- School of Digital Technologies, Tallinn University, Tallinn, Estonia
Introduction: The evolving labour market requirements amidst digital transformation necessitate robust skills intelligence for informed decision-making and adaptability. Novel technologies such as Big Data, Machine Learning, and Artificial Intelligence have significant potential for enhancing skills intelligence.
Methods: This study bridges the gap between theory and practice by designing a novel software artefact for skills intelligence management. With its systematic framework for identifying skills intelligence elements, an assessment instrument, and an implementation methodology, the artefact ensures a thorough approach to skills intelligence management.
Results: The artefact was demonstrated in 11 organisations. Feedback collected from interviews, focus group sessions, and observations (N = 19) indicated that the artefact is a feasible starting point for implementing or systematising skills intelligence management. Participants suggested improvements but concurred that the systematic approach enhances skills intelligence data collection and quality.
Discussion: The study shows that the artefact facilitates the application of advanced technologies in skills intelligence management. Additionally, it contributes a set of principles for effective skills intelligence management, fostering a broader conversation on this critical topic. Participants’ feedback underscores the artefact’s potential and provides a basis for further refinement and application in diverse organisational contexts.
Introduction
The rapidly evolving needs of the labour market place higher demands on workforce skills, and the increased dynamism of the labour market needs to be compensated with more frequent and adaptive upskilling of workers (Cedefop, 2020b). Skills intelligence is the outcome of expert-driven identification, analysis, synthesis and presentation of qualitative or quantitative information about skills and the labour market, drawn from multiple sources and adjusted to the needs of different users (Cedefop, 2019). It is pivotal in informed decision-making (Cedefop, 2020a) to ensure efficient workforce skills management. Skills intelligence helps address skills gaps through quick and automated data analysis for dynamic needs (Cedefop, 2020b; Maldonado-Mariscal et al., 2023). Novel digital technologies, particularly Artificial Intelligence (AI), enhance decision-making for personalised training, recruitment and Human Resources processes, but this requires quality data and collaboration from stakeholders (DiRomualdo et al., 2018; Maldonado-Mariscal et al., 2023). Organisations often need more suitable technology infrastructure and support in data collection and analysis standardisation (Patil and Priya, 2024).
This study seeks to bridge this gap by enhancing the understanding and application of skills intelligence within a single organisation’s context. The current study is one cycle embedded into a more extended study that explores how novel digital technologies can be leveraged in collectively adapting to skills instability. Artefacts generated in the previous cycles form the foundation of the current study. The first study proposed a conceptual data-driven competency management platform to mediate competency-related data between stakeholder organisations to generate skills intelligence (Kusmin et al., 2017). The second study (Kusmin et al., 2018) explored how competency information could be mediated between universities and business organisations to advance workforce skills-related cooperation. The central artefact of the study was a functional software application based on the concept from the first study: a prototypical competency-management platform for universities, business organisations and students. The results of the study suggested that to achieve effective cooperation around workforce competencies, all stakeholders need to go through organisational changes, and thus, it was advised to explore the context of different organisations. The current study is a logical continuation that aims to develop conceptual software further to support the implementation of skills intelligence management practices in business organisations. This reflects a shift towards addressing individual organisations’ unique needs and challenges to realise the benefits of skills intelligence on a more granular level. To achieve this, the following research questions were formulated:
• What are the core elements of skills intelligence management in an organisation?
• How can skills intelligence management be effectively implemented in an organisation?
• How to evaluate the current skills intelligence situation in an organisation?
• What are the core principles for supporting skills intelligence management implementation?
Our paper presents a novel artefact for skills intelligence management, including an implementation methodology and evaluation instrument. We identify challenges related to skills intelligence and explore the concept’s strategic importance beyond human resources. The proposed framework aims to improve skills-related data quality, providing organisations with a foundation for leveraging Big Data (BD) to facilitate the application of novel technologies such as Artificial Intelligence (AI) and Machine Learning (ML) in Human Resources (HR) processes. A significant contribution of the paper is bridging the gap between practice and theory to foster a broader conversation around skills intelligence.
This article is organised as follows. First, we provide an overview of the background, discussing the current skills instability and the subsequent need for enhanced skills intelligence. We explore potential technologies that can improve the skills intelligence landscape and their data processing requirements. We identify the research gap, formulate the research questions, and outline the study’s objectives. Next, we detail the study’s methodology and describe its iterative process. Following this, we present the designed artefact and its contributions to knowledge. Finally, we discuss the implications, limitations, and directions for future research.
Our analysis demonstrates that current skills intelligence practices face significant challenges like sporadic and unstructured data collection. Despite its growing importance, skills intelligence has yet to be widely recognised, indicating a need for broader dissemination and standardisation. Participants confirmed that skills intelligence extends beyond HR, providing strategic advantages. Inter-organisational cooperation emerged as crucial, with shared challenges addressed collaboratively. Implementing a systematic approach to skills intelligence management is essential for its effective application, paving the way for future enhancements through novel technologies.
Theoretical background
Skills instability and skills intelligence
Digital transformation has caused significant shifts in the labour market, leading to a rapid worldwide increase in skills instability. New technological advancements cause new skills to emerge, existing skills to evolve, and others to expire due to automation (Gartner Inc., 2020). The COVID-19 pandemic accelerated this pace, making digital technologies imperative for work, learning, and social activities, necessitating digital skills for all jobs (European Commission, 2021; Méndez-Domínguez et al., 2023). To remain competitive, there is an increasing need for constant upskilling and reskilling (Cedefop, 2020b). Life-long learning supports citizens in strengthening their skills throughout life, allowing them to thrive in fast-evolving workplaces (European Commission, 2016).
Organisations require accurate forecasts about future work requirements to make informed decisions about upskilling, training, or recruitment (Bonen and Loree, 2021). CEDEFOP, the European Centre for the Development of Vocational Training, defines skills intelligence as the outcome of an expert-driven process of analysing, synthesising and presenting quantitative or qualitative information on the labour market and skills (Cedefop, 2019). However, the information provided by CEDEFOP is mainly helpful for policymaking and less so for company-specific needs (Maldonado-Mariscal et al., 2023). This article focuses on the organisation-specific aspects of skills intelligence, characterised by higher dynamism for making short-term data-driven HR decisions. With the labour market changing rapidly, there is an urgency to employ novel digital technologies to promote these processes.
