkers of cardiovascular risk, a new metabolomics study, but this time targeted on these molecules of interest, was carried out on a large cohort of patients (n = 1,876). They had shown that elevated levels of choline, TMAO, and betaine all showed dose-dependent associations with the presence of CVD and multiple individual CVD phenotypes, including peripheral arterial disease (PAD), coronary heart disease (CHD), and history of MI. An association between increased risk of all CVD phenotypes and elevated systemic levels of these 3 metabolites, choline, TMAO, and betaine, was confirmed after adjustment for traditional cardiac risk factors and medication use.
Finally, the use of animal models once again validated the pro-atherosclerotic effect of a diet rich in choline or TMAO. The analyses showed an increase in atherosclerosis and a correlation between the size of the atherosclerotic plaques and the plasma level of TMAO, independently of other classical markers such as the level of cholesterol, triglycerides, lipoproteins, or glycemia, but dependent on the presence of intestinal microbiota. Choline consumption increased aortic lesions and atherosclerosis in the animals by a factor of 3, through a mechanism involving pro-atherogenic aortic macrophages and the gut microbiota. Finally, the researchers suggested the involvement of the hepatic enzyme, Flavin monooxygenase type 3 (FMO3), because its expression level in a liver biopsy was correlated with the level of plasma TMAO in mice and in humans.
In the New England Journal of Medicine published in 2013 (Tang et al., 2013), Prof. Hazen’s team confirmed these observations by performing a new targeted metabolomics study on a cohort of patients (n = 4,007) eligible for coronary angiography and followed for 3 years, and demonstrated the link between PC metabolism by the gut microbiota and cardiovascular risk. TMAO, choline, and betaine were measured in plasma using a mass spectrometer classically used in medical analysis laboratories (ABSCIEX Q TRAP 5500) coupled to LC. In addition, they conducted a study in 40 participants supplemented with dietary PC, as well as a capsule containing a deuterium-labelled PC (d9-PC). Supplementation increased the concentration of TMAO and d9-TMAO in the plasma and urine of these patients. For the 6 patients who had received antibiotic treatment for 1 week, plasma detection of TMAO and d9-TMAO was abolished. 1 month later, the microbiota had been restored and the patients had been re-supplemented, allowing to confirm the human gut microbiota-dependent increase in plasma TMAO and d9-TMAO after PC consumption.
In all patients, after adjusting for traditional risk factors and other baseline co-variates, high plasma TMAO levels remained a significant predictor of the risk of severe cardiovascular events. They observed a gradual increase in risk with increasing TMAO levels. When the components of major adverse cardiovascular events were analyzed separately, high levels of TMAO remained significantly associated with an increased risk of death (hazard ratio, 3.37; 95% CI, 2.39 to 4.75; p < 0.001) and non-fatal MI or stroke (hazard ratio, 2.13; 95% CI, 1.48 to 3.05; p < 0.001). The inclusion of TMAO as a covariate had resulted in a significant improvement in risk estimation compared with traditional risk factors [improvement in net reclassification, 8.6% (p < 0.001); improvement in integrated discrimination, 9.2% (p < 0.001); C statistic, 68.3% versus 66.4% (p = 0.01)]. Moreover, a study on a cohort of 760 healthy women at baseline, shows that long-term increases in TMAO over 10 years were associated with higher CHD risk (Heianza et al., 2020).
Other clinical studies had also shown the prognostic role for other cardiac pathologies such as heart failure. Elevated plasma TMAO was associated with more advanced left ventricular diastolic dysfunction and was associated with poor prognosis after adjustment for cardio-renal indices (Tang et al., 2015). In a recent meta-analysis, 10 articles (12 studies) involving 13,425 participants from 2014 to 2021 were considered to examine the prognostic value of TMAO in heart failure (Li et al., 2022). A high level of TMAO correlated with “major adverse cardiovascular events” and all-cause mortality in heart failure (RR: 1.28, 95% CI: 1.17, 1.39, p < 0.0001, random-effects model and RR: 1.35, 95% CI: 1.28, 1.42, p < 0.0001, random-effects model, respectively). Consistent results were obtained in all subgroups examined as well as in the sensitivity analysis.
