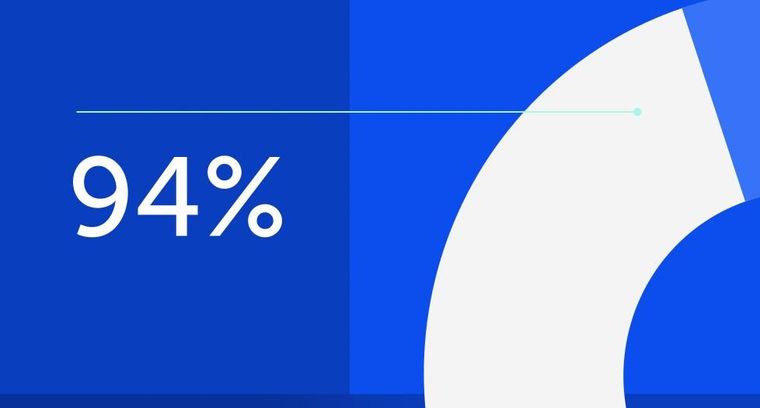
94% of researchers rate our articles as excellent or good
Learn more about the work of our research integrity team to safeguard the quality of each article we publish.
Find out more
SYSTEMATIC REVIEW article
Front. Artif. Intell., 03 July 2023
Sec. AI for Human Learning and Behavior Change
Volume 6 - 2023 | https://doi.org/10.3389/frai.2023.1162454
This article is part of the Research TopicArtificial Intelligence for EducationView all 11 articles
Introduction: Adaptive learning opportunities and individualized, timely feedback are considered to be effective support measures for students' writing in educational contexts. However, the extensive time and expertise required to analyze numerous drafts of student writing pose a barrier to teaching. Automated writing evaluation (AWE) tools can be used for individual feedback based on advances in Artificial Intelligence (AI) technology. A number of primary (quasi-)experimental studies have investigated the effect of AWE feedback on students' writing performance.
Methods: This paper provides a meta-analysis of the effectiveness of AWE feedback tools. The literature search yielded 4,462 entries, of which 20 studies (k = 84; N = 2, 828) met the pre-specified inclusion criteria. A moderator analysis investigated the impact of the characteristics of the learner, the intervention, and the outcome measures.
Results: Overall, results based on a three-level model with random effects show a medium effect (g = 0.55) of automated feedback on students' writing performance. However, the significant heterogeneity in the data indicates that the use of automated feedback tools cannot be understood as a single consistent form of intervention. Even though for some of the moderators we found substantial differences in effect sizes, none of the subgroup comparisons were statistically significant.
Discussion: We discuss these findings in light of automated feedback use in educational practice and give recommendations for future research.
Writing is a fundamental, versatile, and complex skill (Graham, 2019; Skar et al., 2022) that is required in a variety of contexts. Shortcomings in writing skills can thus hinder personal, academic, and professional success (Freedman et al., 2016; Graham et al., 2020). A basic aim of educational systems worldwide is to teach students to become competent writers; however, evidence suggests that while some students may achieve this goal, not all do (National Center for Educational Statistics, 2012, 2017; Graham and Rijlaarsdam, 2016). The situation is even further complicated by the fact that there is a large group of students from different language backgrounds who aspire to become competent writers in (English as) a second or foreign language and who are not always able to meet expectations (Fleckenstein et al., 2020a,b; Keller et al., 2020).
Writing skills are influenced by a variety of factors (Graham, 2018). Interindividual differences between writers are especially problematic as students with weak writing skills learn less in all school subjects compared to their more highly skilled classmates (Graham, 2019). In order to counteract this disadvantage, writing skills need to be promoted more in school. However, educational institutions often lack the time and personnel resources to do this. Graham (2019) reviewed 28 studies on writing instruction at school, identifying major indicators of inadequacy, including the insufficient instructional time devoted to writing (Brindle et al., 2015) and the absence of the use of digital tools for writing (Coker et al., 2016; Strobl et al., 2019; Williams and Beam, 2019).
In addition to high-quality, evidence-based teaching practice, digital technologies can be an asset in the individual promotion of writing skills. Automated writing evaluation (AWE) systems are able to assess students' writing performance, produce individualized feedback, and offer adaptive suggestions for writing improvement. Several individual empirical investigations have already looked into the employment of writing interventions with automated feedback tools, and some have investigated their effect on writing performance—with heterogeneous findings. Relevant moderators of effectiveness, however, have seldom been analyzed. The purpose of this study is to integrate the quantitative empirical literature on the subject of automated feedback interventions with a meta-analytic approach. Beyond the overall effect of automated feedback on student writing, we are particularly interested in moderating effects of learner and treatment characteristics.
Formative assessment serves to provide individualized learning support through a combination of (1) (standardized) learning progress evaluation, (2) individual task-related feedback, and (3) adaptive support for learners (Souvignier and Hasselhorn, 2018; Böhme and Munser-Kiefer, 2020). Implementing formative assessments is a challenge for educational systems, especially when it comes to higher-order competencies that require complex written responses from students. Assessing complex language performance as a necessary basis of individual feedback is a key challenge for teachers (Zhu and Urhahne, 2015; Fleckenstein et al., 2018). Especially judgment biases (e.g., tendencies toward leniency or severity; Jansen et al., 2019, 2021) and the use of simple heuristics in text assessment (e.g., text length; Fleckenstein et al., 2020a,b) can lead to inaccurate judgments of students' performance. Recent technological developments in the field of Artificial Intelligence (AI)—like AWE systems—can assist in the process of formative writing assessment.
The procedure of automatically scoring and evaluating students' written work through machine learning (ML) and natural language processing (NLP) techniques is known as automated writing evaluation (AWE; Bennett and Zhang, 2015). NLP is a subfield of AI that deals with the interaction between computers and humans using natural language. It involves the development of algorithms and systems that can understand, interpret, and generate human language. This includes ML algorithms, which learn from a large dataset of language examples and human ratings. When trained accordingly, AWE systems can evaluate a range of features of written text, including grammar, spelling, clarity, coherence, structure, and content. Based on these text features, they can assign scores to new texts and provide feedback to the writer (AWE feedback; Hegelheimer et al., 2016; Hockly, 2018).
AWE technology is utilized in a variety of educational contexts (Correnti et al., 2022), mainly for summative assessment purposes. Especially high-stakes standardized tests like the Graduate Record Exam (GRE) and the Test of English as a Foreign Language (TOEFL) have been using AWE technology for an automatic evaluation of students' writing (Zhang, 2021). In recent years, many tools have been developed to transfer this technology to low-stakes in-class writing tasks. The two major potentials of AWE with respect to formative assessment in writing are (a) assessment in terms of automatic evaluation of linguistically complex student responses and (b) individualized support through immediate and specific feedback based on students' performance. Various studies have demonstrated the quality of AWE assessment (Shermis, 2014; Perin and Lauterbach, 2018; Rupp et al., 2019; Zawacki-Richter et al., 2019). This review, however, focuses on the second part: Feedback that is based on the automated assessment. In the field of technology-supported writing instruction, this typically means supporting learners by providing adaptive automatic feedback on different textual aspects. While automatic assessment is not the central subject of this meta-analysis, it is the necessary foundation for adaptive feedback and individualized support. Therefore, automated assessment is an important inclusion criterion for the studies considered in this meta-analysis.
