Corrigendum: The impact of pedagogical agents' gender on academic learning: a systematic review
- 1Aix Marseille Univ, CNRS, LIS UMR 7020, Marseille, France
- 2Aix Marseille Univ, CNRS, LPC, Marseille, France
- 3Pôle pilote Ampiric, Institut National Supérieur du Professorat et de l'Éducation, Aix-Marseille Université, Marseille, France
Virtual learning environments often use virtual characters to facilitate and improve the learning process. These characters, known as pedagogical agents, can take on different roles, such as tutors or companions. Research has highlighted the importance of various characteristics of virtual agents, including their voice or non-verbal behaviors. Little attention has been paid to the gender-specific design of pedagogical agents, although gender has an important influence on the educational process. In this article, we perform an extensive review of the literature regarding the impact of the gender of pedagogical agents on academic outcomes. Based on a detailed review of 59 articles, we analyze the influence of pedagogical agents' gender on students' academic self-evaluations and achievements to answer the following questions: (1) Do students perceive virtual agents differently depending on their own gender and the gender of the agent? (2) Does the gender of pedagogical agents influence students' academic performance and self-evaluations? (3) Are there tasks or academic situations to which a male virtual agent is better suited than a female virtual agent, and vice versa, according to empirical evidence? (4) How do a virtual agent's pedagogical roles impact these results? (5) How do a virtual agent's appearance and interactive capacities impact these results? (6) Are androgynous virtual agents a potential solution to combatting gender stereotypes? This review provides important insight to researchers on how to approach gender when designing pedagogical agents in virtual learning environments.
1. Introduction
Pedagogical agents are virtual characters in digital environments used to improve learning in educational settings (Mohtadi et al., 2014; Schroeder et al., 2017). They can take on different roles, such as expert, mentor, or motivator (Baylor and Kim, 2005). As shown in a meta-analytic review of 43 studies by Schroeder et al. (2013), pedagogical agents can have a positive effect on students' free recall ability, knowledge retention, and transfer of prior knowledge to new situations or problems. However, some characteristics of pedagogical agents may impact the learning process: for instance, how realistic the virtual agents' appearance is Baylor and Kim (2004), the way they communicate with learners, verbally or nonverbally, positively or negatively (Gratch et al., 2007; Pecune et al., 2016), or the way they deliver feedback, using voice, text, or both (Kim and Baylor, 2016).
Virtual agents' gender is another feature that users can perceive from the agents' appearance (Lee, 2003). Yet few studies have evaluated the impact of pedagogical agents' gender, which is surprising considering the amount of research in Social Cognition documenting the impact of the gender of both learners and teachers on academic learning. Social Cognition and human-to-human studies are particularly interesting in the domain of virtual agents, as research shows that individuals have a propensity to interact with virtual agents as if they were human (Nass and Moon, 2000). Research in Social Cognition and Cognitive Psychology can, therefore, be enlightening for understanding users' perception of virtual characters and the effect of these perceptions on their performance. This is why we present some major Social Cognition research on the impact of learners and teachers' gender on learners' academic outcomes. For instance, Sansone (2019) conducted a survey on the link between high school students' beliefs about women's abilities in math and science and their teacher's gender, finding that students were less likely to report that men are better than women in math/science when assigned to female teachers. Teachers' behavior can also impact girls' and boys' learning differently: a large scale survey conducted by Forgasz and Leder (1996) showed that students who perceived their math teachers to be interested in them as individuals were more likely to have functional beliefs about themselves in mathematics, and this was more critical for female learners than male learners. Core beliefs represent general and strongly held views about ourselves, others, and the world; they influence the way we react in different circumstances. Functional beliefs are rational thought patterns that are generally useful for individuals to achieve their goals (Ellis, 1962). In the forementioned study, math teachers' behaviors seemed to favor boys over girls: boys had more interactions with their teachers, teachers were more tolerant of boys' misbehavior, and they had higher expectations of boys (Forgasz and Leder, 1996). A meta-analysis conducted by Lindberg et al. (2010) from 242 studies published between 1990 and 2007 indicated that while male and female learners performed similarly in mathematics, female students reported higher anxiety, more discomfort, and lower interest and self-efficacy in math classes than male students. Parents themselves tend to attribute different explanations for their children's academic performance depending on their gender: they explain their sons' mathematical success as due to their natural talent, whereas they explain their daughters' as due to their effort (Yee and Eccles, 1988). These results were replicated by Räty et al. (2002) who also found that parents of boys evaluated their child's mathematical competence as higher than parents of girls, and parents of girls perceived them as surpassing boys in reading. Despite this, parents still attributed competence in reading as resulting from the effort of girls but to the natural talent of boys. By explaining their daughters' success in math as due to effort, the authors suggested that parents may undermine both their own and their daughters' estimation of their daughters' success in mathematics, hence raising possible doubts about their future success in a domain that they think gets increasingly complicated; meanwhile, they may encourage boys to develop greater confidence in their future success (Yee and Eccles, 1988).
All these differences reflect the influence of gender stereotypes that lead people to consider men to be better at math than women, and women to be better in liberal arts -such as literature, e.g.,- than men. In addition, studies have shown that the fear of being negatively stereotyped in a skill area produces negative thoughts, which in turn reduce individuals' working memory capacity and impair learning and performance (Schmader and Johns, 2003). This phenomenon, called Stereotype Threat (Steele and Aronson, 1995), applies to different stereotypes and social groups, such as boys in reading tests (Pansu et al., 2016) and girls and women in math tests (Régner et al., 2014). The effects of Stereotype Threat can be reduced using different strategies, such as reading a story about a successful role model before taking a test (Bagès and Martinot, 2011; Bagès et al., 2016).
Presenting pedagogical agents as role models could be a potential solution for reducing the effects of Stereotype Threat. Researchers designing agents should take into account the gender of both learners and pedagogical agents to adapt the agent to the learners. The advantages of adapting virtual agents to participants have been demonstrated in several studies. For instance, in Vilaro et al. (2021), participants (all Black women) liked Black female agents for being artificial, hence creating a sense of trust and freedom where participants could avoid inherent biases and racism. In virtual learning environments, research has shown the impact of virtual agents' gender on human-agent interactions (refer to Section 3.4). However, the gender of pedagogical virtual agents is rarely considered an important characteristic in the design of virtual learning environments, whereas most pedagogical agents are human-like, and their gender can have an impact on academic outcomes (refer to Section 3.5). In terms of perception, various studies have shown that male virtual agents are rated as more powerful (Nunamaker et al., 2011), more expert (Nunamaker et al., 2011), and more knowledgeable (Baylor and Kim, 2004), whereas female agents are rated as more likable (Nunamaker et al., 2011) and more attractive (Lunardo et al., 2016). These attributes are important in learning environments, as competent and expert agents improve learners' performance (Baylor and Kim, 2004), and likable and attractive agents improve learners' self-perception including their self-efficacy (feeling of achievement) (Rosenberg-Kima et al., 2008), which may help improve their performance (Plant et al., 2009).
