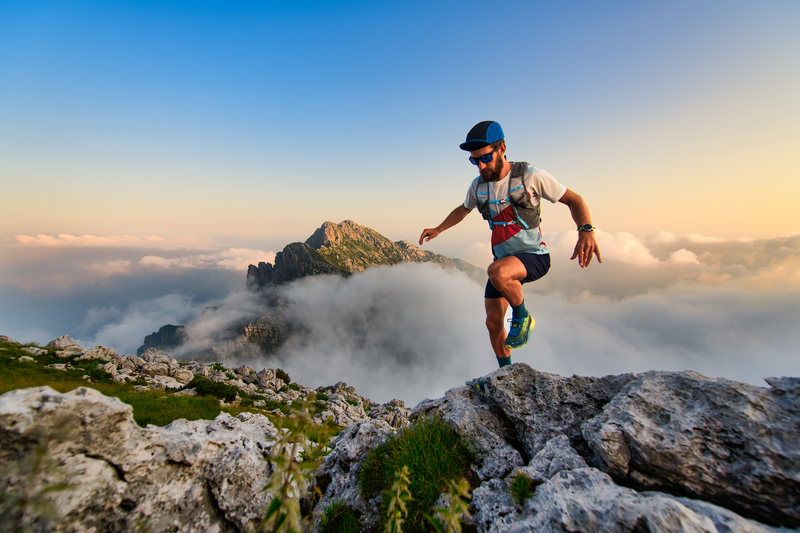
94% of researchers rate our articles as excellent or good
Learn more about the work of our research integrity team to safeguard the quality of each article we publish.
Find out more
ORIGINAL RESEARCH article
Front. Artif. Intell. , 30 September 2022
Sec. Medicine and Public Health
Volume 5 - 2022 | https://doi.org/10.3389/frai.2022.910216
This article is part of the Research Topic Biomedical Informatics Applications in Rare Diseases View all 6 articles
There are over 6,000 different rare diseases estimated to impact 300 million people worldwide. As genetic testing becomes more common practice in the clinical setting, the number of rare disease diagnoses will continue to increase, resulting in the need for novel treatment options. Identifying treatments for these disorders is challenging due to a limited understanding of disease mechanisms, small cohort sizes, interindividual symptom variability, and little commercial incentive to develop new treatments. A promising avenue for treatment is drug repurposing, where FDA-approved drugs are repositioned as novel treatments. However, linking disease mechanisms to drug action can be extraordinarily difficult and requires a depth of knowledge across multiple fields, which is complicated by the rapid pace of biomedical knowledge discovery. To address these challenges, The Hugh Kaul Precision Medicine Institute developed an artificial intelligence tool, mediKanren, that leverages the mechanistic insight of genetic disorders to identify therapeutic options. Using knowledge graphs, mediKanren enables an efficient way to link all relevant literature and databases. This tool has allowed for a scalable process that has been used to help over 500 rare disease families. Here, we provide a description of our process, the advantages of mediKanren, and its impact on rare disease patients.
It is a challenge in translational research to efficiently bridge discoveries in biomedical research with clinical application. To address this issue, the National Center for Advancing Translational Sciences (NCATS) launched the Biomedical Data Translator program to accelerate translational medical research for furthering research discoveries and clinical implementation (Biomedical Data Translator Consortium, 2019). As part of this program, The Hugh Kaul Precision Medicine Institute (PMI) at the University of Alabama at Birmingham (UAB) Heersink School of Medicine has developed the artificial intelligence (AI) reasoning tool, mediKanren. mediKanren uses knowledge graphs (KGs) produced by teams in the NCATS Biomedical Data Translator program to provide a standardized way of describing the relationship between biomedical concepts (e.g., diseases, symptoms, drugs, proteins, and genes) (Biomedical Data Translator Consortium, 2019). KGs are organized into “triples” which consist of an edge connecting two concepts by a predicate (e.g., “gene A—regulates—gene B” or “gene B—is related to—disease Y”). Unlike typical literature searches, mediKanren triples with related concepts can be linked together, thus providing knowledge that would have otherwise required higher order thinking to establish these connections from multiple sources. Additionally, mediKanren returns this knowledge in a format that allows users to recognize both well-established concept connections and those that may not be well-known. Both types of connections enable the identification of novel therapeutics for rare and complex diseases.
There is a dire need for identifying treatment options for rare and complex diseases. Over 6,000 unique rare diseases have been identified, and it is estimated that over 300 million people worldwide are affected by a rare disease (Nguengang Wakap et al., 2020). Of these rare diseases, approximately 95% do not have an FDA-approved drug treatment and 80% are genetic in origin1. Similarly, complex diseases include disorders that involve multiple genes, have gene-environment interactions, and/or encompass related and unrelated symptoms that may not respond to standard treatments. As the clinical application of genome sequencing continues to expand, there is a greater chance for families and individuals to receive an accurate diagnosis. However, this increasing number of rare diagnoses puts pressure on the scientific research community to characterize these diseases and on clinicians to match patients with treatment options (Tabor and Goldenberg, 2018).
PMI has created a research consultation service that analyzes patient cases and uses mediKanren to aid in treatment identification and in disease characterization. In addition to mediKanren, the PMI research consultation program consists of a team of researchers, clinicians, and undergraduate analysts, of various disciplines, including neurobiology, precision oncology, genomic medicine, pharmacology/toxicology, and computer science. The research consultation service consists of (i) analyses of health-related data, including genetic data, of an individual's diagnosed medical conditions or the symptoms of their undiagnosed conditions and (ii) a consultation process that provides insight into the knowledge returned by mediKanren, either in the form of a physician report that outlines mediKanren-identified therapeutic options or a research report that outlines strategies for furthering disease characterization. It is important to note that the consultation process works in partnership with, but does not replace the role of, the participant's physician. In this article, we explain how the PMI research consultation process works, how mediKanren is used in rare and complex diseases, as well as demonstrate this process in selected cases where a therapeutic option was identified.
mediKanren is a reasoning engine developed under the NIH NCATS Biomedical Data Translator program (Biomedical Data Translator Consortium, 2019). It is based on miniKanren, a family of embedded logic programming languages especially suited for programming with pure relations and constraints. A medical knowledge graph is transformed into a database format that mediKanren then imports, exposing the graph to the programmer as a set of relations. These relations may be used like any other miniKanren relation, efficiently supporting queries in any direction by making use of database indexes. Typical relations built from a knowledge graph describe concepts (e.g., drugs, genes, diseases) and relationships between these concepts.