When clinical studies such as those described above provide a sufficient level of evidence of the potential of a new biomarker, learned societies work to establish a consensus of interest in order to be able to propose recommendations on diagnostic or therapeutic approaches applicable in current practice. This is how this document from the European Society of Cardiology entitled “2021 ESC Guidelines on cardiovascular disease prevention in clinical practice” was written (Visseren et al., 2021). The recommendations are classified according to the level of evidence of effectiveness with a scale of I to III, for respectively, “I: Evidence that a given treatment or procedure is beneficial,” “II: Conflicting evidence and/or a divergence of opinion about the usefulness/efficacy,” “III: Evidence or general agreement that the given treatment or procedure is not useful/effective.” Depending on their class, they are then Class I, “recommended,” Class II, “may be considered,” or III “should not be used.” To date, the TMAO assay has not yet reached sufficient maturity to be taken into account in these recommendations and as TMAO exhibits complex genetic, dietary, and hormonal factor regulation, the use of TMAO as clinical biomarkers remains controversial by some key opinion leaders (Patanè, 2020). The latest ESC recommendation in 2021 proposes the use of SCORE-2. SCORE2—an updated algorithm adapted to identify people at higher risk of developing CVD across Europe (SCORE2 working group and ESC Cardiovascular risk collaboration, 2021).
It is therefore the experts in the field who will be able to assess the value of TMAO as a biomarker of CVD risk. It is now more than 10 years since this biomarker was discovered by an untargeted metabolomics study, the road is long and winding, and perhaps one or more other biomarker metabolites will have to be found to be used in combination with TMAO to reinforce the interest of this metabolomic approach to improve cardiovascular risk prediction.
3 How clinical metabolomics could help to better diagnose or prognose patients by fingerprinting analysis?
In the previous section, we have just seen the role of metabolomics to discover new biomarkers based on clinical studies. However, a single metabolite alone cannot often be a biomarker capable of predicting and/or diagnosing a pathology. A consensus in the community seems to be towards the use of a metabolic fingerprinting containing a panel of metabolites, more robust and bringing more weight in statistical models (Dias and Koal, 2016). In this second section, we have chosen to illustrate the interest that clinical metabolomics could have as a tool to measure the metabolic fingerprint in order to improve patient management. Through the examples selected in this section, we will emphasize the general workflow.
