- 1Department of Didactics and Educational Organisation, University of Seville, Seville, Spain
- 2Department of Economics and Business Sciences, Private Technical University of Loja, Loja, Ecuador
Artificial Intelligence in Education (AIEd) offers advanced tools that can personalize learning experiences and enhance teachers’ research capabilities. This paper explores the beliefs of 425 university teachers regarding the integration of generative AI in educational settings, utilizing the UTAUT2 model to predict their acceptance and usage patterns through the Partial Least Squares (PLS) method. The findings indicate that performance expectations, effort expectancy, social influence, facilitating conditions, and hedonic motivation all positively impact the intention and behavior related to the use of AIEd. Notably, the study reveals that teachers with constructivist pedagogical beliefs are more inclined to adopt AIEd, underscoring the significance of considering teachers’ attitudes and motivations for the effective integration of technology in education. This research provides valuable insights into the factors influencing teachers’ decisions to embrace AIEd, thereby contributing to a deeper understanding of technology integration in educational contexts. Moreover, the study’s results emphasize the critical role of teachers’ pedagogical orientations in their acceptance and utilization of AI technologies. Constructivist educators, who emphasize student-centered learning and active engagement, are shown to be more receptive to incorporating AIEd tools compared to their transmissive counterparts, who focus on direct instruction and information dissemination. This distinction highlights the need for tailored professional development programs that address the specific beliefs and needs of different teaching philosophies. Furthermore, the study’s comprehensive approach, considering various dimensions of the UTAUT2 model, offers a robust framework for analyzing technology acceptance in education.
1 Introduction
Possessing a belief entail possessing a perceived truth, which subsequently dictates the subject’s actions and behavior in both advantageous and disadvantageous manners. The rapid pace of cultural, social, and technological transformation necessitates a reevaluation of personal beliefs, much like values, within the dynamic social reality we inhabit.
Beliefs, in their most expansive sense, wield transformative power over our cognition, opinions, competencies, and attitudes. They extend beyond mere personal constructs and are significantly influenced by the broader cultural milieu. As Jiménez (2019, p. 16) aptly articulates, “Beliefs are general ideas that we have about phenomena; they help us interpret, predict and control the events that occur and thus make decisions.” This conceptualization of beliefs is paramount in understanding their role in shaping our actions and behaviors, particularly for educators who must recognize that their pedagogical choices are profoundly influenced by their beliefs.
For a considerable duration (Brody and Day, 1993), it has been posited that in the realm of teaching, the significance and value that educators ascribe to their beliefs delineate the meaning of education and its various components (such as content, technology, didactic strategies, evaluation methods, etc.), and consequently influence their conduct in the educational process, their perception of it, and the nature of their interactions with students. Clark and Peterson (1986), pioneers in this research domain, assert that teachers’ cognitive frameworks impact their perceptions of students, their instructional planning, and their classroom behavior.
Montanares and Junod (2018, p. 94) further propose that “knowledge of teachers’ beliefs is important to the extent that it allows teachers a greater degree of awareness, responsibility and control in the choices of epistemological teaching models.”
The formation of teachers’ beliefs is influenced by a diverse array of variables, encompassing their professional experiences as educators and researchers, the nature of their training, their pedagogical education, ideological inclinations, interactions with colleagues, and the characteristics and ideology of the educational institution and community in which they operate (Arancibia-Herrera et al., 2024; Palacios-Rodríguez et al., 2023).
From the perspective of didactic research, investigations into beliefs have pursued various lines and orientations, including teaching strategies (Montanares and Junod, 2018; Cabero-Almenara et al., 2024), instructional practices (Rodríguez-Sosa and Solis-Manrique, 2017; Al-Adwan & Al-Debei, 2024), discipline-specific pedagogy (Casimiro, 2022), inclusive education (Friesen et al., 2023), the pedagogical utility of questioning (Mante-Estacioa and Tupas, 2023), STEM education (Alghamdi, 2023), and the role of emotions in professional teaching practices (Vettori et al., 2022).
A significant research trajectory has also examined beliefs about educational possibilities and the integration of Information and Communication Technologies (ICT). This research has evolved to focus on specific analyses, such as perceptions regarding the TPACK model’s dimensions in ICT integration (Ifinedo et al., 2020), the efficacy of video in language instruction (Waluyo and Apridayani, 2021), and attitudes toward incorporating ICT into educational practice (Li et al., 2019; Hoareau et al., 2021). Additionally, studies have explored how beliefs about technological competencies influence ICT integration (Cheng et al., 2022), the potential of ICT to support students with dyslexia (Bice and Tang, 2022), its effectiveness in lower education levels (Hoareau et al., 2021), and its applicability across various disciplines (García et al., 2022).
To summarize the significance of teachers’ beliefs concerning ICT application, Tondeur et al. (2017) conducted a meta-analysis and concluded: (1) a bidirectional relationship exists between pedagogical beliefs and ICT usage, (2) beliefs often act as perceived barriers, (3) specific beliefs are linked to specific types of ICT usage, (4) beliefs play a crucial role in professional development, and (5) the school context significantly influences beliefs about ICT.
