- 1School of Pharmaceutical Sciences, University of Geneva, Geneva, Switzerland
- 2Institute of Pharmaceutical Sciences of Western Switzerland, University of Geneva, Geneva, Switzerland
Unlike other systems such as plants, microorganisms or fungi, human cells are not proficient in eliciting the production of defense compounds in response to external stresses and threats. Human metabolism is essentially based on a set of primary metabolites that participate in the various regulatory events of cells and tissues. The challenge is therefore to maintain homeostasis and allow the survival of the individual through the modulation of existing endogenous metabolic pathways with a relatively stable set of ubiquitous compounds. Since these complex regulatory phenomena are potentially subject to multiple influences, assessing their overall variability, as achieved by most conventional approaches, is not sufficiently informative. The experimental evaluation of several factors acting simultaneously on the metabolome is paramount. Because metabolomics involves the characterization of multivariate metabolic phenotypes, such a methodology requires specific data analysis tools to fully exploit the relevant information considering the different factors, as well as their respective impact on metabolite levels. The investigation of high-dimensional multifactorial data in metabolomics opens new challenges and requires the development of innovative experimental strategies involving structured designs of experiments to assess cause-effect associations and offer deeper insight into relevant biological information. In the future, key outputs should not only consider lists of metabolites, but also include their specific variation related to each effect that can be identified and/or quantified, thus allowing accurate biochemical and functional relationships to be highlighted.
Exploring metabolic modulations
The recent development of high-throughput Omics technologies has led to the collection of large numbers of signals to characterize the complexity of a sample. Among these innovative approaches, metabolomics is now recognized as an efficient tool for a better understanding of physiological mechanisms that allow an organism to survive, grow, and develop. The maintenance of homeostasis is at the basis of living beings’ survival by controlling the biochemical events necessary for the proper functioning of vital processes. While other organisms such as plants, fungi or microbes may produce secondary defense metabolites, the modulation and adaptation of existing endogenous metabolic pathways is the only mechanism for human (and more broadly mammalian) cells to respond to external stressors. Measuring metabolites as substrates and products of enzyme-catalyzed reactions needed for proper cell functioning and maintenance constitutes therefore a very efficient strategy to characterize its biochemical state (Wishart, 2019). Besides the challenges of measuring the metabolome leading to multiple sources of variations which will not be discussed here, e.g., sample storage and analytical variability, (Blaise et al., 2021), exploring biological variability constitutes also an important question.
In metabolomics, multivariate models are mainly used to rank the variables according to their contribution to the model components (Boccard and Rudaz, 2014). The latter are used to explore the data, and detect potential trends or relevant groupings of observations. One of the first major challenges in achieving this goal is that, even after adapted pretreatment, metabolomic datasets are often characterized by high dimensionality, i.e., the number of variables (peaks, chemical shifts, ions, etc.) can be very large, while the number of observations remains usually low. Unsupervised methods assume that variability is related to biological information. However, the intrinsic complexity of the phenomena under investigation is often substantial and this type of approach may thus be of limited use. Supervised modeling can use the knowledge of the experimental groups to optimize their discrimination. When prediction accuracy is satisfactory and sufficiently robust, it is then possible to assign new observations to one class or another (e.g., healthy vs. cancer tissue), but in most cases, the model is not an end in itself and the prediction of individuals is only one of the outputs.
For the scientist, both approaches allow subsets of metabolites associated with different situations to be highlighted, e.g., a physiological phenotypic signature or a pathological condition. However, the observable levels of metabolites forming biochemical fingerprints are intrinsically influenced by many endogenous processes and responses to external influences or stresses, making the estimation of global biological variability of little relevance in most cases. When a massive alteration of a specific pathway occurs, the variability observed on a subset of variables may be highlighted and lead to a relevant biological interpretation. However, the occurrence of mixed effects will largely depend on the biological matrix under investigation. While variability can be limited by analyzing specific matrices and/or biological compartments (e.g., specific tissue or cell types), the observed abundance of a primary metabolite in a biological fluid flowing through organs such as blood results from a combination of many contributions. As a consequence, the specificity of such metabolic modulations remains difficult to evaluate and the reductionist approach of assessing one source of variability at a time will ignore this complexity.