In this article, we present an extensive state of the art focusing on the effects of pedagogical agents' gender in virtual learning environments. We explore the impact of gender on the users' perceptions of agents and on their learning.
This article is organized as follows. In the next section, we explain our methodology used to conduct the state of the art and particularly how we used the PRISMA method to select relevant articles (Webster and Watson, 2002). In Section 3, the selected articles are summarized in Tables 1, 2 to provide a comprehensive review of research on the impact of pedagogical agents' gender on learners' performance and self-perception in academic domains. We discuss the articles summarized in the tables in Section 3.4 and Section 3.5. In Section 3.4, we address research highlighting the impact of virtual agents' gender on users' perceptions. In Section 3.5, we focus on pedagogical agents and the impact of their gender on learners' academic outcomes. The last section discusses what could be done in future research on virtual learning environments to reduce gender stereotypes and improve learners' performance, and the important research questions that arise from this review.

Table 1. Summary of articles on perceptive studies of virtual agents depending on their gender, regardless of the application domain.
2. Methods
2.1. Search Strategy
This article examines research on the impact of virtual agents' gender on learners but also more generally on users' behavior and perceptions. For this purpose, we reviewed articles from the Web of Science database over 21 years from 2000 to 2021. To collect the relevant studies, we conducted an online database search with the query gender+(“virtual agent*” OR “virtual character*”). This systematic review was conducted according to the PRISMA guidelines presented in Figure 1 (Webster and Watson, 2002) as follows: (1) scanning databases and starting with the major contributions in the leading journals, (2) reviewing the citations for the articles identified in step 1 to determine prior articles that should be considered, and (3) identifying articles citing the key articles identified in the previous steps. We used Google Scholar for the last step. A total of 120 articles were retained after following these steps.
2.2. Selection of Articles
From this set of articles, we selected empirical studies analyzing the effect of virtual agents' gender on users' perceptions, behaviors, and academic outcomes. We only took into account embodied virtual agents (i.e., we excluded studies on vocal assistants). We focused on Western culture and, thus, only selected papers relating to this culture. We eliminated articles only about avatars (users embodying a virtual agent) which were mainly about video games. In the end, we retained a set of 59 articles. We distinguished two types of research articles: Perceptive studies of virtual agents depending on their gender, regardless of the application domain, and research studies on the impact of gendered virtual agents in the context of a learning task.
This systematic review focuses on how virtual agents are designed, and the impact of their gender on different academic outcomes (motivation, learning, interest), but also on participants' perceptions of the agents. The research questions guiding this review are as follows:
1. Do students perceive virtual agents differently depending on their own gender and the gender of the agent?
2. Does the gender of pedagogical agents influence students' academic performance and self-evaluations?
3. Are there tasks or academic situations to which a male virtual agent is better suited than a female virtual agent, and vice versa, according to empirical evidence?
4. How do a virtual agent's pedagogical roles impact these results?
5. How do a virtual agent's appearance and interactive capacities impact these results?
6. Are androgynous virtual agents a potential solution to combatting gender stereotypes?
3. Systematic Review of Virtual Agents' Gender and Its Impact on Users' Perceptions and Academic Outcomes
In this section, we first review the different measures used to assess users' perceptions of agents, users' learning, and self-evaluations. We then highlight the persistence of gender stereotypes in human-machine interactions by presenting research on users' perceptions of virtual agents depending on their gender (Section 3.4). Second, we focus on pedagogical agents and discuss research that shows the effect of their gender on learners (Section 3.5).
3.1. Subjective Measures of Users' Perceptions of Agents'
Most of the studies used post-experience questionnaires to assess users' perceptions of virtual agents. Likert scale items were used to determine participants' stereotyped attributions of the agents, corresponding to communal traits stereotypically associated with women (e.g., affectionate, compassionate, sensitive, inviting, helpful), agency traits stereotypically associated with men (e.g., arrogant, ambitious, aggressive, courageous, and decisive), and competence traits associated more often with men (e.g., knowledgeable, intelligent, expert, credible, creative, innovative and organized) (Lee, 2003; Nunamaker et al., 2011; Feng et al., 2017; Khashe et al., 2017; van der Lubbe and Bosse, 2017; Kantharaju et al., 2018; Sczesny et al., 2018). Another questionnaire was sometimes used to determine which stereotypical gendered traits users applied to the agents (Kulms et al., 2011). This scale, the Bem Sex Role Inventory (BSRI) developed by Bem (1974), measures the construction of the gender schema of individuals, aims to highlight androgyny, and questions the usual dichotomy of female/male gendered traits stereotypically attributed to people. The BSRI consists of 20 positive items stereotypically associated with men (e.g., independent, analytical), 20 other positive items stereotypically associated with women (e.g., compassionate, loves children), and 20 other positive neutral items (e.g., tactful, reliable). The agents' gender perception was evaluated with a 5-point Likert sliding scale, e.g., with men=1, androgynous=4, and women=7 (Lee, 2003; McDonnell et al., 2009; Niculescu et al., 2009; Nag and Yalçın, 2020). Other social attitude perceptions were also assessed with Likert scale items, such as the perceived friendliness, trustworthiness, likability, and social presence of the agent (Lee, 2003; Guadagno et al., 2007; Nunamaker et al., 2011; Lunardo et al., 2016; Khashe et al., 2017; Akbar et al., 2018). Social presence is particularly important as it provides individuals with the possibility of developing a relationship or having a social interaction with one another, as they recognize each other as "social beings" (Biocca et al., 2003). Social presence is commonly defined as the sensation of being in the presence of a real person and having access to their feelings (Biocca, 1997), and can be assessed with a 5-item survey (e.g., “I feel that the person is watching me and is aware of my presence”) (Bailenson et al., 2001) and the Networked Minds Questionnaire (e.g., “The other individual didn't notice me in the room”) (Biocca et al., 2001), as used by Kulms et al. (2011).