Related to the theme of beliefs is the work on the “degree of acceptance of technologies” by teachers. Various models have been employed to analyze technology acceptance among potential users. The initial model, the Technology Acceptance Model (TAM) formulated by Davis (1989), posits that the intention to use technology is influenced by two primary dimensions: perceived usefulness and perceived ease of use, which in turn affect attitudes toward ICT, determining intentions to use and actual usage. This model has been utilized to analyze different technologies, such as virtual training (Rodríguez-Sabiote et al., 2023), augmented reality (Barroso et al., 2018), and immersive reality (Cabero et al., 2023; Palacios-Rodríguez et al., 2024a).
In contrast to the Technology Acceptance Model (TAM), Venkatesh et al. (2003) synthesized various proposed acceptance models, including TAM, to develop the Unified Theory of Acceptance and Use of Technology (UTAUT). This model aims to elucidate the acceptance and utilization of technology, predicated on four primary dimensions: performance expectancy, effort expectancy, social influence, and facilitating conditions. Subsequently, Venkatesh et al. (2012) refined the model, introducing the UTAUT2, which incorporates three additional dimensions: hedonic motivation (the pleasure derived from using the technology), price value, and the degree of automatic technology use. This reformulated model has garnered increasing adoption among researchers, as noted by Tamilmani et al. (2021) and García et al. (2022), compared to its predecessors.
It is noteworthy that our study exclusively considers the first of the new variables introduced in UTAUT2—hedonic motivation. The variables of price value and automatic technology use were deemed irrelevant for the specific objectives of our research, thus configuring the model as depicted in Figure 1.
Understanding, according to various studies (Gansser and Reich, 2021; Al-Adwan and Al-Debei, 2024; Marikyan and Papagiannidis, 2023), the following dimensions are articulated within the UTAUT and UTAUT2 frameworks:
1. Performance Expectancy (PE): The degree to which an individual believes that the use of Artificial Intelligence in Education (AIEd) enhances their performance in relevant activities.
2. Effort Expectancy (EE): The degree to which an individual perceives that using AIEd will be free from excessive effort.
3. Social Influence (SI): The extent to which an individual perceives pressure from significant others (e.g., family, friends, colleagues) to adopt AIEd.
4. Facilitating Conditions (FC): The degree and availability of resources and support that facilitate the adoption and use of AIEd.
5. Hedonic Motivation (HM): The pleasure or enjoyment derived from using AIEd.
6. Attitude Toward Use (ATT): An individual’s intention to employ AIEd in their educational practices.
7. Behavioral Intention (BI): The extent to which an individual uses AIEd in their professional teaching activities.
It should be noted that the UTAUT and UTAUT2 models have been widely employed to assess the acceptance of various technologies. Within the UTAUT framework, research has explored the acceptance of the metaverse (Lee and Kim, 2022), mobile devices (Mojarro et al., 2019), and virtual reality (Ustun et al., 2023). Meanwhile, UTAUT2 has been applied to analyze the acceptance of technologies such as augmented reality (Huang, 2020), the metaverse (Al-Adwan and Al-Debei, 2024), virtual training platforms (Zacharis and Nikolopoulou, 2022), artificial intelligence (Gansser and Reich, 2021), and its educational applications (Strzelecki, 2023).
In short, these models enable us to comprehend whether teachers are inclined to use Educational Artificial Intelligence (AIEd), the extent of its usage, and the factors influencing these decisions, such as peer opinions, prior experiences, perceived value, and ease of use.
The recent advancements in Artificial Intelligence, particularly since the introduction of ChatGPT-3, have significantly impacted educational research. This progress has spurred investigations into attitudes, acceptance levels, necessary training, and the role of teachers’ beliefs in shaping students’ utilization of AI in educational settings. The importance of training educators in AI for both pedagogical and research purposes has also been highlighted (Alenezi et al., 2023; Tongfei, 2023; González-Mayorga et al., 2024; Temitayo et al., 2024).
Teacher beliefs are influenced by age, as demonstrated by Yuk and Lee (2023), who explored the experiences, perceptions, knowledge, concerns, and intentions of Generation Z students compared to older generations of educators. Their findings indicate generational differences in the adoption of technology in teaching.
Regarding AI, Adekunle et al. (2022) found that teachers’ confidence in teaching AI predicts their intention to incorporate AI into their instruction, suggesting that educators’ beliefs about AI’s usefulness and educational relevance are crucial. However, these beliefs are not uniform and vary depending on the discipline and educational level (Delgado et al., 2024).
From a psychoeducational perspective, two contrasting positions regarding conceptions of learning and teaching are prevalent: behaviorist and constructivist perspectives. The behaviorist approach suggests that knowledge is transmitted to the learner, while the constructivist perspective posits that knowledge is constructed subjectively and through social interaction (Arancibia, 2022). Choi et al. (2023) found that teachers with constructivist beliefs are more likely to integrate AI into their teaching than those with transmissive orientations.