Each relationship between concepts is a claim (e.g., Drug X treats Disease Y) expressed as a graph edge in subject-verb-object form, where the subject and object are the concepts, and the verb is a predicate (e.g., “negatively regulates”) coming from a standardized ontology. A graph edge comes with annotations that describe features such as information provenance and various forms of evidence, such as publications, supporting the claim expressed by the edge.
Each concept is referenced by a CURIE2 (Compact Uniform Resource Identifier) and comes with annotations describing features such as a category (e.g., “gene”) coming from a standardized ontology, a human-readable name, and information provenance. In many cases, two or more concepts are synonyms, using different CURIEs to name the same underlying concept. In other cases, two or more concepts are not exactly equivalent but should be treated as synonyms for a particular reasoning use case. For these reasons, mediKanren supports optional concept normalization, allowing any concept to be substituted for one of its situationally declared synonyms. This enables the discovery of paths through a graph that would otherwise have been disconnected due to edges that mention different CURIEs for the same underlying concept. Because a CURIE can be an obscure way to refer to a concept, mediKanren also supports full-text search over the human-readable English names of concepts. This allows a user, in this case the student analysts, to type in a more familiar name, or even just part of a name, and discover one or more corresponding CURIEs.
The source code for mediKanren is covered under the MIT Free Software license, and is available at https://github.com/webyrd/mediKanren; however, mediKanren requires data contained in knowledge graphs, which require a separate download from a third-party. Contact the authors for help evaluating new uses of mediKanren.
mediKanren consumes structured data, as opposed to free text. Within NCATS Translator, teams dedicated to the construction of structured data are called “Knowledge Providers” (KPs), and the data sets they produce are known as “knowledge graphs” (KGs) (Biomedical Data Translator Consortium, 2019). Knowledge graphs are derived from various sources, including scientific free text, semi-structured data from electronic health records, and pre-curated biomedical databases. Each KG team is responsible for making their knowledge conform to a common ontology, known as a “Biolink” (Biomedical Data Translator Consortium, 2019). One central team in NCATS Translator is responsible for equating identities between knowledge graphs, creating a special KG called the “Node Normalizer”.
After being consented to the research and agreeing to participate, each participant's research consultation process is tracked as an individual PMI case. PMI uses UAB's HIPAA-compliant ShareFile3 system to store participants' consultation-related information. After agreeing to participate in the PMI research consultation service, participants themselves or their physicians upload health information through the file-drop feature of UAB ShareFile. Alternatively, HIPAA-trained PMI staff upload the data into the participant's data folder in UAB ShareFile after the participant shares his or her data through the initial participant questionnaire. This data includes participants' health information such as their genetic information (i.e., genetic report, whole-genome or whole-exome sequencing data), medical history, clinical symptoms, imaging reports, and lab tests. Additionally, any data collected from the outcome survey that is given to participants after the consultation process is kept in UAB Sharefile.
The project management tool, Airtable4, is used to manage and track PMI cases as they pass through the consultation pipeline. No protected health information (PHI) is included in Airtable; only de-identified information is tracked using a unique PMI case identification number. De-identified information that is included in the Airtable case-tracking system includes genetic variants, Human Phenotype Ontology (HPO) terms, strictly limited participant information (year of birth, sex, state/country), and case status (e.g., intake, case analysis, report draft).
For both the initial participant questionnaire and outcome survey, PMI uses the Qualtrics Research Suite5. Through a business associate agreement with Qualtrics that covers HIPAA, PHI is collected and stored in Qualtrics. Responses to the questionnaire and survey are only accessible to authorized personnel included in the IRB study protocol.
Once the genetic and clinical information is gathered, a case is assigned to an analyst. The case analysis process has two stages. The first stage consists of gathering information from databases and published literature on what is known about the gene(s) and the encoded protein(s). Frequently used databases include GeneCards, NCBI Gene, gnomAD, ClinGen, UniProt, the Human Protein Atlas, and the Research Collaboratory for Structural Bioinformatics (RCSB) Protein Data Bank; a full list of resources is provided in Supplementary Table 1 (Berman et al., 2000; U.S. National Library of Medicine, 2004; Rehm et al., 2015; Uhlén et al., 2015; Karczewski et al., 2020; Safran et al., 2021; UniProt Consortium, 2021). These sources are consulted to understand the molecular and biological function of the gene harboring the variant, as well as its cellular and tissue localization, protein structure and important domains, related pathways, and the human disorders associated with the gene or variant. This stage provides the context and background information needed to hypothesize the disease mechanism, which is the second stage of the case analysis process. In the second stage, the specific genetic variant is scrutinized to determine its potential impact on protein function, i.e., biological implication. This is accomplished by determining the location of the variant with regard to important protein domains or regions and previously reported pathogenic variants, as well as obtaining functional studies or model organism data published in peer-reviewed literature. The four major categories of biological implications include loss of activity, toxic activity, underactivity, and overactivity (Might and Crouse, 2022). For each of these categories, there are several therapeutic options to consider, as well as queries that can be formulated for mediKanren.
In cases where up-regulating or down-regulating a gene could be beneficial, mediKanren helps identify drugs or compounds, as well as additional gene targets, that may accomplish the desired outcome. Results from mediKanren are then exported into a spreadsheet to allow the analysts to filter and sort to pull out relevant therapeutic options, with each result linked to an article in PubMed. Analysts read these articles to validate the result and determine the strength of evidence for the therapeutic(s) by considering the concentration of the drug used, the preclinical or clinical nature of the evidence, and the magnitude of the drug response. Top drug candidates are then prioritized based on accessibility, bioavailability, and safety.