Feedback is generally considered to be one of the most effective factors influencing student learning. This is not only shown by a solid empirical research base (d = 0.62; Hattie, 2022) but is also consistent with teachers' professional beliefs (Fleckenstein et al., 2015). For writing feedback in particular, a meta-analysis by Graham et al. (2015) showed effect sizes ranging from d = 0.38 to d = 0.87, depending on the source of the feedback. Despite these positive findings, process-oriented feedback, in particular, is rarely used by teachers in the classroom as it requires a lot of time and effort (Graham and Hebert, 2011). Feedback has a particularly positive effect on learner performance when it is given in a timely manner when it clarifies the gap between current performance and learning goal, when it reduces cognitive load, and when it is task-related, specific, and detailed (Mory, 2004; Hattie and Timperley, 2007; Shute, 2008; Black and Wiliam, 2009).
In the context of automated text evaluation, the quality of machine judgments is often evaluated on the basis of their agreement with human judgments. In terms of reliability and validity, many studies have come up with satisfactory results in this regard (Shermis, 2014; Rupp et al., 2019; Latifi and Gierl, 2021). Human raters do not necessarily outperform technology in all areas of text evaluation. With respect to segmenting and analyzing texts, experts tend to make coding errors, whereas with respect to recognizing relationships between concepts, human raters have been shown to be superior to technology (Burkhart et al., 2020). Moreover, both human and machine ratings can be affected by judgment bias in that certain text features are disproportionately included in the judgments (Perelman, 2014; Fleckenstein et al., 2020a,b).
Especially for writing complex and long texts, the evidence of the effectiveness of automated feedback has been described heterogeneously (Stevenson and Phakiti, 2014; McNamara et al., 2015; Strobl et al., 2019). In addition, Graham et al. (2015) noted that few randomized controlled experimental studies had been published. Review articles have either looked at the use of digital technologies in writing instruction in general (Williams and Beam, 2019; Al-Wasy, 2020) or focused on tools and how they work rather than their effectiveness (Allen et al., 2016; Strobl et al., 2019; Deeva et al., 2021).
More recent systematic reviews on the effectiveness of AWE feedback provided an overview of the relevant empirical studies and identified research gaps (Nunes et al., 2021; Fleckenstein et al., 2023). However, they did not quantify the effect of automated feedback on performance and, thus, could not empirically investigate the heterogeneity of effects.
Two very recent meta-analyses have examined the effect of AWE systems on writing performance (Zhai and Ma, 2021; Ngo et al., 2022). Ngo et al. (2022) performed a meta-analysis of AWE systems within the context of second or foreign language education. They found an overall between-group effect size of g = 0.59 and investigated several moderating variables, including publication data, population data, and treatment data. Zhai and Ma (2021) also included studies on first language writing in their meta-analysis and found an effect size of g = 0.86 for AWE on overall writing quality. However, as outcome measures, the authors included holistic scores only, leaving out individual components of writing performance. The authors found significant moderating effects of educational level, target language learners, and genre of writing.
Our meta-analysis goes beyond the scope of the previous meta-analyses concerning methodological and theoretical considerations. Like Ngo et al. (2022), we used a three-level model with random effects to perform the meta-analysis. However, whereas both previous meta-analyses included post-test data only, we included pre-test performance in the between-group analyses to achieve a more accurate effect size estimation (Morris, 2008). This is especially relevant when drawing on non-randomized primary data (i.e., quasi-experimental designs), for which an equal distribution of pre-test scores across groups cannot be assumed. Furthermore, we used robust variance estimation (RVE) to account for the dependence of effect sizes. Like Zhai and Ma (2021), we included L1 and L2 writers; however, we did not limit the range of outcomes and thus covered holistic and analytic measures of writing performance. We also investigated relevant moderators that have been neglected so far, including the type and level of outcome, the type of control condition, and the time of measurement.
This meta-analysis addresses the two following research questions:
RQ1: What is the overall effect of automated feedback tools on student learning based on an integration of primary studies?
RQ2: To what extent is the effect of automated feedback tools moderated by sample, intervention, and outcome characteristics?
The analysis of the articles was conducted following the PRISMA (Preferred Reporting Items for Systematic Reviews and Meta-Analyses) model (Moher et al., 2009). This model provides an evidence-based minimum set of items for reporting reviews and meta-analyses. The selection and coding process for the articles was based on these standards.
In order to be included in the meta-analysis, studies needed to meet all of the pre-specified criteria regarding population, intervention, comparators, outcomes, and study design (PICOS) as specified below:
• Population: Students in primary, secondary and post-secondary education (ISCED level 1-7; UNESCO Institute for Statistics, 2012).
• Intervention: Automated writing evaluation (AWE) providing individualized or adaptive feedback to individual students.
• Comparators: Students receiving no feedback, non-automated feedback (e.g., teacher or peer feedback), or a less extensive form of AWE feedback.
• Outcomes: Writing performance (holistic or analystic) on a revision or transfer task.
• Study design: Experimental or quasi-experimental study designs with at least one treatment condition and one control condition.
Furthermore, studies had to be published in scholarly journals in order to be included. Studies investigating computer-mediated feedback by teachers or peers and studies on constructed responses in the context of short-answer formats were not considered in this meta-analysis.
The literature search was conducted in several literature databases (i.e., Ovid, PsycArticles, PsycInfo, Web of Science Core Collection, and ERIC), using various combinations of keywords: “automated writing evaluation;” “automated essay scoring;” writing + computer-assisted; writing + computer-based; writing + “intelligent tutoring system;” writing + “automated feedback;” writing + “electronic feedback;” writing + digital + feedback; writing + digital + scaffolding.
The literature search yielded in a total of N = 4,462 reports. After removing duplicates, individual abstracts were screened using the open-source software ASReview (Van de Schoot et al., 2021) for screening prioritization. The tool uses Machine Learning to assist researchers in the process of reviewing large numbers of scientific abstracts. Active learning models iteratively improve their predictions in ordering the abstracts for presentation to the researcher. This procedure has been shown to reduce the number of abstracts to be screened to <40% while retaining a detection rate of 95% of the relevant publications (Ferdinands, 2021). So the goal of ASReview is to help researchers reduce the time and effort required to conduct a literature review, while also improving the quality and comprehensiveness of the review. Based on this, n = 125 full texts were screened, identifying n = 20 studies that met the inclusion criteria. Figure 1 provides an overview of the literature search and screening process according to the PRISMA guidelines. Following the identification of relevant studies, a coding scheme was developed, and all studies were coded by two independent coders. Any coding that differed was discussed and reviewed by the first co-authors of this paper and corrected if necessary. The variables that were coded and included in the moderator analyses are described in Section 4.5.
Figure 1. Flow-chart of the literature search and screening process (adapted from Moher et al., 2009).
The standardized mean differences, also known as Cohen's d, between treatment and control conditions were calculated using the R package esc (Lüdecke, 2019). For studies that did not report raw statistics (e.g., means and standard deviation), we calculated Cohen's d based on other statistical indices (e.g., F- or t-values).