Furthermore, studies have shown that teachers’ beliefs about learning impact their use of ICT in teaching and the frequency of its use. This includes research on general ICT usage (Prestridge, 2017; Bahçivan et al., 2018; Li et al., 2019; Arancibia-Herrera et al., 2024; Palacios-Rodríguez et al., 2024a, 2024b) and specific technologies such as mixed reality (Marín et al., 2023), the Moodle platform (Arancibia, 2022), and digital whiteboards (Burke et al., 2018).
2 Materials and methods
2.1 Research objectives
The research was carried out in the 2023–24 academic year and pursued three general objectives:
• Teachers must know the degree of acceptance of the AIEd.
• Determine if their constructivist and transmissive perspective on teaching determines the degree of acceptance.
• Validate the diagnostic instrument is used reliably.
To respond to these objectives, an “ex post facto” study was developed (Hernández-Sampieri and Mendoza, 2018), constructing the model presented below (Figure 2).
The model allowed the following hypotheses to be formulated:
1. HE1 Performance Expectancy (PE) positively predicts AIEd Behavioral Intention (BI).
2. HE2 Effort Expectancy (EE) positively predicts the AIEd Behavioral Intention (BI).
3. HE3 Social Influence (SI) positively predicts AIEd Behavioral Intention (BI).
4. HE4 Facilitating Conditions (FC) positively predict the AIEd Behavioral Intention (BI).
5. HE5 Hedonic Motivation (HM) positively predicts the intention to use AIEd.
6. HE6 Hedonic Motivation (HM) positively predicts AIEd Behavioral Intention (BI).
7. HE7 Attitude Toward Use (ATT) positively predict AIEd Behavioral Intention (BI).
8. HE8-H9-H10-H11-H12: Transmissive Pedagogical Beliefs (TPB) have a positive influence UTAUT2 dimensions.
9. HE13-H14-H15-H16-H17: Constructivist pedagogical beliefs have a positive influence UTAUT2 dimensions.
2.2 Sample
The research sample consisted of 425 professors from the Private Technical University of Loja (UTPL, Ecuador), of whom 233 (54.8%) were men and 192 (45.2%) were women, the rest, did not want to share the gender. The majority were between 31 and 40 years old (f = 163, 38.4%) and between 41 and 50 (f = 157, 36.9%). Professors who belonged to different areas of knowledge (Table 1).
The participants carried out their professional activity in the UTPL’s face-to-face modality (f = 110, 25.9%), the distance modality (f = 201, 47.3%), or both modalities (f = 114, 26.8%).
Faculty, what questions did they rate themselves, from 0 to 10, on the technical and didactic mastery that they considered they had regarding ICT? They reached an average score of 8.04 regarding technical mastery, with a standard deviation of 1.41, and a mean of 8.04 concerning the didactic domain, in this case with a standard deviation of 1.48.
2.3 Instrument
The instrument was made up of three large blocks: the first collected information on the characteristics of the person who completed it: gender, age, faculty/center where they taught, perception of their technical mastery for the management of the ICT, and perception of its didactic domain for the incorporation of ICT in training; the second, which analyzed the degree of acceptance of the AIEd, created from the instruments developed by different authors (Huang, 2020; Al-Adwan and Al-Debei, 2024; Strzelecki, 2023); and the third, which sought to know the teacher’s transmissive or constructivist pedagogical belief, adopted from the work of Choi et al. (2023).
The instrument had 30 items in total: 5 for the first part, 25 for the second, and 10 for the third. It was also administered via the Internet.
3 Results
3.1 Degree of acceptance and pedagogical beliefs
The means and standard deviations obtained for all the questionnaire items will be presented initially (Table 2).
As can be seen, the average values achieved are generally above the average value of the scale, which was 3.5. At the same time, it can be noted that the scores achieved in the standard deviations, which in some cases exceed two points, reflect a substantial dispersion of the data and, therefore, of the answers offered by the teachers.
Table 3 presents the dimensions of the questionnaire section that analyzed the degree of acceptance of the technology, as well as the mean scores and standard deviation found.
It should be noted that in all cases, the average scores exceed the value of 3.5, which suggests a high degree of acceptance of the AIEd by teachers and a high intention to use it (5.75).
Table 4 offers the average scores achieved in the dimensions referring to the analysis of the teachers’ constructivist and transmissive pedagogical beliefs. This clearly shows that teachers tend toward a constructivist style. As can be seen, there is mostly a perception that teachers assume constructivist positions for developing formative actions (6.49) versus transmissive actions (3.63). However, the high score achieved in the standard deviation found in the transmissive option should also be highlighted, which implies a high dispersion in the answers offered.
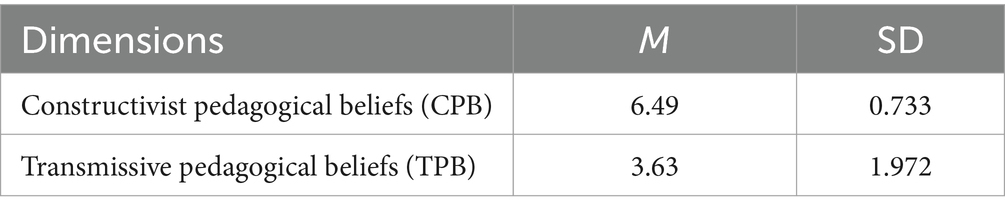
Table 4. Means and standard deviations of the dimensions referring to teachers’ pedagogical beliefs.