3.2. Objective Measures of Learning
The impact of pedagogical agents on users' learning can be assessed by measuring users' performance in an exercise by comparing different conditions: for example, virtual agents with different behaviors (Chang et al., 2019), the presence of gendered virtual agents (Kim and Wei, 2011), or virtual agents with different genders (Kim, 2013). Performance can be measured with different problem-solving tests: using knowledge retention (using past knowledge to solve a problem, Sajjadi et al., 2020), recall (the ability to remember items, Wirzberger et al., 2019), or transfer learning (using past knowledge to solve new problems, Makransky et al., 2019). In addition to performance, researchers can also evaluate response times and effort. Effort can be measured by comparing the number of problems solved (that are not necessarily correct) in different problem-solving tests (Krämer et al., 2016). Response times correspond to the duration required to solve a problem (Hayes et al., 2010).
3.3. Users' Self-Evaluations
In learning situations, other more subjective measures than performance are rated using Likert-scale items. These measures include the interest in a task or a domain, (e.g., “I will take a hard sciences course as an elective,” Rosenberg-Kima et al., 2008), beliefs about the utility of a task or a domain (e.g., “I would have many good career opportunities if I was a hard science major,” Plant et al., 2009), learners' self-efficacy as in feeling capable of performing a task (e.g., “I can achieve high grades in math,” Kim and Wei, 2011), learners' self-regulation to regulate their behaviors to succeed in a task (e.g., “I kept track of my progress,” Baylor and Kim, 2004), learners' motivation assessed with the Situational Motivation Scale (SIMS) (Guay et al., 2000) which includes 16 items about the motivation to work on tasks (e.g., “Because I am doing it for my own good,” Krämer et al., 2016), learners' enjoyment (e.g., “How much did you enjoy preparing for the exam?,” Shiban et al., 2015), their perceived learning effectiveness (e.g., “I gained a good understanding of the basic concepts of the materials,” Sajjadi et al., 2020), and their mental demand to know how much mental and perceptual activity was required (thinking, deciding, calculating, etc.), e.g., “Was the task easy or demanding?” (Hart and Staveland, 1988; Pezzullo et al., 2017).
3.4. Evidence of the Persistence of Gender Stereotypes in Human-Machine Interactions
We have summarized the selected studies on users' perceptions of virtual agents depending on their gender in each line of the following table. We stated agents' characteristics, the number of male and female participants with their average age, tasks of the study, the observed measures, and the study's results. Some acronyms are present in this table. We used MA for Male Agent(s) and FA for Female Agent(s). In the same logic, MP is used for Male Participant(s), and FP for Female Participant(s).
The studies presented in Table 1 show that gender stereotypes persist in human-machine interactions. Users' behavior varies according to the gendered appearance of virtual agents. For example, in De Angeli and Brahnam (2006), the female virtual agent received several violent sexual propositions and even rape threats; the male virtual agent received only a few sexual propositions, none of them violent (“gently presses my lips to yours into a small kiss”), and the other sexual comments made during the interactions with the male virtual agent targeted his girlfriend. In a similar study by Brahnam and De Angeli (2012), users interacted with several pairs of female/male agents, including child agents, White agents, Black agents, and “old” agents. The female agents were the target of significantly more sexual discourse, comments on their appearance, and swear words than the male agents; this was even true for the pair of child agents. Other features of agents influenced the conversational topics, such as their age and appearance: users talked more about jobs, achievements, and money with old agents dressed in formal clothing than with any other pair of agents. However, gender stereotypes still applied to this category, since users interacted more with the older male agent about these topics than with the older female agent.
An agent's gender also has a direct influence on participants' decisions. For instance, Lee (2003) reported that users followed more advice from virtual agents when their gender stereotypically matched the topic (e.g., a female agent and cosmetics, a male agent and sports). In this study, the female virtual agent presented as particularly feminine. This result should, thus, be verified in a separate study using a female virtual agent presenting a sport-oriented appearance to determine whether these results are due solely to gender and not to the agents' presentation (clothes and make-up). In Guadagno et al. (2007), the male virtual agent was more persuasive when perceived to be computer-controlled rather than human-controlled. The opposite was true for the female virtual agent. The authors concluded that these results may have been due to gender stereotypes, specifically by the “participants” expectations for interacting with a computer being more consistent with masculine stereotypes (e.g., competent), whereas expectations for interacting with a human are more consistent with feminine stereotypes (e.g., warm)."Not only the gender of a virtual agent but even their perceived masculinity can influence participants' decisions. In a decision-making game where virtual agents made a monetary offer to male participants, the number of offers accepted was higher with the agents perceived as more masculine (Świdrak et al., 2021). The same results were obtained in a similar study for male participants; in contrast, female participants accepted more offers from the agents than male participants but were only influenced by the offer itself, not by the agents' perceived masculinity (Świdrak et al., 2021).
An agent's gender also has an impact on how users perceive the agent in terms of stereotypical traits attributed to men and women (Sczesny et al., 2018). In a study by Nunamaker et al. (2011), the male agent was perceived as more powerful, whereas the female agent was perceived as more likable. Even when male and female agents wore the same clothes, exhibited the same verbal and non-verbal behaviors, and had their faces blurred (thus lacking salient indicators of gender), the female agent was rated higher for warmth than the male agent; however, they were rated similarly for dominance, a trait typically associated with men (Antonio Gómez-Jáuregui et al., 2021). In contrast, Kulms et al. (2011), found in their main experiment that participants did not ascribe more masculine traits to the male agents nor more feminine traits to the female agents, unlike in their pretest with 14 participants using still pictures of the same virtual agents. The authors concluded that stereotyped attributions became less important when participants could interpret the behavior of the agents. However, a study by Ait Challal and Grynszpan (2018) contradicts this conclusion: the female agent was rated as less agreeable than the male agent when using high direct gaze. The authors suggested that participants were less tolerant of dominance when expressed by a female agent. Gender stereotypes associated with users' gender can also impact the ratings of virtual agents. In a study by Mousas et al. (2018), male participants reported feeling more at ease and comfortable with a zombie agent than female participants; they also liked the zombie agent more than the female participants did. The authors concluded that gender stereotypes may have influenced the results because stereotypes call for men to be calmer in the face of fear and embarrassment/disgust and to report milder emotional reactions.
Contexts stereotypically associated with one gender may also have an impact on participants' preferences as to the gender of agents: in two experiments conducted by ter Stal et al. (2020), elderly participants preferred still pictures of female agents in a healthcare context. According to the authors, this result could be due to the task—health coaching—being associated with female gender stereotypes. In addition, male agents were rated as more authoritarian and expert than female agents. In a study by Gulz et al. (2007), when virtual agents presented university programs in computer engineering, participants' interest was higher in feminine and masculine agents as compared to “neutral” agents (a less feminine female agent and a less masculine male agent). However, participants who ranked the less feminine female agent as the best presenter chose her because they believed that she could make more girls interested in computer engineering (“she seems young and nice, and I think she would make more girls interested”); and participants who ranked the feminine female agent as the worst presenter chose her because she was a woman who did not seem to belong in that context (“as I said, a woman feels more welcoming than a man, but she looked so styled, which I don't like”). These results show that gender stereotypes apply to the appearance of female agents.
