- 1School of Computational and Communication Science and Engineering, Nelson Mandela African Institution of Science and Technology (NM-AIST), Arusha, Tanzania
- 2Department of Computer Science and Engineering at the College of Informatics and Virtual Education, The University of Dodoma, Dodoma, Tanzania
In recent years, AI technologies have become indispensable in social and industrial development, yielding revolutionary results in improving labor efficiency, lowering labor costs, optimizing human resource structure, and creating new job demands. To reap the full benefits of responsible AI solutions in Africa, it is critical to investigate existing challenges and propose strategies, policies, and frameworks for overcoming and eliminating them. As a result, this study investigated the challenges of adopting responsible AI solutions in the Academia-Private sectors for Anglophone Africa through literature reviews, expert interviews, and then proposes solutions and framework for the sustainable and successful adoption of responsible AI.
Many African countries are envisioning to achieve the United Nations Sustainable Development Goals (SDGs) target and also become middle-income countries with a semi-industrialized economy by 2030 (Johnston, 2016; Tjoa and Tjoa, 2016; Pedersen, 2018; Vinuesa et al., 2020). Realizing this industrialization target requires basic and advanced skills in emerging technologies, specifically the exploitation of Fourth Industrial Revolution (4IR's) technologies, particularly Artificial Intelligence (AI) and Machine Learning (ML) (Cioffi et al., 2020; Hamdan et al., 2020; Kshetri, 2020; Felice et al., 2022; Noman et al., 2022). Notably, AI has evolved in many phases since its inception to an indispensable role in solving many societal and industrial challenges. Hence, it can significantly impact the Global South by transforming public services delivery in strategic developmental sectors like healthcare, infrastructure, data ecosystem, digital economy, environmental conservation, and agriculture (Benke and Benke, 2018; Heymann et al., 2018; Srivastava, 2018; Nensa et al., 2019; Ifenthaler, 2020; Lopez-Jimenez et al., 2020; Kipkorir-Songol et al., 2021; Kaack et al., 2022). Additionally, AI can provide a means for small and medium-scale industries and businesses to thrive and improve the country's GDP growth rate (Kushwaha and Kar, 2020; Hansen and Bøgh, 2021; Sharma et al., 2022). For instance, in the years 2017, 2018, and 2019, the AI for Good Global Summit organized by ITU, explored different AI solutions that can yield long-term benefits and help achieve the SDGs (Artificial Intelligence ITU for Global Good, 2018; Cioffi et al., 2020; Floridi et al., 2020; Walsh et al., 2020; Holzmeyer, 2021). Such solutions include AI-based prediction of disease outbreaks, AI-enabled drones for power lines inspection, and using AI to help farmers improve crop production with less water, to mention a few (Chen et al., 2019; Jha et al., 2019; Talaviya et al., 2020; Zhang et al., 2020; Abdullahi et al., 2021; Kolozsvari et al., 2021; Mehta et al., 2021; Rovira-Sugranes et al., 2022). Despite AI's enormous promises and opportunities for sustainable development in many other sectors, Anglophone African countries are still not fully prepared to adopt its full benefits (Vinuesa et al., 2020; Wareham, 2021). Lack of preparedness to harness the benefit of responsible AI in the Academia-Private sector has led to failures and challenges to most of the implemented AI-based solutions and interventions that would have solved most of Anglophone Africa's notorious social and economic challenges (Magrabi et al., 2019; Raaijmakers, 2019; Al Mutawa and Rashid, 2020; Briceño, 2020; Chassagnon and Dohan, 2020; Radakovich et al., 2020; Abioye et al., 2021).
Therefore, for the Academia-Private sectors in Anglophone Africa to adopt the full benefits of responsible AI solutions, it is necessary to investigate the existing challenges. Thereafter, establish strategies and mechanisms for overcoming these challenges and lay a foundation for the Anglophone African countries' current and future workforce in AI. Consequently, this study used data collection approaches including online survey and document review. In the review, the study obtained articles published between 2017 and 2022 from the google scholar, science direct, IEEE Xplore and other sources. The search used the following keywords: responsible AI, machine learning (ML), and challenges of AI solutions, and inclusion criteria based on their titles, abstract, and full-text as illustrated in Figure 1. Whereas through the use of PRISMA (Page et al., 2021) approach, a set of queries in the identification stage, the authors identified a total of 646 publications from the database search, then, 231 publications were removed as duplicates, and the remaining 415 were filtered to remove the 307 irrelevant publications based on their titles. Then, the 108 publications were screened to remove 77 publications based on their abstracts. Lastly, the remaining 31 publications were screened based on their full text, and as a result, 21 publications were removed. In the end, only 10 publications were eligible to be included in the review with equal consensus from all authors. Furthermore, forward snowballing process was applied to the 10 eligible articles which were included in the review. Hence, as a result, 4 new eligible articles were added in the review, making a total of reviewed articles to be 14.