First of all, clinical metabolomics analysis is performed on a human biospecimen which has a care, research or other purpose, and which requires to take into account the ethical and legal aspects - the most important of which is permission (The Belmont Report, 2014). 1) In the United States, Institutional Review Boards (IRBs) are responsible for the regulatory oversight of research involving human research subjects (National Archives and Records Administration, 2018) 2) In other countries, an Independent Ethics Committees (IECs) may be responsible. They are typically directed by the Nuremberg Code, Declaration of Helsinki and guidelines established by the Council for the International Organization of Medical Sciences (CIOMS) and the principles of Good Clinical Practice (GCP).
If care is the goal of clinical metabolomics, then the biospecimen should be analyzed in a clinical chemistry laboratory, and the analyses will be performed according to the following overall protocol flow (Figure 2):
1. Samples collection, transport, aliquoting
2. Quenching and metabolites extraction
3. Sample drying and pellet resuspension
4. Chromatography separation (GC or LC)
5. Mass spectrometry or NMR (except step 2 to 4 for NMR)
6. Data processing, metabolites identification and data analysis
7. Validation and transmission of the results
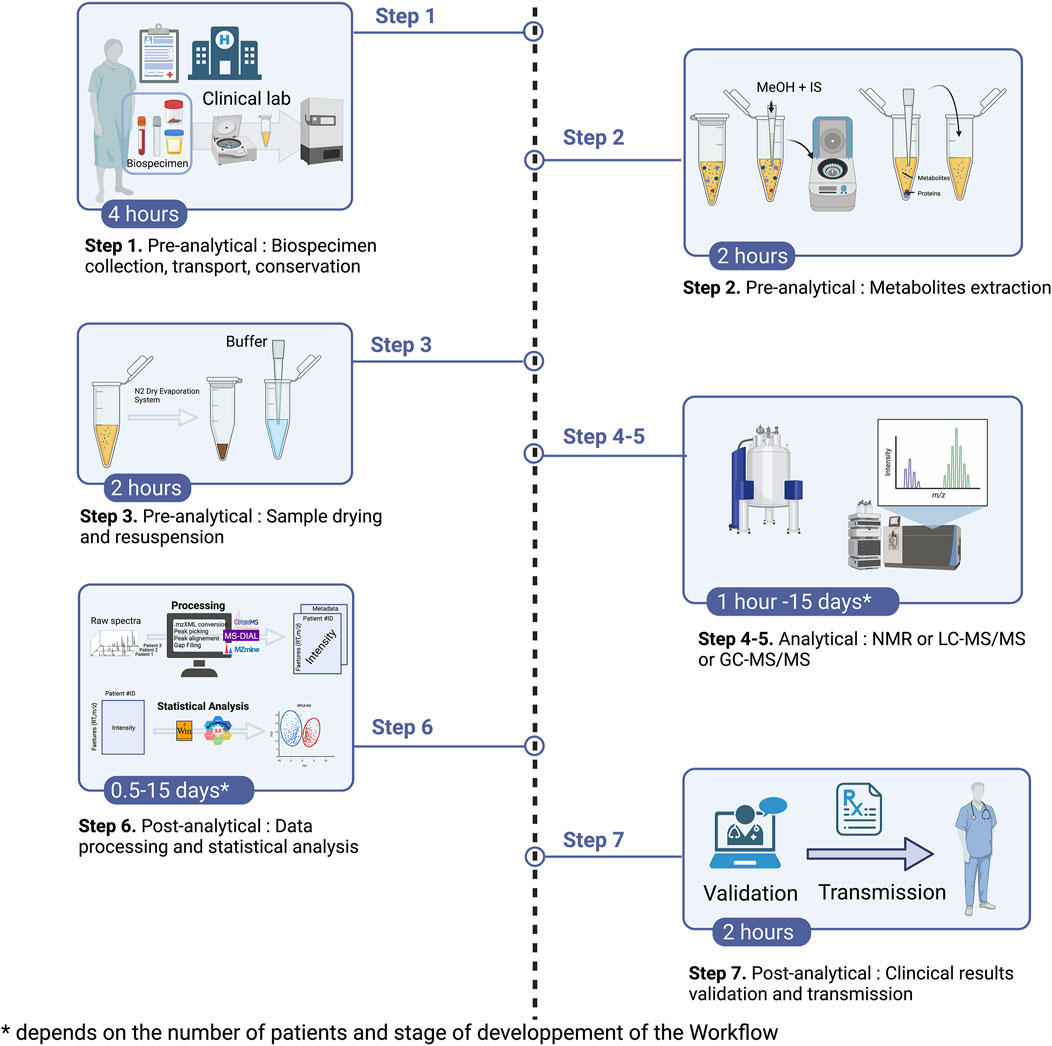
FIGURE 2. Clinical metabolomics’ overall protocol flow. Abbreviation: IS, internal standard; N2, Nitrogen.
First, the objective is to obtain a robust statistical model with a learning and validation cohort. Then the sample of a patient will be analyzed with this model and the result returned to the patient and/or clinician within 1–2 working days. Hence, the turn-around time for metabolomic fingerprinting should takes about 1–2 days. However, this turn-around time is only important in relation to the targeted pathology or the usefulness of the information for therapeutic management. The current turnaround time for clinical metabolomics analysis therefore excludes the possibility of using it, today, as emergency biomarkers. However, it remains compatible with all pathologies for which the vital prognosis will not be engaged in case of a delay of more than 2 days. This seems to be particularly well indicated, for example, for the diagnosis of rare diseases, cancers, or chronic diseases, for which diagnostic errancy can considerably delay patient management (over several years or even a decade). And this workflow will require great reproducibility potentially in both analytical, e.g., NMR or LC- and GC-MS and the automated data handling pipelines.