Enhancing skills intelligence management with digital technologies
Digital technologies, including Big Data, Artificial Intelligence, and Machine Learning, transform HR processes by enabling personalised, just-in-time training, predictive workforce planning, and strategic decision-making (DiRomualdo et al., 2018; Patil and Priya, 2024). These advancements in digital tools are integral to enhancing skills intelligence management by providing more detailed data regarding candidate and employee competencies, attitudes, perceptions, and behaviors. This data enables personalised, context-specific training and learning support when combined with other relevant information.
Analytics capabilities help anticipate each individual’s future learning needs and most effective training methods while providing insights into the drivers of effective training and pinpointing methods to generate measurable performance improvements. Despite these advancements, HR analytics faces challenges such as insufficient data quality, standardisation and integration (Angrave et al., 2016; Patil and Priya, 2024). The theoretical models of BD’s contribution to Human Resource Management (HRM) are still scarce in empirical application (Garcia-Arroyo and Osca, 2021). Organisations must understand which data are needed for skills intelligence inferences and their quality requirements to ensure accurate and relevant outcomes.
Research on analytics and BD in HR has mainly revolved around what should be done, but there needs to be more understanding of how it can be done in different contexts and the subsequent results (Angrave et al., 2016). The collaborative nature of skills intelligence demands a collective effort from stakeholders in gathering, analysing, and interpreting data to achieve a nuanced understanding of skills dynamics and enable informed decision-making (Maldonado-Mariscal et al., 2023). Gathering and aggregating skills-related information from different organisations and applying ML approaches can help accelerate the feedback loop between skills intelligence stakeholders (Kusmin et al., 2018).
Related work
Table 1 provides an overview of the most relevant scientific literature analysed to assess the current knowledge on using novel technologies in HR and improving skills intelligence. The existing literature predominantly focuses on theoretical frameworks, sector-specific applications, and the broad potential benefits of skills intelligence and digital HR transformation. However, empirical studies providing actionable insights and practical guidelines for organisations to integrate these technologies into their HR practices effectively are limited.
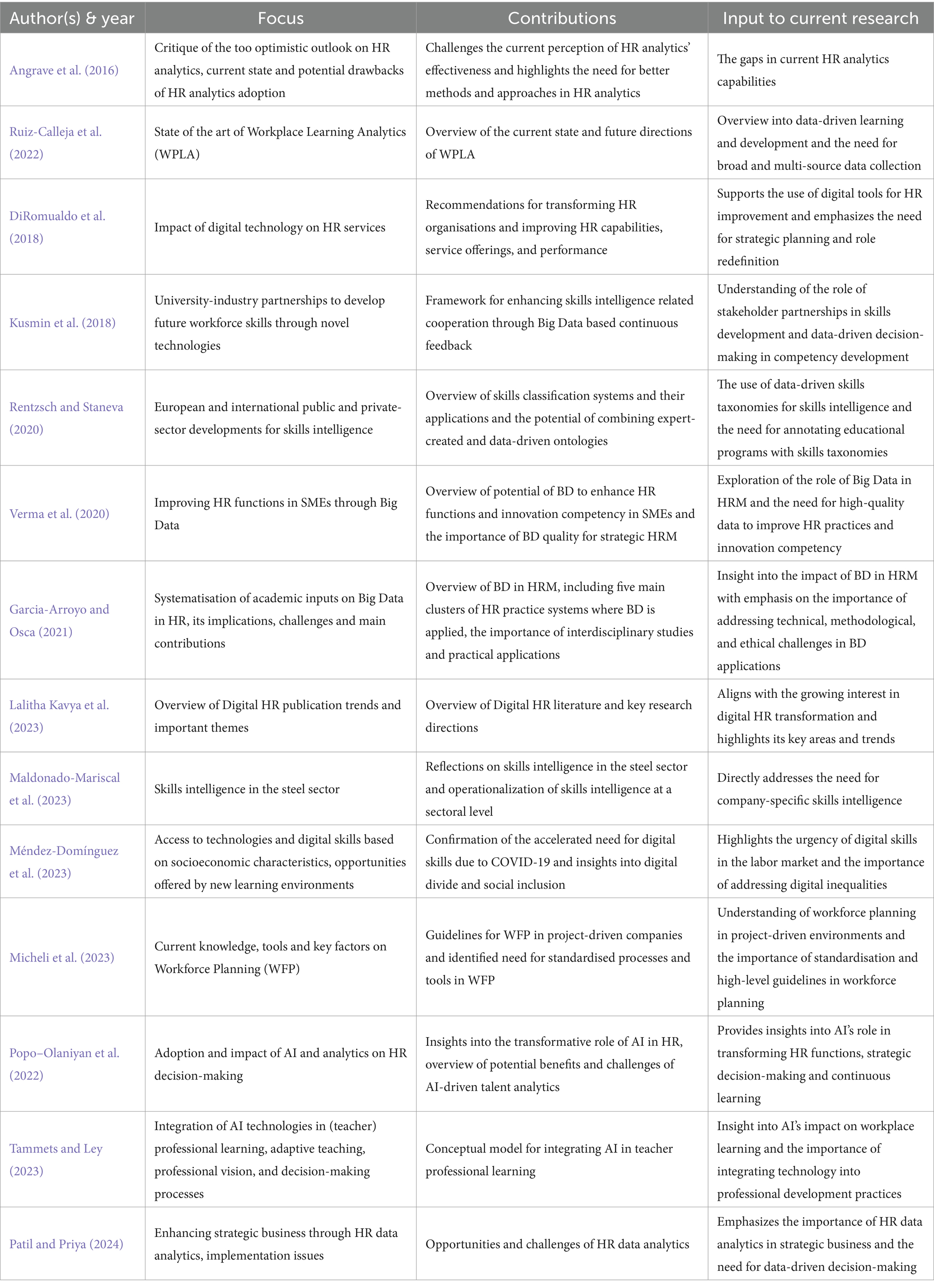
Table 1. Reviewed literature with focus, main contributions and overview of input to the current research.
The literature overview uncovered the following gaps in research related to skills intelligence:
Sector-specific limitations
Many studies, such as those by Rentzsch and Staneva (2020) and Maldonado-Mariscal et al. (2023), focus on sector-specific applications of skills intelligence (e.g., the steel sector, European public and private sectors). These studies do not address the broader applicability of skills intelligence across various industries.
Lack of standardisation
Research by Micheli et al. (2023) highlights the need for more standardisation in workforce planning processes, leading to heterogeneous approaches among different organisations. Standardised practices and guidelines that can be universally applied are needed.