Furthermore, the expert interview and discussion comprised 44 respondents from Anglophone African countries. The response indicated that thirty-three (75%) were male and eleven (25%) were female, as shown in Figure 2A; four (9.1%) respondents were between 18 to 24 years old, fifteen (34.1%) were between 25 to 34 years old, eighteen (40.9%) were between 35 to 44 years old, and seven (15.9%) were between 45 to 54 years old, as shown in Figure 2C. Whereas, the role of respondents in the AI sector in their current country includes eight (8) academicians, thirteen (13) data scientists, five (5) ICT policy makers, three (3) ICT consultants, five (5) innovators, and ten (10) students. Moreover, the data indicate that the respondents have been in the AI sector as follows, about 82.5% have been in the sector for less than 5 years, about 10% have been in the sector for 5 to 10 years, about 5% have been in the sector for 10 to 15 years, and about 2.5% have been in the sector for more than 15 years as shown in Figure 2B. The study review and expert interview laid the groundwork for proposing the adoption strategy of responsible AI in the Academia-Private sectors for Anglophone Africa.
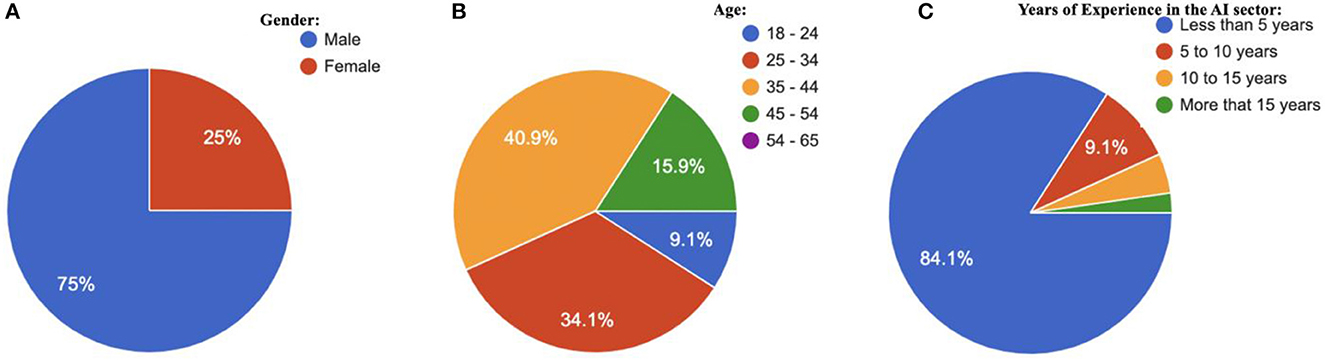
Figure 2. Description of the participants in terms of (A) Gender, (B) Age, and (C) the years of experience in the AI sector.
The general identified and reported challenges (C1-C11) include the following (Schoeman et al., 2017; Alami et al., 2020; Gwagwa et al., 2020a,b, 2021; How et al., 2020; Valle-Cruz et al., 2020; Arakpogun et al., 2021; Sobrino-García, 2021; Amankwah-Amoah and Lu, 2022; López et al., 2022; Zagabathuni and Zagabathuni, 2022; Eke, 2023)1:
C1 Inadequate AI infrastructure for the effective development and long-term implementation of responsible solutions.
C2 There is a scarcity of African-origin datasets that are relevant, reliable, and of high quality to support research, development, and innovation.
C3 The majority of existing datasets are still paper-based, and most organizations are unwilling to digitize them.
C4 Data is underutilized in decision-making and policy-making, even when the data is available.
C5 Inadequate data management skills and knowledge.
C6 There are no policies, guidelines, regulations, or strategies in place to facilitate the long-term adoption of AI-based solutions.
C7 Lack of AI expertise, limited capabilities, and skills to develop appropriate solutions.
C8 There are no clear specifications or approaches for implementing AI solutions.
C9 Inconsistent funding for AI-related projects.
C10 Misconceptions and Lack of Awareness and knowledge about AI Technology.
