- Institute of Work Science, Ruhr University Bochum, Bochum, Germany
Purpose: The discourse on the human-centricity of AI at work needs contextualization. The aim of this study is to distinguish prevalent criteria of human-centricity for AI applications in the scientific discourse and to relate them to the work contexts for which they are specifically intended. This leads to configurations of actor-structure engagements that foster human-centricity in the workplace.
Theoretical foundation: The study applies configurational theory to sociotechnical systems’ analysis of work settings. The assumption is that different approaches to promote human-centricity coexist, depending on the stakeholders responsible for their application.
Method: The exploration of criteria indicating human-centricity and their synthesis into configurations is based on a cross-disciplinary literature review following a systematic search strategy and a deductive-inductive qualitative content analysis of 101 research articles.
Results: The article outlines eight criteria of human-centricity, two of which face challenges of human-centered technology development (trustworthiness and explainability), three challenges of human-centered employee development (prevention of job loss, health, and human agency and augmentation), and three challenges of human-centered organizational development (compensation of systems’ weaknesses, integration of user-domain knowledge, accountability, and safety culture). The configurational theory allows contextualization of these criteria from a higher-order perspective and leads to seven configurations of actor-structure engagements in terms of engagement for (1) data and technostructure, (2) operational process optimization, (3) operators’ employment, (4) employees’ wellbeing, (5) proficiency, (6) accountability, and (7) interactive cross-domain design. Each has one criterion of human-centricity in the foreground. Trustworthiness does not build its own configuration but is proposed to be a necessary condition in all seven configurations.
Discussion: The article contextualizes the overall debate on human-centricity and allows us to specify stakeholder-related engagements and how these complement each other. This is of high value for practitioners bringing human-centricity to the workplace and allows them to compare which criteria are considered in transnational declarations, international norms and standards, or company guidelines.
1. Introduction
Human-centered and responsible artificial intelligence (AI) applications are of key concern in current national and trans-national proposals for declarations and regulations, such as the US Blueprint AI Bill of Rights or the EU AI Act, of norming initiatives of the International Organization for Standardization (ISO), and of company guidelines, e.g., the Microsoft Responsible AI declaration or SAP’s Guiding Principles for AI. At the same time, there is an academic-driven research debate to which different research communities contribute. This article sheds light on the criteria of human-centricity and how they are considered in academic publications. Whether and how they are treated in political declarations and industry norms will be part of the discussion.
Scholars elaborate on the meaning of human-centricity either of AI as a technology (Zhu et al., 2018; Ploug and Holm, 2020; How et al., 2020b), AI applications related to the work context (Jarrahi, 2018; Wilson and Daugherty, 2018; Gu et al., 2021), or job characteristics of work contexts in which AI applications are implemented (Romero et al., 2016; Kluge et al., 2021; Parker and Grote, 2022). Systematic overviews on these criteria show that contributing researchers are from a wide range of disciplines and include certain use fields such as healthcare, manufacturing, education, or administration, as well as the work processes of software development itself (Wilkens et al., 2021a). The thematic foci vary depending on the discipline and field of use. While researchers from the human-computer interaction (HCI) community describe human-centered AI as an issue of AIs’ trustworthiness and related safety culture (Shneiderman, 2022) and thus combine technological characteristics with organizational characteristics, researchers in psychology consider human-centricity as an issue of job design where AI applications support operators’ authority and wellbeing (e.g., De Cremer and Kasparov, 2021), which means that they combine organizational and individual characteristics. Researchers in engineering and manufacturing most likely address AI-based assistance to compensate for individual weaknesses in the production flow (Mehta et al., 2022) and thus relate technological and organizational characteristics to the individual, but with another concept of man than prevalent in psychology (Wilkens et al., 2021a). The number of coexisting definitions emphasizing different criteria can easily be interpreted as contradictory or controversial. We ask whether there is a system that allows us to relate different criteria to each other from a higher order. Reflecting on human-centricity requires a consideration of the perspectives on human-AI interaction (Anthony et al., 2023), the context characteristics of where AI is in use (Widder and Nafus, 2023), the individual demands of employees who are confronted with technology, and the responsibilities of stakeholders who are in charge of it (Polak et al., 2022). This is why we apply configurational theory (Mintzberg, 1993, 2023) to the meaning of the human-centricity of AI at work.
Basically, AI is a term for software applications dedicated to detecting patterns based on neural networks and various machine learning (ML) algorithms nowadays, aiming at copying human intelligence on a computational basis but without any parallel to human intelligence in terms of the underlying learning process (Wilkens, 2020; Russell and Norvig, 2021). The characteristics of AI evolve with the different waves of technology development (Launchbury, 2017; Xu, 2019), and definitions change accordingly. AI applications from the second wave of AI development can be described as pre-trained and fine-tuned machines having “the ability to reason and perform cognitive functions such as problem-solving, object and word recognition, and decision-making” (Hashimoto et al., 2018, p. 70). In the current third wave, scholars emphasize artificial general intelligence in terms of “intelligent agents that will match human capabilities for understanding and learning any intellectual task that a human being can” (Fischer, 2022, p. 1). Conversational Large Language Models give an example in this direction, and the high-speed dissemination of the non-licensed version of ChatGPT III shows that generative AI is not necessarily officially implemented in a work context but is prevalent due to high individual user acceptance, leading to continuous application in operational tasks. This challenges all fields of the private and public sectors and fosters the need to specify and reflect on the criteria of human-centricity against the background of technology development on the one hand and the characteristics of the use fields on the other. Current state-of-the-art research argues that there is a need for a contextualized understanding of AI at work and corresponding research methods (Anthony et al., 2023; Widder and Nafus, 2023). We transfer this consideration to the reflection on the human-centricity of AI, as the technology only belongs to work contexts while being promoted by a group of incumbents.
The research community in organization studies is well known for context-related distinctions, avoiding one-best-way or one-fits-all thinking. Scholars rather search for typologies under which conditions and characteristics matter most and thus lead to contextualized understandings of challenges and related performative practices (Miller, 1986; Mintzberg, 1993, 2023; Greckhamer et al., 2018). This consideration has already been applied to the first reflections on human-centered AI in work contexts (Wilkens et al., 2021b), but definitions of human-centricity often claim to be universal or at least disregard the contextual background they have been stated for. Our argument is that different definitions and criteria of human-centricity result from different research communities or peer groups with different use fields, functions, or responsibilities explicitly or implicitly in mind. This includes considerations like who is in charge of promoting a criterion in concrete developments and operations.
A configurational approach is proposed to be helpful in understanding from a higher order when a criterion of human-centricity is highlighted for generating solutions and when it can be subordinated or neglected in the face of specific context-based responsibility. Our aim of analysis is to identify typical configurations of human-centered AI in the organization and to specify and distinguish the meaning and relevance of human-centricity against the background of who is in charge of a specific work context. A deep understanding of context requires ethnographic research (Anthony et al., 2023; Widder and Nafus, 2023) but can be systematically prepared by a cross-disciplinary literature review, giving attention to contexts and determining which community emphasizes which criteria and why. This contributes to a common ground in theory development on human-centered AI as it enables systematizing various findings from the many research communities elaborating on this topic. It also provides practitioners with guidance in deciding which criteria matter most for which purpose and peer group and allows them to estimate when to focus on selected criteria and when to broaden their perspective while taking alternative views.
A reflection on human-centricity in connection with AI and work is a sociotechnical system perspective by its origin, as the three entities of technology, human agency, and organization with their institutional properties are interrelated (Orlikowski, 1992; Strohm and Ulich, 1998). How a sociotechnical system perspective can be combined with a configurational approach will be outlined in the next section. In the third section, we explain the research method of a systematic literature review, including search strategy and data evaluation. Based on this, we outline the research findings first by an analytical distinction of eight criteria of human-centricity and, in the second step, by contextualizing and synthesizing them to seven configurations of actor-structure engagements. The concluding discussion and outlook feeds the results back to norming initiatives and emphasizes further empirical validation in future research.
2. Configurational perspective on human-centered AI in sociotechnical systems
Configurational theory is an approach among scholars in organizational studies that focuses on the distinction of typologies. Typologies are based on “conceptually distinct [organizational] characteristics that commonly occur together” (Meyer et al., 1993, p. 1175; see also Fiss et al., 2013). The analysis is related to equifinality by explaining episodic outcomes instead of separating between independent and dependent variables, which is nowadays also described as causal complexity by scholars promoting a neo-configurational approach (Misangyi et al., 2017). This is how and why configurational thinking is distinguished from contingency theory, which is drilled to find a context-related best fit between organizational practices and external demands (Meyer et al., 1993). Configurational theory calls for alternative qualitative research methods and initiates its own movement in data analysis (Fiss et al., 2013; Misangyi et al., 2017).
Mintzberg (1979, 1993, 2023) is one of the most well-known researchers in configurational theory, with a distinction between structurational configurations originally known as structure in fives (Mintzberg, 1979, 1993) and recently readjusted while giving more attention to stakeholders and agency in addition to structural characteristics. Mintzberg (2023) outlines seven configurations deduced from the impact of five actor groups in terms of operators, middle managers, C-level managers, support staff and analysts, experts for standardizing the technostructure, as well as organizational culture, and external stakeholders such as communities, governments, or unions.
The core idea is that organizations can activate different mechanisms of coordination, communication, standardization, decentralization, decision-making, and strategizing to gain outcomes and that there is no one best way to do it. The diagnosis and understanding of the organizational mechanisms of being performative are crucial for activating them. From a research point of view, it is interesting to note that organizations can, however, be clustered and distinguished by configurations that represent ideal types of success while gaining a specific organizational shape (Mintzberg, 1979, 2023).