Implementation challenges
Studies such as those by DiRomualdo et al. (2018) and Patil and Priya (2024) discuss the transformative potential of novel digital technologies in HR but do not provide detailed, empirical evidence on how organisations can overcome implementation challenges, particularly in data collection and analysis standardisation.
Focus on theoretical approaches
While reviews like Angrave et al. (2016) and Garcia-Arroyo and Osca (2021) provide critical analyses and theoretical insights, there is a scarcity of empirical data and practical case studies that demonstrate successful implementation of skills intelligence and digital HR practices in real-world settings.
Inter-organisational cooperation
Research by Kusmin et al. (2018) suggests that organisational changes are needed to achieve effective cooperation around workforce competencies. However, more insight is needed into how individual organisations can implement these changes to facilitate such cooperation.
The current seeks to bridge these gaps by thoroughly exploring how skills intelligence can be effectively managed and implemented within a single organisation’s context. It is the first to combine theoretical and practical aspects through empirical research and the development of practical tools and methodologies to standardise and successfully implement skills intelligence management across diverse organisational contexts.
Methods
Research method
Due to the nature and context of the problem, the study followed design science (DS) research principles. To scaffold the procedures of design science research, the research was guided by the design science research methodology (DSRM) by Peffers et al. (2007). Based on the methodology, the study followed a design and development-centred approach, which entails three activities: design and development, demonstration, and evaluation. By proposing an approach for skills intelligence management, planning and evaluation, this study builds on the earlier works that addressed these challenges and outlined similar solutions for broader application across multiple organisations (Kusmin et al., 2017, 2018, 2019).
Research activities
The study’s central artefact was developed and improved over four increments. The first increment built on the authors’ previous studies and complemented them with kernel theories on various skills intelligence concepts, analytics, BD, AI, and ML in HRM, novel technologies for WPL and workforce planning, and the more practical COBIT program initiation approach. Figure 1 depicts each increment’s research inputs, artefact evolution, demonstration methods, and primary evaluation focuses.
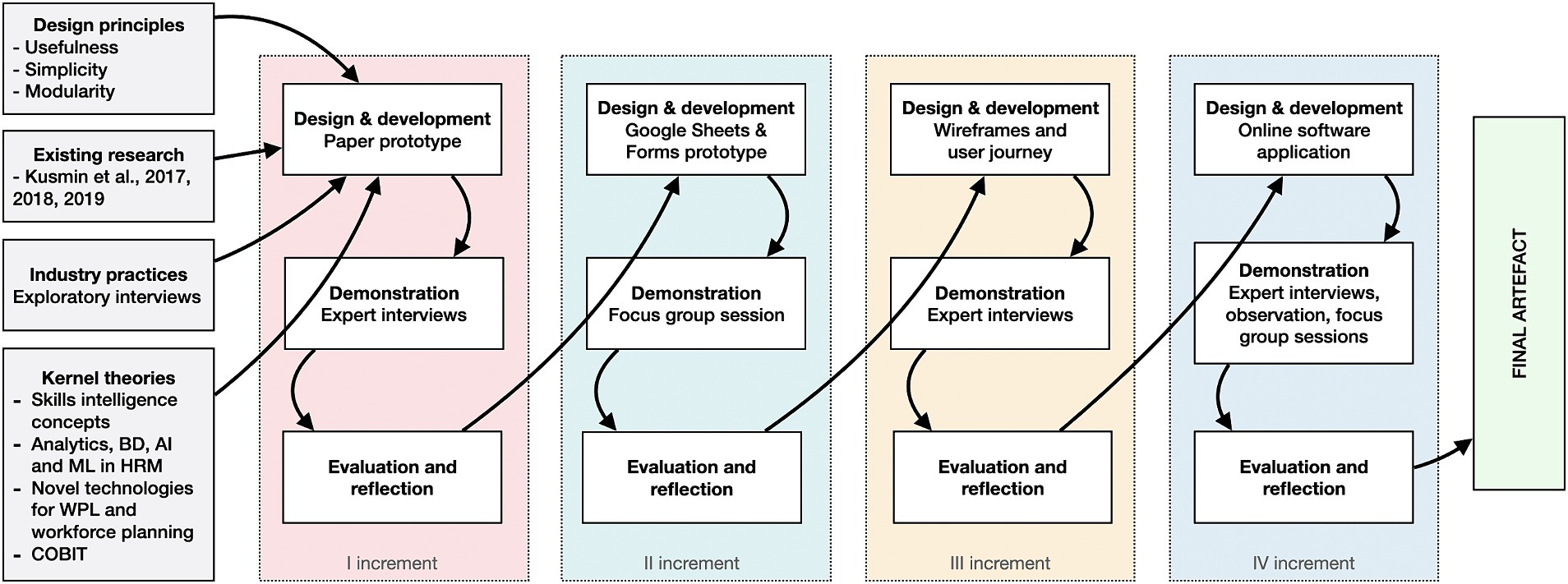
Figure 1. Research inputs, artefact evolution, demonstration methods, and main evaluation focuses of each increment.
Design and development
In the design and development phase, the primary artefact created and iteratively improved was a software application supporting skills intelligence management in an organisation. The artefact was developed by integrating “sub-artefacts”: a framework for mapping skills intelligence management key elements, a methodology for skills intelligence management implementation, and a skills intelligence situation assessment instrument. The software development process followed agile development principles and was, respectively, designed to prioritise usefulness, simplicity and modularity. From a usefulness perspective, the artefact needs to provide immediate benefits to early adopters regardless of the small user base. Thus, the system was somewhat tailored to the needs of the participants. To ensure the fast uptake of the system, another important design aspect was simplicity, which necessitated a strong emphasis on UI/UX considerations. Finally, the system was designed to ensure modularity in both its functional and architectural aspects.
The artefact evolved through four iterations and took several forms: a paper-based prototype, a functional prototype using Google Sheets and Forms, a UI/UX-focused wireframe prototype to specify the user journey, and finally, the completed functional software system.
Primary artefact: a software platform for skills intelligence management
The primary artefact, a software platform, consolidates skills intelligence management into a structured and integrated system, presenting a thorough and transparent process for skills intelligence management activities. After evaluations, the results are summarised and visualised to users. Based on this information, stakeholders can meet to establish targets, agree on future activities and allocate tasks and responsibilities. Users have an overview of activities and statuses on the main dashboard between evaluation periods. Figure 2 is an example of a wireframe of the software platform depicting the evaluation step of the methodology. The source code of the software platform server1 and client2 are available on public GitHub repositories.