C11 There are no clear roadmaps for AI adoption.
Despite the fact that the study was limited to investigating challenges that the Academia-Private sector faces in innovating responsible AI in Anglophone African countries, the recommended adoption strategies and solutions can be applied in other African countries. In general, the major contributions of this study are summarized as follows:
• Proposed strategies, policies and frameworks to overcome and eliminate challenges for responsible AI adoption in Academia-Private sector in Anglophone Africa.
• Brought together each tech spaces, technology hubs and centers in the AI ecosystem.
• Pointed out the cutting-edge insights and research outputs of developing a responsible AI in Anglophone Africa.
• Explored the Academis-Private sector of Responsible AI that drives the economic development of the Anglophone Africa.
• Highlighted the motivation for further development of responsible AI to stimulate Academia-Private sector in Anglophone Africa.
The remainder of this study includes a discussion of the proposed adoption strategies and framework for responsible AI adoption in the Academia-Private sector, as well as a call to action to all AI stakeholders in Anglophone African countries and Africa as a whole.
Solutions for responsible AI adoption in Anglophone Africa for the academia-private sector
Table 1 categorizes the solutions to the identified challenges for responsible AI adoption into six groups (A1–A6). Each group contains at least one solution (S) that could be used to help the Academia-Private sector in Anglophone Africa adopt responsible AI more smoothly. These solutions will hasten the successful adoption of AI-powered solutions and their application in the Anglophone African Academia-Private sector.
A framework for adoption of responsible AI in Anglophone Africa
The study proposes a framework for implementing responsible AI in Anglophone Africa, as shown in Figure 3. The framework is built around three guiding pillars: capacity development and raising awareness; research development and project management; and responsible innovation and sustainable entrepreneurship. All of the adoption strategies and solutions presented in Table 1 are supported by the framework's three pillars. The capacity development and awareness creation pillar focuses on using formal education and on-the-job experience to prepare the Academia-Private sector in Anglophone Africa for responsible AI adoption. The pillar for research development and project management will be realized by supporting responsible AI research, project, and development by local and regional governments in Anglophone Africa. The pillar for responsible innovation and sustainable entrepreneurship is in charge of creating AI-related innovation ecosystems in Anglophone Africa by allocating budget and funding for AI investments. The use of this framework by digital policymakers, technologists, and leaders will strengthen their capacities to face the challenges (technical, legal, social, and ethical) of responsible AI adoption and facilitate the habit of applying appropriate solutions to a specific challenge. Furthermore, this framework will serve as a tool for increasing diversity in AI-related activities and enhancing the adoption and implementation of responsible AI policies by local and regional governments across Anglophone African countries.
Case studies of AI adoption in Anglophone Africa
There are several case studies regarding the adoption of AI in Anglophone Africa in different domains, including healthcare, education, agriculture, digital economy, infrastructure, and data ecosystem. Some of the case studies include M-SHULE4 for education, Computer-Aided Detection for Tuberculosis (CAD4TB) (Murphy et al., 2020) for healthcare, AGROBOT for agriculture,5 Kudi6 for the digital economy, Artificial Intelligence for Digital Response (AIDR),7 and Dr. Elsa Health Assistant technology for healthcare (Development and Challenges, 2019; Intelligence et al., 2020; Proposal and Elsa, 2020).8 This study, on the other hand, used Dr. Elsa Health Assistant technology for healthcare as an example to identify some of the challenges to AI adoption in Tanzania.
The Dr. Elsa Health Assistant system employs artificial intelligence (AI) approaches to help medical professionals in rural areas by providing symptom assessment, diagnostic decision support, next step recommendations, and disease outbreak prediction. The Tool is provided to healthcare professionals through a mobile application on a phone or tablet and performs like an experienced pediatrician on a tablet. The smartphone app generates a differential diagnosis and evidence-based recommendations for the patient's next step when the demographics, vital signs, symptoms, and test results of the patient are entered. It is intended to help low-cadre healthcare providers make decisions about their patients through efficient symptom evaluation, strengthening their competence to improve patient outcomes.