By design, return of results is not guided by any automatically calculated score from mediKanren. Instead, mediKanren is currently calibrated to return all (e.g., rare disease, drug) associations that are detectable with trusted data sets, and analysts manually review the results. Analysts predominantly use factors to evaluate the results that are not currently available in machine-processable data, including the meaning of text in returned articles, additional articles discovered by the analysts, current preclinical and clinical studies, and subject matter expertise on the case review team. Supplementary Table 1 resources also fall under the category of low machine accessibility, and thus resources that mediKanren currently does not know. Today, there is still a substantial amount of evidence required to produce a plausible research report that is hard to connect with automated methods.
In other domains, supervised labeling or benchmark data plays a major role. However, in our domain, no existing labeling or benchmark data sets rate efficacy for (e.g., rare disease, drug) pairs under consideration, due to two main factors. First, is the rareness of the diseases and their treatments. Second, is the significant analyst effort necessary to create each plausible labeled example (e.g., the research report). We have discussed publishing a corpus of cases which have risen to the level of a physician or research report (today over twenty participant cases), but at the project's current state of development, it is not yet clear how to publish such a corpus in a useful way.
In cases where an FDA-approved drug or well-studied nutraceutical is identified and predicted to positively impact the underlying disease mechanism, the results are vetted by the PMI clinical team. The proposed therapeutics that are deemed relatively safe by the medical directors are included in a research report that is shared with the participant's physician(s) of choice. This report includes a cover letter, participant background information, case analysis (i.e., gene/protein information and hypothesized variant impact), the mediKanren-identified therapeutic(s), and relevant publications.
A subset of cases was selected to highlight the utility of mediKanren and successful outcomes of the PMI program. These cases did not have a therapeutic option initially and were matched with at least one therapeutic through the case analysis process and subsequent use of mediKanren that went on to be trialed in a patient.
In some cases, genetic variants lead to decreased activity of the protein (partial loss-of-function or hypomorph) or total lack of protein function (total loss-of-function or amorphic). In cases where the disease is dominant (one allele is affected), one allele remains functional. In these scenarios, identifying a strategy that can increase the activity of the remaining protein or gene may compensate for the lost function and rescue the harmful effects of the gene variant.
One such case is a pediatric male participant hemizygous for an inherited missense variant in the gene TMLHE6, i.e., the protein epsilon-trimethyllysine hydroxylase, a key member of the carnitine biosynthesis pathway. Clinically, the participant experienced daily seizures, delayed speech development, and poor fine motor skills. During case analysis, an article was identified that reported another patient with a TMLHE variant that occurred near the PMI participant's variant (Nava et al., 2012). The functional analysis described in the article characterized the TMLHE variant as a loss-of-function, leading to an increase in trimethyllysine, the precursor of carnitine biosynthesis, and a slight decrease in carnitine levels (Nava et al., 2012). As a result, the PMI participant's variant was predicted to also result in a loss-of-function. Based on this analysis, the analyst used mediKanren to identify therapeutic strategies to compensate for the loss of trimethyllysine hydroxylase activity and decrease in carnitine biosynthesis. A mediKanren query for increasing carnitine revealed different forms of carnitine, including the FDA-approved dietary supplement levocarnitine (Figure 1). A research report outlining these findings, i.e., the case analysis and mediKanren results, was generated and sent to the participant's physician. Upon their review, the physician decided to move forward with a levocarnitine supplementation trial with their patient. Reports from the participant's family revealed that levocarnitine supplementation led to improvement of mobility. However, this information is anecdotal, and a full clinical trial is necessary to conclude that carnitine supplementation has a positive outcome for patients with this variant and others with similar impact on gene function.
Figure 1. Flowchart illustrating the workflow of the TMLHE case using mediKanren. Based on the conclusions of the case analysis, the analyst used mediKanren to identify therapeutic strategies to compensate for the loss of trimethyllysine hydroxylase activity and decrease in carnitine biosynthesis. One hundred and thirteen concepts were returned by mediKanren using the SemMedDB KG that were predicted to increase carnitine, and of those, levocarnitine is FDA-approved and supporting literature was reviewed to confirm the result. TMLHE, Trimethyllysine Hydroxylase, Epsilon; CURIE, compact uniform resource identifier; UMLS, Unified Medical Language System; FDA, Food and Drug Administration.
Finding the TMLHE treatment involved identifying a drug or supplement that would increase metabolites within the carnitine biosynthesis pathway, thereby compensating for the loss of trimethyllysine hydroxylase function. In this instance, the desired result was returned as a single triple “levocarnitine increases carnitine.” When results are connected in a single triple, we refer to these as one-hop queries.
Another common scenario encountered is where one-hop mediKanren queries do not lead to direct therapeutic outcomes, meaning there are no direct connections between the modulators and the gene. This could be the result of limited information known about the gene of interest or few potential therapeutics that target related cellular processes or pathways.
An example of this scenario was presented with two pediatric participants whose genetic sequencing revealed pathogenic missense variants in the gene RHOBTB27 (Rho Related BTB Domain Containing 2). Clinically, these participants presented with seizures, severe global developmental delay and regression, paroxysmal movement disorder, and hypotonia. Missense variants in the RHOBTB2 protein have been linked to decreased dendritic development, resulting in decreased dendritic arborization (Straub et al., 2018). Case analysis revealed both participant variants are in the ubiquitin domain of the RHOBTB2 protein, which is important for the degradation of RHOBTB2. Functional studies in Drosophila sp., along with case reports of affected individuals, have linked missense RHOBTB2 protein variants to decreased Rho GTPase protein degradation, increased protein accumulation in the central nervous system, and subsequent neuronal degradation, conferring a phenotype of developmental delay and epileptic encephalopathy (Belal et al., 2018; Straub et al., 2018).
The proposed therapeutic approach in these cases was to search for RHOBTB2 gene downregulators. However, there was a lack of direct RHOBTB2 inhibitors in the existing knowledge graphs in mediKanren at the time (Figure 2A). Therefore, another approach was taken. RHOBTB2 itself has regulators, which provide additional targets for mediKanren queries. For example, the gene E2F18, i.e., the protein E2F transcription factor 1, was identified by mediKanren as a positive regulator of RHOBTB2 transcription (Freeman et al., 2008). As demonstrated in Figure 2B, a subsequent query of E2F1 gene downregulators led to the identification of Celecoxib (Celebrex) (Dembo et al., 2005). This drug was selected because it was FDA-approved, well-tolerated in pediatric patients, and able to cross the blood-brain barrier. A research report for Celecoxib was generated and sent to each participant's physician. Upon their review, both physicians decided to move forward with a trial of Celecoxib in their patients.