3.2 Model validation
Structural analysis models have garnered increasing attention in social research due to their ability to explore both explicit and implicit variables, facilitating their integration in comprehensive models (Alaminos et al., 2015). These models provide a robust framework for examining complex relationships between multiple variables, making them especially useful in fields that require the analysis of latent constructs.
In the context of Structural Equation Modeling (SEM), two primary methodologies are commonly employed: covariance-based SEM and Partial Least Squares (PLS). The selection of methodology depends on the characteristics of the data and the research objectives. In this study, the PLS approach was chosen due to its flexibility, particularly its lack of reliance on the assumption of multivariate normality of the data, which is a prerequisite in covariance-based SEM methods. PLS is particularly suitable when dealing with small sample sizes and models with higher complexity, as it allows the estimation of parameters even when normality is not met (Hair et al., 2017).
For the implementation of the PLS approach, the SmartPLS software was used. This software is widely recognized in the field of SEM for its efficiency in handling PLS-based analysis. The analysis followed the standard phases typically associated with structural equation modeling using PLS, as described in the literature (Sampeiro, 2019). These phases include the specification of the measurement and structural models, the evaluation of the outer model (convergent and discriminant validity), and the assessment of the inner model (path coefficients and the explained variance).
The Cronbach’s Alpha coefficient was initially applied to evaluate the reliability of the different constructs contemplated in the proposed model (Table 5).
According to several authors (Mateo, 2004), exceeding 0.7 indicates that all the levels obtained are adequate.
Regarding the loadings or simple correlations of the indicators with their respective constructs, the different values obtained are presented in Table 6. It is essential to remember that for an indicator to be considered part of a construct, it must have a loading greater than 0.7 (Carmines and Zeller, 1979).
As can be seen, all values have a loading greater than 0.7. Therefore, no items were eliminated during this phase of the analysis.
The next step involves analyzing the composite reliability (CR) related to the internal consistency of the indicators that examine the latent variables. This value allows us to determine if each indicator measures the same thing and whether the latent variable is well represented. The minimum appropriate value is considered to be 0.7. In addition, convergent validity is calculated to determine whether a group of indicators represents a single underlying construct. This value is calculated using the Average Variance Extracted (AVE). To ensure a good fit of the model, it is considered that the value of AVE must be greater than 0.5, which means that more than 50% of the variance of the construct is explained by the indicators (Bagozzi and Yi, 1988). The results are shown in Table 7.
Continuing with obtaining discriminant validity, which determines whether each established construct is significantly different from the others, two approaches are used: the Fornell-Larcker criterion (Table 8) and cross-factor loadings (Table 9).
The Fornell-Larcker criterion is based on the fact that the average extracted variance of a construct must be greater than the variance that said construct shares with the other constructs in the model. Similarly, the correlations between the constructs must be less (in absolute value) than the square root of the average variance extracted. This condition is verified by analyzing that the values on the diagonal, which correspond to the square root of the average extracted variance, are more significant than the values off the diagonal, which represent the correlations between constructs. This condition is met in all observed cases.
The analyses carried out up to this point lead to the conclusion that the items included in the questionnaire exhibit acceptable levels of reliability and show high consistency concerning the dimensions in which they are located in the model. Next, the formulated structural model is analyzed by generating standardized regression coefficients (path coefficients), Student’s t values and R2 (R-squared). These data provide information on the percentage of variance of the constructs explained by their predictor variables and, therefore, allow the viability of the developed model to be evaluated (Figure 3).
The data from the predictive model indicate that Behavioral Intention (BI) is 75.2%, explained by the rest of the variables, suggesting that these variables strongly influence use behavior. Similarly, Attitude Toward Use (ATT) is explained by 78.6% of the rest of the variables, indicating a significant influence of the variables on intention to use. Furthermore, it is observed that the constructivist and transmissive pedagogical belief variables positively impact the model.
The Student t-test is applied to the path values (path coefficients) using the Bootstrap technique to determine the significance of the scores achieved. This resampling method allows us to estimate the sample distribution and calculate confidence intervals for the path coefficients, which helps to evaluate their statistical significance more robustly (Table 8). All coefficients show significance p < 0.005, which implies statistically significant relationships between the analyzed variables.
Finally, to evaluate the structural model’s fit quality, we use the Standardized Root Mean Square Residual (SRMR) indicator, which registers a value of 0.061. This value is less than 0.08 (Bagozzi and Yi, 1988), which suggests a satisfactory fit of the model. Our study also reveals more significant loading in the model dimensions for teachers with constructivist pedagogical beliefs than those with transmissive beliefs regarding performance expectations, effort expectations, social influence, and facilitating conditions.