The configurational theory was originally focused on the analysis and description of organizational characteristics but was also supposed to serve as a framework for the analysis of the individual and group level, respectively, a “sociotechnical systems approach to work group design” (Meyer et al., 1993, p. 1186; see also Suchman, 2012). This is exactly how Orlikowski (1992) explained sociotechnical systems with three interrelated entities: technology, human actors, and the organizational institutional context. From this perspective, technology is not a context-free object but is interpreted and enacted by human agents under organizational characteristics, which also leads to different meanings of technology when applied to and enacted in different settings (Orlikowski, 2000, 2007). The inseparability between social and technological entities was later described as entanglement and sociomateriality (Orlikowski and Scott, 2008; Leonardi, 2013).
However, configurational theory and methods are not very common in sociotechnical system analysis and can only be loosely applied by a few scholars (e.g., Pava, 1986; Badham, 1995). A reason might be that the approach gained great attention in organization studies but is often counterintuitive to the research methods applied in engineering and psychology, both disciplines with a strong emphasis on causality and linear thinking, which are adjoining disciplines elaborating on sociotechnical system thinking but with distinct research traditions and methods in use (Herzog et al., 2022). It is interesting to note that the detection of patterns is a mutual interest between ML approaches and organizational configurational theory but that the system-dynamic-based acyclic thinking of configurational theory is untypical of how ML methods currently work.
The reason we suggest elaborating on a configurational approach is that there is no single or prior group in charge of a human-centered AI application in work settings; instead, many disciplines and stakeholders involved from different levels of hierarchy and professions from inside and outside the organization contribute to the same topic. Consequently, there is a high plausibility that different approaches and stakeholders contribute to human-centricity and that there is no one best way or mastermind orchestration but different ways of enacting selected criteria dedicated to the human-centricity of AI at work. This is why we aim to explore these configurations and reflect them as a starting point to enhance the human-centricity of AI in organizations with respect to their contributions and limitations.
3. Literature review on the human-centricity of AI at work
3.1. Search strategy and data evaluation
To identify the most typical configurations in current academic writings and underlying fields of AI application, it is necessary to include a wide range of publications in the search strategy and to analyze the research contributions as systematically as possible. Since research on human-centered AI or work with AI is not limited to the management field but also includes disciplines such as work science, psychology, medicine, computer and information science, or even philosophy and sociology, we conduct a cross-disciplinary literature review with a systematic search strategy (Snyder, 2019). As a starting point, we use the 79 articles already identified from the review by Wilkens et al. (2021a), leading to the distinction of five criteria regarding trustworthiness and explainability, compensating individual deficits, protecting health, enhancing individual potential, and specifying responsibilities. Aligned with the guidelines of Page et al. (2021; see also Figure 1), we then systematically searched the Scopus and Web of Science databases for the keywords “human-centered” or “human” and “artificial intelligence” or “AI,” as well as various synonyms, spellings, and their German translations. To consider the different publication strategies of the targeted disciplines, we included books, book chapters, journal articles, and conference papers and did not focus on discipline-specific journal ratings. By using boolean operators, we were able to identify a total of 715 additional articles. In the set of articles, we included all English and German language results but excluded articles in other languages that only had an English abstract or those that have not yet been published. In the second step, we screened all articles based on their abstracts and checked whether they contributed directly or indirectly to work to exclude those contributions with a pure focus on human-centered technology but without even an indirect reference to work. We also excluded papers with a pure interest in humanoid robots but without any interest in human-centered work. A human-technical focus facing technical design differed from a sociotechnical perspective and was therefore eliminated for the purpose of our analysis. However, the indirect reflection of work seemed to be of high relevance, which means that we did not exclude contributions when it became obvious that authors consider the technology relevant for future work settings or if they describe the work process of software development itself even though they do not name it work. In the third step, we delved deeper and analyzed the articles based on their full texts. We excluded all articles that only mentioned the relevant keywords in the title or abstract but did not discuss them in detail in the text. This search strategy resulted in a total set of 101 articles, of which 70 followed a theoretical-conceptual approach and 31 an empirical approach. Most of the authors of the articles were from the fields of computer science and engineering. However, due to the interdisciplinary scope of the articles, they were complemented by co-authors from the fields of management studies, psychology, ergonomics, and social science, as well as healthcare and education, to mention the most common backgrounds of co-authors.
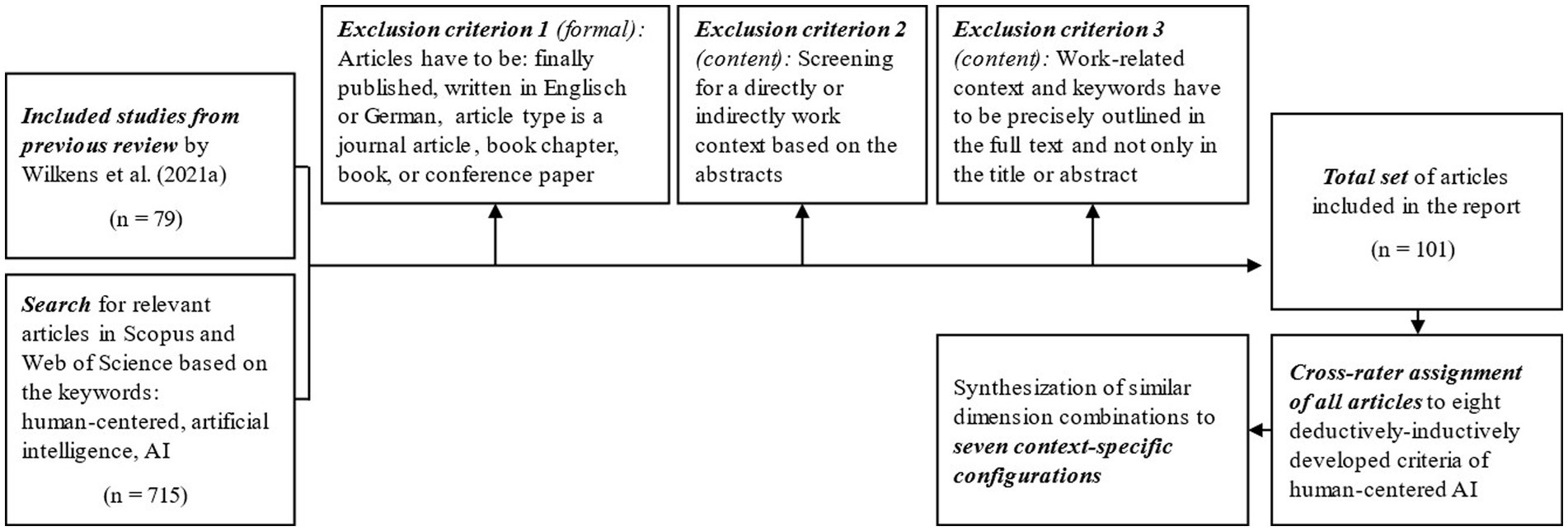
Figure 1. Flow diagram of the search strategy process, according to Page et al. (2021).
Since we do not aim to quantify the literature but are interested in the underlying structure of its content, we followed a content analysis approach while analyzing the literature (Kraus et al., 2022). This involved reading the articles in their entirety by the authors and identifying dimensions of human-centered AI at work or human-centered work with AI. Therefore, the overall data evaluation process was twofold. The first step was analytical and aimed at the specification of dimensions and criteria indicating different meanings of human-centricity while working with AI. Here, we followed a deductive-inductive approach and used the five categories explored by Wilkens et al. (2021a) as deductive starting points and complemented and redefined them in several stages with cross-rater validation among all three authors by further inductively explored categories. Distinctions between categories are made when there are different meanings reflecting the underlying aim and intent of a human-centered approach. Homogeneity in intent and debate leads to a single category. Separable debates lead to the proposition of a further category (see Table 1). As a first result, we specified eight criteria for human-centered AI at work or working with AI.
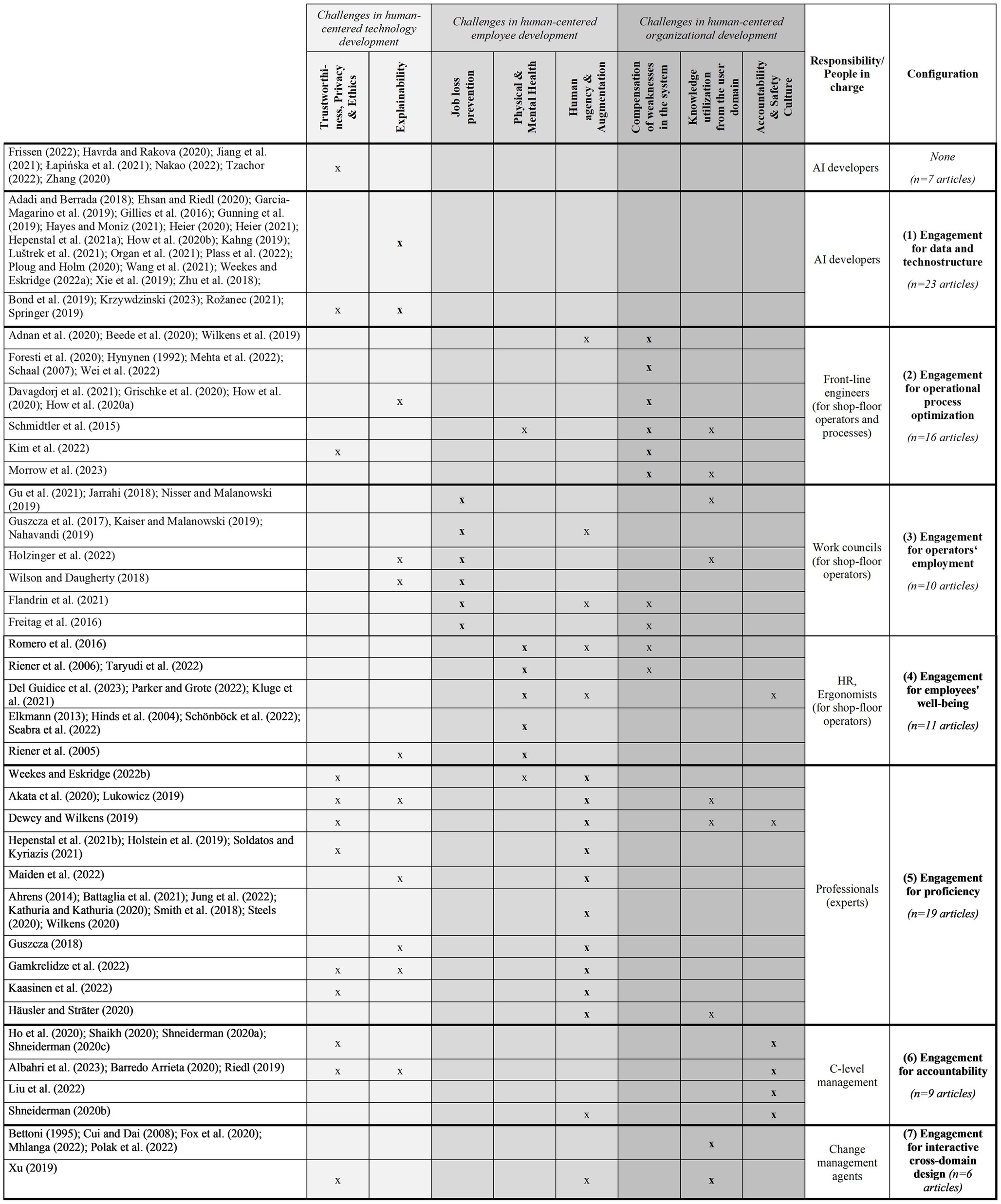
Table 1. Assignment of the articles from the literature review to the human-centered AI criteria and their condensation into configurations.