3.1 Example of metabolic fingerprint for COVID-19
In order to build a multi-metabolite biomarker model on the observed effects of COVID-19 disease, and as shown before, the sample set was divided into cohort for training and cohort for validation (Kimhofer et al., 2020). Samples were analyzed by a targeted metabolomics approach with both NMR using Bruker in vitro diagnostic research (IVDr) methods specifically on lipoprotein subclass analysis (n = 112), and MS for quantitative amino acid analysis (n = 35). In this particular case, it should be noted that all the metabolites used for the acquisition of the fingerprint were known and their dosage “validated” for in vitro diagnosis. The data were then analyzed using multivariate analysis such as principal component analysis and partial orthogonal least squares discriminant analysis (OPLS-DA). The training cohort consisted of SARS-CoV-2 positive (n = 7) and negative patients (n = 8), adjusted for age and sex. OPLS-DA model scores, including projections of validation sample scores (whole cohort n = 42), indicated large systematic metabolic differences in plasma of SARS-CoV-2-positive individuals compared with control patients and was used to construct an exceptionally strong (AUROC = 1) hybrid NMR-MS model that enabled detailed metabolic discrimination between the groups. The predictions of the validation samples from patients with the disease were 100% accurate, resulting in a sensitivity, specificity, and positive and negative predictive values of the model equal to 1, which is superior to currently used medical biology tests (PCR and/or serology). Although the objective of this work was not to propose a diagnostic test for SARS-CoV-2 infection, the models and data presented here show the possibility of developing such a test with other samples and a validation of the method.
3.2 Some examples of metabolic fingerprint for cancer diagnosis
In oncology, and more specifically in solid tumors, single biomarkers are used for cancer follow-up, but are not sufficiently effective for screening or diagnosis. In this context, metabolomics and the acquisition of metabolic fingerprints represent an interesting tool to detect early-stage cancers, i.e., before the appearance of clinical signs. In this section, some examples of application will be mentioned, considering that metabolomics approaches in oncology have been recently reviewed by Schmidt et al. (2021). Metabolic fingerprinting of two biological matrices has shown particular interest in the evaluation of different solid tumors. First of all, a recent literature review presents multiple examples of the use of metabolomics as a method for studying the urine of cancer patients (Dinges et al., 2019). In four studies using MS, comparing the metabolic profiles of urine from bladder cancer patients and healthy subjects, OPLS- DA and PLS-DA models with cross-validation (Pasikanti et al., 2010; Huang et al., 2011; Pasikanti et al., 2013) or a preliminary test cohort (Issaq et al., 2008) revealed excellent sensitivities and specificities (100% in both cases). In these examples, global non-targeted metabolomic analyses, on a large set of features (RT, and m/z) i.e., known and unknown molecules, were used to build a model. It should be noted that this type of modeling probably still does not seem applicable to the clinic today because the physician will not know exactly where does the metabolic signature (or information) in the fingerprint come from. On the other hand, all these studies demonstrate the high detection capacity of clinical metabolomics on urine samples for the diagnosis of urinary tract cancers, in which the measured biofluid is in direct contact with the tumor. Beyond the diagnostic value, metabolomics has proven to be a powerful method in the differentiation of bladder tumor stage and grade. For example, five MS studies have successfully differentiated tumor stage, grade, and recurrence status (Dinges et al., 2019). Urine is also an interesting fluid for other cancers such as ovarian cancer, where 90% of patients are diagnosed at an advanced metastatic stage (III or IV), and have a 5-year life expectancy of less than 30%. An NMR metabolomics study (n = 102) established an OPLS-DA model, validated by a permutation test (R2 = 0.77 and Q2 = 0.6). The validation test (n = 20) correctly predicted classification in 100% of cases.
And, finally, blood is the most commonly used biofluid for metabolomics studies. The metabolomics approach was applied to serum samples from patients with advanced metastatic breast cancer (n = 39) versus localized early disease (n = 46) (Jobard et al., 2014). NMR analysis followed by multivariate OPLS modeling was used in this study to define the metabolomic signature of advanced metastatic human breast cancer. The model able to distinguish between patients with metastatic and early breast cancer was obtained and validated with an external test group (n = 112 patients; sensitivity 89.8%, specificity 79.3%).
In all cases, it is important to pay attention to how the authors use such supervised multivariate statistics and how many patients have been recruited. The values of R2 and Q2 are essential for the proper evaluation and robustness of the model obtained. Too many studies come to hasty conclusions with predictability values (Q2) lower than 0.5, which would be less accurate than flipping a coin. And few studies provide receiver operating characteristics (ROC) curves with associated confidence intervals, the golden standard method in biomedical fields for performance assessment (Xia et al., 2013b).
This innovative approach would allow the identification of predictive biomarkers of therapeutic response, notably to immune checkpoint inhibitors. This question is currently addressed by Pr. Toffart in a prospective clinical trial conducted on 60 patients with non-small cell lung cancer (NSCLC) at the CHU Grenoble-Alpes (NCT04189679) (Sannicolo et al., 2021). Expected results would enable the identification of metabolite fingerprints rather than a single metabolite biomarker, to predict disease evolution under treatment.