4 Discussion and conclusions
The conclusions of our study point in different directions. Firstly, the instrument’s reliability has been demonstrated to exhibit reasonably high levels when analyzing the dimensions identified from the UTAUT2 model and the constructivist and transmissive pedagogical perspectives. These values align with those found by Choi et al. (2023) and Cabero-Almenara et al. (2024).
Simultaneously, the model formulated to analyze the degree of teachers’ acceptance of AIEd proved reliable and valid. The results confirmed the significance of 16 of the 17 formulated hypotheses, with the exception of the hypothesis relating to Effort Expectancy (EE) in Attitude Toward Use (ATT). This discrepancy could be attributed to the ease of using generative AI tools and the lack of knowledge or training among teachers. Future research should consider directly addressing questions about the degree of technical and didactic mastery specifically related to AIEd, rather than to technologies in general.
It is essential to highlight that Attitude Toward Use (ATT) is the most significant and influential dimension concerning Behavioral Intention (BI). Therefore, the intention to use fundamentally determines and directs the actual use by the teacher. Moreover, the results indicate that teachers with constructivist beliefs are more likely to integrate AIEd into teaching than those with transmissive orientations. This finding corroborates the results achieved by Choi et al. (2023). However, in this study, the acceptance model used was the UTAUT2. Only in the case of Effort Expectancy (EE) did teachers with transmissive beliefs establish a higher score load, potentially indicating their perception of greater difficulty in using AIEd.
The findings of this study have both practical and theoretical implications for understanding AIEd. To our knowledge, this is one of the first empirical studies to address teachers’ perceptions of AIEd through the UTAUT2 technology acceptance model. Previous studies have utilized the TAM model of technology acceptance (Choi et al., 2023) or the UTAUT2 model with university students (Strzelecki, 2023).
This study developed a conceptual model derived from the UTAUT2 and integrated the potential significance of teachers’ transmissive or constructivist pedagogical beliefs for the use of AIEd. Through a structural equation modeling (SEM) analysis, it is posited that the proposed model offers an adequate explanation of teachers’ intentions to use AIEd. This study has highlighted the importance of considering Performance Expectancy (PE), Effort Expectancy (EE), Social Influence (SI), Facilitating Conditions (FC), and Hedonic Motivation (HM) in predicting the acceptance of AIEd and its impact on attitudes and behavioral intentions to use (Al-Adwan & Al-Debei, 2024).
The findings reveal that teachers with a more constructivist approach positively correlate with the perceived usefulness and effectiveness of AIEd, leading to a higher likelihood of adoption. Consequently, educational institutions must promote a constructivist-oriented pedagogical perspective while providing teacher training programs to familiarize them with AIEd and enhance their digital competencies for integration into the educational environment.
However, this research has limitations that should be addressed in future studies. Firstly, the extent to which respondents were exposed to AIEd was not considered. Additionally, the demographic characteristics of the teachers, such as age, gender, and subjects taught, were not included in the analyses but will be addressed in future work currently in preparation.
Finally, incorporating qualitative data would provide a more comprehensive investigation into the determinants influencing teachers’ acceptance of AIEd. Combining qualitative methods, such as focus group interviews or nominal group techniques, could enable researchers to unravel the underlying mechanisms involved in this acceptance process.
Data availability statement
The raw data supporting the conclusions of this article will be made available by the authors, without undue reservation.
Ethics statement
Ethical approval was not required for the studies involving humans because Permission is not required for this type of study where the data is anonymous and informed consent is obtained from the participants. The studies were conducted in accordance with the local legislation and institutional requirements. Permission is not required for this type of study where the data is anonymous and informed consent is obtained from the participants. Waived the requirement of written informed consent for participation from the participants or the participants’ legal guardians/next of kin because Permission is not required for this type of study where the data is anonymous and informed consent is obtained from the participants.
Author contributions
JC-A: Conceptualization, Data curation, Formal analysis, Funding acquisition, Investigation, Methodology, Project administration, Resources, Software, Supervision, Validation, Visualization, Writing – original draft, Writing – review & editing. AP-R: Conceptualization, Data curation, Formal analysis, Funding acquisition, Investigation, Methodology, Project administration, Resources, Software, Supervision, Validation, Visualization, Writing – original draft, Writing – review & editing. ML-A: Conceptualization, Data curation, Formal analysis, Funding acquisition, Investigation, Methodology, Project administration, Resources, Software, Supervision, Validation, Visualization, Writing – original draft, Writing – review & editing. PA-A: Conceptualization, Data curation, Formal analysis, Funding acquisition, Investigation, Methodology, Project administration, Resources, Software, Supervision, Validation, Visualization, Writing – original draft, Writing – review & editing.
Funding
The author(s) declare that financial support was received for the research, authorship, and/or publication of this article. This study was funded by the Spanish State Programme to Promote Scientific and Technological Research and its Transfer, within the framework of the Spanish State Plan of Scientific, Technical and Innovative Research 2021–2023. Spanish Ministry of Science and Innovation. Reference number: PID2022-136430OB-I00.