The second step of analysis reflected the analytically separated criteria and synthesized them into seven configurations. The synthesis results from (1) the coincidence of criteria related to a dominant criterion while reflecting (2) the actor groups in charge of the application of the set of criteria. Publications were systematized and finally assigned to a configuration against this background. To give an illustration with selected examples for the treatment of dominant and supporting criteria: Weekes and Eskridge (2022a) emphasized technological characteristics for fostering explainability but went further in a second publication (Weekes and Eskridge, 2022b) on “Cognitive Enhancement of Knowledge Workers,” in which they reflect human agency and augmentation. This is why the same authors can be represented in different configurations by different publications—in this case, in the engagement for data and technostructure with the first paper and the engagement for proficiency with the second paper. In their second study, Weekes and Eskridge (2022b) also referred to individual health and trustworthiness as subordinate criteria to the dominant one. Romero et al. (2016) overlapped with them in the overall set of criteria, but employees’ physical and mental health were in the foreground, while the optimization of operational processes and human agency are considered supporting criteria. Therefore, Romero et al. (2016) were assigned to the engagement for employees’ wellbeing. Even though authors overlap on two criteria, the focus of the article and the dominant perspective can differ (see Table 1).
The synthesis also includes the stakeholders in charge of a criterion and, respectively, the surrounding criteria. People in charge are not always explicitly mentioned but sometimes remain implicit. To gain access to the implicit assumptions, Mintzberg’s organizational actors’ description (Mintzberg, 2023, p. 17) serves as a blueprint for specifying the addressed audience. To add an illustration for this challenge, Shneiderman (2020a,b,c) stresses accountability and safety culture as important issues in human-centered AI, but without naming responsible actors. However, from a contextualized organizational understanding, it is obvious that this is an overall C-level responsibility and that the top management team can be specified as the actor in charge.
Once the (1) coincidence of criteria and the (2) actor groups in charge are identified, it becomes apparent that the eight criteria of human-centered AI at work result in seven configurations. Considering the distribution of the criteria according to the frequency of their occurrence per configuration, the relative weighting reveals that each of the seven configurations is based on one dominant criterion, most likely surrounded by one or two other criteria, which reinforces the synthesis into seven configurations (see Table 2). Adding total numbers to the configurations, we observed that there were 23 reviewed publications with a core emphasis on the first configuration, the engagement for data and technostructure, 16 with an emphasis on the second engagement for operational process optimization, 10 with an emphasis on the third engagement for operators’ employment, 11 with an emphasis on the fourth engagement for employees’ wellbeing, 19 with an emphasis on the fifth engagement for proficiency, 9 with an emphasis on the sixth engagement for accountability, and 6 with an emphasis on the seventh engagement for interactive cross-domain design. A smaller number of publications related to a configuration does not indicate a lower relevance but only that there is currently less emphasis on the criterion or that the overall research community elaborating on a specific configuration is smaller. The differences in the distribution can rather be interpreted as a sign that relevant criteria, e.g., facing challenges in organizational development, can easily be overseen if the group of scholars representing them stands behind the dominant discourse with another emphasis, e.g., facing challenges in technology development.
3.2. Criteria of human-centered AI and how they lead to configurations
We identified eight criteria of human-centricity; two of them were discussed as challenges of human-centered technology development, three of them as challenges of human-centered employee development, and three of them as challenges of human-centered organizational development (see Table 1). A broader group of scholars asks how reliable and supportive AI-based technology is for individual decision-making and operations. They face the challenges of human-centered technology development with two criteria that are of key concern. The criterion of trustworthiness, privacy, and ethics means that the data structure is unbiased and that there is no ethical concern with respect to collecting and/or using the data. The goal is for AI to operate free from discrimination and provide reliable and ethical outcomes. The criterion of explainability means that the technology provides transparency about the data in use, how they are interpreted, and what error probability remains when using AI for decision support. The aim here is to enhance technology acceptance while giving helpful information to the user. Even though both criteria relate to the same challenges of the data structure, which is why they were comprised by Wilkens et al. (2021a), the underlying aim and intent differ in such a way that we propose to treat them separately.
Another group of scholars faces the challenges of human-centered employee development. The coding process explored three criteria. The first criterion results from an overall debate primarily addressed in social science. It is the prevention of job loss. Empirical findings show that new technologies, as well as digitalization and AI, lead to an increase in jobs at the level of economies, and a specific group of jobs, e.g., standardized tasks in manufacturing, logistics, or administration, can be reduced (Petropoulos, 2018; Arntz et al., 2020). As a single employee or group of employees might suffer these effects, the criterion can matter at the company level, which leads to the discourse of preventing employees from negative consequences due to new technologies. With the criterion of physical and mental health, scholars give emphasis to the protection of employees while aiming at preventing them from negative influences such as heavy loads, chemical substances, stressful interactions, etc., which they have to cope with while performing operational tasks. This is a group of scholars with a background in ergonomics and a stable category that already occurred in the review from Wilkens et al. (2021a). The criterion of human agency and augmentation is a further stable outcome of the coding process. The category is taken into consideration across certain disciplines. The meaning is to design and use technology in such a manner that employees are in control of the technology (Legaspi et al., 2019) while performing tasks in direct interaction with AI and experiencing empowerment and further professionalization through the human-AI interaction.
A third overall dimension is related to the challenges of human-centered organizational development. The meaning of human-centricity is to reflect human needs and potentials, as well as weaknesses and negligence, to keep systems and interactions going and make them safe and reliable. One criterion is the compensation of weaknesses and system optimization. This explores a rather deficit-oriented perspective on the human being because of fatigue, unstable concentration, or limits in making distinctions on the basis of human sensors. AI is considered an approach to compensate for these weaknesses (Wilkens et al., 2021a). However, this is not for drawing a rather negative picture of the human being but to keep the system working and optimize processes where there would otherwise be negative system outcomes. The aim of this human-centered approach is high precision, failure reduction, high speed, and high efficiency. The criterion of integration of user domain knowledge gives attention to the connection between the domain of software development and the user domain. More traditionally, this is user-centered design and tool development, an approach that has been advocated for almost 30 years (see Fischer and Nakakoji, 1992). In current further development, it is not primarily the end-user need but the integration of user domain knowledge in the software development process to make the technology better and more reliable on the system level due to feedback loops between these domains and the expertise resulting from user domain knowledge. The clue is higher proficiency in technology development through job design principles across domains. Finally, there is the criterion of accountability and safety culture based on the meaning of human-centricity: a long-term benefit from AI requires reliable systems and organizational routines that guarantee this reliability. The goal is to provide and implement clear process descriptions and checklists that foster high levels of responsibility at the system level.
These eight criteria related to three dimensions can comprise seven contextualized configurations of an actor-structure engagement, specifying who is in charge of fostering what criteria, the (acceptable) limitations of the approach, and the need to elaborate on a broader view of the system level. While all seven configurations are each based on a dominant criterion, one criterion represents an exception. Trustworthiness, privacy, and ethics support almost all configurations and can thus be classified as a necessary overall condition (see Table 2; Wilkens et al., 2021b).
Note: Percentages indicate the distribution of the human-centered AI criteria per configuration. The weighting is based on the absolute number of articles assigned to the dimensions.
The configuration (1) engagement for data and technostructure identified from 23 publications under leading authorship from computer science is based on the criterion explainability of AI and is often brought by AI developers in charge of technical applications from outside the user domain to the specific workplace. This criterion is supported by trustworthiness, privacy, and ethics. The impact from outside the organization includes a wide range of industries, from manufacturing, business, healthcare, and education to the public sector. The quality of the technology itself is an issue of human-centricity, but without reflecting other criteria with respect to the employee or organizational development of the absorbing organizations. This means that high-end technology affects the standards and technostructure of other organizations without considering the consequences. However, those who develop technology have a guideline for keeping the developed tool’s quality as high as possible.
The second configuration detected from 16 publications is the (2) engagement for operational process optimization. Those who are in charge face the challenges of organizational development with respect to operators’ workflows. The primary criterion is the compensation of weaknesses for high system outcomes in terms of accuracy, quality, and efficiency. Authors in engineering are prevalent in this class. A combination of employee development-related criteria occurs in some writings, but the contextualized approach is dedicated to process design. The responsibility is especially taken by line management engineers who follow design principles for optimizing system outcomes while compensating for human weaknesses with the help of sophisticated technology.