4 Discussion
Untargeted metabolomics help to identify new biomarkers. Several examples in the literatures exists (Dang et al., 2009). Here, we have seen through the example of TMAO, the potential of metabolomics for the discovery of new biomarkers of pathology. It took more than 10 years, including the most limiting step of the field, i.e., the structural identification of metabolites and their role in pathophysiology. But beyond its role in biomarker discovery, we have seen that metabolomics could also allow the identification of a metabolic phenotype (metabotype) of different kind of pathologies, with the possibility of creating supervised models, that allow medical analysis laboratories to propose to clinicians the stratification of patients. Supervised machine learning algorithms, or multivariate regression models should be useful to build the predictive model for patient stratification. The challenge here for the hospital is as much in the recruitment of qualified personnel, as in the computer equipment necessary to achieve such a technological feat. Finally, there are still a number of challenges to be met in the field, such as the development of reference materials (Lippa et al., 2022), standards, methods and kits (medical device regulatory, FDA, CE/IVD), approved metabolomics software, reference laboratories, and external quality program will be necessary to improve the quality, robustness and validation of these approaches. Clinical metabolomics is totally in line with the evolution of medicine, a personalized medicine and would allow better upstream selection of patients responding to new therapies and diagnosis or prognosis of patients.
Author contributions
ALG conceptualized, wrote the first draft, ALG, CP, and BT review the final version.
Funding
ALG received grant support from the Fondation Université Grenoble Alpes and Fondation Air Liquide, FINOVI, ANR AMN, Association Vaincre la mucoviscidose and Gregory Lemarchal.
Conflict of interest
The authors declare that the research was conducted in the absence of any commercial or financial relationships that could be construed as a potential conflict of interest.
Publisher’s note
All claims expressed in this article are solely those of the authors and do not necessarily represent those of their affiliated organizations, or those of the publisher, the editors and the reviewers. Any product that may be evaluated in this article, or claim that may be made by its manufacturer, is not guaranteed or endorsed by the publisher.
References
Alseekh, S., Aharoni, A., Brotman, Y., Contrepois, K., D’Auria, J., Ewald, J., et al. (2021). Mass spectrometry-based metabolomics: a guide for annotation, quantification and best reporting practices. Nat. Methods 18, 747–756. doi:10.1038/s41592-021-01197-1
Bar, N., Korem, T., Weissbrod, O., Zeevi, D., Rothschild, D., Leviatan, S., et al. (2020). A reference map of potential determinants for the human serum metabolome. Nature 588, 135–140. doi:10.1038/s41586-020-2896-2
Bouatra, S., Aziat, F., Mandal, R., Guo, A. C., Wilson, M. R., Knox, C., et al. (2013). The human urine metabolome. PLoS One 8, e73076. doi:10.1371/journal.pone.0073076
Ceglarek, U., Leichtle, A., Brügel, M., Kortz, L., Brauer, R., Bresler, K., et al. (2009). Challenges and developments in tandem mass spectrometry based clinical metabolomics. Mol. Cell Endocrinol. 301, 266–271. doi:10.1016/j.mce.2008.10.013
Chang, K. H., Cheng, M. L., Tang, H. Y., Huang, C. Y., Wu, H. C., and Chen, C. M. (2022). Alterations of sphingolipid and phospholipid pathways and ornithine level in the plasma as biomarkers of Parkinson’s disease. Cells 11, 395. doi:10.3390/CELLS11030395
Dang, L., White, D. W., Gross, S., Bennett, B. D., Bittinger, M. A., Driggers, E. M., et al. (200946272009). Cancer-associated IDH1 mutations produce 2-hydroxyglutarate. Nat 462, 739–744. doi:10.1038/nature08617
Dias, D. A., and Koal, T. (2016). Progress in metabolomics standardisation and its significance in future clinical laboratory medicine. EJIFCC 27, 331–343.