In addition to context, agents' roles can also influence how users perceive them. When female agents were presented as assistants to elderly people in their daily life, participants found them to be more worth interacting with, more useful, efficient, and well designed, and more captivating, exciting, engaging, and attractive than male agents (Esposito et al., 2021). However, in a similar experiment with silent agents, the agents' gender did not affect the participants in terms of the same criteria (Esposito et al., 2021). Voices could have influenced the perceived agents' masculinity/femininity, but this was not measured in the studies. In a different study, expert agents were rated as more credible than motivational agents regardless of their gender (Kantharaju et al., 2018).
However, a recent study by Nag and Yalçın (2020) contradicts previous research on how humans perceive virtual agents depending on their gender: still pictures of male and female agents were generally rated similarly for agency (traits typically associated with men: ambitious, aggressive, courageous, decisive) and competence (traits typically associated with men: creative, intelligent, innovative, organized), but not for communion (traits typically associated with women: affectionate, compassionate, sensitive, inviting, helpful) where female agents were rated higher. A limitation of this study is that the female and male agents were quite similar in appearance. This being said, the results of the study tend to be coherent with the evolution of gender stereotypes reported by Eagly et al. (2020) for the perception of agency and competence traits perception in interpersonal interactions: the gap in agency and competence in favor of men has reduced. However, the communion traits are still largely attributed to women. This raises the question of whether the evolution in the perception of stereotypes in human-human interactions shown by Eagly et al. (2020) can be observed similarly in human-virtual agent interactions.
Based on the research presented above, it seems that male virtual agents are perceived as more competent, especially regarding stereotypically male-related topics. They appear as better suited to represent a pedagogical virtual tutor in STEM fields (Science, Technology, Engineering, and Mathematics) since these fields are perceived as masculine (Makarova et al., 2019). In the next section, we focus more specifically on research on pedagogical agents and the impact of their gender on users' academic outcomes.
3.5. The Effect of Virtual Agents' Gender on Academic Outcomes
We have summarized the selected studies on the impact of gendered virtual agents in the context of a learning task in each line of the following table. We stated agents' characteristics, the number of male and female participants with their average age, tasks of the study, the observed measures, and the study's results. Some acronyms are present in this table. We used MA for Male Agent(s) and FA for Female Agent(s). In the same logic, MP is used for Male Participant(s), and FP for Female Participant(s).
Various studies on virtual learning environments (Table 2) have reported that the gender of a pedagogical agent may have an impact on the learning performance of users. In a recent article, Makransky et al. (2019) showed that young girls performed better on scientific tasks (in terms of learning and transfer learning) when taught by a virtual female scientist than by a virtual drone. The opposite was true for boys. The researchers argued that boys identified with the drone, while girls identified with the female agent. However, research opposing human-like vs. robot-like agents does not take into account other factors that may influence how girls learn. In a study by Shiban et al. (2015), female learners were more motivated and interested in math when trained by a female agent as compared to a male agent. However, they obtained better results with the male agent, which may be explained by their perception of the agents' appearances: the male agent was older and wore a tie, while the female agent was young and pretty. According to the authors, the participants' performance improved because the male agent was perceived as an expert, and virtual agents perceived as experts have been shown to improve learners' performance (Baylor and Kim, 2004). The researchers also concluded that the female participants' motivation and interest improved with the female agent because there were more female participants in the study and because of the agent's similarity (in age and gender) to them, in line with the “similarity hypothesis” (also found in Rosenberg-Kima et al. 2008). This argument is supported by Bandura's social cognitive learning theory: people often learn by imitating people whom they perceive as similar (or superior: higher in rank or status) to them and who are, therefore, accepted as social role models (Bandura and National Inst of Mental Health, 1986). This theory also bears out in a study by Plant et al. (2009), where a female agent raised participants' self-efficacy by delivering a message on the benefits of engineering, resulting in better performance and more interest in math.
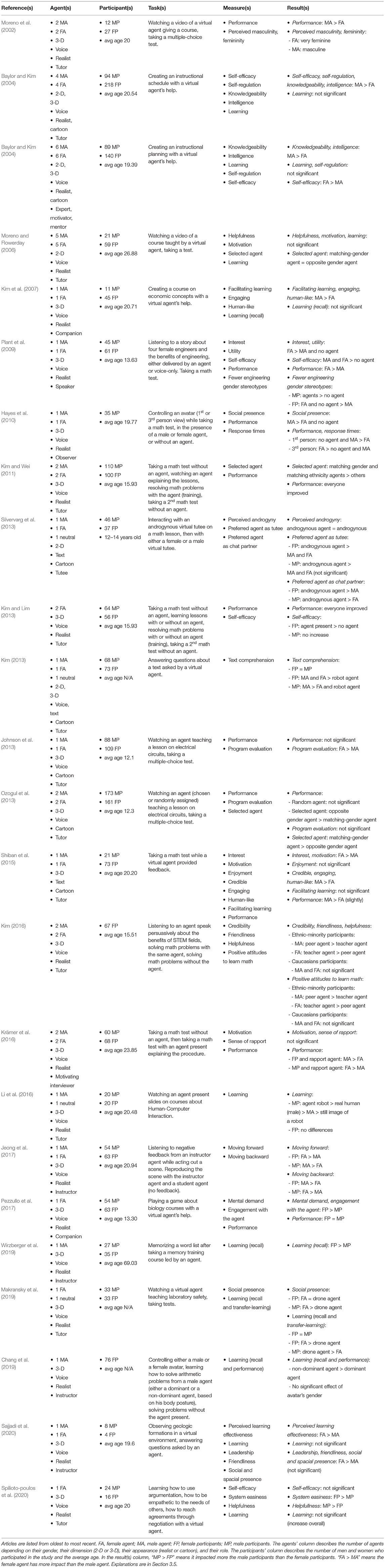
Table 2. Summary of research studies on the impact of gendered virtual agents in the context of a learning task.