An interview with the founder of Dr. Elsa was conducted to identify some of the challenges to AI adoption in Tanzania, and it was revealed that there are some challenges that hinder the Tool's adoption in the country. Some of these challenges include lack of supportive healthcare infrastructure, unavailability of locally located servers and poor network availability; technology literacy and small talent pool; lack of regulatory frameworks; and limited access to high-performance computing. These challenges are briefly discussed as follows:
Lack of supportive health infrastructure, locally located servers and poor network availability: The founder pointed out that, most health facilities, especially public health facilities, do not have digital systems/hardware that we can leverage for AI technology. Either they do not have any technology at all and are relying on paper-based systems, or they have an electronic health records system which utilizes the Government of Tanzania Health Operations Management Information System (GOT-HOMIS) (Thadeus Kissima and Mushi, 2021) or AfyaCare9 government systems. These are very difficult to integrate with Dr. Elsa's zool. In addition, network availability is also a problem. That is, the network is unpredictable, especially in remote areas of Tanzania. Furthermore, health data is sensitive and is encouraged to be stored within Tanzanian territory. However, server storage and cloud computing for locally located servers are expensive. An offering from an international cloud provider for $15 per month is more than TZS 800,000 for a local alternative. Also, many hosting providers in Tanzania just resell server space from international providers. Notably, these challenges can be addressed by establishing an open AI infrastructure, as depicted by A2 (S8) in Table 1 and Figure 3.
Technology Literacy and small talent pool: The founder also revealed that, technology literacy is still low in rural Tanzania, and there are still surprising perceptions around AI technology. The founder also reported several misconceptions about AI solutions. Some users were concerned if the founder is linked to freemasons because of entering their data into the system. The founder quotes some positive feedback, “I permit my data to be uploaded into your system since one of my colleagues was cured after using the system”. Additionally, the founder said that “there are many programmers in Tanzania, not as many as we would like, but still enough that we can find programmers when needed”. The biggest problem is finding “engineers who not only know programming language but solve given problems effectively and then come back to optimize for efficiency”. Moreover, “the AI talent pool is very small, and the little that does exist is focused on data-centric machine learning and has little-to-no exposure to other forms of AI”. Notably, these challenges can be tackled through leadership and management, as depicted in A1 (S1 to S7) in Table 1 and Figure 3.
Regulatory Framework: According to the founder, there are a lot of uncertainties because there are vague regulations governing “data” and “data transfer,” and there are no regulations governing algorithmic decision-making. The whole world is still trying to solve these problems, but it just means we are not sure about what the new laws will mean. This challenge can be solved using A5 (S11), as shown in Table 1 and Figure 3.
Call for action
AI stakeholders are encouraged to develop and deploy solutions equitably and ethically to facilitate the smooth adoption of responsible AI in Anglophone Africa for the Academia-Private sector. As a result, the Academia-Private sector must be proactive in harnessing responsible AI solutions for economic development and investment and establish an effective and sustainable roadmap for innovating responsible AI solutions in Anglophone Africa. In addition, the Academia-Private sector should increase the capacity building of human resources and the enabling infrastructure for AI adoption while recalibrating its laws and regulations regarding AI-friendly policies. Furthermore, the Academia-Private sector should set clear and guiding factors that determine successful, responsible AI innovations, including communication channels, perceived attributes of innovation, type of innovation-decision, nature of the social system, and efforts of change agents. Failure to make these enhancements and tap into the proposed framework and recommended solutions would result in the forced adoption of obsolete and rudimentary AI solutions that would not be adequate for Africa's developmental goals and the needs of its populations.
Limitations and future works
The challenges and solutions of adopting responsible AI in the Academia-Private sectors for Anglophone Africa presented in this study are limited to the opinions of AI experts and researchers; thus, the general community's opinions are not included in the findings and will remain a piece of future work. Furthermore, the proposed framework for the sustainable and successful adoption of responsible AI is a theoretical solution and also, there is a lack of investigation for equity and inclusivity in the study. Therefore, the study recommends future works to conduct an empirical evaluation of the framework and also investigate equity and inclusion in AI across Anglophone Africa. Despite these limitations and recommendations, the achieved outcomes remain significant to the study area and the collected data.
Data availability statement
The raw data supporting the conclusions of this article will be made available by the authors, without undue reservation.
Author contributions
RS, SD, JM, JL, NE, and TK ideated, designed, and coordinated inputs from literature review and other experts in the area to unlock adoption of AI for responsible solutions in Academia-Private sector for Anglophone Africa. JM, RS, and JL wrote introduction, methodology, and challenges for adopting AI in Academia-Private sector. SD, TK, and NE wrote the proposed solutions for adopting AI and explained on how they can be used in one case study for successful adoption of AI in Academia-Private sector for Anglophone Africa. All authors reviewed the paper as final editor.