Sub-artefact: skills intelligence management framework
The Skills Intelligence Management Planning, Implementation and Evaluation (SIMPIE) framework (depicted in Figure 3) was developed to specify the initial functional requirements of the artefact. This was done by first summarising the findings from Kusmin et al. (2017) and Kusmin et al. (2018), depicted in Figure 4, then mapping the critical elements of skills intelligence management: stakeholders, processes, data types, and tools and technologies and identifying the relationships between these elements. It is important to note that the elements in the framework serve as a reference and are not intended to be a comprehensive collection. Visualising the framework elements is primarily intended for the initial SIMPIE activities, serving as a template and guidance. However, it also acts as a visual reference when onboarding new participants to skills intelligence management processes.
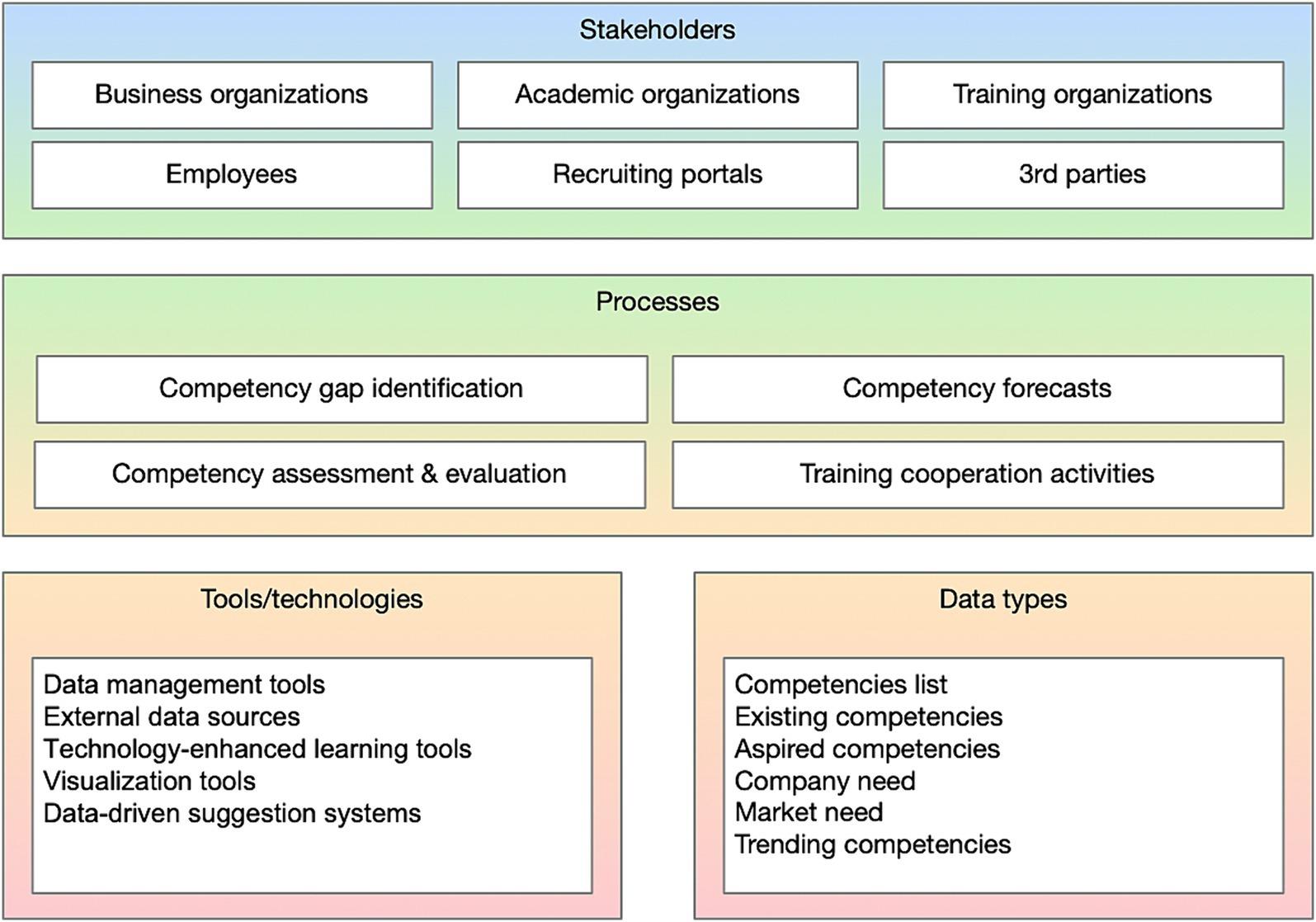
Figure 4. Visualization of the skills intelligence management framework elements adapted from Kusmin et al. (2017, 2018).
Sub-artefact: skills intelligence situation assessment instrument
The skills intelligence situation assessment instrument was developed to help organisations understand their skills intelligence-related data priorities and current data quality. The instrument provides a clear list of activities all participants must follow in skills intelligence management processes. As the first assessment activity, the participants list the activities (processes) that depend on skills intelligence and map the data types used in these processes. The participants identify the data source(s) and their respective supervisors for each data type. Each of the data types is then assessed against previously agreed quality criteria. This results in a matrix of quality criteria, data types, their scores, and feedback to data source supervisors. During the assessment process, participants are requested to evaluate each data type against the quality criteria on a Likert scale ranging from 1 (poor) to 5 (excellent). The matrix provides two sets of skills intelligence inferences based on the combined assessment results. First, it calculates the average quality score for each criterion across all examined data types. This represents how well the data meets specific quality aspects, such as how complete the data is on average. Secondly, it provides an overall quality score for each data type, representing its strengths and weaknesses across multiple criteria, e.g., completeness, timeliness and accessibility.
The organisation can plan and prioritise the actions needed to improve skills intelligence based on the results. Lower data type quality scores indicate a need to redesign the process for collecting, storing, and communicating this data type and include the stakeholders who prioritised this data type higher. Low scores across a particular quality criterion indicate more technical problems: for example, the data collection frequency is low (timeliness), or the established data models are too vague for their intended purposes (precision). Additionally, the results will serve as a baseline for the subsequent evaluation to calculate the KPIs. The prototypes of the instrument can be seen in Figure 5.
Sub-artefact: skills intelligence management implementation methodology
The platform functionalities were incrementally combined into a comprehensive methodology based on the COBIT program initiation approach phases (ISACA, 2018). Using the COBIT system, where each implementation phase must answer a particular question, seven similar phases were mapped: scope, situation, targets, plan, implementation, evaluation, and sustainment. The implementation phases are depicted in Figure 6.