Dinges, S. S., Hohm, A., Vandergrift, L. A., Nowak, J., Habbel, P., Kaltashov, I. A., et al. (2019). Cancer metabolomic markers in urine: Evidence, techniques and recommendations. Nat. Rev. Urol. 16, 339–362. doi:10.1038/s41585-019-0185-3
Heianza, Y., Ma, W., DiDonato, J. A., Sun, Q., Rimm, E. B., Hu, F. B., et al. (2020). Long-term changes in gut microbial metabolite trimethylamine N-oxide and coronary heart disease risk. J. Am. Coll. Cardiol. 75, 763–772. doi:10.1016/j.jacc.2019.11.060
Huang, Z., Lin, L., Gao, Y., Chen, Y., Yan, X., Xing, J., et al. (2011). Bladder cancer determination via two urinary metabolites: A biomarker pattern approach. Mol. Cell Proteomics 10, M111.007922. doi:10.1074/mcp.M111.007922
Issaq, H. J., Nativ, O., Waybright, T., Luke, B., Veenstra, T. D., Issaq, E. J., et al. (2008). Detection of bladder cancer in human urine by metabolomic profiling using high performance liquid chromatography/mass spectrometry. J. Urol. 179, 2422–2426. doi:10.1016/J.JURO.2008.01.084
Jobard, E., Pontoizeau, C., Blaise, B. J., Bachelot, T., Elena-Herrmann, B., and Trédan, O. (2014). A serum nuclear magnetic resonance-based metabolomic signature of advanced metastatic human breast cancer. Cancer Lett. 343, 33–41. doi:10.1016/J.CANLET.2013.09.011
Karu, N., Deng, L., Slae, M., Guo, A. C., Sajed, T., Huynh, H., et al. (2018). A review on human fecal metabolomics: Methods, applications and the human fecal metabolome database. Anal. Chim. Acta 1030, 1–24. doi:10.1016/j.aca.2018.05.031
Kimhofer, T., Lodge, S., Whiley, L., Gray, N., Loo, R. L., Lawler, N. G., et al. (2020). Integrative modeling of quantitative plasma lipoprotein, metabolic, and amino acid data reveals a multiorgan pathological signature of SARS-CoV-2 infection. J. Proteome Res. 19, 4442–4452. doi:10.1021/acs.jproteome.0c00519
Letertre, M. P. M., Giraudeau, P., De Tullio, P., Rudaz, S., Choi, Y. H., and Luchinat, C. (2021). Nuclear magnetic resonance spectroscopy in clinical metabolomics and personalized medicine: Current challenges and perspectives. Front. Mol. Biosci. 8, 698337. doi:10.3389/fmolb.2021.698337
Li, X., Fan, Z., Cui, J., Li, D., Lu, J., Cui, X., et al. (2022). Trimethylamine N-oxide in heart failure: A meta-analysis of prognostic value. Front. Cardiovasc Med. 9, 817396. doi:10.3389/FCVM.2022.817396
Lippa, K. A., Aristizabal-Henao, J. J., Beger, R. D., Bowden, J. A., Broeckling, C., Beecher, C., et al. (2022). Reference materials for MS-based untargeted metabolomics and lipidomics: a review by the metabolomics quality assurance and quality control consortium (mQACC). Metabolomics 18, 24. doi:10.1007/S11306-021-01848-6
Long, T., Hicks, M., Yu, H. C., Biggs, W. H., Kirkness, E. F., Menni, C., et al. (2017). Whole-genome sequencing identifies common-to-rare variants associated with human blood metabolites. Nat. Genet. 49, 568–578. doi:10.1038/ng.3809
Lotta, L. A., Pietzner, M., Stewart, I. D., Wittemans, L. B. L., Li, C., Bonelli, R., et al. (2021). A cross-platform approach identifies genetic regulators of human metabolism and health. Nat. Genet. 53, 54–64. doi:10.1038/s41588-020-00751-5
National Archives and Records Administration (2018). Code of federal regulations. 45 CFR Part 46 (2018-07-19) Protection of Human Subjects. Available at: https://www.ecfr.gov/on/2018-07-19/title-45/subtitle-A/subchapter-A/part-46#sp45.1.46.a (accessed January 11, 2023).