Figure 2. Flowchart illustrating the workflow of the RHOBTB2 case using mediKanren. (A) Based on the conclusions of the case analysis, the analyst used mediKanren to identify therapeutic strategies that will regulate RHOBTB2 gene expression. Ten concepts were returned by mediKanren using the SemMedDB KG that were predicted to regulate RHOBTB2 expression, but none of the concepts were drugs or compounds. Therefore, a two-hop approach was taken with E2F1, a gene that was identified as a regulator of RHOBTB2. (B) A second query was run to look for downregulators of E2F1, which resulted in 212 concepts. After filtering for drugs, sorting by relevance, and manually reviewing for FDA-approved compounds that pass the blood-brain barrier, Celecoxib was identified. RHOBTB2, Rho Related BTB Domain Containing 2; CURIE, compact uniform resource identifier; UMLS, Unified Medical Language System; E2F1, E2F Transcription Factor 1.
Finding the RHOBTB2 treatment pathway involved combining a drug-to-gene relation (Celecoxib downregulates E2F1) with a gene-to-gene relation (E2F1 positively regulates RHOBTB2). Pathways of this shape are referred to as two-hop pathways, and queries to find two-hop pathways are known as two-hop queries.
mediKanren is also invaluable when it comes to situations where a condition may have several standard of care treatments, yet a patient still does not achieve relief after trying multiple treatment options. An example of this scenario was a young adult female whose family reached out to PMI to find additional therapeutic options for their daughter's cyclic vomiting. Cyclic vomiting syndrome is a disorder that is generally debilitating, resulting in episodes of severe nausea and vomiting. These symptoms, nausea and vomiting, became the targets for mediKanren queries to help identify all therapeutics that could prevent these episodes. The results with the most support, i.e., the most publications, were common antiemetics such as Ondansetron (Zofran), which were already tried by the participant. However, in the long tail of the results, as shown in Figure 3, where there were only a few publications associated with each result, isopropyl alcohol was reported to prevent nausea (Smiler and Srock, 1998; Cotton et al., 2007; Beadle et al., 2016). Specifically, the cited publications referred to nasally inhaled isopropyl alcohol. This was a simple and safe strategy the young woman could try at the onset of her nausea to prevent vomiting episodes. Upon review by PMI medical directors, this therapy was shared with the participant's physician. This treatment was reported by the participant to provide relief from her nausea and vomiting symptoms. While some could characterize this finding as a “forgotten fact”, anecdotally, this strategy of preventing nausea is a well-known trick among nurses who need to combat nausea. However, when taken out of that context, it would be considered a “forgotten fact” as it appeared in the long tail of results for treating or preventing nausea and vomiting.
Figure 3. Flowchart illustrating the workflow of the cyclic vomiting syndrome case using mediKanren. (A) Over 422 concepts were returned by mediKanren using the SemMedDB and RTX KGs that were predicted to treat or prevent nausea and/or vomiting. (B) Ondansetron was the concept with the most publications, whereas isopropyl alcohol was located in the tail-end of the results with only three publications. CURIE, compact uniform resource identifier; UMLS, Unified Medical Language System; HP, human phenotype.
When the news of SARS-CoV-2 began to loom in early 2020, the PMI team applied the case analysis process for rare disorders to identify repurposable drugs for COVID-19. As reports detailing the predicted molecular mechanisms of infection and replication were released, a list of host and viral protein targets was prioritized and became the subjects of mediKanren queries (Figure 4). One target of interest, the endothelial cell surface protein TMPRSS2, was determined to be the receptor by which the virus entered lung tissue, and as a result, the strategy was to identify FDA-approved drugs that decrease TMPRSS2 gene expression and therefore, decrease the ability of the virus to infect the host's lung tissue (Matsuyama et al., 2010). A mediKanren query for TMPRSS2 gene regulators revealed that androgens induce TMPRSS2 expression, thus a therapeutic strategy of androgen deprivation logically would decrease TMPRSS2 expression. Accordingly, discussions regarding a prescription medication known as Degarelix were initiated between PMI and the United States Veterans Affairs Office of Research and Development. Degarelix is a prescription medicine used in the treatment of advanced prostate cancer that works as a luteinizing hormone-releasing hormone (LHRH) antagonist, thus leading to lowered testosterone levels in the body. As a result, the Hormonal Intervention for the Treatment in Veterans With COVID-19 Requiring Hospitalization (HITCH) (NCT04397718) was initiated to test this hypothesis (Nickols et al., 2021). Although the HITCH trial reported that androgen suppression through Degarelix treatment did not improve outcomes in a small cohort of men hospitalized with COVID-19, other trials found proxalutamide, an androgen receptor antagonist, was an effective treatment for non-hospitalized patients with COVID-19 (Cadegiani et al., 2021; McCoy et al., 2021; Nickols et al., 2022). Therefore, we cannot exclude the possibility that androgen suppression may reduce time to clinical remission and/or reduces the rate of hospitalization for patients with COVID-19.
Figure 4. Flowchart illustrating the workflow of SARS-CoV-2 drug repurposing using mediKanren. Over 64 pro-viral and anti-viral targets were queried in mediKanren for negative and positive regulators, respectively. Over 1,300 drugs were returned by mediKanren using the RTX-KG2. These drugs were sorted by the number of publications and number of targets and manually reviewed to prioritize FDA-approved and safe drugs for testing in VeroE6 cells.
In addition to identifying anti-androgen drugs as potential preventative repurposable drugs for COVID-19, over 1,300 other drugs were identified with proposed antiviral activity using mediKanren (de Wilde et al., 2015). These drugs were then ranked by the analysts according to the amount of evidence, safety and accessibility, and predicted interactions using molecular simulations. The top-ranked candidates were then validated in VeroE6 cells and successful candidates were further tested in a lung-organoid model of SARS-CoV-2 infection at Dr. Kevin Harrod's lab at UAB. One of the most promising mediKanren drug candidates was D-α-tocopherol polyethylene glycol succinate (TPGS), which was later found to interact with the SARS-CoV-2 RNA-dependent RNA polymerase and synergize with Remdesivir, thereby inhibiting SARS-CoV-2 replication in VeroE6 cells (Pacl et al., 2021).