Phase 1, “Scope”, corresponds to the COBIT program initiation phase that answers the question “What are the drivers?” However, the Scope asks, “Who are the drivers?” This phase consists of stakeholder identification and scope definition, which can be done through simple open-ended interviews. During the interview, the participants analyse the generic framework structure and map the most critical elements in the organisation’s context. The interview can serve as a tool for snowball sampling as participants can suggest other people who should be included in the process.
Phase 2, “Situation”, corresponds to the COBIT problems and opportunities definition phase. It answers the question, “Where are we now?”. The first activity of this phase is aligning specific organisation details with the corresponding framework elements. For instance, the framework element “stakeholders” could include “strategic management,” “HR,” or even more specifically, an actual person’s name. Similarly, the process “competency suggestions” could be “provide personal suggestions for horizontal career development opportunities.” The second activity of the phase is skills intelligence evaluation, which is conducted with the skills intelligence evaluation instrument.
Phase 3, “Targets”, outlines the expected outcomes of the process and answers the question “Where do we want to be?” The first action is to analyse the outcomes of the skills intelligence matrix to prioritise the data types and quality indicators that need to be improved and establish the expected values for the subsequent evaluation. The second activity is to specify and prioritise actions. The suggested form for this activity is focus group sessions.
Phase 4, “Plan”, answers “What needs to be done?”. The results of phase 3 are represented as scenarios, which, in turn, are presented as an IS architecture to outline its development plan. The suggested form for this phase is collaborative design sessions.
Phase 5, “Implementation”, answers the question “How do we get there?” and is devoted to developing and implementing processes and information systems. This phase differs from the previous phases in that it should follow the principles of software development projects. This means that the Implementation phase might be iterative.
Phase 6, “Evaluation” (“Did we get there?”), is a follow-up evaluation via the skills intelligence evaluation instrument. The results are analysed, discussed and communicated to the stakeholders. This phase can be carried out individually or in groups.
Phase 7, “Sustainment”, seeks to answer the question, “How do we keep the momentum going?” Based on the results of the previous phases, stakeholders’ feedback is collected via interviews, surveys, or other methods. These should be categorised, combined with the KPIs, and phrased as activities to improve the framework implementation.
Demonstration
As the artefact design and development activities followed agile principles, there were several demonstration points. This was done to minimise risks through a faster feedback loop: the artefact was incrementally improved, and each improvement was demonstrated to make minor adjustments based on participant feedback. The artefact was demonstrated in four key phases: a paper-based prototype testing the methodology that formed the functional requirements of the artefact, a Google Sheets and Forms-based functional prototype, wireframes depicting the user journey through the software artefact, and the final functional software. The first two prototypes can be seen in 6. The final software was deployed online and was publicly accessible, and the source code for both the client and server side was uploaded to public repositories in GitHub.
The initial sample was a convenience sample (Teddlie and Yu, 2007) in a project-based IT organisation, which was expanded through snowball sampling (ibid). In total, the participants (N = 19) originated from 11 organisations: a project-based IT software development service provider, a software product provider, the IT departments of two energetics organisations, a retail chain, one government IT organisation, a change management organisation, an international technology organisation and three IT startups. One organisation declined to participate because the shared information would be too sensitive.
The solution’s objectives were qualitative and sought to describe how the new artefact is expected to support the novel problem (Peffers et al., 2007). Thus, qualitative methods were used, including unstructured interviews (preliminary, pre-design), semi-structured interviews (increments I, III, IV), a focus group session (increment II), and observation (increment IV). Preliminary interviews confirmed previous research and explored the first participating organisation. Participants (N = 5) described their activities related to skills intelligence, discussed data types and processes, and helped identify additional participants through snowball sampling.
The paper prototype was tested through expert interviews divided into three phases: introducing the initial framework and methodology, assessing the skills intelligence situation using paper cards, and answering survey questions on data quality. Quality scores were calculated, followed by a discussion of the procedure’s feasibility and usefulness.
The second artefact version, a Google Sheets and Forms prototype, was tested in a focus group session with four initial and two new participants (N = 6) from the same organisation. The session mirrored the expert interviews, including discussions on skills intelligence, a self-assessment form, and the improved assessment process using Google tools. Participants completed another self-assessment to gain insight into changes in their perceptions.
The third artefact version, consisting of wireframes for a skills intelligence management platform, focused on usability and effectiveness. Expert interviews with two previous and four new participants (N = 6) from different organisations evaluated the concept’s feasibility, identified UI/UX and other shortcomings, and suggested improvements.
The final functional software version was demonstrated through expert interviews or focus group sessions with two original and seven new participants (N = 9). In this phase, all demonstrations were organised over Microsoft Teams, and the participant(s) or the researcher shared the screen alternately. Participants used the software while the researcher observed, noting navigation times, confusing parts, and missing functionalities. In one case, a technical issue required screen sharing, with the researcher following the participant’s commands. At the end of the session, the participants answered questions addressing the artefact’s feasibility, effectiveness, usability and functionality.
Evaluation
The demonstration aimed to gather feedback to evaluate various aspects of the artefact, including feasibility, effectiveness, usability, and functionality, and to collect suggestions for improvement. Participants were asked targeted questions to explore their perception of the artefact.
Feasibility:
○ Participants discussed whether implementing the system in their organisation would be possible and how difficult it would be.
○ They described the benefits, obstacles, and challenges of implementation.
○ They reflected on the availability of resources (time, money, and people) in their organisation.
Usability:
○ Participants were asked about the intuitiveness and simplicity of the system.
○ They discussed the learning curve and whether they could use the system independently without the researcher’s guidance.
○ They described the challenges and misunderstandings encountered while using the system.
Effectiveness:
○ Participants evaluated whether the system solves the stated problems and how well it does so.
○ They addressed whether the system could help them achieve their goals better.
○ They were asked if they knew of any better analogues.
○ Functionality:
○ Participants discussed the functionalities they expected from the system.
○ They evaluated whether the system performed as anticipated.
○ They reported any errors they encountered.
Participants’ discussions and reflections on these areas highlighted both the strengths and areas for system improvement. The targeted questions helped us understand their perceptions of refining and enhancing the artefact. The following sections delve into an in-depth system evaluation, informed by the participants’ detailed feedback.
Feasibility
Participants were generally willing to use the system if the benefits were clear. Challenges included potential resistance and the need for training. Having a designated person in charge of skills intelligence management was seen as a crucial requirement for effective implementation. The system was considered less valuable in organisations where managers have fewer employees, and in two cases (a government organisation and one startup), it was not considered beneficial. Larger organisations are actively exploring potential applications of AI and ML in competency and HR-related practices, with the largest organisations already implementing these technologies. Participants from these organisations strongly agreed on the topic’s urgency and considered the artefact and methodology feasible stepping stones toward further advancements.