Funding
This work was carried out with the aid of a grant from Artificial Intelligence for Development in Africa Program, a program funded by Canada's the International Development Research Centre, Ottawa, Canada, and the Swedish International Development Cooperation Agency.
Conflict of interest
The authors declare that the research was conducted in the absence of any commercial or financial relationships that could be construed as a potential conflict of interest.
Publisher's note
All claims expressed in this article are solely those of the authors and do not necessarily represent those of their affiliated organizations, or those of the publisher, the editors and the reviewers. Any product that may be evaluated in this article, or claim that may be made by its manufacturer, is not guaranteed or endorsed by the publisher.
Footnotes
1. ^WORLD VIEW Look to Africa to advance artificial intelligence.
2. Ethics and Governance of Artificial Intelligence for Health | OpenWHO. Available online at: https://openwho.org/courses/ethics-ai (accessed August 7, 2022).
3. Center Africa, S. T. E. M. Available online at: https://www.stemcenter-africa.com/ (accessed August 7, 2022).
4. ^M-Shule - Together We Learn, Together We Succeed. Available online at: https://m-shule.com/ (accessed August 7, 2022).
5. ^Agrobot: Agricultural Robots. Available online at: https://www.agrobot.com/ (accessed August 7, 2022).
6. ^Nomba. Available online at: https://nomba.com/ (accessed August 7, 2022).
7. ^AIDR: Artificial Intelligence for Disaster Response. Available online at: http://aidr.qcri.org/ (accessed August 7, 2022.).
8. ^Elsa Health | AI for clinical decision support. Available online at: https://www.elsa.health/ (accessed August 7, 2022).
9. ^AFYACARE. Available online at: http://www.afyacare.co.id/ (accessed August 7, 2022).
References
Abdullahi, S. B., Muangchoo, K., Abubakar, A. B., Ibrahim, A. H., and Aremu, K. O. (2021). Data-driven AI-based parameters tuning using grid partition algorithm for predicting climatic effect on epidemic diseases. IEEE Access 9, 55388–55412. doi: 10.1109/ACCESS.2021.3068215
Abe, E. N., and Chikoko, V. (2020). Exploring the factors that influence the career decision of STEM students at a university in South Africa. Int. J. STEM Educ. 7, 1–4. doi: 10.1186/s40594-020-00256-x
Abioye, S. O., Oyedele, L. O., Akanbi, L., Ajayi, A., Delgado, J. M. D., Bilal, M., et al. (2021). Artificial intelligence in the construction industry: A review of present status, opportunities and future challenges. J. Build. Eng. 44, 103299. doi: 10.1016/j.jobe.2021.103299
Al Mutawa, M., and Rashid, H. (2020). “Comprehensive review on the challenges that impact artificial intelligence applications in the public sector,” in Proceedings of the 5th, NA International Conference on Industrial Engineering, and Operations Management 2078–2087
Alami, H., Rivard, L., Lehoux, P., Hoffman, S. J., Cadeddu, S. B. M., Savoldelli, M., et al. (2020). Artificial intelligence in health care: laying the Foundation for Responsible, sustainable, inclusive innovation in low- and middle-income countries. Glob. Heal. 16, 1–6. doi: 10.1186/s12992-020-00584-1
Amankwah-Amoah, J., and Lu, Y. (2022). Harnessing AI for business development: a review of drivers and challenges in Africa. Prod. Plan. Control 2022, 1–10. doi: 10.1080/09537287.2022.2069049
Arakpogun, E. O., Elsahn, Z., Olan, F., and Elsahn, F. (2021). Artificial Intelligence in Africa: Challenges and Opportunities. Stud. Comput. Intell. 935, 375–388. doi: 10.1007/978-3-030-62796-6_22
Benke, K., and Benke, G. (2018). Artificial intelligence and big data in public health. Int. J. Environ. Res. Public Health 15, 2796. doi: 10.3390/ijerph15122796
Briceño, J. (2020). Artificial intelligence and organ transplantation: challenges and expectations. Curr. Opin. Organ Transpl. 25, 393–398. doi: 10.1097/MOT.0000000000000775
Chassagnon, G., and Dohan, A. (2020). Artificial intelligence: from challenges to clinical implementation. Diagn. Interv. Imaging 101, 763–764. doi: 10.1016/j.diii.2020.10.007
Chen, H., Yang, B., Pei, H., and Liu, J. (2019). Next generation technology for epidemic prevention and control: data-driven contact tracking. IEEE Access 7, 2633–2642. doi: 10.1109/ACCESS.2018.2882915
Cioffi, R., Travaglioni, M., Piscitelli, G., Petrillo, A., and De Felice, F. (2020). Artificial intelligence and machine learning applications in smart production: Progress, trends, directions. Sustainability 12, 1–24. doi: 10.3390/su12020492
Development, C., and Challenges, H. (2019). Building Dr. Elsa : A Digital Health Assistant for East Africa.