Morris (2008) recommended an effect size calculation based on the mean pre-post change in the treatment group minus the mean pre-post change in the control group, divided by the pooled pre-test standard deviation. This method was shown to be superior in terms of bias, precision, and robustness to the heterogeneity of variance. Thus, whenever pre-test values were available, they were considered in addition to the post-test values (also see Lipsey and Wilson, 2001; Wilson, 2016; Lüdecke, 2019). In further analyses, we conducted the same model but without considering the corresponding pre-test values to evaluate potential differences in results.
It has been found that Cohen's d tends to overestimate the true effect size when the study sample size is small (Grissom and Kim, 2005), which is the case in some of the included primary studies. Therefore, all Cohen's d values were converted into Hedges' g, which is an unbiased estimator that takes into account the sample sizes (Hedges, 1981):
To verbally classify the effect sizes, we used a heuristic derived from the distribution of effects in this research field. This considered the 33rd and 67th percentile of the absolute value of all effects found in this meta-analysis: effects smaller than the 33rd were described as small, effects between the 33rd and 67th percentiles were described as medium, and effects greater than the 67th percentile were described as large (see Kraft, 2020, for a discussion on how to classify effect sizes; Jansen et al., 2022).
We combined the effect sizes of the included studies by applying a three-level model with random effects to take into account that several studies of our meta-analysis reported more than one effect size (Geeraert et al., 2004; Konstantopoulos, 2011; Cheung, 2014; Van den Noortgate et al., 2015; Assink and Wibbelink, 2016). This three-level model considers three levels of variance: variance of the extracted effect sizes at level 1 (sampling variance); variance between effect sizes of a single study at level 2 (within-study variance); and variance between studies at level 3 (between-study variance). Thus, this hierarchical model accounts for the variation of effect sizes between participants (level 1), outcomes (level 2), and studies (level 3).
The multilevel approach is a statistical approach that does not require the correlations between outcomes within primary studies to be known in order to estimate the covariance matrix of the effect sizes. Instead, the second level of the three-level meta-analytic model accounts for sampling covariation (Van den Noortgate et al., 2013). Also, the three-level approach allows for examining differences in outcomes within studies (i.e., within-study heterogeneity) as well as differences between studies (i.e., between-study heterogeneity). If a study reported multiple effect sizes from the same sample that could not be treated as independent from each other, we accounted for this non-independence by using the cluster-robust inference method (also called robust variance estimation; RVE; Sidik and Jonkman, 2006; Hedges et al., 2010; Tipton and Pustejovsky, 2015). This estimation allows for the integration of statistically dependent effect sizes within a meta-analysis without the need for knowledge of the covariance structure among the effect sizes. Furthermore, we conducted moderator analyses to test variables that may reduce within-study or between-study heterogeneity. For these analyses, the three-level random effects model can easily be extended by study and effect size characteristics into a three-level mixed-effects model.
The amount of heterogeneity (i.e., τ2), was estimated using the restricted maximum-likelihood estimator (Viechtbauer, 2005). In addition to the estimate of τ2, the Q-test for heterogeneity (Cochran, 1954) and the τ2 statistic (Higgins and Thompson, 2002) are reported. In case any amount of heterogeneity is detected (i.e., τ2>0, regardless of the results of the Q-test), a prediction interval for the true effect is provided (Riley et al., 2011). The regression test (Sterne and Egger, 2005), using the standard error of the observed outcomes as a predictor, is used to check for funnel plot asymmetry. The analysis was carried out using R (version 4.1.2; R Core Team, 2021) and the metafor package (Viechtbauer, 2010) to perform the meta-analyses. In addition, we used the clubSandwich package (Pustejovsky, 2022) to perform the cluster-robust inference method.
In combination with the consideration of heterogeneity in our data and calculated effect sizes, we performed several moderator analyses. Moderator variables can be used to provide a more meaningful interpretation of the data and reduce the heterogeneity of the overall effect. First, we identified possible moderator variables from the full texts of the primary studies: sample characteristics (educational level and language status); Intervention characteristics (treatment duration and type of control condition); outcomes characteristics (time of measurement, type of outcome, and outcome level). Second, the n = 20 studies included in the meta-analysis were coded by two authors of this study. Third, based on the final codes, the primary studies were divided into subgroups or factors that potentially explain the variance of the observed overall effect. In the following, the coded variables are explained in more detail.
Studies that examined the effect of individual AWE feedback in high school (secondary level) were separated from studies that investigated higher education (tertiary level) students.
As a sample characteristic, we coded language status into L1 for first or majority language contexts and L2 for second or foreign language contexts.
Interventions differed greatly in their duration, ranging from 50 min to one semester. Thus, we categorized intervention duration into short (one or two sessions) and long (more than two sessions).
The studies differed in their design with respect to the control group. In some studies, the control condition received no feedback of any kind on their writing; in other studies, the control condition received a different kind of feedback than the intervention group, such as teacher feedback, peer feedback, or a less extensive form of AWE feedback.
The reported effects were classified as either post-test performance (directly after the intervention) or follow-up performance (time gap between intervention and test.
Most studies on AWE feedback consider either the performance on a text revision or the performance on a different writing task. These outcomes differ in their conceptualization, as a successful revision can be considered performance improvement and a successful transfer to a new task can be considered learning.
Furthermore, outcomes were categorized according to the level of detail. Outcomes were considered holistic when the effect referred to a total score or grade for the whole text. Analytic outcomes were further differentiated for effects concerning language aspects (e.g., grammar and mechanics) or content aspects (e.g., unity and number of subthemes) of the text.
A total of k = 84 effect sizes involving N = 2,828 learners from 20 studies were included in the analysis. The observed effects ranged from −1.73 to 2.99, with the majority of estimates being positive (70.24%). The estimated average effect size based on the three-level model with random effects was g = 0.55 (SE = 0.17) and differed significantly from zero (z = 3.18, p < 0.001). The comparison of the three-level model with the conventionally two-level model showed a significantly better fit for the three-level model, based on the likelihood ratio test (X2 = 150.36; p < 0.001). Therefore, the application of the three-level model would better explain the between-group comparison data.
According to the Q-test, the effect showed significant heterogeneity (see Table 1). The estimated variance values were τ2 for level 3 = 0.51 and τ2 for level 2 = 0.02. A 95% prediction interval for the estimated effect is given by −1.02 to 2.12. Hence, although the average effect is estimated to be positive, in some studies, the true effect may, in fact, be negative.
As a further analysis, we examined whether the overall effect sizes differ when we ignore pre-test values from primary studies that provided them and calculated the effect sizes based only on the post-test values from the studies in concern (see Table 2). We observed an estimated average effect of g = 0.77 (SE = 0.20). The observed effects ranged from −1.14 to 3.61, with the majority of estimates being positive (70.24%). Therefore, the average effect differed significantly from zero (z = 3.87, p < 0.001). However, a Wald test showed that both effect sizes did not significantly differ from each other (Q = 0.67, p = 0.414).