However, other research has demonstrated a positive effect of male agents as compared to female agents in pedagogical tasks. For instance, in two experiments conducted by Baylor and Kim (2004), a virtual agent helped participants create a schedule. The agent's gender did not impact learning but did affect self-efficacy, which increased more in the first experiment with the male agent than the female agent; the contrary occurred in the second experiment for both male and female participants. The researchers suggested that there was a bias in the first experiment, as participants rated the male agent as more interesting and useful than the female agent. In the second experiment, participants viewed the female agent as less expert and knowledgeable than the male agent, despite receiving the same instructions from both agents; some research has indicated that agents perceived as less intelligent could lead to greater self-efficacy (Baylor and Kim, 2005). In a similar experiment by Kim et al. (2007), the researchers introduced a female and a male pedagogical agent to help students design an e-learning course, which included creating a schedule. Students working with the male agent rated him higher on facilitating learning, being engaging, and being human-like than students working with the female agent. Notably, the male agent had a more positive impact than the female agent on the participants' interest and learning in terms of recall (the ability to remember what the agent said during the task).
Other factors may also come into play in studies on the impact of virtual agents on learning. In Moreno et al. (2002), participants watched a video of a virtual agent presenting a course on blood pressure, followed by a multiple-choice test. The results of this study suggest that the participants learned more from the male agent than from the female one. The researchers suggested that this might be because the female tutor did not conform to the stereotype of men as teachers. The first study showed that participants in this experiment perceived the female agent as very feminine, while the male agent was found to be very masculine. This may be due to a difference in the participants' interpretation of the female agent as being “too feminine” to be suitable for the role of tutor. The study did not address how participants perceived the agents' expertise or seek to determine any possible interactions between perceived expertise, the perceived agent's femininity, and performance on the test. Gender, while important, must be taken into account in combination with other features. For instance, Krämer et al. (2016) analyzed the impact of pedagogical agents' gender and their behavior on adults' motivation, effort, and performance in math. They found that the simple presence of a female virtual agent in a learning situation did not increase women's motivation and learning. However, when the agent displayed human-like non-verbal behavior by aligning with the participants' non-verbal behavior (Gratch et al., 2007), the participants' performance and effort improved. This kind of behavior, called rapport, is defined in social psychology as the establishment of a positive relationship between interactants by way of a positive attitude (e.g., acquiescence, smiles), mutual attention (e.g., mutual gaze), and coordination of behaviors (e.g., synchrony, mimicry) (Tickle-Degnen and Rosenthal, 1990). This research shows the importance of the pedagogical agents' behavior combined with their gender as providing a positive impact on academic outcomes. Agents' behavior is especially important as it could negatively impact learners' academic outcomes, as shown in an experiment by Chang et al. (2019) where a male “dominant” pedagogical agent impaired female participants' performance and recall in arithmetic problems, compared to a male “non-dominant” agent.
The research presented above highlights the importance of pedagogical agents' gender on learning. Different studies appear to yield contradictory results, on one hand, that learning improves when virtual agents' gender matches the learner's, but on the other hand that male virtual agents could be better suited to improving learning. Interestingly, Section 3.4 shows that male agents are perceived as more competent than female agents, and users follow more advice from a male agent than a female one on topics stereotypically perceived as masculine. However, the studies featuring a female agent in STEM fields (Science, Technology, Engineering, and Mathematics) presented in this section show that female agents have a positive influence on academic outcomes: they improve learning, self-efficacy, interest, and motivation, despite the fact that STEMs are perceived as masculine (Makarova et al., 2019).
4. Discussion
4.1. The Question of Pedagogical Agents' Gender
Based on the research presented above, one could surmise that, in general, male pedagogical agents are better suited to improving academic outcomes than female agents. However, systematically relying on male pedagogical agents could have an adverse impact: for instance, designing only male agents for learning purposes in STEM fields could strengthen gender stereotypes. As highlighted by West et al. (2019), the gender bias of interactive systems not only perpetuates stereotypes but also reinforces and extends them. The stereotypes modeled through interactive systems generate behaviors that go beyond the sphere of the virtual environment by conveying a harmful image of women. For instance, in a study by Dill et al. (2008), still pictures of men and women in suits or male and female characters acting in highly stereotypical ways were shown to participants. Male participants exposed to negative female stereotypes were significantly more tolerant of a real-life instance of sexual harassment and exhibited greater rape myth acceptance. As for representation in STEM fields, as noted by Sansone (2019), the lack of female role models can lead female students to believe that men are better than women in STEM fields. The lack of virtual female role models in virtual learning environments may have the same impact. Accordingly, more STEM experts represented with virtual female characters could help decrease gender stereotypes in STEM fields.
Some research has explored the use of androgynous virtual agents to counter gender stereotypes. In earlier studies, participants tended to apply the labels of “man” or “woman” to androgynous agents. For instance, in Niculescu et al. (2009), participants classified androgynous virtual characters as male or female, depending on the participants' gender and other parameters such as which virtual characters they had seen before. Even for genderless agents such as a wooden mannequin, the participants perceived their gender depending on how they perceived their walking motions (McDonnell et al., 2009). Recent research has shown more promising results in terms of gender stereotypes. In Nag and Yalçın (2020), results for androgynous agents show a linear trend that positions their scores for the perceived agency, communion, and competence in between those for female and male agents. The authors, thus, believe that androgynous agents could help mitigate male and female stereotypes. Although participants in their first experiment tended to believe that the androgynous virtual agents were men, when the authors modified the agents in question for their main experiment, participants correctly perceived them as androgynous after reading a definition of an androgynous agent.
What about androgynous pedagogical agents in an educational context? Silvervarg et al. (2013) supposed, but with caution, that students could identify with an androgynous agent by ascribing their own gender to them, thus making them a suitable role model. Indeed, in their experiment with children aged 12-14, participants perceived an androgynous pedagogical agent as not clearly a boy nor clearly a girl, but they generally assigned themselves a gender to their androgynous virtual tutee, boy or girl. The authors supposed students could, therefore, have more freedom to construct and ascribe gender, as their pedagogical agent's gender choice is personal rather than imposed. They also supposed androgynous agents could diminish gender stereotypes, as their appearances are genderless. Applying our own gender to an androgynous agent to make them a suitable role model is an interesting hypothesis. However, we do not know what the gender participants applied to the androgynous agent or why. More research on androgynous agents has to be done in an educational context to help determine, e.g., whether androgynous agents are perceived as masculine, feminine, neutral, man, woman, or genderless depending on the context and the role of the agent. Since STEM fields are considered masculine fields (Makarova et al., 2019), participants could perceive an androgynous agent as a man, even though they could perceive them as not clearly a boy nor a girl in terms of appearance. This could reinforce the stereotype of STEM fields as more suitable for men than women. Agents' role is also particularly important, as Brahnam and Weaver (2015) stated there are more female assistant agents than male ones. They showed the example of a webpage that provides virtual agents, four of the five virtual agents are female and they assist people at airports or serve as talking mannequins for fashion and museum exhibits. The male agent was called a “virtual doctor” and provided health tips and hospital information. We can emit the hypothesis that one could perceive androgynous virtual assistants as women, hence reinforcing gender stereotypes. For this research on androgynous virtual agents, we recommend measuring how participants feel toward the androgynous agents, as not being able to perceive someone as a man or a woman may induce insecurity and unease in some people (Nass and Brave, 2005).