The potential for effective application was perceived to be higher if integrated with in-house tools: participants were worried that otherwise, the employees would have yet another piece of software where they would have to create accounts and remember their passwords.
In project-based organisations, strategic management, business unit leads, and salespeople showed more interest than HR or training managers due to their potential to increase the transparency of the organisation’s collective skillset. Many participants stressed that the system must comply with data privacy regulations and the European Union’s General Data Protection Regulation (GDPR) to be adopted. Feasibility evaluation results are summarised in Table 2.
Effectiveness
From the perspective of increasing skills intelligence in the organisation, the artefact was considered more effective in organisations with higher skills variability, which may be explained by the fact that higher skills variability leads to greater skills instability. On the other hand, participants from organisations with more firmly established technology and competency requirements found the artefact could have been more effective. This may be attributed to their lack of exposure to skills instability.
After the skills intelligence situation assessment, most participants felt better informed about increasing skills intelligence and the general situation in the organisation. However, their ability to systematically list skills intelligence-related activities decreased. The reasoning might be that they needed to be fully aware of the complexity of the topic prior to the assessment. A marginal improvement was reported in insight into colleagues’ skills in intelligence-related activities. To improve effectiveness, participants suggested including qualitative insights to supplement numerical data. Participants agreed that the system should be highly configurable. Multiple recommendations were made about the base information, e.g., processes, data types, and quality criteria.
From the broader perspective of reducing skills instability overall, participants were concerned that convincing organisations to share their statistics with other skills intelligence stakeholders could be challenging. It was suggested that incentives be explored to persuade organisations to contribute. Table 3 provides an overview of effectiveness evaluation results.
Usability
Usability was the most addressed topic during the interviews, and participants suggested many ideas for improvement. Some of these suggestions were contradictory. For example, while some participants found the skills intelligence situation assessment scoring matrix too bothersome and preferred to insert scores using a questionnaire, others felt that this would make the process too long and not provide a clear overview of all the scores they submitted. One participant recommended using gamification and sliders for scoring.
Participants suggested providing more detailed score descriptions and agreeing on definitions and terms. They also recommended integrating the system with existing tools or implementing single sign-on (SSO). Additionally, they proposed offering pre-made templates for a more straightforward setup. Most participants saw a need for a workshop to standardise terminology to avoid nomenclature barriers. Lastly, to avoid language barriers and promote inclusivity, it was suggested that localisation be implemented for users who need to be more fluent in English. Usability evaluation results are listed in Table 4.
Functionality
The most prominently reported inconsistency regarding functionality was the need for actual skills intelligence data collection functionality in the artefact. While participants understood that the framework and methodology focus on generating meta-data concerning skills intelligence-related data, many expected to be able to use the same platform for both purposes. Multiple participants stated that the platform should encompass all methodology phases, including target-setting, implementation, and sustainment. Additionally, they suggested including a dashboard to visualise all evaluation results compared to the defined targets. This feature would provide evidence of improvement and, in turn, increase motivation. Lastly, it was suggested that the platform should include a recommendations engine to assist with the roadmap in the planning phase. Based on the data types and quality criteria combinations in the situation assessment report, the system could use ML or AI principles to provide a clear plan for the organisation to improve their skills and intelligence.
In addition to contextual functionality evaluation, two expert interviews were conducted to evaluate the technological feasibility of the software. The application was subjected to black box testing to reveal inaccuracies or missing functioning in the software, errors in performance or data structures, and shortcomings in user experience. The interviews lasted one hour and were carried out by a senior quality engineer and a senior software engineer. The functionality evaluation results are summarised in Table 5.
Results and discussion
Skills intelligence management principles
The study contributed to knowledge by designing and developing a series of artefacts that addressed the research questions, which helped map the core principles for implementing skills intelligence management. The study identified five key principle categories for effectively implementing skills intelligence management: illustrate, clarify, tailor, support, and sustain, as illustrated in Table 6.
Most of these principles emerged as pervasive themes across the sub-artefacts. However, their application should consider the context: e.g. the subsequent activities differ when implementing skills intelligence management in general or developing supporting applications.
Other findings
Participants’ feedback confirmed the research problem’s relevance, highlighting that organisations face numerous challenges related to skills intelligence. One significant finding is that the term “skills intelligence” is not widely used in organisations – participants needed an explanation of the term. However, after being provided with examples, all participants reported that they understood the concept and encountered it daily, although under different names.
Participants affirmed that the importance of skills intelligence extends beyond the HR department. Insight into skills demand and supply is considered a strategic advantage for organisations. They agreed that inter-organisational cooperation in this area is increasingly vital. On several occasions, participants were surprised to learn that their coworkers had faced similar skills and intelligence challenges, which could be solved through cooperation.
Most participants acknowledged encountering skills intelligence challenges to varying degrees. While some viewed these challenges as minor inconveniences, others saw significant opportunities for improvement and had initiated plans to address potential solutions. Most agreed that a systematic approach to skills intelligence management would enhance its application on a broader scale within and across organisations. Establishing a formalised structure for collecting skills data would benefit the collective by laying a solid foundation for advanced statistics, forecasts, and other machine learning opportunities. Designated structures for improving skills intelligence also unify vocabulary and expand the term’s adoption. However, there are concerns regarding the broader scale of the research problem—reducing skills instability through advanced skills intelligence across labour market stakeholders. Participants were hesitant when asked whether their organisations would agree to share statistics from their improved skills and intelligence. It was recommended that possible incentives be explored to encourage them to agree.
Industrial implications
Standardising “skills intelligence” can facilitate better understanding and communication within and across organisations. The study confirms that skills intelligence is not just an HR concern but holds strategic importance for the entire organisation. Insight into skills demand and supply provides a critical market advantage, and participants agreed that inter-organisational cooperation in this area is becoming increasingly important. However, a broader conversation on the topic is necessary, as its importance has yet to be widely recognised.
Implementing the proposed framework could enhance organisational effectiveness and improve employee well-being by taking the first step towards high-quality skills intelligence in Big Data to enable evidence-based decision-making in workplace learning and organisational strategy. Effective implementation of the framework and its software platform offers opportunities for higher quality and more standardised data exchange and communication between organisations. This promotes collaboration among skills intelligence stakeholders to support lifelong learning.