The third configuration is (3) engagement for operators’ employment with a key criterion of preventing employees, especially front-line shop-floor operators, from job loss, which could be explored in 10 publications from interdisciplinary author groups. This approach to human-centricity is often discussed as the back side of the medal when the technostructure or the optimization of operational processes—both configurations were just outlined—are considered in an isolated manner. This perspective gives prior emphasis to employee development and is also surrounded by further criteria related to technology or organizational development. Those who are in charge, e.g., work councils from inside the organization or unions from outside, aim at keeping employment within a company high—often not just as a means but also as an end. Those who feel responsible for keeping employment high within the company have a starting point for their inquiry and also an approach to further criteria fostering operators’ employment.
The fourth configuration prevalent in 11 publications is the (4) engagement for employees’ wellbeing, emphasizing physical and mental health, especially of operators. Co-authors represent this expertise. Their focus is enriched by further criteria related to employee or organizational development. Technology is often not specified in this configuration but is prevalent as an initial point to reflect on human-centricity. Another crucial point is that the whole job profile—and not just a single task—is reflected against the background of AI applications. The groups proposed to be in charge of this configuration are HR staff members or ergonomists.
With the fifth configuration, (5) engagement for proficiency, deduced from 19 publications with authors from a wide range of disciplines, the focus shifts from operators, often considered shop-floor operators, to different individual experts within the organization who are responsible for decision-making and solutions with critical impact, e.g., in medical diagnosis, surgeries, or business development. These experts are often at the medium or top level within the organization. The key criterion is human agency and augmentation, most likely supported by the criteria of trustworthiness and explainability of AI. The issue is hybrid intelligence for specific tasks and decisions, not necessarily whole job profiles. The addressed experts are often not organized by others or confronted with new technology but decide its application themselves. This is why they can focus on the quality of the technology and the outcome for their individual profession, often at the middle level of an organization.
The sixth configuration, (6) engagement for accountability, with an underlying number of nine publications from different disciplines, further shifts the focus to the C-level managers in charge of decisions affecting the overall organizational development. It is the accountability and safety culture, especially at critical interfaces within and across organizations, that is the key criterion for this configuration of human-centricity. The criterion is often enriched by the trustworthiness of the AI application. This underlines that the top management team pursues other criteria of human-centricity than, e.g., the work councils or HR managers.
The final configuration was detected in six publications situated in different disciplines: (7) Engagement for interactive cross-domain design faces another challenge of organizational development: knowledge utilization from the user domain in the process of AI development. This perspective currently gains great attention in co-creation and co-design research (Russo-Spena et al., 2019; Li et al., 2021; Suh et al., 2021). In the search field of human-centered AI, the perspective is rather new and currently leads to a bi-directional exchange of knowledge to reach high reliability and safety for AI applications. This configuration is of key concern for work processes in software development companies and user domain firms. It is especially organizational development or change management experts who take responsibility for this perspective and criterion. This configuration builds bridges to the first configuration and aims at AI applications that are adaptable to a firm’s standards and technostructure and thus also avoid negative side effects, as especially anticipated in the second and third configurations of operational process optimization and operators’ employment.
A configuration is related to the fields of responsibility of organizational internal or external stakeholders who are in charge of human-centered outcomes in a sub-field of an overall process or design. This is why the identified configurations can be specified and aligned to Mintzberg’s (2023) actor-structure constellations (see Figure 2). The search for configurations revealed that no mastermind covers all criteria when contextualizing the human-centricity of AI at work but that each criterion needs to be advocated by responsible stakeholders. This leads to distinct approaches across hierarchy and expertise within organizations and makes it challenging to fulfill the overall mission of human-centered AI in the workplace.
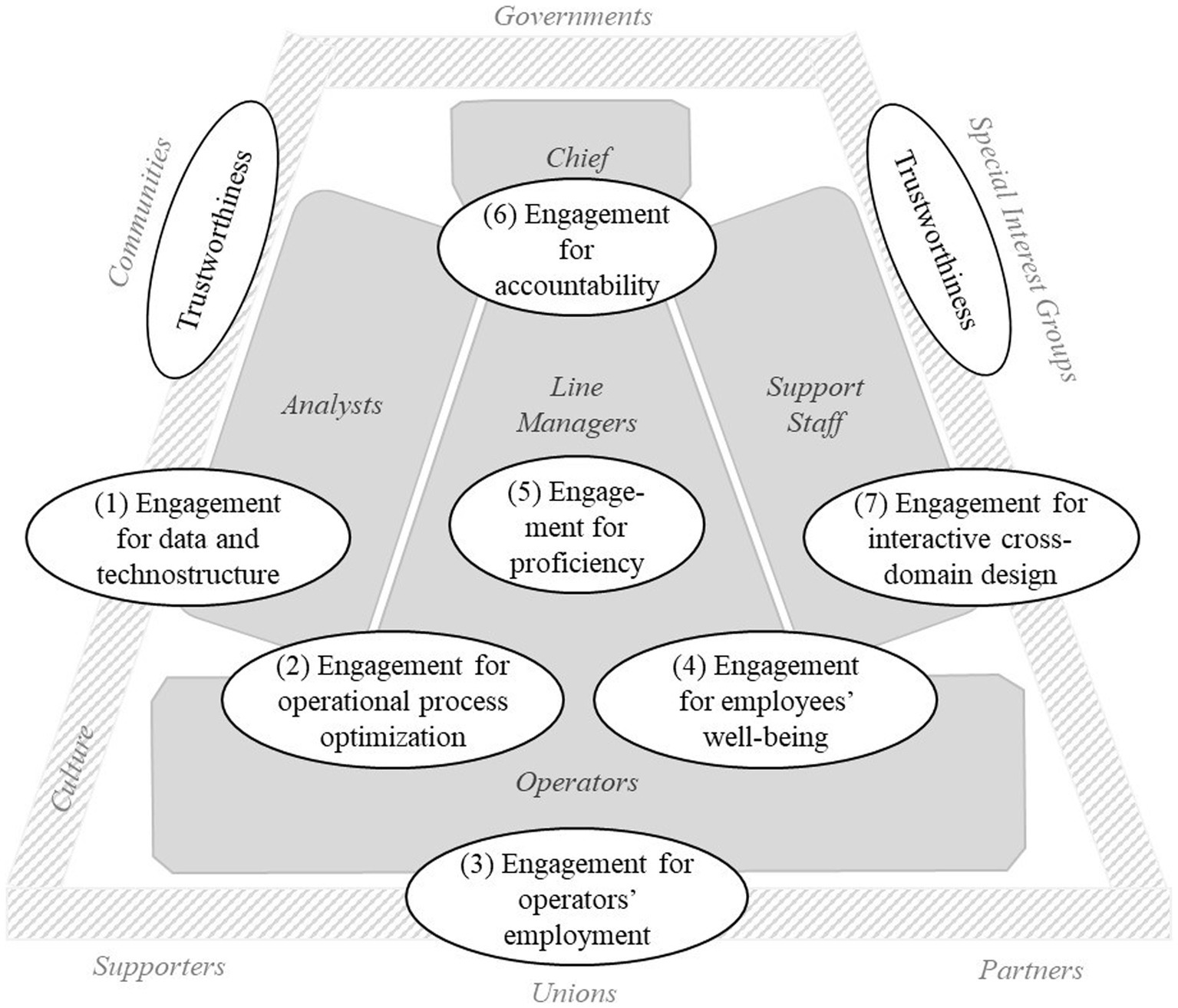
Figure 2. Actor-structure engagements of human-centered AI in organizational contexts. Adaptation of Mintzberg’s organizational actors (Mintzberg, 2023, p. 17, gray structure in the background; use of figure authorized by Mintzberg via email) by the actor-structure engagements of human-centered AI explored in the literature review (white cells).
However, the actor-structure engagement for selected criteria is a feasible approach for those with responsibilities and promotes the development as long as the stakeholders acknowledge additional perspectives and contributions from other domains or positions. To get to more integrative solutions, a first step could be to align two or three configurations with each other, e.g., the engagement for data and technostructure with the engagement for proficiency. This is especially helpful when they complement each other, e.g., the engagement for interactive cross-domain design allows to cope with the limitations that go hand in hand with the engagement for data and technostructure and to foster employees’ wellbeing.
4. Discussion, limitations, and outlook on future research
The systematic literature review across certain disciplines explores criteria of human-centricity while integrating AI in the work context. We could identify a variety of criteria that either face challenges of human-centered technology development, human-centered employee development, or human-centered organizational development. With this distinction, we could further develop already existing classifications (Wilkens et al., 2021a) and substantiate that the reflection on AI at work goes beyond issues of human-technology interaction but also includes organizational processes, structures, and policies. A further advancement is the synthesis of the eight analytically distinguishable criteria into seven context-related configurations, specifying the actor-structure engagement behind these criteria. Depending on the organizational sub-unit and the typical stakeholders involved in that unit, one criterion takes precedence and is supported by other criteria, while other criteria tend to be neglected. Considering the identified engagements for human-centricity against Mintzberg’s (2023) model of organizational configurations, it becomes obvious that all structural parts and related actors—operators, line managers, C-level managers, analysts, and support staff—are involved and in charge. The identified eight criteria of human-centricity and seven configurations of enacting and contextualizing them complement each other meaningfully and lead to a holistic overall approach. However, there is no actor-structure configuration, including all criteria, as a kind of mastermind approach.