4.2. Virtual Agents' as Social Role Models in Learning Environments
Some research, though still very limited, has explored the use of virtual agents to increase learners' performance and interest in mathematics. For example, Rosenberg-Kima et al. (2008) showed the effectiveness of a female virtual agent engineer in interesting women in STEM fields. In a video, the agent, who was similar in gender to the participants (who were all women), presented a story about successful female role models in STEM fields. This led to a change in participants' attitudes toward science, as shown with a 7-point scale questionnaire. Women in the female virtual agent condition were less likely to endorse traditional STEM stereotypes than those in the male virtual agent condition and were more likely to believe that women could succeed in STEM fields. Gender stereotypes still persisted: the participants were slightly more likely to believe in STEM usefulness with a male virtual agent engineer. In a similar study by Plant et al. (2009), male and female participants performed better and were more interested in engineering after interacting with a female agent, as their self-efficacy and their ratings about STEM usefulness improved. Interestingly, male participants were less likely to endorse traditional STEM stereotypes in the presence of an agent, male or female; but female participants were less likely to endorse traditional STEM stereotypes with a female agent or without any agent, than with a male one. Another similar study by Rosenberg-Kima et al. (2010) showed that Black virtual agents had a more positive impact on STEM interest and STEM gender stereotypes for Black women, whereas female virtual agents (Black or White) had a more positive impact on White women on the same criteria. This research shows the importance of other factors, such as virtual agents' ethnic background, performance, and interest in math.
Finally, several studies have shown that pedagogical agents used as learning companions can simulate social interactions (Kim and Baylor, 2006) and the potential impact of a virtual agent's gender on education. However, only few studies have explored the use of a virtual pedagogical companion to counteract the effects of Stereotype Threat (refer to Introduction). Research on Social Cognition has shown the positive impact of social role models to counteract ST effects (Bagès et al., 2016). Studies have shown that female participants do not immediately see female scientists as potential role models simply by interacting with them; they begin to perceive female scientists as role models when they establish personal connections with them (Buck et al., 2008). In the field of virtual agents, virtual rapport has been studied as a means to create this type of relationship between virtual agents and users (Gratch et al., 2007). As reported by Krämer et al. (2016), the mere presence of a female agent did not improve participants' performance and effort. However, when agents were able to create a virtual rapport, participants' performance and effort were shown to improve.
Based on the research presented above, not only is the gender of pedagogical agents important, but so is their behavior (Krämer et al., 2016; Chang et al., 2019), their role (Baylor and Kim, 2004; Kim, 2016), and their ethnicity (Rosenberg-Kima et al., 2010; Kim, 2016). Girls may see a female pedagogical agent as a role model who influences their motivation to exert effort to learn (Shiban et al., 2015). A study by Pfeifer and Lugrin (2018) shows that female social robots can be role models to female students: female students learned better with a female robot in a stereotypically masculine domain. Virtual characters can be used to embody social models and, thus, change the learner's attitudes and motivation; as described earlier, a female role model who succeeds in math can reduce Stereotype Threat effects. Combining research on social cognition and virtual agents, we recommend counteracting Stereotype Threat effects for girls and women in math by using a virtual agent representing a hardworking female social role model (Bagès et al., 2016) able to establish rapport with the learners (Gratch et al., 2007), of similar ethnicity to the learners (Kim, 2016) and slightly older than them (Bagès and Martinot, 2011). When the role model is younger or the same age as the learners, they can lose motivation by feeling unable to match their role model's achievements; if the model is too old, they will not identify with them. A pedagogical agent should, thus, embody the role of a knowledgeable and motivational person; this has been demonstrated by student preferences and by the proven positive impact these types of agents have on education (Kim and Baylor, 2016).
4.3. Improved Learning or Better Inclusion?
An ethical tension between two competing goals arises in all domains: skill learning (where the research presented above favors the use of a male virtual agent), vs. better inclusion of girls and women (via the use of a female virtual character embodying a successful role model in the domain). Prior research is not robust enough to prove the superiority of a male agent in all fields and for all audiences. Some questions remain unanswered in the literature, to our knowledge: Would using the same androgynous character but presented as male, female, or neutral by the experimenter have an impact on academic outcomes in scientific or other domains? What would be the impact of systematically using a virtual agent of the same gender as the learners?
Regarding the second question, using only successful male models in mathematics with boys could reinforce gender stereotypes. Women are aware of the negative stereotype about their mathematical skills that create a hostile environment for them. Research by Stokes et al. (1995) reported that when women find a friendly environment, they are more likely to stay employed. One solution to reconciling the two goals, at least in STEM fields, would be to use successful female role models to explain how they managed to perform well: in Bagès et al. (2016), students took a math test after reading a story about a social model, female or male depending on the condition. The stories differentiated between models: the hardworking model put in the effort and spent time learning his or her lessons to perform well, the gifted model was naturally good at math, and the neutral model gave no explanation for his or her success. Girls' performance increased with the hardworking model: they performed at the same level as boys, whether the model was a boy or a girl. There was no impact on boys' performance. In contrast, in a similar study, boys' performance also increased with a hardworking model, regardless of gender (Bagès and Martinot, 2011). Furthermore, when the role model did not explain his or her success in math, both girls' and boys' scores improved with a female role model. As the lack of female role models may lead female students to believe that men are better than women in STEM fields Sansone (2019), it could be interesting to combine the results of (Bagès et al., 2016) and our hypothesis that a successful virtual female role model in STEM fields could help mitigate gender stereotypes. Female virtual agents who act as successful social models in mathematics and explain how they succeeded through their effort and hard work may be a potential solution to counteracting Stereotype Threat effects. Another question then arises: in the long term, what would be the impact of presenting only these kinds of female virtual agents to boys?