Felice, F. D., Petrillo, A., Luca, C. D., and Baffo, I. (2022). Artificial Intelligence or Augmented Intelligence? Impact on our lives, rights and ethics. Procedia Comput. Sci. 200, 1846–1856. doi: 10.1016/j.procs.2022.01.385
Floridi, L., Cowls, J., King, T. C., and Taddeo, M. (2020). How to Design AI for social good: seven essential factors. Sci. Eng. Ethics 26, 1771–1796. doi: 10.1007/s11948-020-00213-5
Gwagwa, A., Kachidza, P., and Siminyu, K. (2020b). “Responsible artificial intelligence in Sub-Saharan Africa: landscape and general state of play,” AI4D Africa (Artificial Intell. Dev. Africa) 24.
Gwagwa, A., Kazim, E., Kachidza, P., Hilliard, A., Siminyu, K., Smith, M., et al. (2021). Road map for research on responsible artificial intelligence for development (AI4D) in African countries: The case study of agriculture. Patterns 2, 100381. doi: 10.1016/j.patter.2021.100381
Gwagwa, A., Kraemer-Mbula, E., Rizk, N., Rutenberg, I., and De Beer, J. (2020a). Artificial Intelligence (AI) Deployments in Africa: Benefits, Challenges and Policy Dimensions. African J. Inf. Commun. 26, 1–28. doi: 10.23962/10539/30361
Hamdan, A., Hassanien, A. E., Razzaque, A., and Alareeni, B. (2020). The Fourth Industrial Revolution: Implementation of Artificial Intelligence. Cham: Springer Nature. doi: 10.1007/978-3-030-62796-6
Hansen, E. B., and Bøgh, S. (2021). Artificial intelligence and internet of things in small and medium-sized enterprises: A survey. J. Manuf. Syst. 58, 362–372. doi: 10.1016/j.jmsy.2020.08.009
Heumann, S. (2018). Benchmarking national AI strategies: Why and how indicators and monitoring can support agile implementation. Available at SSRN 3502283. doi: 10.2139/ssrn.3502283
Heymann, E., Heymann, E., Koerner, K., Körner, K., Schattenberg, M., Schattenberg, M., et al. (2018). Digital economics.
Holzmeyer, C. (2021). Beyond ‘AI for Social Good' (AI4SG): social transformations—not tech-fixes—for health equity. Interdisc. Sci. Rev. 46, 94–125. doi: 10.1080/03080188.2020.1840221
How, M. L., Cheah, S. M., Chan, Y. J., Khor, A. C., and Say, E. M. P. (2020). Artificial intelligence-enhanced decision support for informing global sustainable development: a human-centric AI-thinking approach. Information 11, 39. (2020). doi: 10.3390/info11010039
Intelligence, A., Learning, M., and Assistant, E. H. (2020). Integrated, Intelligent Healthcare to Improve Pediatric Health Outcomes in Tanzania.
Jha, K., Doshi, A., Patel, P., and Shah, M. (2019). A comprehensive review on automation in agriculture using artificial intelligence. Artif. Intell. Agric. 2, 1–12. doi: 10.1016/j.aiia.2019.05.004
Johnston, R. B. (2016). “Arsenic and the 2030. Agenda for sustainable development,” in Arsenic Research and Global Sustainability-Proceedings of the 6th International Congress on Arsenic in the Environment, AS 12–14. doi: 10.1201/b20466-7
Kaack, L. H., Donti, P. L., Strubell, E., Kamiya, G., Creutzig, F., Rolnick, D., et al. (2022). Aligning artificial intelligence with climate change mitigation. Nat. Clim. Chang. 12, 518–527. doi: 10.1038/s41558-022-01377-7
Kiemde, S. M. A., and Kora, A. D. (2022). Towards an ethics of AI in Africa: rule of education. AI Ethics 2, 35–40. doi: 10.1007/s43681-021-00106-8
Kipkorir-Songol, M., Mzee-Awuor, F., and Maake, B. (2021). Adoption of artificial intelligence in agriculture in the developing nations: a review. J. Lang. Technol. Entrep. Africa 12, 1–21.