Comparing the prevalent criteria of human-centricity as deduced from the academic discourse with the proposals for responsible AI declarations and regulations, it becomes obvious that outlines such as the EU AI Act (European Parliament, 2023) primarily face the two challenges of human-centered technology development. This is also the case for the industry norm ISO/IEC TR 24028:2020 (2020). Interestingly, the recently published proposal of the US Blueprint AI Bill of Rights goes beyond and considers the integration of user-domain knowledge in the AI development process and operators’ wellbeing as crucial points in addition to technology development (The White House, 2022). The industry norm ISO 9241-210:2019 (2019) gives emphasis to physical and mental health, especially mental load while interacting with technology. Even though the norm does not address AI explicitly, it can serve as a guideline for standards as long as more specific AI-related norms for human-AI interaction are missing. However, it also becomes obvious that other challenges of human-centered employee development and human-centered organizational development, especially with respect to human agency and augmentation and related process descriptions in job design, are neglected in comparison to the more traditional outlines of human wellbeing. This will be a future task. There is a rising number of organizations such as Microsoft, SAP, Bosch, or Deutsche Telekom that have company guidelines or codex agreements (Deutsche Telekom, 2018; Robert Bosch, 2020; SAP SE, 2021; Microsoft Corporation, 2022). They tend to include challenges of technology, employee, and organizational development but, at the same time, tend to be more vague in what criteria are addressed. However, it is interesting to note that accountability and safety culture gain attention in these declarations at the company level. This underlines C-level responsibility in the overall firm strategy. To date, only a few companies have published these guidelines. Future research will have to compare in more detail which criteria elaborate on an industry norm or are even an issue of legal regulation that tends to remain in the background and what the implications are when criteria are weighted unequally.
The overall implication of the norming initiatives is that, from an organizational actor perspective, these standards are supposed to be integrated into organizations by stakeholders from the legal departments, almost belonging to the support staff. Consequently, this group of stakeholders might have a higher impact in the future. While AI developers in the scientific discourse are in charge of the criteria due to formalization and regulation, they will rather be represented by lawyers in the practical context. This group of stakeholders could not be identified in such a clear manner from the conducted literature review. A higher engagement of lawyers, which can be expected in the future, can further foster the emphasis on human-centricity on the one hand.
On the other hand, this bears the risk that other criteria of human-centricity outlined in this review with a stronger emphasis on employee development and organizational development, which are less standardized so far, tend to be neglected or that the responsibility for human-centricity is delegated to the legal departments in organizations and not located where the AI development takes place (see Widder and Nafus, 2023). At least, there is a risk of overemphasizing technology-related criteria in comparison to the broader view provided in this article. A coping strategy could be to consider the technology-related criteria of human-centered AI as a necessary condition and to add on sufficient conditions related to the specific use field as proposed in the maturity model by Wilkens et al. (2021b).
The criteria and configurations explored in the systematic literature review need further empirical validation in the next step. This validation includes the analytical distinction of the named criteria and the context-specific consistency of the proposed configurations. Moreover, an empirical analysis should elaborate on further operationalizing the assumed related performative practices and outcomes. Another issue of empirical validation is to test whether configurations lead to a holistic perspective when integrating them or if there are shortcomings or differences due to power differences among the representing stakeholders, probably leading to crowding-out effects. The preferred approach for data evaluation is qualitative comparative analysis (QCA), as it is a mature concept especially developed for exploring configurations (Miller, 1986, 2017; Fiss et al., 2013; Misangyi et al., 2017).
The aim of the presented review was to elaborate on a common ground in human-centered AI at work, with an emphasis on the academic debate. The value and uniqueness of the approach lie in the contextualization of criteria and the stakeholders in charge of them. This allows us to better understand how human-centricity belongs to the work context while being enacted by a group of stakeholders. This also explains the co-existence of different engagements for human-centricity and that this can even generate an advantage as long as the criteria complement and do not crowd out each other.
Data availability statement
The original contributions presented in the study are included in the article/supplementary material, further inquiries can be directed to the corresponding author.
Author contributions
UW: Data curation, Investigation, Methodology, Validation, Writing – original draft, Conceptualization, Project administration. DL: Data curation, Investigation, Methodology, Validation, Writing – original draft, Visualization. VL: Conceptualization, Data curation, Investigation, Project administration, Validation, Writing - review & editing.
Funding
The author(s) declare financial support was received for the research, authorship, and/or publication of this article. The study was funded by Competence Center HUMAINE: Transfer-Hub of the Ruhr Metropolis for human-centered work with AI (human-centered AI network), Funding code: BMBF 02L19C200.
Conflict of interest
The authors declare that the research was conducted in the absence of any commercial or financial relationships that could be construed as a potential conflict of interest.
Publisher’s note
All claims expressed in this article are solely those of the authors and do not necessarily represent those of their affiliated organizations, or those of the publisher, the editors and the reviewers. Any product that may be evaluated in this article, or claim that may be made by its manufacturer, is not guaranteed or endorsed by the publisher.
References
Adadi, A., and Berrada, M. (2018). Peeking inside the black-box: a survey on explainable artificial intelligence (XAI). IEEE Access 6, 52138–52160. doi: 10.1109/ACCESS.2018.2870052
Adnan, H. S., Matthews, S., Hackl, M., Das, P. P., Manaswini, M., Gadamsetti, S., et al. (2020). Human centered AI design for clinical monitoring and data management. Eur. J. Pub. Health 30:86. doi: 10.1093/eurpub/ckaa165.225
Ahrens, V. (2014). Industrie 4.0: Ein humanzentrierter Ansatz als Gegenentwurf zu technikzentrierten Konzepten. Working paper der Nordakademie Nr. 2014-05. Elmshorn.
Akata, Z., Balliet, D., Rijke, M., Dignum, F., Dignum, V., Eiben, G., et al. (2020). A research agenda for hybrid intelligence: augmenting human intellect with collaborative, adaptive, responsible, and explainable artificial intelligence. Computer 53, 18–28. doi: 10.1109/MC.2020.2996587
Albahri, A. S., Duhaim, A. M., Fadhel, M. A., Alnoor, A., Baqer, N. S., Alzubaidi, L., et al. (2023). A systematic review of trustworthy and explainable artificial intelligence in healthcare: assessment of quality, bias risk, and data fusion. Informat. Fusion 96, 156–191. doi: 10.1016/j.inffus.2023.03.008
Anthony, C., Bechky, B. A., and Fayard, A. (2023). “Collaborating” with AI: taking a system view to explore the future of work. Organ. Sci. 34, 1672–1694. doi: 10.1287/orsc.2022.1651
Arntz, M., Gregory, T., and Zierahn, U. (2020). Digitalisierung und die Zukunft der Arbeit. Wirtschaftsdienst 100, 41–47. doi: 10.1007/s10273-020-2614-6
Badham, R. (1995). “Managing sociotechnical change: a configuration approach to technology implementation,” in The symbiosis of work and technology, ed. J. Benders, J. Haande, and D. Bennett London: Taylor & Francis Ltd., 77–94.
Barredo, A. A., Díaz-Rodríguez, N., Del Ser, J., Bennetot, A., Tabik, S., Barbado, A. B. A. A., et al. (2020). Explainable artificial intelligence (XAI): concepts, taxonomies, opportunities and challenges toward responsible AI. Information Fusion 58, 82–115. doi: 10.1016/j.inffus.2019.12.012
Battaglia, E., Boehm, J., Zheng, Y., Jamieson, A. R., Gahan, J., and Fey, A. M. (2021). Rethinking autonomous surgery: focusing on enhancement over autonomy. Eur. Urol. Focus 7, 696–705. doi: 10.1016/j.euf.2021.06.009
Beede, E., Baylor, E., Hersch, F., Iurchenko, A., Wilcox, L., Ruamviboonsuk, P., et al. (2020). “A human-centered evaluation of a deep learning system deployed in clinics for the detection of diabetic retinopathy” in Proceedings of the 2020 CHI conference on human factors in computing systems (New York, NY: Association fpr Computing Machinery), 1–12.
Bettoni, M. C. (1995). Kant and the software crisis: suggestions for the construction of human-centred software systems. AI & Soc. 9, 396–401. doi: 10.1007/BF01210590
Bond, R., Mulvenna, M. D., Wan, H., Finlay, D. D., Wong, A., Koene, A., et al. (2019). “Human centered artificial intelligence: weaving UX into algorithmic decision making,” In 2019 16th international conference on human-computer interaction (RoCHI) (Bucharest, RO), 2–9.
Cui, X., and Dai, R. (2008). A human-centred intelligent system framework: meta-synthetic engineering. International Journal of Intelligent Information and Database Systems 2, 82–105. doi: 10.1504/IJIIDS.2008.017246
Davagdorj, K., Bae, J. W., Pham, V. H., Theera-Umpon, N., and Ryu, K. H. (2021). Explainable artificial intelligence based framework for non-communicable diseases prediction. IEEE Access 9, 123672–123688. doi: 10.1109/access.2021.3110336
De Cremer, D., and Kasparov, G. (2021). AI should augment human intelligence, not replace it. Harv. Bus. Rev. 18
Del Giudice, M., Scuotto, V., Orlando, B., and Mustilli, M. (2023). Toward the human–centered approach. A revised model of individual acceptance of AI. Hum. Resour. Manag. Rev. 33:100856. doi: 10.1016/j.hrmr.2021.100856
Deutsche Telekom, AG (2018). Digital ethics guidelines on AI. Available at: https://www.telekom.com/resource/blob/544508/ca70d6697d35ba60fbcb29aeef4529e8/dl-181008-digitale-ethik-data.pdf
Dewey, M., and Wilkens, U. (2019). The bionic radiologist: avoiding blurry pictures and providing greater insights. npj Digital Medicine 2, 1–7. doi: 10.1038/s41746-019-0142-9
Ehsan, U., and Riedl, M. O. (2020). “Human-centered explainable AI: towards a reflective sociotechnical approach” in 2020 international conference on human-computer interaction (HCII) (Copenhagen, DK: Springer, Cham), 449–466.
Elkmann, N. (2013). Sichere Mensch-Roboter-Kooperation: Normenlage, Forschungsfelder und neue Technologien. Zeitschrift für Arbeitswissenschaft 67, 143–149. doi: 10.1007/BF03374401
European Parliament (2023). Artificial intelligence act, P9_TA(2023)0236. Available at: https://www.europarl.europa.eu/doceo/document/TA-9-2023-0236_DE.html
Fischer, G. (2022). A research framework focused on ‘AI and humans’ instead of ‘AI versus humans. Interaction Design and Architecture(s) – IxD&A Journal. doi: 10.55612/s-5002-000
Fischer, G., and Nakakoji, K. (1992). Beyond the macho approach of artificial intelligence: empower human designers – do not replace them. Knowledge-Based Systems Journal, Special Issue on AI in Design 5, 15–30. doi: 10.1016/0950-7051(92)90021-7
Fiss, P. C., Cambré, B., and Marx, A. (2013). “Configurational theory and methods in organizational research” in Research in the sociology of organizations. eds. P. C. Fiss, B. Cambré, and A. Marx (Emerald Group Publishing Limited), 1–22.