Overall, this study not only contributes to knowledge generation but also has the potential to make a significant contribution to reducing skills instability by equipping organisations with practical tools to adapt to the changing landscape skills in the modern workplace by moving towards high-quality big data and employing novel technologies such as ML and AI for evidence-based decision making.
Limitations and future work
It is essential to acknowledge the limitations of the study. First, the study was constrained by a small sample size, which might limit the robustness and generalizability of the findings. Additionally, the absence of quantitative evaluation means that the outcomes rely heavily on qualitative assessments, which can introduce a degree of subjectivity. The generalizability and transferability of the results to different contexts has yet to be tested, as the research was primarily conducted within a few organisations, predominantly in the IT sector. This narrow focus raises the need to explore the broader applicability of the findings. A longitudinal study is necessary to fully understand the effects, benefits, and challenges associated with implementing the framework. Such an approach would provide valuable insights into how the framework performs over time and across various organisational contexts.
However, these limitations open new lines for future research. One potential area of inquiry involves applying and evaluating the framework and methodology in diverse organisational contexts across different domains, countries, and cultures. Additionally, while the current study relied primarily on expert interviews for evaluation and validation, a longitudinal study examining the framework’s application within an organisation could offer valuable insights into the dynamics and evolution of its implementation. From a practical standpoint, developing software artefacts using different technologies focusing on innovative human-computer interaction approaches could yield interesting results. One of the more interesting suggestions by the study participants was to include a recommendations engine that would apply ML or AI principles to the gathered meta-data and provide a roadmap for skills intelligence improvement or implementation. Finally, this research highlights that realising the full potential of skills intelligence in the labour market depends heavily on stakeholder cooperation. This presents an opportunity to investigate how data exchange and communication between organisations evolve after implementing effective skills intelligence management systems.
Conclusion
This paper has presented an artefact for skills intelligence management planning and evaluation in organisations, complete with an implementation methodology, skills intelligence situation evaluation instrument, and implementation principles. By drawing on participant feedback and an extensive review of current practices, we have developed a nuanced understanding of the challenges and opportunities for enhancing skills intelligence in the modern workplace.
Our analysis has highlighted several challenges organisations face regarding skills intelligence, including the sporadic and unstructured collection of skills-related data. Despite the increasing importance of skills intelligence for workforce adaptability, the term itself needs to be more widely recognised, indicating a need for broader dissemination and standardisation.
Participants affirmed that skills intelligence extends beyond HR, offering a strategic advantage. Inter-organisational cooperation emerged as a crucial factor, as many discovered that shared challenges could be addressed collaboratively. A systematic approach to skills intelligence management was essential for its effective application.
We have examined the current state of skills intelligence data collection and identified significant gaps and limitations. To address these, we recommend implementing a comprehensive framework that leverages high-quality skills intelligence Big Data for evidence-based decision-making. This would enhance organisational effectiveness and employee well-being by promoting standardised data exchange and communication.
Our study’s findings suggest that future research should apply the framework across diverse contexts to assess its generalizability, including longitudinal studies and exploring novel technologies for skills intelligence improvement. Developing software artefacts with innovative human-computer interaction approaches could yield interesting results. Participants suggested including a recommendation engine that applies ML or AI principles to the gathered meta-data to enhance skills intelligence.
However, concerns exist regarding the broader scale of reducing skills instability through advanced skills intelligence across labour market stakeholders. Participants were hesitant about sharing statistics generated from improved skills intelligence, indicating a need to explore incentives for encouraging such cooperation.
In conclusion, this research underscores the strategic importance of skills intelligence and highlights the necessity of stakeholder cooperation for its full realisation. While limitations such as a small sample size and a focus on the IT sector suggest further research, this study provides a foundation for future work to adapt to the changing skills landscape. By promoting a proactive, interdisciplinary approach and fostering greater collaboration, we can work towards a future where skills intelligence supports lifelong learning and organisational strategy.
Data availability statement
The datasets presented in this article are not readily available because the data is qualitative and very case-specific, concerning existing situations and attitudes in business organisations. The participants were ensured that the raw data will not be published. Requests to access the datasets should be directed to K-LK, a3VzbWluQHRsdS5lZQ==.
Ethics statement
Ethical approval was not required for the studies involving humans because the research did not include any vulnerable populations. The data collected for the study did not contain any sensitive data and the study posed very low risk for the participants. The studies were conducted in accordance with the local legislation and institutional requirements. Written informed consent for participation was not required from the participants or the participants’ legal guardians/next of kin in accordance with the national legislation and institutional requirements because the study was non-invasive, and the interviews and observations were carried out in a relaxed and familiar situation to the participants, to ensure that their feedback was natural. Verbal consent was acquired.
Author contributions
K-LK: Conceptualization, Data curation, Formal analysis, Funding acquisition, Investigation, Methodology, Project administration, Resources, Software, Supervision, Validation, Visualization, Writing – original draft, Writing – review & editing. PN: Conceptualization, Supervision, Writing – original draft. TL: Conceptualization, Supervision, Writing – original draft.
Funding
The author(s) declare that no financial support was received for the research, authorship, and/or publication of this article.
Conflict of interest
The authors declare that the research was conducted in the absence of any commercial or financial relationships that could be construed as a potential conflict of interest.
Publisher’s note
All claims expressed in this article are solely those of the authors and do not necessarily represent those of their affiliated organizations, or those of the publisher, the editors and the reviewers. Any product that may be evaluated in this article, or claim that may be made by its manufacturer, is not guaranteed or endorsed by the publisher.
Footnotes
References
Angrave, D., Charlwood, A., Kirkpatrick, I., Lawrence, M., and Stuart, M. (2016). HR and analytics: why HR is set to fail the big data challenge. Hum. Resour. Manag. J. 26, 1–11. doi: 10.1111/1748-8583.12090
Bonen, T., and Loree, J. (2021). How to forecast skills in demand: a primer. Future skills Centre. Available at: https://fsc-ccf.ca/wp-content/uploads/2021/04/How-to-Forecast-Skills-In-Demand-Report-April2021-EN.pdf
Cedefop. (2019). Crafting skills intelligence. European Centre for the Development of vocational training. Available at: https://www.cedefop.europa.eu/en/blog-articles/crafting-skills-intelligence
DiRomualdo, A., El-Khoury, D., and Girimonte, F. (2018). HR in the digital age: how digital technology will change HR’s organization structure, processes and roles. Strateg. HR Rev. 17, 234–242. doi: 10.1108/shr-08-2018-0074
European Commission. (2016). A new skills agenda for Europe: Working together to strengthen human capital, employability and competitiveness (COM(2016) 381 final). Available at: https://eur-lex.europa.eu/legal-content/EN/TXT/?uri=CELEX%3A52016DC0381
Cedefop. (2020a). European skills agenda for sustainable competitiveness, social fairness and resilience. Available at: https://ec.europa.eu/social/BlobServlet?docId=22832&langId=en
Cedefop (2020b). Osnabruck declaration on vocational education and training as an enabler of recovery and just transitions to digital and green economies. Thessaloniki: Author. Available at: https://www.cedefop.europa.eu/files/osnabrueck_declaration_eu2020.pdf (Accessed July 24, 2024).