Disentangling mixed effects
As cells reside in specific local conditions, their biochemical processes and functions are influenced by their close biological surroundings. A first step in the direction of disentangling these multiple influences is to solve or simplify the spatial context under investigation. For that purpose, specific in vitro systems involving particular genetically characterized cell types in a controlled environment may greatly help to study metabolic regulations in the context of a given local tissue neighborhood. Many efforts have been made to improve the translation of results obtained on in vitro systems to in vivo situations. From the perspective of the experimental strategy conception, design of experiments constitutes an efficient methodology to systematically decompose and quantify cause-and-effect relationships between factors and outputs (Boccard and Rudaz, 2020). As such, it offers practical solutions to disentangle the intrinsic confusion arising from the measurement of endogenous metabolites, but adapted methods are needed to account for the high-dimensional nature of datasets involving potential interactions between multiple compounds. When an experimental design involving several factors is implemented, it is then possible to evaluate the contribution of each of the variables to the different effects (main effects and possible interactions) and rank them as closely as possible to real conditions. Even more interestingly, it is possible to decompose the variability of each metabolite according to the effects involved using a cumulative contribution expressed as a percentage of its total variance (Gonzalez-Ruiz et al., 2017). In this way, the specific proportion of the variability observed for a given metabolite can be associated with each effect. Ranking the metabolites according to the specific proportion of their observed variability for a given effect is mandatory for a more efficient investigation of complex biological modulations (Gonzalez-Ruiz et al., 2019). This output is essential when one wishes to go beyond a global observation of variability to understand specific modulations with respect to homeostasis maintenance, e.g., by comparing a given combination of factor levels to the reference control. Furthermore, the residual part that cannot be explained by the experimental factors can also be valuable to assess possible external contributions to the biological variability. In the perspective of biomarker detection, this part of variability can also constitute a relevant criterion to validate, or on the contrary invalidate, the choice of a subset of metabolites. Indeed, if the natural variability of the levels of a metabolite is too important, e.g., due to inter-individual differences, its relevance as a biomarker could be limited. In vitro experiments and genetically well-characterized organisms are often used to limit the biological variability, and the possibility to assign experimental parameters is particularly well adapted for the use of experimental design. However, it is not always possible or desirable to use such controlled systems to investigate a biological question, and cohort studies constitute an interesting alternative. In this case, additional sources of variability linked to individual characteristics may further increase the complexity of metabolic modulations and a longitudinal follow-up is often desirable (Gagnebin et al., 2020). Taking into account the pairings between measurements, the metabolic background of each individual submitted to multiple influences constitutes a reference and the comparison with this basal situation makes it possible to mitigate its specificities. The choice of a proper timing for sampling is then crucial to provide a reliable picture of the studied phenomenon and offer kinetic insights into metabolic processes. Indeed, the apparent lack of modulations observed at some sampling points may be due to delayed or faster metabolic alterations than expected in the initial experimental protocol. Thus, sample collection should be properly scheduled to allow modeling of the temporal evolution based on relevant time points.
Making mechanistic hypotheses more reliable
Reducing overall biological variability by computing ratios between pairs of metabolite levels as biomarkers represent another computational approach that will certainly continue to gain importance for describing complex biochemical homeostatic phenomena. It may constitute an internal normalization by reporting intensities in a relative way, and modulations of ratios between metabolites may indeed highlight patterns in metabolic pathways that are associated with a given condition or phenotype. While modern high-throughput analytical technologies have massively increased the possibilities to evaluate metabolite relationships, it does not seem relevant to explore all combinations in a systematic way. Knowledge-driven strategies will be of great help, as meaningful metabolite ratios might be efficiently selected on the basis of the proportion of the different effects included in the experimental design, as well as the reaction paths between metabolites involved in multiple interconnected pathways. Such an approach will better contextualize metabolomic results by highlighting biochemically relevant associations between metabolite ratios, thus offering more consistent biological hypotheses (Boccard et al., 2021).