5. Conclusion
In this article we have presented a systematic review of research on perceptive studies of virtual agents depending on their gender, regardless of the application domain; and on the impact of gendered virtual agents in the context of a learning task. Each study has been performed in a specific learning context with specific pedagogy, design of the virtual environment, duration of the interaction, modality of interaction, physical environment, etc. These elements of context may have an impact on the users' perceptions and learning outcomes. The limitation of this article is that we have not considered all these contextual specificities. Further analysis could take into account these contextual elements to provide a more global view of the impact of virtual agents' gender in academic learning. Nevertheless, the present systematic review enables us to draw some conclusions by answering each question stated in Section 2.2.
Do Students Perceive Virtual Agents' Differently Depending on Their Own Gender and the Gender of the Agent?
As individuals communicate with virtual agents by applying social rules and expectations as social beings (Nass and Moon, 2000), it is not surprising that they also apply gender stereotypes to virtual agents and their interactions with them (refer to Section 3.4). Female virtual agents are usually seen as less expert, less knowledgeable, and less powerful than male virtual agents (Baylor and Kim, 2004; Nunamaker et al., 2011), and they are also usually perceived as more likable and attractive than male virtual agents (Nunamaker et al., 2011; Lunardo et al., 2016). Those perception differences can even affect people's decisions (Lee, 2003; Świdrak et al., 2021).
Given the empirical results, we propose to respond simultaneously to question 2 (Does the gender of pedagogical agents influence students' academic performance and self-evaluations?) and question 3 (Are there tasks or academic situations to which a male virtual agent is better suited to than a female virtual agent, and vice versa, according to empirical evidence?). The review conducted in this article could lead to the belief that male pedagogical agents are better suited than female agents to improve academic outcomes, especially in male-dominated scientific fields like STEM fields (Makarova et al., 2019). However, research also shows that female pedagogical agents can improve learners' performances in these fields (Rosenberg-Kima et al., 2008; Plant et al., 2009). Some studies have shown that using female virtual agents as social role models can increase female participants' self-efficacy (Rosenberg-Kima et al., 2008), and both male and female participants' interest (Plant et al., 2009; Rosenberg-Kima et al., 2010), and performances (Plant et al., 2009). These results are especially relevant to addressing Stereotype Threat effects, a phenomenon that illustrates how and why female students' performance in math can be impaired by gender stereotypes (Spencer et al., 1999). Previous research showed that stereotype threat effects can be counteracted by introducing a female positive role model who looks like a learner so that they can identify with her (Bagès et al., 2016). Such a positive role model could be embodied by a virtual pedagogical agent (Rosenberg-Kima et al., 2008), and used to reduce stereotype threats in STEM fields.
How Do a Virtual Agent's Pedagogical Roles Impact These Results?
Individuals tend to listen more to agents whose gender stereotypically matches the context or gender roles such as female virtual agents with cosmetics and male virtual agents with sports (Lee, 2003), female virtual agents in contexts involving social influence (Khashe et al., 2017), and female virtual agents in an assistant role (Esposito et al., 2021). The role of a virtual pedagogical agent has been studied in the academic context, using expert, motivator, and mentor agents (Baylor and Kim, 2004). Expert agents are older than students, authoritative, strictly informative, and knowledgeable. Motivator agents are enthusiastic and not seen as particularly knowledgeable, they are mostly used to elicit motivation. As for mentor agents, they are slightly older than students, are knowledgeable, and are also used to elicit motivation. They are a mix of expert agents and motivator agents (Baylor and Kim, 2005). Researchers should take agents' roles into account when designing a pedagogical agent, as a female pedagogical agent designing as a mentor agent can improve learners' performance (Plant et al., 2009), but a female pedagogical agent designing as a motivator agent may not be effective on learners' performance (Shiban et al., 2015). In conclusion, the impact of the virtual agent's gender depends on several aspects related to the role of the agent. This role is indeed reflected through for instance the appearance but also the discourse of the agent.
How Do a Virtual Agent'S Appearance and Interactive Capacities Impact These Results?
The topics of individuals' interactions with virtual agents differ depending on their gendered appearance: in studies by De Angeli and Brahnam (2006) and Brahnam and De Angeli (2012), female virtual agents received significantly more violent sexual propositions, more rape threats, more comments on their appearance, and more swear words compared to male virtual agents who received few sexual propositions, most of them targeting their girlfriends. Moreover, the degree of perceived masculinity and femininity can influence men's decisions, as shown in Świdrak et al. (2021) where male participants were persuaded more by masculine agents than feminine agents, regardless of the agents' gender. Agents' appearance can influence their perceived role, as an old agent wearing a tie could be perceived more as an expert than a young agent (Shiban et al., 2015). As seen in the question above, the agents' role is important in academic situations. In addition to agents' roles and appearance, research has shown the importance of a positive relationship between learners and pedagogical agents (Krämer et al., 2016). This research tends to show that a female social model embodied by a pedagogical agent able to establish a positive relationship with learners may counteract Stereotype Threat effects and, thus, improve women's performance, interest, and self-efficacy in mathematics.
Are Androgynous Virtual Agents' a Potential Solution to Combatting Gender Stereotypes?
This question is quite difficult to answer as to our knowledge, few studies have explored the use of androgynous virtual agents to counter gender stereotypes in the academic context. Silvervarg et al. (2013) used an androgynous pedagogical agent in an educational context and showed participants, although evaluating the agent as “not clearly a boy nor a girl,” tend to ascribe a binary gender (boy or girl) to the agent. The authors, thus, cautiously supposed that students could ascribe their own gender to an androgynous agent, thus giving them more freedom and making the agent a suitable role model, known to be beneficial for academic outcomes. However, the results did not show what gender participants ascribe to the agent, nor why. The way individuals ascribe gender to an androgynous or a genderless agent should be studied more. In particular, does this gender attribution depend on the context or agents' role? Since STEM fields are considered masculine fields (Makarova et al., 2019), participants could think androgynous agents are men. This could reinforce the stereotype of STEM fields as more suitable for men than women. As for agents' roles, there are more female virtual assistants than male ones (Brahnam and Weaver, 2015). Some developers admitted female virtual assistants are usually used because they evoke gender stereotypes: women are expected to serve, help, and nurture others. Androgynous virtual assistants could then be considered women, and reinforce harmful stereotypes about women. Researchers and developers who want to use androgynous agents to combat gender stereotypes should be very careful, as the opposite effect can occur.
Data Availability Statement
The original contributions presented in the study are included in the article/supplementary material, further inquiries can be directed to the corresponding author/s.
Author Contributions
MA wrote the article. MO and IR supervised the article and corrected it. All authors contributed to the article and approved the submitted version.
Funding
This work was carried out within the pilot center Ampiric, funded by the French State's Future Investment Program (PIA3/France 2030) as part of the “Territories of Educational Innovation” action.