To examine a publication bias, we used a funnel plot to see whether there is a symmetry of effect sizes, as they should be evenly distributed on both sides of the centered line, which represents the overall average effect sizes across all unique samples (Figure 2). In addition, we ran an Egger's test to evaluate the statistical significance of the asymmetry of the funnel plot by using the squared standard errors of the effect size estimates as a predictor in the meta-regression (Sterne and Egger, 2005). The results of the test confirmed that our funnel plot asymmetry is not different from zero (b = −0.76, SE = 0.69, z = −1.09, p = 0.27, 95% CI [−2.12 - 0.60]), indicating that there are no conspicuous data characteristics, producing an asymmetric inverted funnel plot. Therefore, we can assume the absence of a significant publication bias.
To test our hypotheses, we computed a random effects model with subgroup and regression effects of our coded moderator variables (see Table 3). For verbal classification, we used the 33rd percentile (g = 0.23) and the 67th percentile (g = 0.60) of the absolute values of the effects. Thus, effects below g = 0.23 were classified as “small,” effects between g = 0.23 and g = 0.60 were classified as “medium,” and effects above g = 0.60 were classified as “large.” The moderators were grouped into three categories: sample characteristics, intervention characteristics, and outcome characteristics.
We found medium effects for both secondary level (0.50) and tertiary level (0.58) that were both significantly different from zero. The difference between the two effects was not statistically significant (Q = 0.03, p = 0.854).
For samples with the target language as L1, we found a medium effect (0.40); for those with an L2 background, the effect can be categorized as large (0.72). Both effects significantly differed from zero. The effects did not significantly differ from each other (Q = 0.82, p = 0.365).
Long interventions of more than two sessions showed a large significant effect (0.66), whereas short interventions of one or two sessions showed a small non-significant effect (0.18). However, the difference between the two effects was not statistically significant (Q = 1.32, p = 0.250).
For those Intervention groups that were compared to a control condition without any kind of feedback, the effect was significant and of medium size (0.59). When compared to a group with a different kind of feedback, the medium effect (0.40) was not significantly different from zero. However, the difference between effects was not statistically significant (Q = 0.16, p = 0.684).
For both post-test performance (0.57) and follow-up performance (0.27), we found effects that fall in the medium category. However, the follow-up effect did not significantly differ from zero, whereas the effect on post-test performance did. The difference between the effects was marginally significant on the 10%-level (Q = 2.71, p = 0.099).
The medium effect (0.27) for revision tasks as the outcome (performance) was not significantly different from zero. For transfer tasks (learning), the effect was large and significant (0.65). Again, the difference between effects was not statistically significant (Q = 1.75, p = 0.186).
The effects of the three outcomes were all of medium-large size (holistic: 0.50; content: 0.57; language: 0.61), and they were all significantly different from zero. The three effects did not significantly differ from each other (Q = 0.51, p = 0.773).
In the following, we discuss the central findings of this meta-analysis. Before we provide insight into automated feedback use in educational practice and give recommendations for future research, we briefly summarize our findings regarding the overall effect of AWE feedback and the moderator analyses.
This meta-analysis examined the overall effect of AWE feedback on writing performance by collecting 84 effect sizes from 20 primary studies with a total of 2,828 participants. A medium effect size of g = 0.55 was obtained using a three-level random-effects model. The findings support the use of AWE feedback to facilitate students' writing development.
The effect size is in line with prior meta-analytic research by Ngo et al. (2022), who found an overall between-group effect of g = 0.59. However, it is considerably smaller than the effect of g = 0.86 found by Zhai and Ma (2022). This variance in effect sizes may be due to the fact that the latter meta-analysis did not use a three-level model for their data analysis. Thus, they did not account for the dependence of effects reported within one study. They also did not include pre-test performance in their model; however, neither did Ngo et al. (2022).
Our robustness check showed that neglecting the pre-test performance in this research area could lead to an overestimation of the overall effect size (g = 0.77). However, this effect—although verbally classified as a large effect—did not significantly differ from the medium effect found in the original analysis.
Since the data showed significant heterogeneity, we investigated the impact of several potential moderators, including characteristics of the sample, the intervention, and the outcome. Even though for some of the moderators, we found substantial differences in effect sizes, none of the subgroup comparisons were statistically significant. This should be kept in mind when verbally classifying the effect sizes. In the following, we interpret our findings in light of previous research, especially the two recent meta-analyses by Ngo et al. (2022) and Zhai and Ma (2022).
Sample characteristics included the educational level and the language context. We differentiated for secondary and tertiary level, finding similar effects of medium size for both. This is contrary to the findings by Ngo et al. (2022) and Zhai and Ma (2022), who both found larger effects for post-secondary learners compared to learners at secondary level. However, both previous meta-analyses only included a very limited number of primary studies drawing on secondary-level samples (k = 3 resp. k = 6). Thus, it can be assumed that AWE feedback is similarly effective in both contexts. In terms of language context, the effect was large for L2 and medium for L1 contexts. Zhai and Ma (2022) reported a similar finding when comparing learners of English as a second or foreign language with native English speakers.
We found a large effect for long-term AWE feedback treatments but only a small effect for short interventions. This is in line with Ngo et al. (2022), who even found a small negative effect for short durations (≤ 2 weeks). The difference between medium and long intervention durations in Zhai and Ma (2022), however, was also not statistically significant. Zhai and Ma (2022) did also not find a significant effect for feedback combination (AWE only vs. AWE + teacher vs. AWE + peer). We took a slightly different approach and investigated different control conditions, some of which did not receive any feedback treatment and some of which received a different feedback treatment (e.g., teacher or peer feedback). Contrary to expectations, the medium-size effects did not differ significantly for the two types of control conditions.
Even though many studies in this field report post-test as well as follow-up outcomes, neither of the two prior meta-analyses investigated this as a moderator. We found the overall effect on post-test performance to be of medium size and not significantly different from zero; the effect on follow-up performance was small and did not significantly differ from zero. Again, in direct comparison, the difference between effects did not reach statistical significance. Neither of the previous meta-analyses looked into the type of outcome (i.e., performance vs. learning), even though this is a striking difference between studies that could explain the heterogeneity. To our surprise, the effect for revision tasks (performance improvement) was small, whereas the effect for transfer tasks (learning) was large. Only the latter differed significantly from zero. This indicates that AWE feedback does have an impact on learning to write rather than on situational performance enhancement. Unfortunately, the number of studies available does not suffice to investigate interactions of type of outcome with other moderator variables. Zhai and Ma (2022) only investigate holistic text quality as an outcome. Ngo et al. (2022) investigated outcome measure as a moderator with seven categories, finding effect sizes that ranged from g = 0.27 (Grammar and Mechanics) to g = 0.83 (Vocabulary). However, the effect sizes did not significantly differ from each other, probably due to small subgroup sizes. In our analysis of holistic and analytic (content, language) outcomes, we found very similar effects of medium size. More research on outcome measures as moderators of AWE feedback effectiveness is needed to investigate differential effects more closely.
Even though effects differed in size for some of the moderators, these differences were not statistically significant. Thus, the detected heterogeneity may be explained by variables other than the ones that we attended to in our moderator analyses. Thus, in future research, additional moderators need to be investigated. In other learning contexts, the type of feedback has been shown to moderate effectiveness (Van der Kleij et al., 2015; Wisniewski et al., 2020; Mertens et al., 2022). In the context of AWE feedback, we need more primary studies that compare different types of feedback or at least provide sufficient information on the details of their feedback intervention. Moreover, the design and presentation of automated feedback have rarely been investigated (for an exception, see Burkhart et al., 2020).