Flandrin, P., Hellemans, C., Van der Linden, J., and Van de Leemput, C. (2021). “Smart technologies in hospitality: effects on activity, work design and employment. A case study about chatbot usage” in 2021 17th proceedings of the 17th “Ergonomie et Informatique Avancée” conference (New York, NY: Association for Computing Machinery), 1–11. doi: 10.1145/3486812.3486838
Foresti, R., Rossi, S., Magnani, M., Bianco, C. G. L., and Delmonte, N. (2020). Smart society and artificial intelligence: big data scheduling and the global standard method applied to smart maintenance. Engineering 6, 835–846. doi: 10.1016/j.eng.2019.11.014
Fox, J., South, M., Khan, O., Kennedy, C., Ashby, P., and Bechtel, J. (2020). OpenClinical. net: artificial intelligence and knowledge engineering at the point of care. BMJ Health Care Informatics 27. doi: 10.1136/bmjhci-2020-100141
Freitag, M., Molzow-Voit, F., Quandt, M., and Spöttl, G. (2016). “Aktuelle Entwicklung der Robotik und ihre Implikationen für den Menschen” in Robotik in der Logistik: Qualifizierung für Fachkräfte und Entscheider. eds. F. Molzow-Voit, M. Quandt, M. Freitag, and G. Spöttl (Wiesbaden, GE: Springer Fachmedien Wiesbaden), 9–20.
Frissen, V. (2022). “Working with big data and Al: toward balanced and responsible working practices” in Digital innovation and the future of work. eds. H. Schaffers, M. Vartiainen, and J. Bus (New York, NY: River Publishers), 111–136. doi: 10.1201/9781003337928
Gamkrelidze, T., Zouinar, M., and Barcellini, F. (2022). “Artificial intelligence (AI) in the workplace: a study of stakeholders’ views on benefits, issues and challenges of AI systems” in Proceedings of the 21st congress of the international ergonomics association (IEA 2021) (Cham: Springer International Publishing), 628–635.
Garcia-Magarino, I., Muttukrishnan, R., and Lloret, J. (2019). Human-Centric AI for trustworthy IoT systems with explainable multilayer perceptions. IEEE Access 7, 125562–125574. doi: 10.1109/ACCESS.2019.2937521
Gillies, M., Lee, B., d’Alessandro, N., Tilmanne, J., Kulesza, T., Caramiaux, B., et al. (2016). “Human-Centred machine learning” in Proceedings of the 2016 CHI conference extended abstracts on human factors in computing systems (New York, NY: Association for Computing Machinery), 3558–3565.
Greckhamer, T., Furnari, S., Fiss, P. C., and Aguilera, R. V. (2018). Studying configurations with qualitative comparative analysis: best practices in strategy and organization research. Strateg. Organ. 16, 482–495. doi: 10.1177/1476127018786487
Grischke, J., Johannsmeier, L., Eich, L., Griga, L., and Haddadin, S. (2020). Dentronics: towards robotics and artificial intelligence in dentistry. Dent. Mater. 36, 765–778. doi: 10.1016/j.dental.2020.03.021
Gu, H., Huang, J., Hung, L., and Chen, X. A. (2021). Lessons learned from designing an AI-enabled diagnosis tool for pathologists. Proc. ACM Hum. Comput. Interact. 5, 1–25. doi: 10.1145/3449084
Gunning, D., Stefik, M., Choi, J., Miller, T., Stumpf, S., and Yang, G. Z. (2019). XAI—Explainable artificial intelligence. Sci. Robot. 4, 1–2. doi: 10.1126/scirobotics.aay7120
Guszcza, J. (2018). Smarter together: why artificial intelligence needs human-centered design. Deloitte Rev. 22, 36–45.
Guszcza, J., Harvey, L., and Evans-Greenwood, P. (2017). Cognitive collaboration: why humans and computers think better together. Deloitte Rev. 20, 7–30.
Hashimoto, D. A., Rosman, G., Rus, D., and Meireles, O. R. (2018). Artificial intelligence in surgery: promises and perils. Ann. Surg. 268, 70–76. doi: 10.1097/sla.0000000000002693
Häusler, R., and Sträter, O. (2020). “Arbeitswissenschaftliche Aspekte der Mensch-Roboter-Kollaboration” in Mensch-Roboter-Kollaboration. ed. H. J. Buxbaum (Wiesbaden, GE: Springer Fachmedien Wiesbaden), 35–54.
Havrda, M., and Rakova, B. (2020). “Enhanced wellbeing assessment as basis for the practical implementation of ethical and rights-based normative principles for AI” in 2020 IEEE international conference on systems, man, and cybernetics (SMC) (Toronto, ON: IEEE), 2754–2761.
Hayes, B., and Moniz, M. (2021). “Trustworthy human-centered automation through explainable AI and high-fidelity simulation” in 2020 international conference on applied human factors and ergonomics (AHFE) (Cham: Springer), 3–9.
Heier, J. (2021). “Design intelligence-taking further steps towards new methods and tools for designing in the age of AI” in Artificial intelligence in HCI: Second international conference, AI-HCI international conference (HCII) (Cham: Springer), 202–215.
Heier, J., Willmann, J., and Wendland, K. (2020). “Design intelligence-pitfalls and challenges when designing AI algorithms in B2B factory automation” in Artificial intelligence in HCI: first international conference (Cham: Springer), 288–297.
Hepenstal, S., Zhang, L., Kodagoda, N., and Wong, B. W. (2021b). “A granular computing approach to provide transparency of intelligent systems for criminal investigations” in Interpretable artificial intelligence: a perspective of granular computing. eds. W. Pedrycz and S.-M. Chen, vol. 937 (Cham: Springer)
Hepenstal, S., Zhang, L., and Wong, B. W. (2021a). “An analysis of expertise in intelligence analysis to support the design of human-centered artificial intelligence,” in 2021 IEEE international conference on systems, man, and cybernetics (SMC) Melbourne, Australia (IEEE), 107–112
Herzog, M., Wilkens, U., Bülow, F., Hohagen, S., Langholf, V., Öztürk, E., et al. (2022). “Enhancing digital transformation in SMEs with a multi-stakeholder approach” in Digitization of the work environment for sustainable production. ed. P. Plapper (Berlin, GE: GITO Verlag), 17–35.
Hinds, P., Roberts, T., and Jones, H. (2004). Whose job is it anyway? A study of human-robot interaction in a collaborative task. Hum. Comput. Interact. 19, 151–181. doi: 10.1207/s15327051hci1901%262_7
Ho, C. W., Ali, J., and Caals, K. (2020). Ensuring trustworthy use of artificial intelligence and big data analytics in health insurance. Bull. World Health Organ. 98, 263–269. doi: 10.2471/blt.19.234732
Holstein, K., McLaren, B. M., and Aleven, V. (2019). “Designing for complementarity: teacher and student needs for orchestration support in AI-enhanced classrooms” in Proceedings of the 20th international conference on artificial intelligence and education (New York: Springer International Publishing), 157–171.
Holzinger, A., Saranti, A., Angerschmid, A., Retzlaff, C. O., Gronauer, A., Pejakovic, V., et al. (2022). Digital transformation in smart farm and forest operations needs human-centered AI: challenges and future directions. Sensors 22:3043. doi: 10.3390/s22083043
How, M.-L., and Chan, Y. J. (2020). Artificial intelligence-enabled predictive insights for ameliorating global malnutrition: a human-centric AI-thinking approach. AI 1, 68–91. doi: 10.3390/ai1010004
How, M.-L., Cheah, S.-M., Chan, Y. J., Khor, A. C., and Say, E. M. P. (2020a). Artificial intelligence-enhanced decision support for informing global sustainable development: a human-centric AI-thinking approach. Information 11, 1–24. doi: 10.3390/info11010039
How, M.-L., Cheah, S.-M., Khor, A. C., and Chan, Y. J. (2020b). Artificial intelligence-enhanced predictive insights for advancing financial inclusion: a human-centric AI-thinking approach. BDCC 4, 1–21. doi: 10.3390/bdcc4020008
Hynynen, J. (1992). Using artificial intelligence technologies in production management. Comput. Ind. 19, 21–35. doi: 10.1016/0166-3615(92)90004-7
ISO 9241-210:2019 (2019). Ergonomics of human-system interaction — Part 210: human-centred design for interactive systems.
ISO/IEC TR 24028:2020 (2020). Information technology — Artificial intelligence — Overview of trustworthiness in artificial intelligence.
Jarrahi, M. H. (2018). Artificial intelligence and the future of work: human-AI symbiosis in organizational decision making. Bus. Horiz. 61, 577–586. doi: 10.1016/j.bushor.2018.03.007
Jiang, J., Karran, A. J., Coursaris, C. K., Léger, P. M., and Beringer, J. (2021). “A situation awareness perspective on human-agent collaboration: tensions and opportunities” in HCI international 2021-late breaking papers: multimodality, eXtended reality, and artificial intelligence: 23rd HCI international conference (HCII), vol. 39 (New York: Springer International Publishing), 1789–1806.