Nicholson, J. K., and Lindon, J. C. (2008). Systems biology: Metabonomics. Nature 455, 1054–1056. doi:10.1038/4551054a
Pasikanti, K. K., Esuvaranathan, K., Ho, P. C., Mahendran, R., Kamaraj, R., Wu, Q. H., et al. (2010). Noninvasive urinary metabonomic diagnosis of human bladder cancer. J. Proteome Res. 9, 2988–2995. doi:10.1021/pr901173v
Pasikanti, K. K., Esuvaranathan, K., Hong, Y., Ho, P. C., Mahendran, R., Raman Nee Mani, L., et al. (2013). Urinary metabotyping of bladder cancer using two-dimensional gas chromatography time-of-flight mass spectrometry. J. Proteome Res. 12, 3865–3873. doi:10.1021/pr4000448
Patanè, S. (2020). Long-term changes in gut microbial metabolite TMAO, CHD risk, and its complex regulatory network. J. Am. Coll. Cardiol. 75, 3100–3101. doi:10.1016/j.jacc.2020.03.077
Playdon, M. C., Sampson, J. N., Cross, A. J., Sinha, R., Guertin, K. A., Moy, K. A., et al. (2016). Comparing metabolite profiles of habitual diet in serum and urine. Am. J. Clin. Nutr. 104, 776–789. doi:10.3945/ajcn.116.135301
Psychogios, N., Hau, D. D., Peng, J., Guo, A. C., Mandal, R., Bouatra, S., et al. (2011). The human serum metabolome. PLoS One 6, e16957. doi:10.1371/journal.pone.0016957
Roberts, L. D., Souza, A. L., Gerszten, R. E., and Clish, C. B. (2012). Targeted metabolomics. Curr. Protoc. Mol. Biol. Chapter 30, Unit 30.2.1-24. doi:10.1002/0471142727.mb3002s98
Roux, A., Xu, Y., Heilier, J. F., Olivier, M. F., Ezan, E., Tabet, J. C., et al. (2012). Annotation of the human adult urinary metabolome and metabolite identification using ultra high performance liquid chromatography coupled to a linear quadrupole ion trap-orbitrap mass spectrometer. Anal. Chem. 84, 6429–6437. doi:10.1021/ac300829f
Sannicolo, S., Giaj Levra, M., Le Gouellec, A., Aspord, C., Boccard, J., Chaperot, L., et al. (2021). Identification of a predictive metabolic signature of response to immune checkpoint inhibitors in non-small cell lung cancer: METABO-ICI clinical study protocol. Respir. Med. Res. 80, 100845. doi:10.1016/J.RESMER.2021.100845
Schmidt, D. R., Patel, R., Kirsch, D. G., Lewis, C. A., Vander Heiden, M. G., and Locasale, J. W. (2021). Metabolomics in cancer research and emerging applications in clinical oncology. CA Cancer J. Clin. 71, 333–358. doi:10.3322/caac.21670
Schrimpe-Rutledge, A. C., Codreanu, S. G., Sherrod, S. D., and McLean, J. A. (2016). Untargeted metabolomics strategies—challenges and emerging directions. J. Am. Soc. Mass Spectrom. 27, 1897–1905. doi:10.1007/s13361-016-1469-y
SCORE2 working group and ESC Cardiovascular risk collaboration (2021). SCORE2 risk prediction algorithms: New models to estimate 10-year risk of cardiovascular disease in Europe. Eur. Heart J. 42, 2439–2454. doi:10.1093/eurheartj/ehab309
Shin, S. Y., Fauman, E. B., Petersen, A. K., Krumsiek, J., Santos, R., Huang, J., et al. (2014). An atlas of genetic influences on human blood metabolites. Nat. Genet. 46, 543–550. doi:10.1038/NG.2982
Tang, W. H. W., Wang, Z., Levison, B. S., Koeth, R. A., Britt, E. B., Fu, X., et al. (2013). Intestinal microbial metabolism of phosphatidylcholine and cardiovascular risk. N. Engl. J. Med. 368, 1575–1584. doi:10.1056/NEJMOA1109400
Tang, W. H. W., Wang, Z., Shrestha, K., Borowski, A. G., Wu, Y., Troughton, R. W., et al. (2015). Intestinal microbiota-dependent phosphatidylcholine metabolites, diastolic dysfunction, and adverse clinical outcomes in chronic systolic heart failure. J. Card. Fail 21, 91–96. doi:10.1016/j.cardfail.2014.11.006
The Belmont Report, (2014). Ethical principles and guidelines for the protection of human subjects of research. J. Am. Coll. Dent. 81, 4–13. doi:10.1017/cbo9780511550089.028