The potential to identify publication bias in a certain area of research is one of the strengths of meta-analytic research. We assessed publication bias by testing the asymmetry of the funnel plot, finding no indicator for bias. However, a more thorough analysis of publication bias is needed. In order to find out whether non-significant or small effects of AWE feedback tend to remain unpublished, the respective meta-analysis should include unpublished or non-peer-reviewed primary studies.
The variance in estimated effect sizes across AWE feedback meta-analyses calls for a second-order meta-analysis. The purpose of a second-order meta-analysis is to estimate the proportion of the variance in meta-analytic effect sizes across multiple first-order meta-analyses attributable to second-order sampling error and to use this information to improve the accuracy of estimation for each first-order meta-analytic estimate (Schmidt and Oh, 2013). Thus, a second-order meta-analysis would inform AWE feedback research and provide a more comprehensive understanding of factors influencing AWE feedback effectiveness.
This meta-analysis showed that AWE feedback has a medium positive effect on students' writing performance in educational contexts. However, the heterogeneity in the data suggests that automated feedback should not be seen as a one-size-fits-all solution, and its impact may vary based on factors such as context and learner characteristics, the feedback intervention itself, and outcome measures.
For teachers and school administrators, this implies that AWE feedback can be a useful tool to support students' writing in educational contexts, but its use should be carefully considered and integrated into a comprehensive approach to writing instruction. The use of automated feedback should be combined with other forms of support, such as teacher feedback and individualized learning opportunities, to ensure its effectiveness.
Furthermore, the heterogeneity in the results suggests that automated feedback may not have the same impact on all students. Teachers and administrators should consider the individual needs and characteristics of their students when deciding whether and how to implement automated feedback. Further research is needed to determine the most effective use of automated feedback in different educational contexts and with different populations. Teachers and administrators should keep up to date with developments in the field and use evidence-based practices to inform their decisions.
The original contributions presented in the study are included in the article/supplementary material, further inquiries can be directed to the corresponding author.
JF, LL, and JM contributed to conception and design of the study. JF and LL conducted the literature search, screening procedure, and coding. LL performed the statistical analysis and wrote sections of the manuscript. JF wrote the first draft of the manuscript. All authors have read and approved the submitted version.
The authors declare that the research was conducted in the absence of any commercial or financial relationships that could be construed as a potential conflict of interest.
All claims expressed in this article are solely those of the authors and do not necessarily represent those of their affiliated organizations, or those of the publisher, the editors and the reviewers. Any product that may be evaluated in this article, or claim that may be made by its manufacturer, is not guaranteed or endorsed by the publisher.
Allen, L. K., Jacovina, M. E., and McNamara, D. S. (2016). “Computer-based writing instruction,” in Handbook of Writing Research, eds C. A. MacArthur, S. Graham, and J. Fitzgerald (The Guilford Press), pp. 316–329.
Al-Wasy, B. Q. (2020). The effectiveness of integrating technology in EFL/ESL writing: A meta-analysis. Interact. Technol. Smart Educ. 2020, 33. doi: 10.1108/ITSE-03-2020-0033
Assink, M., and Wibbelink, C. J. (2016). Fitting three-level meta-analytic models in R: A step-by-step tutorial. Quantit. Methods Psychol. 12, 154–174. doi: 10.20982/tqmp.12.3.p154
Bennett, R. E., and Zhang, M. (2015). “Validity and automated scoring,” in Technology and Testing, ed F. Drasgow (London: Routledge), 142–173.
Black, P., and Wiliam, D. (2009). Developing the theory of formative assessment. Educat. Assess. Eval. Accountabil. 21, 5–31. doi: 10.1007/s11092-008-9068-5
Böhme, R., and Munser-Kiefer, M. (2020). Lernunterstützung mit digitalen Unterrichtsmaterialien: Interdisziplinäre Erkenntnisse und Entwicklungsperspektiven. MedienPädagogik 17, 427–454. doi: 10.21240/mpaed/jb17/2020.05.17.X
Brindle, M., Graham, S., Harris, K. R., and Hebert, M. (2015). Third and fourth grade teacher's classroom practices in writing: A national survey. Read. Writ. 29, 929–954. doi: 10.1007/s11145-015-9604-x
Burkhart, C., Lachner, A., and Nückles, M. (2020). Assisting students' writing with computer-based concept map feedback: A validation study of the CohViz feedback system. PLoS ONE. 15, e0235209. doi: 10.1371/journal.pone.0235209
*Cheng, G. (2017). The impact of online automated feedback on students' reflective journal writing in an EFL course. Internet High. Educ. 34, 18–27. doi: 10.1016/j.iheduc.2017.04.002
Cheung, M. W.-L. (2014). Modeling dependent effect sizes with three-level meta-analyses: A structural equation modeling approach. Psychol. Methods 19, 211. doi: 10.1037/a0032968
*Chew, C. S., Idris, N., Loh, E. F., Wu, W. C. V., Chua, Y. P., and Bimba, A. T. (2019). The effects of a1 theory-based summary writing tool on students' summary writing. J. Comput. Assist. Learn. 35, 435–449. doi: 10.1111/jcal.12349
Cochran, W. G. (1954). The combination of estimates from different experiments. Biometrics 10, 101–129. doi: 10.2307/3001666
Coker, D. L., Farley-Ripple, E., Jackson, A. F., Wen, H., MacArthur, C. A., and Jennings, A. S. (2016). Writing instruction in first grade: An observational study. Read. Writ. 29, 793–832. doi: 10.1007/s11145-015-9596-6
Correnti, R., Matsumura, L. C., Wang, E. L., Litman, D., and Zhang, H. (2022). Building a validity argument for an automated writing evaluation system (eRevise) as a formative assessment. Comput Educ. Open. doi: 10.1016/j.caeo.2022.100084
Deeva, G., Bogdanova, D., Serral, E., Snoeck, M., and De Weerdt, J. (2021). A review of automated feedback systems for learners: Classification framework, challenges and opportunities. Comput Educ. 162. doi: 10.1016/j.compedu.2020.104094
Ferdinands, G. (2021). AI-assisted systematic reviewing: Selecting studies to compare bayesian versus frequentist SEM for small sample sizes. Multivar. Behav. Res. 56, 153–154. doi: 10.1080/00273171.2020.1853501
Fleckenstein, J., Keller, S., Krüger, M., Tannenbaum, R., and Köller, O. (2020a). Linking TOEFL iBT® writing rubrics to CEFR levels: Cut scores and validity evidence from a standard setting study. Assess. Writ. 43, 100420. doi: 10.1016/j.asw.2019.100420
Fleckenstein, J., Leucht, M., and Köller, O. (2018). Teachers' judgement accuracy concerning CEFR levels of prospective university students. Lang. Assess. Quart. 15, 90–101. doi: 10.1080/15434303.2017.1421956
Fleckenstein, J., Meyer, J., Jansen, T., Keller, S., and Köller, O. (2020b). Is a long essay always a good essay? The effect of text length on writing assessment. Front. Psychol. 11, 562462. doi: 10.3389/fpsyg.2020.562462
Fleckenstein, J., Reble, R., Meyer, J., Jansen, T., Liebenow, L. W., Möller, J., et al. (2023). “Digitale Schreibförderung im Bildungskontext: Ein systematisches Review,” in Bildung für eine digitale Zukunft, Vol. 15, eds K. Scheiter, and I. Gogolin (Wiesbaden: Springer VS), 3–25. doi: 10.1007/978-3-658-37895-0_1
Fleckenstein, J., Zimmermann, F., Köller, O., and Möller, J. (2015). What works in school? Expert and novice teachers' beliefs about school effectiveness. Frontl. Learn. Res. 3, 27–46. doi: 10.14786/flr.v3i2.162
Freedman, S. W., Hull, G. A., Higgs, J. M., and Booten, K. P. (2016). Teaching writing in a digital and global age: Toward access, learning, and development for all. Am. Educ. Res. Assoc. 6, 23. doi: 10.3102/978-0-935302-48-6_23
*Gao, J., and Ma, S. (2019). The effect of two forms of computer-automated metalinguistic corrective feedback. Lang. Learn. Technol. 23, 65–83.