Conflict of interest
The authors declare that the research was conducted in the absence of any commercial or financial relationships that could be construed as a potential conflict of interest.
Publisher’s note
All claims expressed in this article are solely those of the authors and do not necessarily represent those of their affiliated organizations, or those of the publisher, the editors and the reviewers. Any product that may be evaluated in this article, or claim that may be made by its manufacturer, is not guaranteed or endorsed by the publisher.
References
Adekunle, M., Temitayo, I., Adelana, O., Aruleba, K., and Sunday, S. (2022). Teachers' readiness and intention to teach artificial intelligence in schools. Comp. Educ. 3:100099. doi: 10.1016/j.caeai.2022.100099
Al-Adwan, A. S., and Al-Debei, M. M. (2024). The determinants of gen Z's metaverse adoption decisions in higher education: integrating UTAUT2 with personal innovativeness in IT. Educ. Inf. Technol. 29, 7413–7445. doi: 10.1007/s10639-023-12080-1
Alaminos, A., Francés, F., Penalva, C., and Santacreu, O. (2015). Introduction to structural models in social research. Madrid: PYDLOS Ediciones.
Alenezi, M. A. K., Mohamed, A. M., and Shaaban, T. S. (2023). Revolutionizing EFL special education: how ChatGPT is transforming the way teachers approach language learning. Innoeduca. Int. J. Technol. Educ. Innov. 9, 5–23. doi: 10.24310/innoeduca.2023.v9i2.16774
Alghamdi, A. A. (2023). Exploring early childhood Teachers' beliefs about STEAM education in Saudi Arabia. Early Childhood Educ. J. 51, 247–256. doi: 10.1007/s10643-021-01303-0
Arancibia, M. L. (2022). Analysis of teachers' beliefs and their integration with information and communication technologies in the teaching-learning process. UCOPress.
Arancibia-Herrera, M., Castro-Appelhanz, M. J., and Sigerson, A. (2024). Relationships between conceptions and ICT competencies: study of nine didactic sequences of Chilean teachers. Educ. Res. 50:e260125. doi: 10.1590/S1678-4634202450260125es
Bagozzi, R. P., and Yi, Y. (1988). On the evaluation of structural equation models. J. Acad. Mark. Sci. 16, 74–94. doi: 10.1007/BF02723327
Bahçivan, E., Güneş, E., and Üstündağ, M. (2018). A comprehensive model covering prospective teachers' technology use: the relationships among self, teaching and learning conceptions and attitudes. Technol. Pedagog. Educ. 27, 399–416. doi: 10.1080/1475939X.2018.1479296
Barroso, J., Cabero, J., and Gutierrez, J. J. (2018). The production of learning objects in augmented reality by university students, degree of acceptance of this technology and motivation for its use. Mexican J. Educ. Res. 23, 1261–1283.
Bice, H., and Tang, H. (2022). Teachers' beliefs and practices of technology integration at a school for students with dyslexia: a mixed methods study. (2022). Educ. Inf. Technol. 27, 10179–10205. doi: 10.1007/s10639-022-11044-1
Burke, P., Schuck, S., Aubusson, P., Kearney, M., and Frischknecht, B. (2018). Exploring teacher pedagogy, stages of concern and accessibility as determinants of technology adoption. Technol. Pedagog. Educ. 27, 149–163. doi: 10.1080/1475939X.2017.1387602
Cabero, J., Llorente, C., Palacios, A., and Gallego, Ó. (2023). Degree of acceptance of virtual reality by health sciences students. Int. J. Environ. Res. Public Health 20:5571. doi: 10.3390/ijerph20085571
Cabero-Almenara, J., Palacios-Rodríguez, A., Loaiza-Aguirre, M. I., and Rivas-Manzano, M. D. R. D. (2024). Acceptance of educational artificial intelligence by teachers and its relationship with some variables and pedagogical beliefs. Educ. Sci. 14:740. doi: 10.3390/educsci14070740
Casimiro, L. F. (2022). Beliefs of teachers in service and in training regarding the teaching-learning of English. Mendive 20, 821–839.
Cheng, S., Chang, J., and Romero, K. (2022). Are pedagogical beliefs an internal barrier for technology integration? The interdependent nature of teacher beliefs. Educ. Inf. Technol. 27, 5215–5232. doi: 10.1007/s10639-021-10835-2
Choi, S., Jang, Y., and Kim, H. (2023). Influence of pedagogical beliefs and perceived trust on Teachers' acceptance of educational artificial intelligence tools. Int. J. Human Comp. Interact. 39, 910–922. doi: 10.1080/10447318.2022.2049145
Clark, C. M., and Peterson, P. L. (1986). Teachers' thought processes. Handbook of research on teaching. Nueva York: Macmillan.