European Commission. (2021). 2030 digital compass: The European way for the digital decade. Available at: https://eur-lex.europa.eu/legal-content/EN/TXT/HTML/?uri=CELEX:52021DC0118
Garcia-Arroyo, J., and Osca, A. (2021). Big data contributions to human resource management: a systematic review. Int. J. Hum. Resour. Manag. 32, 4337–4362. doi: 10.1080/09585192.2019.1674357
Gartner Inc. (2020). Do more with data to close critical skill gaps. In Gartner TalentNeuron. Available at: https://emt.gartnerweb.com/ngw/globalassets/en/human-resources/documents/trends/leveraging-skills-adjacencies.pdf
ISACA. (2018). COBIT 2019 framework-introduction and methodology. Available at: www.icasa.org/COBITuse
Kusmin, K.-L., Künnapas, T., Ley, T., and Normak, P. (2019). Perception of Industry 4.0 competency challenges and workplace TEL in the Estonian manufacturing industry In Transforming Learning with Meaningful Technologies. Eds. M. Scheffel, J. Broisin, V. Pammer-Schindler, A. Ioannou, and J. Schneider (Cham: Springer International Publishing), 678–682.
Kusmin, K.-L., Ley, T., and Normak, P. (2017). “Towards a data driven competency management platform for industry 4.0” in Proceedings of the workshop papers of i-know (Vol. 2025, issue 1). CEUR workshop proceedings. eds. M. Ähnelt, O. Bluder, G. Breitfuss, R. Kaiser, R. Kern, and R. Klamma, et al. Available at: https://ceur-ws.org/Vol-2025/paper_hci_4.pdf
Kusmin, K.-L., Tammets, K., and Ley, T. (2018). University-industry interoperability framework for developing the future competences of industry 4.0. IxD & A Interact. Design Architec. 38, 28–45. doi: 10.55612/s-5002-038-002
Lalitha Kavya, M., Sowdamini, T., and Pande, A. (2023). Digital Human Resource Transformation - A Bibliometric Analysis. Acta Univ. Bohem. Merid. 26, 95–120. doi: 10.32725/acta.2023.011
Maldonado-Mariscal, K., Cuypers, M., Götting, A., and Kohlgrüber, M. (2023). Skills intelligence in the steel sector. Mach. Des. 11:335. doi: 10.3390/machines11030335
Méndez-Domínguez, P., Carbonero Muñoz, D., Raya Díez, E., and Castillo De Mesa, J. (2023). Digital inclusion for social inclusion. Case study on digital literacy. Front. Commun. 8:1191995. doi: 10.3389/fcomm.2023.1191995
Micheli, G. J. L., Martino, A., Porta, F., Cravello, A., Panaro, M., and Calabrese, A. (2023). Workforce planning in project-driven companies: a high-level guideline. In Frontiers in Industrial Engineering. Vol. 1. Frontiers Media SA. doi: 10.3389/fieng.2023.1267244
Patil, B. S., and Priya, M. R. S. R. (2024). HR data analytics and evidence based practice as a strategic business partner. Vilakshan-XIMB J. Manag. 21, 114–125. doi: 10.1108/xjm-07-2023-0148
Peffers, K., Tuunanen, T., Rothenberger, M. A., and Chatterjee, S. (2007). A design science research methodology for information systems research. J. Manag. Inf. Syst. 24, 45–77. doi: 10.2753/MIS0742-1222240302
Popo–Olaniyan, O., Elufioye, O. A., Okonkwo, F. C., Udeh, C. A., Eleogu, T. F., and Olatoye, F. O. (2022). Ai-driven talent analytics for strategic hr decision-making in the United States Of America: A Review. Int. J. Manag. Enter. Res. 4, 607–622.
Rentzsch, R., and Staneva, M. (2020). Skills-Matching and Skills Intelligence through curated and data-driven ontologies. In Proceedings of the DELFI Workshops (Bonn: Gesellschaft für Informatik eVz), 46–58.
Ruiz-Calleja, A., Prieto, L. P., Ley, T., Rodríguez-Triana, M. J., and Dennerlein, S. (2022). Learning analytics for professional and workplace learning: A literature review. In Data Driven Approaches in Digital Education: 12th European Conference on Technology Enhanced Learning, EC-TEL 2017, Tallinn, Estonia, 2017 September 12–15, Proceedings 12 (Springer International Publishing) 164–178.
Tammets, T., and Ley, T. (2023). Integrating AI tools in teacher professional learning: a conceptual model and illustrative case. In Frontiers in Artificial Intelligence (Vol. 6). Frontiers Media SA. doi: 10.3389/frai.2023.1255089
Teddlie, C., and Yu, F. (2007). Mixed methods sampling: a typology with examples. J. Mix. Methods Res. 1:NP1. doi: 10.1177/2345678906292430
Keywords: skills intelligence, skills instability, organisation management, organisational communication, evidence-based decision making, decision support system
Citation: Kusmin K-L, Normak P and Ley T (2024) A methodology for planning, implementation and evaluation of skills intelligence management – results of a design science project in technology organisations. Front. Artif. Intell. 7:1424924. doi: 10.3389/frai.2024.1424924
Edited by:
Asif Gill, University of Technology Sydney, AustraliaReviewed by:
Yehia Alzoubi, American University of the Middle East, KuwaitSultana Lubna Alam, Deakin University, Australia
Copyright © 2024 Kusmin, Normak and Ley. This is an open-access article distributed under the terms of the Creative Commons Attribution License (CC BY). The use, distribution or reproduction in other forums is permitted, provided the original author(s) and the copyright owner(s) are credited and that the original publication in this journal is cited, in accordance with accepted academic practice. No use, distribution or reproduction is permitted which does not comply with these terms.
*Correspondence: Kadri-Liis Kusmin, a3VzbWluQHRsdS5lZQ==