Geeraert, L., Van den Noortgate, W., Grietens, H., and Onghena, P. (2004). The effects of early prevention programs for families with young children at risk for physical child abuse and neglect: A meta-analysis. Child Maltreat. 9, 277–291. doi: 10.1177/1077559504264265
Graham, S. (2018). A revised writer (s)-within-community model of writing. Educ. Psycholog. 53, 258–279. doi: 10.1080/00461520.2018.1481406
Graham, S. (2019). Changing how writing is taught. Rev. Res. Educ. 43, 277–303. doi: 10.3102/0091732X18821125
Graham, S., and Hebert, M. (2011). Writing to read: A meta-analysis of the impact of writing and writing instruction on reading. Harv. Educ. Rev. 81, 710–744. doi: 10.17763/haer.81.4.t2k0m13756113566
Graham, S., Hebert, M., and Harris, K. R. (2015). Formative assessment and writing. Element. School J. 115, 523–547. doi: 10.1086/681947
Graham, S., Kiuhara, S. A., and MacKay, M. (2020). The effects of writing on learning in science, social studies, and mathematics: A meta-analysis. Rev. Educ. Res. 90, 179–226. doi: 10.3102/0034654320914744
Graham, S., and Rijlaarsdam, G. (2016). Writing education around the globe: Introduction and call for a new global analysis. Read. Writ. 29, 781–792. doi: 10.1007/s11145-016-9640-1
Grissom, R. J., and Kim, J. J. (2005). Effect Sizes for Research: A Broad Practical Approach. Mahwah, NJ: Lawrence Erlbaum Associates Publishers.
*Hassanzadeh, M., and Fotoohnejad, S. (2021). Implementing an automated feedback program for a foreign language writing course: A learner-centric study: Implementing an AWE tool in a L2 class. J. Comput. Assist. Learn. 37, 1494–1507. doi: 10.1111/jcal.12587
Hattie, J. (2009). The black box of tertiary assessment: An impending revolution. Tertiary Assess. High. Educ. Stud. Outcomes 259, 275.
Hattie, J. (2022). Visible Learning Metax: Feedback. Available online at: http://www.visiblelearningmetax.com/Influences (accessed January 20, 2023).
Hattie, J., and Timperley, H. (2007). The power of feedback. Rev. Educ. Res. 77, 81–112. doi: 10.3102/003465430298487
Hedges, L. V. (1981). Distribution theory for glass's estimator of effect size and related estimators. J. Educ. Stat. 6, 107–128. doi: 10.3102/10769986006002107
Hedges, L. V., Tipton, E., and Johnson, M. C. (2010). Robust variance estimation in meta-regression with dependent effect size estimates. Res. Synth. Methods 1, 39–65. doi: 10.1002/jrsm.5
Hegelheimer, V., Dursun, A., and Li, Z. (2016). Automated writing evaluation in language teaching: Theory, development, and application. CALICO J. 33, I–V. doi: 10.1558/cj.v33i1.29251
Higgins, J. P., and Thompson, S. G. (2002). Quantifying heterogeneity in a meta-analysis. Stat. Med. 21, 1539–1558. doi: 10.1002/sim.1186
Jansen, T., Meyer, J., Wigfield, A., and Möller, J. (2022). Which student and instructional variables are most strongly related to academic motivation in K-12 education? A systematic review of meta-analyses. Psychol. Bullet. 148, 1–26. doi: 10.1037/bul0000354
Jansen, T., Vögelin, C., Machts, N., Keller, S., Köller, O., and Möller, J. (2021). Judgment accuracy in experienced vs. student teachers: Assessing essays in english as a foreign language. Teach. Teacher Educ. 97, 103216. doi: 10.1016/j.tate.2020.103216
Jansen, T., Vögelin, C., Machts, N., Keller, S., and Möller, J. (2019). Das Schülerinventar ASSET zur Beurteilung von Schülerarbeiten im Fach Englisch: Drei experimentelle Studien zu Effekten der Textqualität und der Schülernamen. Psychologie in Erziehung Und Unterricht 2019, art21d. doi: 10.2378/peu2019.art21d
Keller, S. D., Fleckenstein, J., Krüger, M., Köller, O., and Rupp, A. A. (2020). English writing skills of students in upper secondary education: Results from an empirical study in Switzerland and Germany. J. Second Lang. Writ. 48, 100700. doi: 10.1016/j.jslw.2019.100700
*Kellogg, R. T., Whiteford, A. P., and Quinlan, T. (2010). Does automated feedback help students learn to write? J. Educ. Comput. Res. 42, 173–196. doi: 10.2190/EC.42.2.c
Klein, P., and Boscolo, P. (2016). Trends in research on writing as a learning activity. J. Writ. Res. 7, 311–350. doi: 10.17239/jowr-2016.07.03.01
Konstantopoulos, S. (2011). Fixed effects and variance components estimation in three-level meta-analysis. Res. Synth. Methods 2, 61–76. doi: 10.1002/jrsm.35
Kraft, M. A. (2020). Interpreting effect sizes of education interventions. Educ. Research. 49, 241–253. doi: 10.3102/0013189X20912798
*Lachner, A., Burkhart, C., and Nückles, M. (2017). Mind the gap! Automated concept map feedback supports students in writing cohesive explanations. J. Exp. Psychol. 23, 29. doi: 10.1037/xap0000111
Latifi, S., and Gierl, M. (2021). Automated scoring of junior and senior high essays using Coh-Metrix features: Implications for large-scale language testing. Lang. Test. 38, 62–85. doi: 10.1177/0265532220929918
*Lin, M. P. C., and Chang, D. (2020). Enhancing post-secondary writers' writing skills with a chatbot. J. Educ. Technol. Soc. 23, 78–92.