Drug repurposing is a powerful approach to therapeutic discovery for rare and complex diseases. As biomedical discoveries continue to contribute vast datasets and knowledge, an opportunity emerges for AI to assist in translating this biomedical knowledge into a format that can be searched by clinicians and researchers to better understand these diseases and identify therapeutic strategies for patients. In this paper, we discussed how PMI developed the biomedical reasoning AI tool, mediKanren, and the complementary research consultation service that connects patients, researchers, and clinicians to the knowledge contained within mediKanren.
In two rare disease cases, TMLHE and RHOBTB2, analyses of genetic and health-related data and disease-related publications unraveled molecular mechanisms and pathways that provided therapeutic targets for mediKanren. In both cases, a novel therapeutic was discovered, shared with the participants' physicians, and was given to the participant. In a case of cyclic vomiting syndrome, mediKanren captured standard and lesser-known treatment options for alleviating nausea-related symptoms. One of these lesser-known treatment options greatly reduced and helped prevent the onset of nausea and vomiting episodes in a young female. The participant reported a significant improvement in her quality of life due to this mediKanren-identified treatment option that would have not been caught by a simple literature database search. In the complex infectious disease case of SARS-CoV-2, mediKanren unveiled the pathway of androgen-induced expression of TMPRSS2, which ultimately led to a clinical trial of androgen deprivation therapy for decreasing disease severity. Additionally, a search for drugs and compounds related to human SARS anti-viral and pro-viral targets uncovered over 1,300 drug repurposing candidates for screening in a cell model.
At the time of this paper, mediKanren has identified therapeutics that are FDA-approved and relatively safe in over twenty participant cases. For each of these cases, a report was shared with a physician, and it has been confirmed in over half of these cases that the physicians moved forward with the treatments. In addition to the cases mentioned in this paper, other rare disorders that have matched with therapeutic options include non-ketotic hyperglycinemia, MAPK8IP3-related disorder, EGFR-associated acanthosis nigricans, and SETD1A-related disorder. We are currently in the process of submitting IRBs for open-label clinical trials for other cases. Lastly, two mediKanren identified therapeutics have been tested in clinical trials: Degarelix for COVID-19 (NCT04397718) and ketamine for ADNP syndrome (NCT04388774)9 (Nickols et al., 2021).
Unfortunately, most PMI cases do not result in a report to a physician for several reasons. The most common reasons include limited knowledge about a gene or variant, lack of mediKanren-identified therapeutics, or unsuitable therapeutic options (e.g., toxins, cancer treatments, treatments with significant side effect profiles). In these situations, more research is required before any treatment option is considered by a physician. Therefore, collaborations with researchers (basic and translational), foundations, and clinicians treating similar diseases are frequently sought out by PMI. The most fruitful and dynamic collaborations are with researchers who have models for specific genetic disorders and are willing to screen a limited number of drugs.
The Semantic Medline Database (SemMedDB) knowledge graph has been a cornerstone of the PMI consultation service. SemMedDB is a natural language processing (NLP) extraction of abstract-only text from PubMed. The NLP program that produces SemMedDB is SemRep (Kilicoglu et al., 2020). The RTX-KG2 knowledge graph incorporates SemMedDB, which is in turn consumed by mediKanren (Wood et al., 2021). PMI's case analysis process inspired the development of RTX-KG2 after Dr. Steve Ramsey noted how including the research article that supports an edge in the biomedical knowledge graph, especially the specific sentence excerpt on which the edge is based, is critical to enable analysts to maximally leverage human judgment in the interpretation of mediKanren results. Accordingly, RTX-KG2 provides literature provenance information wherever it is available, such as for edges from SemMedDB, Jensen Lab Diseases, and RepoDB. Feedback from PMI based on experiences using RTX-KG2 within mediKanren led to multiple improvements for RTX-KG2 including improvements to semantic type annotations, the documentation of constituent sources, and additions of knowledge sources, such as DGIdb (Wood et al., 2021).
SemMedDB also has shortcomings that reveal research opportunities with high impact, both scientific and humanitarian. For instance, the predicates in SemMed have varied widely in their applicability to PMI's work. Predicates “inhibits” (i.e., biolink:negatively_regulates) and “stimulates” (i.e., biolink:positively_regulates) have been indispensable, while vague predicates such as “associated_with” (i.e., biolink:related_to) have been much less helpful. Additionally, each structured datum in SemMedDB includes the PubMed text sentence with which it matched; the matched text has proved helpful in allowing users to manually throw out low confidence matches before investing time in reading the abstract or article. However, even when full PubMed article text has open access copyright, and is therefore likely to contain useful matches within the text, SemMedDB/SemRep is limited to only reading the abstract.
The authors of SemMedDB/SemRep recently used the BioCreative V benchmark to compare their system with two newer (2016) systems and concluded that the two newer systems performed better on F1 score and much better on recall (Peng et al., 2016; Xu et al., 2016; Kilicoglu et al., 2020). High recall is necessary when the goal is to investigate all possibilities, as is the case for participants who match to only a few or no drugs. Therefore, integration with newer NLP techniques could make a substantial improvement. Lastly, NLP systems cannot access articles behind copyright paywalls. These articles may contain information on life-saving therapeutic options, but unless the research findings become available to the public and accessible by NLP systems, NLP systems cannot help in making the findings accessible.
With the development of mediKanren and the research consultation service, PMI has had a significant impact on many individuals, patient communities, as well as the field of translational science. To scale up this process and help reach more individuals, a few key elements must be addressed.