By connecting substrates and products of the same reaction, metabolic networks modeling constitutes another relevant methodology to link biochemical events defining the metabolism with mechanistic explanations (Amara et al., 2022). Because specific phenotypic signatures may spread over several pathways, the evaluation of the different reaction paths between biomarkers will certainly provide valuable information to better understand the phenomena directing the maintenance of homeostasis. For that purpose, path search strategies, such as the lightest path approach, can incorporate biochemical rules to highlight the most relevant metabolic routes (Frainay and Jourdan, 2017). Better investigating multifactorial modulations and mapping them on spatially resolved metabolic networks will definitely help for an improved understanding of homeostasis maintenance. A scheme summarizing the different steps required for an in-depth evaluation of the different sources of biological variability and their interpretation is proposed in Figure 1.
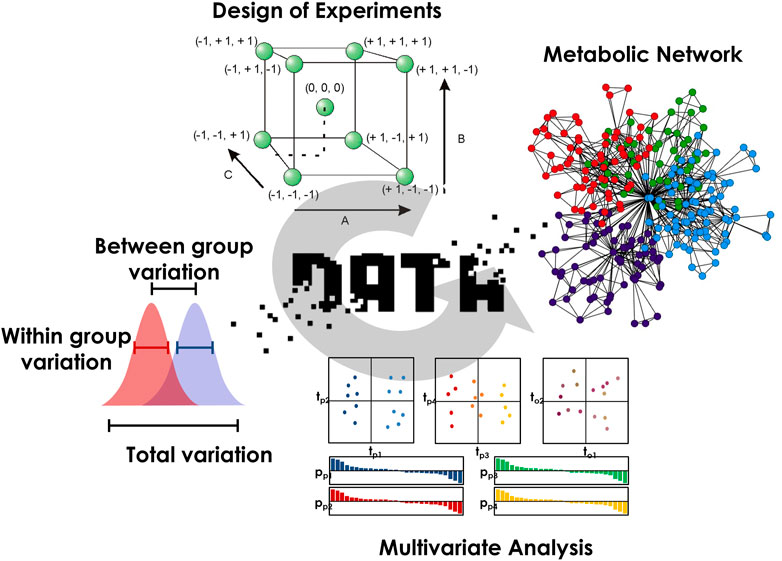
FIGURE 1. The different steps required for an in-depth evaluation of the different sources of variability and their biological interpretation.
To summarize, metabolomics is a rapidly evolving research field currently moving from standard biomarker discovery to a more mechanistic description of biochemical processes leading to specific metabolic phenotypes. The study of multifactorial metabolomic data raises new challenges and calls for the elaboration of novel experimental approaches based on structured designs of experiments, when possible. The investigation of the complex datasets generated will need adapted modelling methods combining variance decomposition and multivariate modelling [e.g., ASCA, AMOPLS (Boccard and Rudaz, 2016)] to provide new insights into cause-and-effect associations. Biochemical and functional relationships need to be more efficiently highlighted using metabolic networks to help selecting relevant metabolite ratios, thus facilitating interpretation. Taken together, these knowledge-driven strategies will hopefully allow a better understanding of the regulations governing inter-connected endogenous biological processes responsible for homeostasis and health.
Data availability statement
The original contributions presented in the study are included in the article/Supplementary Material, further inquiries can be directed to the corresponding author.
Author contributions
JB and SR wrote the manuscript.
Conflict of interest
The authors declare that the research was conducted in the absence of any commercial or financial relationships that could be construed as a potential conflict of interest.
Publisher’s note
All claims expressed in this article are solely those of the authors and do not necessarily represent those of their affiliated organizations, or those of the publisher, the editors and the reviewers. Any product that may be evaluated in this article, or claim that may be made by its manufacturer, is not guaranteed or endorsed by the publisher.