Visseren, F. L. J., MacH, F., Smulders, Y. M., Carballo, D., Koskinas, K. C., Bäck, M., et al. (2021). 2021 ESC Guidelines on cardiovascular disease prevention in clinical practice. Eur. Heart J. 42, 3227–3337. doi:10.1093/eurheartj/ehab484
Wang, Z., Klipfell, E., Bennett, B. J., Koeth, R., Levison, B. S., Dugar, B., et al. (2011). Gut flora metabolism of phosphatidylcholine promotes cardiovascular disease. Nature 472, 57–63. doi:10.1038/nature09922
Wheelock, C. E., Goss, V. M., Balgoma, D., Nicholas, B., Brandsma, J., Skipp, P. J., et al. (2013). Application of ’omics technologies to biomarker discovery in inflammatory lung diseases. Eur. Respir. J. 42, 802–825. doi:10.1183/09031936.00078812
Wikoff, W. R., Anfora, A. T., Liu, J., Schultz, P. G., Lesley, S. A., Peters, E. C., et al. (2009). Metabolomics analysis reveals large effects of gut microflora on mammalian blood metabolites. Proc. Natl. Acad. Sci. U. S. A. 106, 3698–3703. doi:10.1073/pnas.0812874106
Wishart, D. S., Lewis, M. J., Morrissey, J. A., Flegel, M. D., Jeroncic, K., Xiong, Y., et al. (2008). The human cerebrospinal fluid metabolome. J. Chromatogr. B Anal. Technol. Biomed. Life Sci. 871, 164–173. doi:10.1016/j.jchromb.2008.05.001
Wishart, D. S., Guo, A. C., Oler, E., Wang, F., Anjum, A., Peters, H., et al. (2022). HMDB 5.0: The human metabolome database for 2022. Nucleic Acids Res. 50, D622–D631. doi:10.1093/nar/gkab1062
Wishart, D. S. (2016). Emerging applications of metabolomics in drug discovery and precision medicine. Nat. Rev. Drug Discov. 15, 473–484. doi:10.1038/nrd.2016.32
Wishart, D. S. (2019). Metabolomics for investigating physiological and pathophysiological processes. Physiol. Rev. 99, 1819–1875. doi:10.1152/physrev.00035.2018
Xia, J., Broadhurst, D. I., Wilson, M., and Wishart, D. S. (2013a). Translational biomarker discovery in clinical metabolomics: An introductory tutorial. Metabolomics 9, 280–299. doi:10.1007/s11306-012-0482-9
Xia, J., Broadhurst, D. I., Wilson, M., and Wishart, D. S. (2013b). Translational biomarker discovery in clinical metabolomics: an introductory tutorial. Metabolomics 9, 280–299. doi:10.1007/s11306-012-0482-9
Xu, T., Holzapfel, C., Dong, X., Bader, E., Yu, Z., Prehn, C., et al. (2013). Effects of smoking and smoking cessation on human serum metabolite profile: Results from the KORA cohort study. BMC Med. 11, 60. doi:10.1186/1741-7015-11-60
Yousri, N. A., Kastenmüller, G., Gieger, C., Shin, S. Y., Erte, I., Menni, C., et al. (2014). Long term conservation of human metabolic phenotypes and link to heritability. Metabolomics 10, 1005–1017. doi:10.1007/s11306-014-0629-y
Keywords: biochemistry, biomarker discovery, mass spectrometry, clinical laboratory, clinical metabolomics, NMR
Citation: Le Gouellec A, Plazy C and Toussaint B (2023) What clinical metabolomics will bring to the medicine of tomorrow. Front. Anal. Sci. 3:1142606. doi: 10.3389/frans.2023.1142606
Received: 11 January 2023; Accepted: 14 February 2023;
Published: 24 February 2023.
Edited by:
Sophie Ayciriex, Université Claude Bernard Lyon 1, FranceReviewed by:
Reza M. Salek, Bruker, GermanyCopyright © 2023 Le Gouellec, Plazy and Toussaint. This is an open-access article distributed under the terms of the Creative Commons Attribution License (CC BY). The use, distribution or reproduction in other forums is permitted, provided the original author(s) and the copyright owner(s) are credited and that the original publication in this journal is cited, in accordance with accepted academic practice. No use, distribution or reproduction is permitted which does not comply with these terms.
*Correspondence: Audrey Le Gouellec, YWxlZ291ZWxsZWNAY2h1LWdyZW5vYmxlLmZy