Kolozsvari, L. R., Bérczes, T., Hajdu, A., Gesztelyi, R., Tiba, A., Varga, I., et al. (2021). Predicting the Epidemic Curve of the Coronavirus (SARS-CoV-2) Disease (COVID-19) Using Artificial Intelligence. medRxiv 04, 17.20069666. doi: 10.1101/2020.04.17.20069666
Kshetri, N. (2020). Artificial intelligence in developing countries. IT Professional 22, 63–68. doi: 10.1109/MITP.2019.2951851
Kushwaha, A. K., and Kar, A. K. (2020). Micro-foundations of Artificial Intelligence Adoption in Business: Making the Shift. IFIP Adv. Inf. Commun. Technol. 617, 249–260. doi: 10.1007/978-3-030-64849-7_22
López, D. M., Rico-Olarte, C., Blobel, B., and Hullin, C. (2022). Challenges and solutions for transforming health ecosystems in low- and middle-income countries through artificial intelligence. Front. Med. 9, 1–22. doi: 10.3389/fmed.2022.958097
Lopez-Jimenez, F., Attia, Z., Arruda-Olson, A. M., Carter, R., Chareonthaitawee, P., Jouni, H., et al. (2020). “Artificial Intelligence in Cardiology: Present and Future,” in Mayo Clinic Proceedings 95, 1015–1039. doi: 10.1016/j.mayocp.2020.01.038
Magrabi, F., Ammenwerth, E., McNair, J. B., De Keizer, N. F., Hyppönen, H., Nykänen, P., et al. (2019). Artificial intelligence in clinical decision support: challenges for evaluating ai and practical implications. Yearb. Med. Inform. 28, 128–134. doi: 10.1055/s-0039-1677903
Martinez-Plumed, F., and Hernandez-Orallo, J. (2020). Dual Indicators to Analyze AI Benchmarks: Difficulty, Discrimination, Ability, Generality. IEEE Trans. Games 12, 121–131. doi: 10.1109/TG.2018.2883773
Mehta, P. L., Kalra, R., and Prasad, R. (2021). A Backdrop Case Study of AI-Drones in Indian demographic characteristics emphasizing the role of ai in global cities digitalization. Wirel. Pers. Commun. 118, 301–321. doi: 10.1007/s11277-020-08014-6
Murphy, K., Habib, S. S., Zaidi, S. M. A., Khowaja, S., Khan, A., Melendez, J., et al. (2020). Computer aided detection of tuberculosis on chest radiographs: An evaluation of the CAD4TB v6 system. Sci. Rep. 10, 1–11. doi: 10.1038/s41598-020-62148-y
Nayebare, M. (2019). Artificial intelligence policies in Africa over the next five years. XRDS Crossroads, ACM Mag. Students 26, 50–54. doi: 10.1145/3368075
Nensa, F., Demircioglu, A., and Rischpler, C. (2019). Artificial intelligence in nuclear medicine. J. Nucl. Med. 60, 29S−37S. doi: 10.2967/jnumed.118.220590
Noman, A. A., Akter, U. H., Pranto, T. H., and Haque, A. K. M. B. (2022). Machine learning and artificial intelligence in circular economy: a bibliometric analysis and systematic literature review. Ann. Emerg. Technol. Comput. 6, 13–40. doi: 10.33166/AETiC.2022.02.002
Page, M. J., McKenzie, J. E., Bossuyt, P. M., Boutron, I., Hoffmann, T. C., Mulrow, C. D., et al. (2021). The PRISMA 2020 statement: an updated guideline for reporting systematic reviews. Syst. Rev. 10, 1–11. doi: 10.1186/s13643-021-01626-4
Pedersen, C. S. (2018). The UN Sustainable Development Goals (SDGs) are a Great Gift to Business. Procedia CIRP 69, 21–24. doi: 10.1016/j.procir.2018.01.003
Proposal, S. F., and Elsa, P. (2020). Integrated, Intelligent Tools to Support the HIV/AIDS Initiatives and Goals in Tanzania About Dr. Elsa Key Milestones to Date, with Support from dLab HIV/AIDS Scale Up Plan.