Davis, F. (1989). Perceived usefulness, perceived ease of use, and user acceptance of information technology. MIS Q. 13, 319–340. doi: 10.2307/249008
Delgado, N., Campo Carrasco, L., Sainz de la Maza, M., and Etxabe-Urbieta, J. M. (2024). Application of artificial intelligence (AI) in education: the benefits and limitations of AI perceived by teachers in primary education, secondary education and higher education. Interuniv. Elect. J. Teach. Train. 27, 207–224. doi: 10.6018/reifop.577211
Friesen, D., Shory, U., and Lamoureux, C. (2023). The role of self-efficacy beliefs and even educational beliefs on teacher burnout. Soci. Sci. Human. Open 8:100599. doi: 10.1016/j.ssaho.2023.100599
Gansser, O., and Reich, C. (2021). A new acceptance model for artificial intelligence with extensions to UTAUT2: an empirical study in three segments of applications. Technol. Soc. 65:101535. doi: 10.1016/j.techsoc.2021.101535
García, A., Kelly, M. R., and Stamatis, K. (2022). When technology goes unnoticed: teacher beliefs and assumptions about technology use in three 9th grade English classrooms. Pedagogies 17, 54–75. doi: 10.1080/1554480X.2020.1781638
González-Mayorga, H., Rodríguez-Esteban, A., and Vidal, J. (2024). The use of OpenAI's GPT model for the analysis of open texts in educational research. Pixel Bit. J. Media Educ. 69, 227–253. doi: 10.12795/pixelbit.102032
Hair, J. F., Hult, G. T. M., Ringle, C. M., and Sarstedt, C. M. (2017). A Primer on partial least squares structural equation modeling (PLS-SEM). 2nd Edition. Thousand Oaks, CA: Sage Publications Inc.
Hernández-Sampieri, R., and Mendoza, C. P. (2018). Research methodology: Quantitative, qualitative and mixed routes. Madrid: McGraw-Hill.
Hoareau, L., Thomas, A., Tazouti, Y., Dinet, J., Luxembourger, C., and Jarlégan, A. (2021). Beliefs about digital technologies and teachers' acceptance of an educational app for preschoolers. Comput. Educ. 172:104264. doi: 10.1016/j.compedu.2021.104264
Huang, F. H. (2020). (2020). Adapting UTAUT2 to assess user acceptance of an e-scooter virtual reality service. Virtual Reality 24, 635–643. doi: 10.1007/s10055-019-00424-7
Ifinedo, E., Rikala, J., and Hämäläinen, T. (2020). Factors affecting Nigerian teacher educators' technology integration: considering characteristics, knowledge constructs, ICT practices and beliefs. Comput. Educ. 146:103760. doi: 10.1016/j.compedu.2019.103760
Jiménez, E. (2019). Beliefs of higher education teachers about their teaching practice. Uaricha 16, 15–26.
Lee, U. K., and Kim, H. (2022). UTAUT in Metaverse: an “Ifland” case. J. Theor. Appl. Electron. Commer. Res. 17, 613–635. doi: 10.3390/jtaer17020032
Li, Y., Garza, V., Keicher, A., and Popov, V. (2019). Predicting high school teacher use of technology: pedagogical beliefs, technological beliefs and attitudes, and teacher training. Tech Know Learn 24, 501–518. doi: 10.1007/s10758-018-9355-2
Mante-Estacioa, J., and Tupas, R. (2023). Questions as beliefs: investigating teachers' beliefs in reading through inquiry questions. Educ. Inq. 5, 1–20. doi: 10.1080/20004508.2022.2123121
Marikyan, D., and Papagiannidis, S. (2023). Technology Acceptance Model: A review. ed. S. Papagiannidis TheoryHub Book. Available at: https://open.ncl.ac.uk/
Marín, V., Sampedro, B., and Vega, E. (2023). Beliefs of secondary education teachers regarding the use of mixed reality in the classroom. Interuniv. Elect. J. Teach. Educ. 26, 85–97.
Mojarro, A., Duarte, A., Guzmán, M., and Aguaded, I. (2019). Mobile learning in university contexts based on the unified theory of acceptance and use of technology (UTAUT). Journal of New Approaches in Educational Research 8, 7–17. doi: 10.7821/naer.2019.1.317
Montanares, E. G., and Junod, P. A. (2018). Beliefs and teaching practices of university professors in Chile. Elect. J. Educ. Res. 20, 93–103. doi: 10.24320/redie.2018.20.1.1383
Palacios-Rodríguez, A., Cabero-Almenara, J., and Serrano-Hidalgo, M. (2024a). Educación Médica y Carga Cognitiva: Estudio de la Interacción con Objetos de Aprendizaje en Realidad Virtual y Vídeo 360°. Revista de Educación a Distancia 24. doi: 10.6018/red.582741
Palacios-Rodríguez, A., Guillén Gámez, F. D., Cabero Almenara, J., and Gutiérrez Castillo, J. J. (2023). Teacher digital competence in the education levels of compulsory education according to DigCompEdu: the impact of demographic predictors on its development. Interact. Design Archit. J. IxD&A 57, 115–132. doi: 10.55612/s-5002-057-007
Palacios-Rodríguez, A., Llorente-Cejudo, C., Lucas, M., and Bem-haja, P. (2024b). Macroevaluación de la competencia digital docente. Estudio DigCompEdu en España y Portugal. RIED 28. doi: 10.5944/ried.28.1.41379
Prestridge, S. (2017). Examining the shaping of teachers' pedagogical orientation for the use of technology. Technol. Pedagog. Educ. 26, 367–381. doi: 10.1080/1475939X.2016.1258369
Rodríguez-Sabiote, C., Valerio-Peña, A. T., and Batista-Almonte, R. (2023). Validation of a scale of the extended technology acceptance model in the Dominican context: [validation of a scale of the extended technology acceptance model in the Dominican context]. Pixel Bit. J. Med. Educ. 68, 217–244. doi: 10.12795/pixelbit.100352
Rodríguez-Sosa,, and Solis-Manrique, (2017). Teaching beliefs: what is done in the classroom is a consequence of what is thought. Purposes Represent. 5, 07–20. doi: 10.20511/pyr2017.v5n1.155
Sampeiro, V. (2019). Structural equations in educational models: characteristics and phases in their construction. Opening 11, 90–103.