*Link, S., Mehrzad, M., and Rahimi, M. (2022). Impact of automated writing evaluation on teacher feedback, student revision, and writing improvement. Comput. Assist. Lang. Learn. 35, 605–634. doi: 10.1080/09588221.2020.1743323
Lipsey, M. W., and Wilson, D. B. (2001). Practical Meta-analysis. Thousand Oaks, CA: SAGE Publications, Inc.
*Lu, X. (2019). An empirical study on the artificial intelligence writing evaluation system in china CET. Big Data 7, 121–129. doi: 10.1089/big.2018.0151
Lüdecke, D. (2019). ESC: Effect Size Computation for Meta-analysis (Version 0.5.1). doi: 10.5281/zenodo.1249218
*McCarthy, K. S., Roscoe, R. D., Likens, A. D., and McNamara, D. S. (2019). “Checking it twice: Does adding spelling and grammar checkers improve essay quality in an automated writing tutor?” in Artificial Intelligence in Education: 20th International Conference, AIED 2019, Chicago, IL, USA, June 25-29. 2019, Proceedings, Part i 20, Chicago, IL, 270–282. doi: 10.1007/978-3-030-23204-7_23
McNamara, D. S., Crossley, S. A., Roscoe, R. D., Allen, L. K., and Dai, J. (2015). A hierarchical classification approach to automated essay scoring. Assess. Writ. 23, 35–59. doi: 10.1016/j.asw.2014.09.002
Mertens, U., Finn, B., and Lindner, M. A. (2022). Effects of computer-based feedback on lower- and higher-order learning outcomes: A network meta-analysis. J. Educ. Psychol. 114, edu0000764. doi: 10.1037/edu0000764
*Moher, D., Liberati, A., Tetzlaff, J., Altman, D. G., and Group, P. (2009). Preferred reporting items for systematic reviews and meta-analyses: The PRISMA statement. Ann. Internal Med. 151, 264–269. doi: 10.7326/0003-4819-151-4-200908180-00135
*Mørch, A. I., Engeness, I., Cheng, V. C., Cheung, W. K., and Wong, K. C. (2017). EssayCritic: Writing to learn with a knowledge-based design critiquing system. J. Educ. Technol. Soc. 20, 213–223.
Morris, S. B. (2008). Estimating effect sizes from pretest-posttest-control group designs. Organ. Res. Methods 11, 364–386. doi: 10.1177/1094428106291059
Mory, E. (2004). “Feedback Research Revisited,” in Handbook of Research on Educational, Communications and Technology, eds D. Jonasson and M. Driscoll (New York, NY: Routledge), pp. 745–783.
National Center for Educational Statistics (2012). The Nation's Report Card: Writing 2011. Available online at: https://nces.ed.gov/nationsreportcard/pdf/main2011/2012470.pdf (accessed January 20, 2023).
National Center for Educational Statistics (2017). Technical Summary of Preliminary Analyses of NAEP 2017 Writing Assessments. Available online at: https://nces.ed.gov/nationsreportcard/subject/writing/pdf/2017_writing_technical_summary.pdf (accessed January 20, 2023).
Ngo, T. T. N., Chen, H. H. J., and Lai, K. K. W. (2022). The effectiveness of automated writing evaluation in EFL/ESL writing: A three-level meta-analysis. Interact. Learn. Environ. 2022, 1–18. doi: 10.1080/10494820.2022.2096642
Nunes, A., Cordeiro, C., Limpo, T., and Castro, S. L. (2021). Effectiveness of automated writing evaluation systems in school settings: A systematic review of studies from 2000 to 2020. J. Comput. Assist. Learn. 38, 599–620. doi: 10.1111/jcal.12635
*Palermo, C., and Thomson, M. M. (2018). Teacher implementation of self-regulated strategy development with an automated writing evaluation system: Effects on the argumentative writing performance of middle school students. Contempor. Educ. Psychol. 54, 255–270. doi: 10.1016/j.cedpsych.2018.07.002
Perelman, L. (2014). When “the state of the art” is counting words. Assess. Writ. 21, 104–111. doi: 10.1016/j.asw.2014.05.001
Perin, D., and Lauterbach, M. (2018). Assessing text-based writing of low-skilled college students. Int. J. Artif. Intellig. Educ. 28, 56–78. doi: 10.1007/s40593-016-0122-z
Pustejovsky, J. (2022). clubSandwich: Cluster-Robust (Sandwich) Variance Estimators With Small-Sample Corrections. Available online at: https://CRAN.R-project.org/package=clubSandwich (accessed January 20, 2023).
R Core Team (2021). R: A Language and Environment for Statistical Computing. R Foundation for Statistical Computing. Available online at: https://www.R-project.org/ (accessed January 20, 2023).
*Reynolds, B. L., Kao, C.-W., and Huang, Y. (2021). Investigating the effects of perceived feedback source on second language writing performance: A quasi-experimental study. Asia-Pacific Educ. Research. 30, 585–595. doi: 10.1007/s40299-021-00597-3
*Riedel, E., Dexter, S. L., Scharber, C., and Doering, A. (2006). Experimental evidence on the effectiveness of automated essay scoring in teacher education cases. J. Educ. Comput. Res. 35, 267–287. doi: 10.2190/U552-M54Q-5771-M677
Riley, R. D., Higgins, J. P., and Deeks, J. J. (2011). Interpretation of random effects meta-analyses. Br. Med. J. 342, d549. doi: 10.1136/bmj.d549
Rupp, A. A., Casabianca, J. M., Krüger, M., Keller, S., and Köller, O. (2019). Automated essay scoring at scale: A case study in Switzerland and Germany. ETS Res. Rep. Ser. 2019, 1–23. doi: 10.1002/ets2.12249
Schmidt, F. L., and Oh, I. S. (2013). Methods for second order meta-analysis and illustrative applications. Org. Behav. Hum. Decision Process. 121, 204–218. doi: 10.1016/j.obhdp.2013.03.002
Shermis, M. D. (2014). State-of-the-art automated essay scoring: Competition, results, and future directions from a united states demonstration. Assess. Writ. 20, 53–76. doi: 10.1016/j.asw.2013.04.001
Shute, V. J. (2008). Focus on formative feedback. Rev. Educ. Res. 78, 153–189. doi: 10.3102/0034654307313795
Sidik, K., and Jonkman, J. N. (2006). Robust variance estimation for random effects meta-analysis. Comput. Stat. Data Anal. 50, 3681–3701. doi: 10.1016/j.csda.2005.07.019
Skar, G. B., Graham, S., and Rijlaarsdam, G. (2022). Formative writing assessment for change—introduction to the special issue. Assess. Educ. Principl. Pol. Practice 29, 121–126. doi: 10.1080/0969594X.2022.2089488
Souvignier, E., and Hasselhorn, M. (2018). Formatives assessment. Zeitschrift für Erziehungswissenschaft. 21, 693–696. doi: 10.1007/s11618-018-0839-6
Sterne, J. A., and Egger, M. (2005). Regression methods to detect publication and other bias in meta-analysis. Publicat. Bias Meta-Analysis 6, 99–110. doi: 10.1002/0470870168.ch6
Stevenson, M., and Phakiti, A. (2014). The effects of computer-generated feedback on the quality of writing. Assess. Writ. 19, 51–65. doi: 10.1016/j.asw.2013.11.007
Strobl, C., Ailhaud, E., Benetos, K., Devitt, A., Kruse, O., Proske, A., et al. (2019). Digital support for academic writing: A review of technologies and pedagogies. Comput. Educ. 131, 33–48. doi: 10.1016/j.compedu.2018.12.005
*Tang, J., and Rich, C. S. (2017). Automated writing evaluation in an EFL setting: Lessons from china. JALT CALL J. 13, 117–146. doi: 10.29140/jaltcall.v13n2.215
Tipton, E., and Pustejovsky, J. E. (2015). Small-sample adjustments for tests of moderators and model fit using robust variance estimation in meta-regression. J. Educ. Behav. Stat. 40, 604–634. doi: 10.3102/1076998615606099
UNESCO Institute for Statistics (2012). International Standard Classification of Education: ISCED 2011. Available online at: https://uis.unesco.org/sites/default/files/documents/international-standard-classification-of-education-isced-2011-en.pdf (accessed January 20, 2023).