Jung, M., Werens, S., and von Garrel, J. (2022). Vertrauen und Akzeptanz bei KI-basierten, industriellen Arbeitssystemen. Zeitschrift für wirtschaftlichen Fabrikbetrieb 117, 781–783. doi: 10.1515/zwf-2022-1134
Kaasinen, E., Anttila, A. H., Heikkilä, P., Laarni, J., Koskinen, H., and Väätänen, A. (2022). Smooth and resilient human–machine teamwork as an industry 5.0 design challenge. Sustainability 14:2773.b. doi: 10.3390/su14052773
Kahng, M. B. (2019). Human-centered AI through scalable visual data analytics. [dissertation] [Georgia]: Institute of Technology
Kaiser, O. S., and Malanowski, N. (2019). Smart Data und Künstliche Intelligenz: Technologie, Arbeit, Akzeptanz. Working Paper Forschungsförderung 136. Available at: http://hdl.handle.net/10419/216056
Kathuria, R., and Kathuria, V. (2020). “The use of human-centered AI to augment the health of older adults” in HCI international 2020–late breaking posters: 22nd international conference, HCII 2020 (Cham: Springer International Publishing), 469–477.
Kim, J.-W., Choi, Y.-L., Jeong, S.-H., and Han, J. (2022). A care robot with ethical sensing system for older adults at home. Sensors 22:7515. doi: 10.3390/s22197515
Kluge, A., Ontrup, G., Langholf, V., and Wilkens, U. (2021). Mensch-KI-Teaming: Mensch und Künstliche Intelligenz in der Arbeitswelt von morgen. Zeitschrift für wirtschaftlichen Fabrikbetrieb 116, 728–734. doi: 10.1515/zwf-2021-0112
Kraus, S., Breier, M., Lim, W. M., Dabić, M., Kumar, S., Kanbach, D. K., et al. (2022). Literature reviews as independent studies: guidelines for academic practice. Rev. Manag. Sci. 16, 2577–2595. doi: 10.1007/s11846-022-00588-8
Krzywdzinski, M., Gerst, D., and Butollo, F. (2023). Promoting human-centred AI in the workplace. Trade unions and their strategies for regulating the use of AI in Germany. Transfer 29, 53–70. doi: 10.1177/10242589221142273
Łapińska, J., Escher, I., Górka, J., Sudolska, A., and Brzustewicz, P. (2021). Employees’ trust in artificial intelligence in companies: the case of energy and chemical industries in Poland. Energies 14:1942. doi: 10.3390/en14071942
Launchbury, J. (2017). A DARPA perspective on artificial intelligence. DARPA talk, February 15, 2017.
Legaspi, R., He, Z., and Toyoizumi, T. (2019). Synthetic agency: sense of agency in artificial intelligence. Curr. Opin. Behav. Sci. 29, 84–90. doi: 10.1016/j.cobeha.2019.04.004
Leonardi, P. M. (2013). When does technology use enable network change in organizations? A comparative study of feature use and shared affordances. MIS Q. 37, 749–775. doi: 10.25300/MISQ/2013/37.3.04
Li, S., Peng, G., Xing, F., Zhang, J., and Qian, Z. (2021). Value Co-creation in Industrial AI: the interactive role of B2B supplier, customer and technology provider. Ind. Mark. Manag. 98, 105–114. doi: 10.1016/j.indmarman.2021.07.015
Liu, C., Tian, W., and Kan, C. (2022). When AI meets additive manufacturing: challenges and emerging opportunities for human-centered products development. J. Manuf. Syst. 64, 648–656. doi: 10.1016/j.jmsy.2022.04.010
Lukowicz, P. (2019). The challenge of human centric. Digitale Welt 3, 9–10. doi: 10.1007/s42354-019-0200-0
Luštrek, M., Bohanec, M., Barca, C. C., Ciancarelli, M. G. T., Clays, E., Dawodu, A. A., et al. (2021). A personal health system for self-management of congestive heart failure (HeartMan): development, technical evaluation, and proof-of-concept randomized controlled trial. JMIR Med. Inform. 9:e24501. doi: 10.2196/24501
Maiden, N., Lockerbie, J., Zachos, K., Wolf, A., and Brown, A. (2022). Designing new digital tools to augment human creative thinking at work: an application in elite sports coaching. Expert. Syst. 40. doi: 10.1111/exsy.13194
Mehta, R., Moats, J., Karthikeyan, R., Gabbard, J., Srinivasan, D., Du, E., et al. (2022). Human-centered intelligent training for emergency responders. AI Mag. 43, 83–92. doi: 10.1002/aaai.12041
Meyer, A. D., Tsui, A. S., and Hinings, C. R. (1993). Configurational approaches to organizational analysis. Acad. Manag. J. 36, 1175–1195. doi: 10.5465/256809
Mhlanga, D. (2022). Human-centered artificial intelligence: the superlative approach to achieve sustainable development goals in the fourth industrial revolution. Sustainability 14:7804. doi: 10.3390/su14137804
Microsoft Corporation (2022). Microsoft responsible AI standard, v2. Available at: https://query.prod.cms.rt.microsoft.com/cms/api/am/binary/RE5cmFl
Miller, D. (1986). Configurations of strategy and structure: towards a synthesis. Strateg. Manag. J. 7, 233–249. doi: 10.1002/smj.4250070305
Miller, D. (2017). Challenging trends in configuration research: where are the configurations? Strateg. Organ. 16, 453–469. doi: 10.1177/1476127017729315
Mintzberg, H. (1979). The structuring of organizations: a synthesis of the research. Englewood Cliffs, NJ Prentice Hall International.
Mintzberg, H. (1993). Structure in fives: designing effective organizations. Englewood Cliffs, NJ Prentice Hall International.
Mintzberg, H. (2023). Understanding organizations… Finally!: Structuring in sevens. Englewood Cliffs, NJ Berrett-Koehler Publishers.
Misangyi, V. F., Greckhamer, T., Furnari, S., Fiss, P. C., Crilly, D., and Aguilera, R. V. (2017). Embracing causal complexity. J. Manag. 43, 255–282. doi: 10.1177/0149206316679252
Morrow, E., Zidaru, T., Ross, F., Mason, C., Patel, K. D., Ream, M., et al. (2023). Artificial intelligence technologies and compassion in healthcare: a systematic scoping review. Front. Psychol. 13:971044. doi: 10.3389/fpsyg.2022.971044
Nahavandi, S. (2019). Industry 5.0—a human-centric solution. Sustainability 11, 1–13. doi: 10.3390/su11164371
Nakao, Y., Strappelli, L., Stumpf, S., Naseer, A., Regoli, D., and Del Gamba, G. (2022). Towards responsible AI: a design space exploration of human-centered artificial intelligence user interfaces to investigate fairness. Int. J. Hum. Comput. Interact. 39, 1762–1788. doi: 10.1080/10447318.2022.2067936
Nisser, A., and Malanowski, N. (2019). Branchenanalyse chemische und pharmazeutische Industrie: Zukünftige Entwicklungen im Zuge Künstlicher Intelligenz. Working Paper Forschungsförderung, 166. Available at: http://hdl.handle.net/10419/216086
Organ, J. F., O’Neill, B. C., and Stapleton, L. (2021). Artificial intelligence and human-machine symbiosis in public employment services (PES): lessons from engineer and trade unionist, professor Michael Cooley. IFAC-PapersOnLine 387, 387–392. doi: 10.1016/j.ifacol.2021.10.478
Orlikowski, W. J. (1992). The duality of technology: rethinking the concept of technology in organizations. Organ. Sci. 3, 398–427. doi: 10.1287/orsc.3.3.398
Orlikowski, W. J. (2000). Using technology and constituting structures: a practice lens for studying technology in organizations. Organ. Sci. 11, 404–428. doi: 10.1287/orsc.11.4.404.14600
Orlikowski, W. J. (2007). Sociomaterial practices: exploring technology at work. Organ. Stud. 28, 1435–1448. doi: 10.1177/0170840607081138
Orlikowski, W. J., and Scott, S. V. (2008). 10 sociomateriality: challenging the separation of technology, work and organization. Acad. Manag. Ann. 2, 433–474. doi: 10.1080/19416520802211644
Page, M. J., McKenzie, J. E., Bossuyt, P. M., Boutron, I., Hoffmann, T. C., Mulrow, C. D., et al. (2021). Updating guidance for reporting systematic reviews: development of the PRISMA 2020 statement. J. Clin. Epidemiol. 134, 103–112. doi: 10.1016/j.jclinepi.2021.02.003
Parker, S. K., and Grote, G. (2022). Automation, algorithms, and beyond: why work design matters more than ever in a digital world. Appl. Psychol. 71, 1171–1204. doi: 10.1111/apps.12241
Pava, C. (1986). Redesigning sociotechnical systems design: concepts and methods for the 1990s. J. Appl. Behav. Sci. 22, 201–221. doi: 10.1177/002188638602200303
Petropoulos, G. (2018). The impact of artificial intelligence on employment. Praise Work Digital Age, 119–132.
Plass, M., Kargl, M., Nitsche, P., Jungwirth, E., Holzinger, A., and Müller, H. (2022). Understanding and explaining diagnostic paths: toward augmented decision making. IEEE Comput. Graph. Appl. 42, 47–57. doi: 10.1109/mcg.2022.3197957
Ploug, T., and Holm, S. (2020). The four dimensions of contestable AI diagnostics - a patient-centric approach to explainable AI. Artif. Intell. Med. 107, 101901–101905. doi: 10.1016/j.artmed.2020.101901
Polak, S., Schiavo, G., and Zancanaro, M. (2022). Teachers’ perspective on artificial intelligence education: an initial investigation,” in Extended abstracts CHI conference on human factors in computing systems. 1–7
Riedl, M. O. (2019). Human-centered artificial intelligence and machine learning. Hum. Behav. Emerg. Tech. 1, 33–36. doi: 10.48550/arXiv.1901.11184
Riener, R., Frey, M., Bernhardt, M., Nef, T., and Colombo, G. (2005). “Human-centered rehabilitation robotics” in 2005 9th international conference on rehabilitation robotics (ICORR) (Chicago, IL: IEEE), 319–322.