References
Amara, A., Frainay, C., Jourdan, F., Naake, T., Neumann, S., Novoa-Del-Toro, E. M., et al. Networks and graphs discovery in metabolomics data analysis and interpretation. Front. Mol. Biosci. (2022) 9:841373. doi:10.3389/fmolb.2022.841373
Blaise, B. J., Correia, G. D. S., Haggart, G. A., Surowiec, I., Sands, C., Lewis, M. R., et al. Statistical analysis in metabolic phenotyping. Nat. Protoc. (2021) 16:4299–4326. doi:10.1038/s41596-021-00579-1
Boccard, J., and Rudaz, S. Analysis of metabolomics data—a chemometrics perspective. In: S. BROWN, R. TAULER, and B. WALCZAK, editors. Comprehensive chemometrics. Second Edition. Oxford: Elsevier (2020).
Boccard, J., and Rudaz, S. Exploring Omics data from designed experiments using analysis of variance multiblock Orthogonal Partial Least Squares. Anal. Chim. Acta (2016) 920:18–28. doi:10.1016/j.aca.2016.03.042
Boccard, J., and Rudaz, S. Harnessing the complexity of metabolomic data with chemometrics. J. Chemom. (2014) 28:1–9. doi:10.1002/cem.2567
Boccard, J., Schvartz, D., Codesido, S., Hanafi, M., Gagnebin, Y., Ponte, B., et al. Gaining insights into metabolic networks using chemometrics and bioinformatics: Chronic kidney disease as a clinical model. Front. Mol. Biosci. (2021) 8:682559. doi:10.3389/fmolb.2021.682559
Frainay, C., and Jourdan, F. Computational methods to identify metabolic sub-networks based on metabolomic profiles. Briefings Bioinforma. (2017) 18:43–56. doi:10.1093/bib/bbv115
Gagnebin, Y., Pezzatti, J., Lescuyer, P., Boccard, J., Ponte, B., and Rudaz, S. Combining the advantages of multilevel and orthogonal partial least squares data analysis for longitudinal metabolomics: Application to kidney transplantation. Anal. Chim. Acta (2020) 1099:26–38. doi:10.1016/j.aca.2019.11.050
Gonzalez-Ruiz, V., Pezzatti, J., Roux, A., Stoppini, L., Boccard, J., and Rudaz, S. Unravelling the effects of multiple experimental factors in metabolomics, analysis of human neural cells with hydrophilic interaction liquid chromatography hyphenated to high resolution mass spectrometry. J. Chromatogr. A (2017) 1527:53–60. doi:10.1016/j.chroma.2017.10.055
Gonzalez-Ruiz, V., Schvartz, D., Sandstrom, J., Pezzatti, J., Jeanneret, F., Tonoli, D., et al. An integrative multi-omics workflow to address multifactorial toxicology experiments. Metabolites (2019) 9:79. doi:10.3390/metabo9040079
Keywords: metabolomics, design of experiments (DOE), multifactorial datasets, variability decomposition, metabolic networks
Citation: Boccard J and Rudaz S (2023) Why do we need to go beyond overall biological variability assessment in metabolomics?. Front. Anal. Sci. 3:1112390. doi: 10.3389/frans.2023.1112390
Received: 30 November 2022; Accepted: 02 February 2023;
Published: 13 February 2023.
Edited by:
Sophie Ayciriex, Université Claude Bernard Lyon 1, FranceReviewed by:
Nils Hoffmann, Bielefeld University, GermanyNathalie Poupin, Institut National de la Recherche Agronomique de Toulouse, France
Copyright © 2023 Boccard and Rudaz. This is an open-access article distributed under the terms of the Creative Commons Attribution License (CC BY). The use, distribution or reproduction in other forums is permitted, provided the original author(s) and the copyright owner(s) are credited and that the original publication in this journal is cited, in accordance with accepted academic practice. No use, distribution or reproduction is permitted which does not comply with these terms.
*Correspondence: Julien Boccard, anVsaWVuLmJvY2NhcmRAdW5pZ2UuY2g=