One challenge to address is keeping up with the large number of patient referrals to PMI. A current strategy is the creation of formal training modules that will teach UAB undergraduates how to analyze genetic information, assess the variant impact, formulate a molecular hypothesis, and use mediKanren to identify potential therapeutics. Through remote online learning, additional undergraduates at participating institutions can also be trained as precision medicine analysts. By having students go through an onboarding process, they will feel more confident in applying their knowledge and skills to participant cases. Additionally, they will gain experience with each case they take on, thus gradually growing their knowledge and developing their critical thinking skills. This will allow for more students from any background and previous training, or lack thereof, to be a part of the process, and therefore, gain relevant research and medical training while expanding the number of active cases in the program at any given time.
There are also various improvements that can be made to mediKanren, to not only improve the accuracy of the results but also expand access to new features such as knowledge graphs and potential queries that can be run. Lastly, progress is underway to have a web interface for the NCATS Translator project's reasoners, including mediKanren. We hope this effort will ultimately make mediKanren easily usable by all interested parties. PMI plays an active role as a source of subject matter experts (SMEs) for the NCATS Biomedical Data Translator program, providing insights into what features and knowledge graphs would help accelerate and improve the research consultation process and better serve researchers and clinicians.
The original contributions presented in the study are included in the article/Supplementary material, further inquiries can be directed to the corresponding author/s.
The studies involving human participants were reviewed and approved by the University of Alabama at Birmingham Institutional Review Board. Written informed consent to participate in this study was provided by the participants' legal guardian/next of kin.
MM, AC, and WB contributed to the conception and design of mediKanren and the PMI research consultation service. WB designed and implemented the mediKanren graphical user interface. GR designed and implemented the graph database engine and reasoning back-end of mediKanren, made improvements to the mediKanren graphical user interface, and wrote the mediKanren section of the manuscript. AF, CC, JH, WB, GR, and AC wrote the sections of the manuscript. AF and CC wrote the first draft of the manuscript. WB, JH, GR, MP, KH, TT-N, MZ, JO, and NA made technical contributions to mediKanren. SR contributed essential knowledge graphs for mediKanren. NA worked with WB on queries for COVID-19 drug repurposing. MZ wrote advanced mediKanren queries to explore advanced precision medicine queries, with guidance from MP, TT-N, GR, WB, and NA. UAB Precision Medicine Institute performed the case analysis and consultation process of the research consultation service. All authors contributed to manuscript revision, read, and approved the submitted version.
Support for this work was provided by the National Center for Advancing Translational Sciences, National Institutes of Health, through the Biomedical Data Translator Program, awards OT2TR003435, OT2TR002517, and OT2TR003428. Any opinions expressed in this document are those of the Translator community at large and do not necessarily reflect the views of NCATS, individual Translator team members, or affiliated organizations and institutions. Support for MZ was provided by the Harvard College Research Program (HCRP) through the Harvard College Office of Undergraduate Research and Fellowships.
Stephen Barnes, William E. Byrd, Mei-Jan Chen, Andrew B. Crouse, Camerron M. Crowder, Mary E. Crumbley, Madeline Eckenrode, Crayton A. Fargason, Jr., Nathaniel Fehrmann, Aleksandra Foksinska, Kaiwen He, Forest Huls, Matthew Jarrell, Lindsay Jenkins, Meg McCalley, Matthew Might, Tamsyn Osborn, Michael J. Patton, Elizabeth Pollard, Gregory Rosenblatt, Sienna Rucka, Nicholas T. Southern, Thi K. Tran-Nguyen, Jillian Tinglin, and Jordan H. Whitlock.
We thank Jim Cimino and Jake Chen of UAB's Informatics Institute for their ideas and suggestions during the NCATS Translator Feasibility Phase. We also thank Chris Austin, Joni Rutter, Christine Colvis, Noel Southall, and the rest of the current and former NCATS team for their vision and leadership of the Biomedical Data Translator Program. We also thank the other Translator teams for their support and friendly working relationships. We have benefited especially from the advice of: Andrew Su at Scripps; Maureen Hoatlin (formerly of OSHU, now independent), Matt Brush at OSHU, Chris Mungall at LBNL, Chris Bizon at RECNI/UNC, Melissa Haendel at the University of Colorado Anschutz Medical Campus, and Paul Clemons at the Broad Institute. We are also grateful to all of the teams that have provided us knowledge graphs, beginning with Andrew Su and Greg Stupp, who supplied the initial Biolink-compatible versions of SemMedDB. The ROBOKOP knowledge graph from the team at RENCI has also been invaluable. The members of the ARAX team have created and refined multiple versions of the RTX-KG2 knowledge graph specifically for our needs (Wood et al., 2021). Richard Bruskiewich of the SRI Team created a specialized SemMedDB knowledge graph for us, based on Greg Stupp's original data transformation scripts. Bill Baumgartner of the Text Mining Provider team at the University of Colorado Anschutz Medical Campus created a custom drug/gene expression knowledge graph for us. We also thank Deepak Unni and Chris Mungall for helping us ingest their COVID-19 knowledge graph. Will Byrd, Greg Rosenblatt, and Michael Patton greatly benefited from visiting other Translator teams; we thank everyone for their hospitality, especially: Maureen Hoatlin, Melissa Haendel, and Matt Brush for our OSHU visit; Chris Mungall, Marcin Joachimiak, Deepak Unni, and Seth Carbon for our LBNL visit; and Paul Clemons, Jason Flannick, Vlado Dancik, Mathias Wawer, and Marcin von Grotthuss for our visits to the Broad Institute. We also thank Paul Clemons, Steve Ramsey, Melissa Haendel, Chris Bizon, and Eugene Muratov for visiting us at UAB. Gregory Hyde, Andrew Su, and Colleen Xu have contributed to the ongoing virtual case reviews. We thank the teams and institutions that hosted in-person Translator hackathons for their hospitality, as well: Scripps (La Jolla), RENCI (Chapel Hill), OSHU (Portland), NCATS (Bethesda), and ISB (Seattle). We thank everyone who has used mediKanren and given us feedback over the past 4 years. The Biomedical Data Translator Programs' subject-matter experts have been especially helpful, especially Maureen Hoatlin. The online mini hackathons arranged by Karamarie Fecho from RENCI also helped us understand the strengths and limitations of our tools and knowledge sources.
Author JH is employed by Groovescale.