Raaijmakers, S. (2019). Artificial intelligence for law enforcement: challenges and opportunities. IEEE Secur. Priv. 17, 74–77. doi: 10.1109/MSEC.2019.2925649
Radakovich, N., Nagy, M., and Nazha, A. (2020). Artificial intelligence in hematology: current challenges and opportunities. Curr. Hematol. Malig. Rep. 15, 203–210. doi: 10.1007/s11899-020-00575-4
Rovira-Sugranes, A., Razi, A., Afghah, F., and Chakareski, J. (2022). A review of AI-enabled routing protocols for UAV networks: Trends, challenges, future outlook. Ad Hoc. Netw. 130, 102790. doi: 10.1016/j.adhoc.2022.102790
Schoeman, W., Moore, R., Seedat, Y., and Artificial Intelligence, C. Y. J. (2017). “Is South Africa Ready?,” in Accenture 21 doi: 10.4337/9781782547235.NS.6
Sharma, P., Shah, J., and Patel, R. (2022). Artificial intelligence framework for MSME sectors with focus on design and manufacturing industries. Mater. Today Proc. 62, 6962–6966. doi: 10.1016/j.matpr.2021.12.360
Sobrino-García, I. (2021). Artificial intelligence risks and challenges in the Spanish public administration: An exploratory analysis through expert judgements. Adm. Sci. 11, 102. doi: 10.3390/admsci11030102
Srivastava, S. K. (2018). Artificial Intelligence: way forward for India. J. Inf. Syst. Technol. Manag. 15, 1–23. doi: 10.4301/S1807-1775201815004
Talaviya, T., Shah, D., Patel, N., Yagnik, H., and Shah, M. (2020). Implementation of artificial intelligence in agriculture for optimisation of irrigation and application of pesticides and herbicides. Artif. Intell. Agric. 4, 58–73. doi: 10.1016/j.aiia.2020.04.002
Thadeus Kissima, W., and Mushi, L. D. (2021). Health care professional knowledge and attitude towards the use of digital technologies in provision of maternal health services at Tumbi regional referral hospital in Tanzania. J. Med. Res. Innov. 5, e000233–e000233. doi: 10.32892/jmri.233
Tjoa, A. M., and Tjoa, S. (2016). The role of ICT to achieve the UN sustainable development goals (SDG). IFIP Adv. Inf. Commun. Technol. 481, 3–13. doi: 10.1007/978-3-319-44447-5_1
Valle-Cruz, D., Criado, J. I., Sandoval-Almazán, R., and Ruvalcaba-Gomez, E. A. (2020). Assessing the public policy-cycle framework in the age of artificial intelligence: From agenda-setting to policy evaluation. Gov. Inf. Q. 37, 101509. doi: 10.1016/j.giq.2020.101509
Vinuesa, R., Azizpour, H., Leite, I., Balaam, M., Dignum, V., Domisch, S., et al. (2020). The role of artificial intelligence in achieving the Sustainable Development Goals. Nat. Commun. 11, 233. doi: 10.1038/s41467-019-14108-y
Wareham, C. S. (2021). Artificial intelligence and African conceptions of personhood. Ethics Inf. Technol. 23, 127–136. doi: 10.1007/s10676-020-09541-3
Zagabathuni, Y., and Zagabathuni, Y. (2022). Applications, Scope, Challenges for AI in healthcare. Int. J. Emerg. Trends Eng. Res. 10, 195–199. doi: 10.30534/ijeter/2022/041042022
Keywords: Anglophone Africa education, academia-private sector, AI stakeholders, responsible solution, artificial intelligence
Citation: Sinde R, Diwani S, Leo J, Kondo T, Elisa N and Matogoro J (2023) AI for Anglophone Africa: Unlocking its adoption for responsible solutions in academia-private sector. Front. Artif. Intell. 6:1133677. doi: 10.3389/frai.2023.1133677
Received: 29 December 2022; Accepted: 17 March 2023;
Published: 11 April 2023.
Edited by:
Kiran Sree Pokkuluri, Shri Vishnu Engineering College for Women, IndiaReviewed by:
Sadiq Hussain, Dibrugarh University, IndiaCopyright © 2023 Sinde, Diwani, Leo, Kondo, Elisa and Matogoro. This is an open-access article distributed under the terms of the Creative Commons Attribution License (CC BY). The use, distribution or reproduction in other forums is permitted, provided the original author(s) and the copyright owner(s) are credited and that the original publication in this journal is cited, in accordance with accepted academic practice. No use, distribution or reproduction is permitted which does not comply with these terms.
*Correspondence: Ramadhani Sinde, cmFtYWRoYW5pLnNpbmRlQG5tLWFpc3QuYWMudHo=