Strzelecki, A. (2023). To use or not to use ChatGPT in higher education? A study of students' acceptance and use of technology. Interact. Learn. Environ. 4, 1–14. doi: 10.1080/10494820.2023.2209881
Tamilmani, K., Rana, N., Wamba, F., and Dwivedi, R. (2021). The extended unified theory of acceptance and use of technology (UTAUT2): a systematic literature review and theory evaluation. Int. J. Inf. Manag. 57:102269. doi: 10.1016/j.ijinfomgt.2020.102269
Temitayo, I., Adekunle, M., and Tolorunleke, A. (2024). Investigating pre-service teachers' artificial intelligence perception from the perspective of planned behavior theory. Comp. Educ. 6:100202. doi: 10.1016/j.caeai.2024.100202
Tondeur, J., van Braak, J., Ertmer, P. A., and Ottenbreit-Leftwich, A. (2017). Understanding the relationship between teachers' pedagogical beliefs and technology use in education: a systematic review of qualitative evidence. Educ. Technol. Res. Dev. 65, 555–575. doi: 10.1007/s11423-016-9481-2
Tongfei, F. (2023). Practice and exploration of conducting artificial intelligence teacher training in universities under the background of industry education integration. Adult High. Educ. 5. doi: 10.23977/aduhe.2023.051318
Ustun, A. B., Karaoglan-Yilmaz, F. G., and Yilmaz, R. (2023). (2023). Educational UTAUT-based virtual reality acceptance scale: a validity and reliability study. Virtual Reality 27, 1063–1076. doi: 10.1007/s10055-022-00717-4
Venkatesh, V., Morris, M. G., Davis, G. B., and Davis, F. D. (2003). User acceptance of information technology: toward a unified view. MIS Q. 27, 425–478. doi: 10.2307/30036540
Venkatesh, V., Thong, J. I. L., and Xu, X. (2012). Consumer acceptance and use of information technology: extending the unified theory of acceptance and use of technology. MIS Q. 36, 157–178. doi: 10.2307/41410412
Vettori, G., Bigozzi, L., Vezzani, C., and Pinto, G. (2022). The mediating role of emotions in the relationship between beliefs and teachers' job satisfaction. Acta Psychol. 226:103580. doi: 10.1016/j.actpsy.2022.103580
Waluyo, B., and Apridayani, A. (2021). Teachers' beliefs and classroom practices on the use of video in English language teaching. Studies English Lang. Educ. 8, 726–744. doi: 10.24815/siele.v8i2.19214
Yuk, C., and Lee, K. (2023). The AI generation gap: are gen Z students more interested in adopting generative AI such as ChatGPT in teaching and learning than their gen X and millennial generation teachers? Smart Learn. Environ. 10:60. doi: 10.1186/s40561-023-00269-3
Keywords: artificial intelligence, higher education, professor, educational technology, UTAUT2
Citation: Cabero-Almenara J, Palacios-Rodríguez A, Loaiza-Aguirre MI and Andrade-Abarca PS (2024) The impact of pedagogical beliefs on the adoption of generative AI in higher education: predictive model from UTAUT2. Front. Artif. Intell. 7:1497705. doi: 10.3389/frai.2024.1497705
Edited by:
Rosabel Roig-Vila, University of Alicante, SpainReviewed by:
Julio Ruiz Palmero, University of Malaga, SpainFernando Leal-Ríos, Universidad Autónoma de Tamaulipas, Mexico
Copyright © 2024 Cabero-Almenara, Palacios-Rodríguez, Loaiza-Aguirre and Andrade-Abarca. This is an open-access article distributed under the terms of the Creative Commons Attribution License (CC BY). The use, distribution or reproduction in other forums is permitted, provided the original author(s) and the copyright owner(s) are credited and that the original publication in this journal is cited, in accordance with accepted academic practice. No use, distribution or reproduction is permitted which does not comply with these terms.
*Correspondence: Antonio Palacios-Rodríguez, YXByb2RyaWd1ZXpAdXMuZXM=