Van de Schoot, R., de Bruin, J., de Schram, R., Zahedi, P., de Boer, J., de Weijdema, F., et al. (2021). An open source machine learning framework for efficient and transparent systematic reviews. Nat. Machine Intellig. 3, 125–133. doi: 10.1038/s42256-020-00287-7
Van den Noortgate, W., López-López, J. A., Marin-Martinez, F., and Sánchez-Meca, J. (2013). Three-level meta-analysis of dependent effect sizes. Behav. Res. Methods 45, 576–594. doi: 10.3758/s13428-012-0261-6
Van den Noortgate, W., López-López, J. A., Marin-Martinez, F., and Sánchez-Meca, J. (2015). Meta-analysis of multiple outcomes: A multilevel approach. Behav. Res. Methods 47, 1274–1294. doi: 10.3758/s13428-014-0527-2
Van der Kleij, F. M., Feskens, R. C. W., and Eggen, T. J. H. M. (2015). Effects of feedback in a computer-based learning environment on students' learning outcomes: A meta-analysis. Rev. Educ. Res. 85, 475–511. doi: 10.3102/0034654314564881
Viechtbauer, W. (2005). Bias and efficiency of meta-analytic variance estimators in the random-effects model. J. Educ. Behav. Stat. 30, 261–293. doi: 10.3102/10769986030003261
Viechtbauer, W. (2010). Conducting meta-analyses in R with the metafor package. J. Stat. Softw. 36, 1–48. doi: 10.18637/jss.v036.i03
*Wade-Stein, D., and Kintsch, E. (2004). Summary street: Interactive computer support for writing. Cogn. Instruct. 22, 333–362. doi: 10.1207/s1532690xci2203_3
*Wang, Y. J., Shang, H. F., and Briody, P. (2013). Exploring the impact of using automated writing evaluation in english as a foreign language university students' writing. Comput. Assist. Lang. Learn. 26, 234–257. doi: 10.1080/09588221.2012.655300
Williams, C., and Beam, S. (2019). Technology and writing: Review of research. Comput. Educ. 128, 227–242. doi: 10.1016/j.compedu.2018.09.024
*Wilson, D. B. (2016). Formulas Used by the Practical Meta-analysis Effect Size Calculator. Practical Meta-Analysis. Unpublished manuscript: George Mason University. Available online at: https://mason.gmu.edu/~dwilsonb/downloads/esformulas.pdf
Wilson, J., and Czik, A. (2016). Automated essay evaluation software in english language arts classrooms: Effects on teacher feedback, student motivation, and writing quality. Comput. Educ. 100, 94–109. doi: 10.1016/j.compedu.2016.05.004
*Wilson, J., and Roscoe, R. D. (2020). Automated writing evaluation and feedback: Multiple metrics of efficacy. J. Educ. Comput. Res. 58, 87–125. doi: 10.1177/0735633119830764
Wisniewski, B., Zierer, K., and Hattie, J. (2020). The power of feedback revisited: A meta-analysis of educational feedback research. Front. Psychol. 10, 3087. doi: 10.3389/fpsyg.2019.03087
*Zaini, A., and Mazdayasna, G. (2015). The impact of computer-based instruction on the development of EFL learners' writing skills. J. Comput. Assist. Learn. 31, 516–528. doi: 10.1111/jcal.12100
Zawacki-Richter, O., Marín, V. I., Bond, M., and Gouverneur, F. (2019). Systematic review of research on artificial intelligence applications in higher education—where are the educators? Int. J. Educ. Technol. High. Educ. 16, 171. doi: 10.1186/s41239-019-0171-0
Zhai, N., and Ma, X. (2021). Automated writing evaluation (AWE) feedback: A systematic investigation of college students' acceptance. Comput. Assist. Lang. Learn. 2021, 1–26. doi: 10.1080/09588221.2021.1897019
Zhai, N., and Ma, X. (2022). The effectiveness of automated writing evaluation on writing quality: A meta-analysis. J. Educ. Comput. Res. 2022, 7356331221127300. doi: 10.1177/07356331221127300
Zhang, S. (2021). Review of automated writing evaluation systems. J. China Comput. Assist. Lang. Learn. 1, 170–176. doi: 10.1515/jccall-2021-2007
Zhu, M., Liu, O. L., and Lee, H. S. (2020). The effect of automated feedback on revision behavior and learning gains in formative assessment of scientific argument writing. Comput. Educ. 143, 103668. doi: 10.1016/j.compedu.2019.103668
Zhu, M., and Urhahne, D. (2015). Teachers' judgements of students' foreign-language achievement. Eur. J. Psychol. Educ. 30, 21–39. doi: 10.1007/s10212-014-0225-6
* ^References marked with an asterisk indicate studies included in the meta-analysis.
Keywords: technology-based learning, automated writing evaluation, writing instruction, feedback, formative assessment, meta-analysis
Citation: Fleckenstein J, Liebenow LW and Meyer J (2023) Automated feedback and writing: a multi-level meta-analysis of effects on students' performance. Front. Artif. Intell. 6:1162454. doi: 10.3389/frai.2023.1162454
Received: 20 February 2023; Accepted: 30 May 2023;
Published: 03 July 2023.
Edited by:
Christos Troussas, University of West Attica, GreeceReviewed by:
Zoe Kanetaki, University of West Attica, GreeceCopyright © 2023 Fleckenstein, Liebenow and Meyer. This is an open-access article distributed under the terms of the Creative Commons Attribution License (CC BY). The use, distribution or reproduction in other forums is permitted, provided the original author(s) and the copyright owner(s) are credited and that the original publication in this journal is cited, in accordance with accepted academic practice. No use, distribution or reproduction is permitted which does not comply with these terms.
*Correspondence: Johanna Fleckenstein, ZmxlY2tlbnN0ZWluQHVuaS1oaWxkZXNoZWltLmRl
†These authors have contributed equally to this work and share first authorship
Disclaimer: All claims expressed in this article are solely those of the authors and do not necessarily represent those of their affiliated organizations, or those of the publisher, the editors and the reviewers. Any product that may be evaluated in this article or claim that may be made by its manufacturer is not guaranteed or endorsed by the publisher.
Research integrity at Frontiers
Learn more about the work of our research integrity team to safeguard the quality of each article we publish.