Riener, R., Lünenburger, L., and Colombo, G. (2006). Human-centered robotics applied to gait training and assessment. J. Rehabil. Res. Dev. 43, 679–694. doi: 10.1682/jrrd.2005.02.0046
Robert Bosch, GmbH (2020). KI-Kodex von Bosch im Überblick. Available at: https://assets.bosch.com/media/de/global/stories/ai_codex/bosch-code-of-ethics-for-ai.pdf
Romero, D., Stahre, J., Wuest, T., Noran, O., Bernus, P., Fast-Berglund, Å., et al. (2016). “Towards an operator 4.0 typology: a human-centric perspective on the fourth industrial revolution technologies” in 2016 46th international conference on computers and industrial engineering (CIE46) (United States: Computers and Industrial Enfineering), 29–31.
Rožanec, J. M., Zajec, P., Kenda, K., Novalija, I., Fortuna, B., Mladenić, D., et al. (2021). STARdom: an architecture for trusted and secure human-centered manufacturing systems. ArXiv. doi: 10.1007/978-3-030-85910-7_21
Russell, S., and Norvig, P. (2021). Artificial intelligence: a modern approach. Upper Saddle River, NJ Prentice Hall.
Russo-Spena, T. R., Mele, C., and Marzullo, M. (2019). Practising value innovation through artificial intelligence: the IBM Watson case. Journal of creating value 5, 11–24. doi: 10.1177/2394964318805839
SAP SE (2021). SAP’s guiding principles for artificial intelligence. Available at: https://www.sap.com/documents/2018/09/940c6047-1c7d-0010-87a3-c30de2ffd8ff.html
Schaal, S. (2007). The new robotics-towards human-centered machines. HFSP journal 1, 115–126. doi: 10.2976/1.2748612
Schmidtler, J., Knott, V., Hölzel, C., and Bengler, K. (2015). Human centered assistance applications for the working environment of the future. Occupat. Ergon. 12, 83–95. doi: 10.3233/OER-150226
Schönböck, J., Kurschl, W., Augstein, M., Altmann, J., Fraundorfer, J., Freller, L., et al. (2022). From remote-controlled excavators to digitized construction sites. Proc. Comput. Sci. 200, 1155–1164. doi: 10.1016/j.procs.2022.01.315
Seabra, D., Da Silva Santos, P. S., Paiva, J. S., Alves, J. F., Ramos, A., Cardoso, M. V. L. M. L., et al. (2022). The importance of design in the development of a portable and modular Iot-based detection device for clinical applications. J. Phys. 2292:012009. doi: 10.1088/1742-6596/2292/1/012009
Shaikh, S. J. (2020). Artificial intelligence and resource allocation in health care: the process-outcome divide in perspectives on moral decision-making. AAAI Fall 2020 Symposium on AI for Social Good. 1–8.
Shneiderman, B. (2020a). Bridging the gap between ethics and practice. ACM Transact. Interact. Intellig. Syst. 10, 1–31. doi: 10.1145/3419764
Shneiderman, B. (2020b). Human-centered artificial intelligence: reliable, safe & trustworthy. Int. J. Hum. Comput. Interact. 36, 495–504. doi: 10.1080/10447318.2020.1741118
Shneiderman, B. (2020c). Human-centered artificial intelligence: three fresh ideas. AIS Transact. Hum. Comput. Interact. 12, 109–124. doi: 10.17705/1thci.00131
Smith, N. L., Teerawanit, J., and Hamid, O. H. (2018). “AI-driven automation in a human-centered cyber world” in 2018 IEEE international conference of systems, man, and cybernetics (SMC) (Miyazaki, JP: IEEE)
Snyder, H. (2019). Literature review as a research methodology: an overview and guidelines. J. Bus. Res. 104, 333–339. doi: 10.1016/j.jbusres.2019.07.039
Soldatos, J., and Kyriazis, D. (2021). Trusted artificial intelligence in manufacturing: a review of the emerging wave of ethical and human centric AI technologies for smart production. Boston: Now Publishers.
Springer, A. (2019). Accurate, fair, and explainable: Building human-centered AI. [dissertation]. [Santa Cruz]: University of California
Steels, L. (2020). “Personal dynamic memories are necessary to deal with meaning and understanding in human-centric AI” in Proceedings of the first international workshop on new foundations for human-centered AI (NeHuAI) (Santiago de Compostella, ES: CEUR-WS), 11–16.
Strohm, O., and Ulich, E. (1998). Integral analysis and evaluation of enterprises: a multi-level approach in terms of people, technology, and organization. Hum. Fact. Ergon. Manufact. 8, 233–250. doi: 10.1002/(SICI)1520-6564(199822)8:3%3C233::AID-HFM3%3E3.0.CO;2-4
Suchman, L. (2012). “Configuration” in Inventive methods. The happening of the social. eds. C. Lury and N. Wakeford (London: Routledge), 48–60.
Suh, M., Youngblom, E., Terry, M., and Cai, C. J. (2021). AI as social glue: uncovering the roles of deep generative AI during social music composition. Proceedings of the 2021 CHI conference on human factors in computing systems, Yokohama, Japan 582, 1–11
Taryudi, T., Lindayani, L., Purnama, H., and Mutiar, A. (2022). Nurses’ view towards the use of robotic during pandemic COVID-19 in Indonesia: a qualitative study. Open Access Maced J. Med. Sci. 10, 14–18. doi: 10.3889/oamjms.2022.7645
The White House (2022). Blueprint for an AI bill of rights. Making automated systems work for the American people. Available at: https://www.whitehouse.gov/wp-content/uploads/2022/10/Blueprint-for-an-AI-Bill-of-Rights.pdf
Tzachor, A., Devare, M., King, B., Avin, S., and Héigeartaigh, S. Ó. (2022). Responsible artificial intelligence in agriculture requires systemic understanding of risks and externalities. Nat. Mach. Intellig. 4, 104–109. doi: 10.1038/s42256-022-00440-4
Wang, D., Maes, P., Ren, X., Shneiderman, B., Shi, Y., and Wang, Q. (2021). “Designing AI to work WITH or FOR people?” in Extended abstracts of the 2021 CHI conferende in human factors in computing systems (New York, NY: Association for Computing Machinery)
Weekes, T. R., and Eskridge, T. C. (2022a). “Design thinking the human-AI experience of neurotechnology for knowledge workers” in HCI international 2022 – Late breaking papers. Multimodality in advanced interaction environments. Lecture Notes in Computer Science (Berlin: Springer Science + Business Media), 527–545.
Weekes, T. R., and Eskridge, T. C. (2022b). “Responsible human-centered artificial intelligence for the cognitive enhancement of knowledge workers” in Lecture notes in computer science (Berlin: Springer Science + Business Media), 568–582.
Wei, X., Ruan, M., Vadivel, T., and Daniel, J. A. (2022). Human-centered applications in sustainable smart city development: a qualitative survey. J. Interconnect. Netw. 22:2146001. doi: 10.1142/S0219265921460014
Widder, D. G., and Nafus, D. (2023). Dislocated accountabilities in the “AI supply chain”: modularity and developers’ notions of responsibility. Big Data Soc. 10. doi: 10.1177/20539517231177620
Wilkens, U. (2020). Artificial intelligence in the workplace – a double-edged sword. Int. J. Informat. Lear. Technol. 37, 253–265. doi: 10.1108/IJILT-02-2020-0022
Wilkens, U., Cost Reyes, C., Treude, T., and Kluge, A. (2021a). Understandings and perspectives of human-centered AI–A transdisciplinary literature review. GfA Frühjahrskongress, B.10.17.
Wilkens, U., Langholf, V., Ontrup, G., and Kluge, A. (2021b). “Towards a maturity model of human-centered AI–A reference for AI implementation at the workplace” in Competence development and learning assistance systems for the data-driven future. eds. W. Sihn and S. Schlund (Berlin, GE: GITO-Verlag), 179–197.
Wilkens, U., Lins, D., Prinz, C., and Kuhlenkötter, B. (2019). “Lernen und Kompetenzentwicklung in Arbeitssystemen mit künstlicher Intelligenz” in Digitale transformation. gutes arbeiten und qualifizierung aktiv gestalten. eds. D. Spath and B. Spanner-Ulmer (Berlin, GE: GITO-Verlag), 71–88.
Wilson, H. J., and Daugherty, P. R. (2018). Collaborative intelligence: humans and AI are joining forces. Harv. Bus. Rev. 96, 114–123.
Xie, Y., Gao, G., and Chen, X. (2019). “Outlining the design space of explainable intelligent systems for medical diagnosis” in Joint proceedings of the ACM IUI workshops (Los Angeles, USA: CEUR-WS)
Xu, W. (2019). Toward human-centered AI: a perspective from human-computer interactions. Interactions 26, 42–46. doi: 10.1145/3328485
Zhang, Y., Bellamy, R., and Varshney, K. (2020). “Joint optimization of AI fairness and utility: a human-centered approach” in Proceedings of the AAAI/ACM conference on AI, ethics, and society (New York, NY: ACM), 400–406.
Keywords: human-centered, artificial intelligence, AI, work, sociotechnical system, configurational theory, stakeholder
Citation: Wilkens U, Lupp D and Langholf V (2023) Configurations of human-centered AI at work: seven actor-structure engagements in organizations. Front. Artif. Intell. 6:1272159. doi: 10.3389/frai.2023.1272159
Edited by:
Assunta Di Vaio, University of Naples Parthenope, ItalyReviewed by:
Tobias Ley, Tallinn University, EstoniaVictor Lo, Fidelity Investments, United States
Paola Briganti, University of Naples Parthenope, Italy
Copyright © 2023 Wilkens, Lupp and Langholf. This is an open-access article distributed under the terms of the Creative Commons Attribution License (CC BY). The use, distribution or reproduction in other forums is permitted, provided the original author(s) and the copyright owner(s) are credited and that the original publication in this journal is cited, in accordance with accepted academic practice. No use, distribution or reproduction is permitted which does not comply with these terms.
*Correspondence: Uta Wilkens, dXRhLndpbGtlbnNAcnVoci11bmktYm9jaHVtLmRl