The remaining authors declare that the research was conducted in the absence of any commercial or financial relationships that could be construed as a potential conflict of interest.
All claims expressed in this article are solely those of the authors and do not necessarily represent those of their affiliated organizations, or those of the publisher, the editors and the reviewers. Any product that may be evaluated in this article, or claim that may be made by its manufacturer, is not guaranteed or endorsed by the publisher.
The Supplementary Material for this article can be found online at: https://www.frontiersin.org/articles/10.3389/frai.2022.910216/full#supplementary-material
1. ^Global Genes (2021). RARE Disease Facts. Global Genes. Available online at: https://globalgenes.org/rare-disease-facts/ (accessed March 25, 2022).
2. ^W3C (2010). CURIE Syntax 1.0. Available online at: https://www.w3.org/TR/curie/ (accessed March 27, 2022).
3. ^Citrix ShareFile. Available online at: https://www.citrix.com/lp/sharefile/ (accessed March 23, 2022).
4. ^Available online at: https://www.airtable.com/ (accessed March 18, 2022).
5. ^Qualtrics (2005). Qualtrics. Available online at: https://www.qualtrics.com (accessed March 18, 2022).
6. ^NCBI TMLHE trimethyllysine hydroxylase, epsilon [Homo sapiens (human)]. Gene. Available at: https://www.ncbi.nlm.nih.gov/gene/55217 (accessed March 18, 2022).
7. ^NCBI RHOBTB2 Rho related BTB domain containing 2 [Homo sapiens (human)]. Gene. Available at: https://www.ncbi.nlm.nih.gov/gene/23221 (accessed March 17, 2022).
8. ^NCBI E2F transcription factor 1 [Homo sapiens (human)]. Gene. Available online at: https://www.ncbi.nlm.nih.gov/gene/1869 (accessed March 18, 2022).
9. ^NIH National Library of Medicine ClinicalTrials.gov. Low-Dose Ketamine in Children With ADNP Syndrome. Available online at: https://clinicaltrials.gov/ct2/show/NCT04388774 (accessed March 18, 2022).
Beadle, K. L., Helbling, A. R., Love, S. L., April, M. D., and Hunter, C. J. (2016). Isopropyl alcohol nasal inhalation for nausea in the emergency department: a randomized controlled trial. Ann. Emerg. Med. 68, 1.e1–9.e1. doi: 10.1016/j.annemergmed.2015.09.031
Belal, H., Nakashima, M., Matsumoto, H., Yokochi, K., Taniguchi-Ikeda, M., Aoto, K., et al. (2018). De novo variants in RHOBTB2, an atypical Rho GTPase gene, cause epileptic encephalopathy. Hum. Mutat. 39, 1070–1075. doi: 10.1002/humu.23550
Berman, H. M., Westbrook, J., Feng, Z., Gilliland, G., Bhat, T. N., Weissig, H., et al. (2000). The protein data bank. Nucleic Acids Res. 28, 235–242. doi: 10.1093/nar/28.1.235
Biomedical Data Translator Consortium (2019). Toward A universal biomedical data translator. Clin. Transl. Sci. 12, 86–90. doi: 10.1111/cts.12591
Cadegiani, F. A., McCoy, J., Gustavo Wambier, C., Vaño-Galván, S., Shapiro, J., Tosti, A., et al. (2021). Proxalutamide significantly accelerates viral clearance and reduces time to clinical remission in patients with mild to moderate COVID-19: results from a randomized, double-blinded, placebo-controlled trial. Cureus 13, e13492. doi: 10.7759/cureus.13492
Cotton, J. W., Rowell, L. R., Hood, R. R., and Pellegrini, J. E. (2007). A comparative analysis of isopropyl alcohol and ondansetron in the treatment of postoperative nausea and vomiting from the hospital setting to the home. AANA J. 75, 21–26.
de Wilde, A. H., Wannee, K. F., Scholte, F. E. M., Goeman, J. J., Ten Dijke, P., Snijder, E. J., et al. (2015). A kinome-wide small interfering RNA screen identifies proviral and antiviral host factors in severe acute respiratory syndrome coronavirus replication, including double-stranded RNA-activated protein kinase and early secretory pathway proteins. J. Virol. 89, 8318–8333. doi: 10.1128/JVI.01029-15
Dembo, G., Park, S. B., and Kharasch, E. D. (2005). Central nervous system concentrations of cyclooxygenase-2 inhibitors in humans. Anesthesiology 102, 409–415. doi: 10.1097/00000542-200502000-00026
Freeman, S. N., Ma, Y., and Cress, W. D. (2008). RHOBTB2 (DBC2) is a mitotic E2F1 target gene with a novel role in apoptosis. J. Biol. Chem. 283, 2353–2362. doi: 10.1074/jbc.M705986200
Karczewski, K. J., Francioli, L. C., Tiao, G., Cummings, B. B., Alföldi, J., Wang, Q., et al. (2020). The mutational constraint spectrum quantified from variation in 141,456 humans. Nature 581, 434–443. doi: 10.1038/s41586-020-2308-7
Kilicoglu, H., Rosemblat, G., Fiszman, M., and Shin, D. (2020). Broad-coverage biomedical relation extraction with SemRep. BMC Bioinform. 21, 188. doi: 10.1186/s12859-020-3517-7
Matsuyama, S., Nagata, N., Shirato, K., Kawase, M., Takeda, M., and Taguchi, F. (2010). Efficient activation of the severe acute respiratory syndrome coronavirus spike protein by the transmembrane protease TMPRSS2. J. Virol. 84, 12658–12664. doi: 10.1128/JVI.01542-10
McCoy, J., Goren, A., Cadegiani, F. A., Vaño-Galván, S., Kovacevic, M., Situm, M., et al. (2021). Proxalutamide reduces the rate of hospitalization for COVID-19 male outpatients: a randomized double-blinded placebo-controlled trial. Front. Med. 8, 668698. doi: 10.3389/fmed.2021.668698
Might, M., and Crouse, A. B. (2022). Why rare disease needs precision medicine-and precision medicine needs rare disease. Cell Rep. Med. 3, 100530. doi: 10.1016/j.xcrm.2022.100530
Nava, C., Lamari, F., Héron, D., Mignot, C., Rastetter, A., Keren, B., et al. (2012). Analysis of the chromosome X exome in patients with autism spectrum disorders identified novel candidate genes, including TMLHE. Transl. Psychiatry 2, e179. doi: 10.1038/tp.2012.102
Nguengang Wakap, S., Lambert, D. M., Olry, A., Rodwell, C., Gueydan, C., Lanneau, V., et al. (2020). Estimating cumulative point prevalence of rare diseases: analysis of the Orphanet database. Eur. J. Hum. Genet. 28, 165–173. doi: 10.1038/s41431-019-0508-0
Nickols, N. G., Goetz, M. B., Graber, C. J., Bhattacharya, D., Soo Hoo, G., Might, M., et al. (2021). Hormonal intervention for the treatment of veterans with COVID-19 requiring hospitalization (HITCH): a multicenter, phase 2 randomized controlled trial of best supportive care vs best supportive care plus degarelix: study protocol for a randomized controlled trial. Trials 22, 431. doi: 10.1186/s13063-021-05389-0
Nickols, N. G., Mi, Z., DeMatt, E., Biswas, K., Clise, C. E., Huggins, J. T., et al. (2022). Effect of androgen suppression on clinical outcomes in hospitalized men with COVID-19: the HITCH randomized clinical trial. JAMA Netw. Open 5, e227852. doi: 10.1001/jamanetworkopen.2022.7852
Pacl, H. T., Tipper, J. L., Sevalkar, R. R., Crouse, A., Crowder, C., UAB Precision Medicine Institute, et al. (2021). Water-soluble tocopherol derivatives inhibit SARS-CoV-2 RNA-dependent RNA polymerase. bioRxiv. doi: 10.1101/2021.07.13.449251
Peng, Y., Wei, C.-H., and Lu, Z. (2016). Improving chemical disease relation extraction with rich features and weakly labeled data. J. Cheminform. 8, 53. doi: 10.1186/s13321-016-0165-z
Rehm, H. L., Berg, J. S., Brooks, L. D., Bustamante, C. D., Evans, J. P., Landrum, M. J., et al. (2015). ClinGen–the clinical genome resource. N. Engl. J. Med. 372, 2235–2242. doi: 10.1056/NEJMsr1406261
Safran, M., Rosen, N., Twik, M., BarShir, R., Stein, T. I., Dahary, D., et al. (2021). “The GeneCards suite,” in Practical Guide to Life Science Databases, eds I. Abugessaisa and T. Kasukawa (Singapore: Springer Singapore), 27–56. doi: 10.1007/978-981-16-5812-9_2
Smiler, B. G., and Srock, M. (1998). Isopropyl alcohol for transport-related nausea. Anesthesia Analgesia 87, 1214. doi: 10.1213/00000539-199811000-00055
Straub, J., Konrad, E. D. H., Grüner, J., Toutain, A., Bok, L. A., Cho, M. T., et al. (2018). Missense variants in RHOBTB2 cause a developmental and epileptic encephalopathy in humans, and altered levels cause neurological defects in Drosophila. Am. J. Hum. Genet. 102, 44–57. doi: 10.1016/j.ajhg.2017.11.008
Tabor, H. K., and Goldenberg, A. (2018). What precision medicine can learn from rare genetic disease research and translation. AMA J. Ethics 20, E834–E840. doi: 10.1001/amajethics.2018.834
U.S. National Library of Medicine. (2004). National Center for Biotechnology Information (NCBI) Gene. National Center for Biotechnology Information. Available online at: https://www.ncbi.nlm.nih.gov/gene/ (accessed March 17, 2022).
Uhlén, M., Fagerberg, L., Hallström, B. M., Lindskog, C., Oksvold, P., Mardinoglu, A., et al. (2015). Proteomics. Tissue-based map of the human proteome. Science 347, 1260419. doi: 10.1126/science.1260419
UniProt Consortium (2021). UniProt: the universal protein knowledgebase in 2021. Nucleic Acids Res. 49, D480–D489. doi: 10.1093/nar/gkaa,1100
Wood, E. C., Glen, A. K., Kvarfordt, L. G., Womack, F., Acevedo, L., Yoon, T. S., et al. (2021). RTX-KG2: a system for building a semantically standardized knowledge graph for translational biomedicine. bioRxiv. doi: 10.1101/2021.10.17.464747
Keywords: rare disease, precision medicine, drug repurposing, artificial intelligence, biomedical reasoning
Citation: Foksinska A, Crowder CM, Crouse AB, Henrikson J, Byrd WE, Rosenblatt G, Patton MJ, He K, Tran-Nguyen TK, Zheng M, Ramsey SA, Amin N, Osborne J, UAB Precision Medicine Institute and Might M (2022) The precision medicine process for treating rare disease using the artificial intelligence tool mediKanren. Front. Artif. Intell. 5:910216. doi: 10.3389/frai.2022.910216
Received: 01 April 2022; Accepted: 23 August 2022;
Published: 30 September 2022.
Edited by:
Yanji Xu, National Institutes of Health (NIH), United StatesReviewed by:
Tingyou Wang, Northwestern University, United StatesCopyright © 2022 Foksinska, Crowder, Crouse, Henrikson, Byrd, Rosenblatt, Patton, He, Tran-Nguyen, Zheng, Ramsey, Amin, Osborne, UAB Precision Medicine Institute and Might. This is an open-access article distributed under the terms of the Creative Commons Attribution License (CC BY). The use, distribution or reproduction in other forums is permitted, provided the original author(s) and the copyright owner(s) are credited and that the original publication in this journal is cited, in accordance with accepted academic practice. No use, distribution or reproduction is permitted which does not comply with these terms.
*Correspondence: Aleksandra Foksinska, YWZva3NpbkB1YWIuZWR1
Disclaimer: All claims expressed in this article are solely those of the authors and do not necessarily represent those of their affiliated organizations, or those of the publisher, the editors and the reviewers. Any product that may be evaluated in this article or claim that may be made by its manufacturer is not guaranteed or endorsed by the publisher.
Research integrity at Frontiers
Learn more about the work of our research integrity team to safeguard the quality of each article we publish.