Conflict of Interest
The authors declare that the research was conducted in the absence of any commercial or financial relationships that could be construed as a potential conflict of interest.
Publisher's Note
All claims expressed in this article are solely those of the authors and do not necessarily represent those of their affiliated organizations, or those of the publisher, the editors and the reviewers. Any product that may be evaluated in this article, or claim that may be made by its manufacturer, is not guaranteed or endorsed by the publisher.
References
Ait Challal, T., and Grynszpan, O. (2018). “What gaze tells us about personality,” in Proceedings of the 6th International Conference on Human-Agent Interaction (Southampton), 129–137.
Akbar, F., Grover, T., Mark, G., and Zhou, M. X. (2018). “The effects of virtual agents' characteristics on user impressions and language use, in Proceedings of the 23rd International Conference on Intelligent User Interfaces Companion (Tokyo), 1–2.
Antonio Gómez-Jáuregui, D., Giraud, T., Isableu, B., and Martin, J.-C. (2021). Design and evaluation of postural interactions between users and a listening virtual agent during a simulated job interview. Comput. Animat. Virtual Worlds 22, e2029. doi: 10.1002/cav.2029
Astrid, M., Krämer, N. C., and Gratch, J. (2010). “How our personality shapes our interactions with virtual characters-implications for research and development,” in International Conference on Intelligent Virtual Agents (Philadelphia, PA: Springer), 208–221.
Bagès, C., and Martinot, D. (2011). What is the best model for girls and boys faced with a standardized mathematics evaluation situation: a hardworking role model or a gifted role model? Br. J. Soc. Psychol. 50, 536–543. doi: 10.1111/j.2044-8309.2010.02017.x
Bagès, C., Verniers, C., and Martinot, D. (2016). Virtues of a hardworking role model to improve girls” mathematics performance. Psychol. Women Q. 40, 55–64. doi: 10.1177/0361684315608842
Bailenson, J. N., Blascovich, J., Beall, A. C., and Loomis, J. M. (2001). Equilibrium theory revisited: Mutual gaze and personal space in virtual environments. Presence 10, 583–598. doi: 10.1162/105474601753272844
Bandura, A., and National Inst of Mental Health. (1986). Social Foundations of Thought and Action: A Social Cognitive Theory. Princeton, NJ: Prentice-Hall, Inc.
Baylor, A. L., and Kim, Y. (2004). “Pedagogical agent design: The impact of agent realism, gender, ethnicity, and instructional role,” in 7th International Conference in Intelligent Tutoring Systems (Maceió-Alagoas).
Baylor, A. L., and Kim, Y. (2005). Simulating instructional roles through pedagogical agents. Int. J. Artif. Intell. Educ. 15, 95.
Bem, S. L. (1974). The measurement of psychological androgyny. J. Consult. Clin. Psychol. 42, 155. doi: 10.1037/h0036215
Biocca, F. (1997). The cyborg's dilemma: progressive embodiment in virtual environments. J. Comput. Mediat. Commun. 3, JCMC324. doi: 10.1111/j.1083-6101.1997.tb00070.x
Biocca, F., Harms, C., and Burgoon, J. K. (2003). Toward a more robust theory and measure of social presence: review and suggested criteria. Presence 12, 456–480. doi: 10.1162/105474603322761270
Biocca, F., Harms, C., and Gregg, J. (2001). “The networked minds measure of social presence: pilot test of the factor structure and concurrent validity,” in 4th Annual International Workshop on Presence (Philadelphia, PA), 1–9.
Brahnam, S., and De Angeli, A. (2012). Gender affordances of conversational agents. Interact. Comput. 24, 139–153. doi: 10.1016/j.intcom.2012.05.001
Brahnam, S., and Weaver, M. (2015). “Re/framing virtual conversational partners: a feminist critique and tentative move towards a new design paradigm,” in International Conference of Design, User Experience, and Usability (Los Angeles, CA: Springer), 172–183.
Buck, G. A., Clark, V. L. P., Leslie-Pelecky, D., Lu, Y., and Cerda-Lizarraga, P. (2008). Examining the cognitive processes used by adolescent girls and women scientists in identifying science role models: a feminist approach. Sci. Educ. 92, 688–707. doi: 10.1002/sce.20257
Chang, F., Luo, M., Walton, G., Aguilar, L., and Bailenson, J. (2019). Stereotype threat in virtual learning environments: effects of avatar gender and sexist behavior on women's math learning outcomes. Cyberpsychol. Behav. Soc. Network. 22, 634–640. doi: 10.1089/cyber.2019.0106
Cloud-Buckner, J., Sellick, M., Sainathuni, B., Yang, B., and Gallimore, J. (2009). “Expression of personality through avatars: analysis of effects of gender and race on perceptions of personality,” in International Conference on Human-Computer Interaction (Uppsala: Springer), 248–256.
De Angeli, A., and Brahnam, S. (2006). “Sex stereotypes and conversational agents,” in Proceedings of Gender and Interaction: Real and Virtual Women in a Male World (Venice).
Dill, K. E., Brown, B. P., and Collins, M. A. (2008). Effects of exposure to sex-stereotyped video game characters on tolerance of sexual harassment. J. Exp. Soc. Psychol. 44, 1402–1408. doi: 10.1016/j.jesp.2008.06.002
Eagly, A. H., Nater, C., Miller, D. I., Kaufmann, M., and Sczesny, S. (2020). Gender stereotypes have changed: a cross-temporal meta-analysis of US public opinion polls from 1946 to 2018. Am. Psychol. 75, 301. doi: 10.1037/amp0000494
Esposito, A., Amorese, T., Cuciniello, M., Riviello, M. T., Esposito, A. M., Troncone, A., et al. (2021). Elder user's attitude toward assistive virtual agents: the role of voice and gender. J. Ambient. Intell. Humaniz Comput. 12, 4429–4436. doi: 10.1007/s12652-019-01423-x
Feng, D., Jeong, D. C., Krämer, N. C., Miller, L. C., and Marsella, S. (2017). “is it just me?”: evaluating attribution of negative feedback as a function of virtual instructor's gender and proxemics,” in Proceedings of the 16th Conference on Autonomous Agents and MultiAgent System (São Paulo), 810–818.
Forgasz, H. J., and Leder, G. C. (1996). Mathematics classrooms, gender and affect. Math. Educ. Res. J. 8, 153–173. doi: 10.1007/BF03217295
Fox, J., and Bailenson, J. N. (2009). Virtual virgins and vamps: the effects of exposure to female characters” sexualized appearance and gaze in an immersive virtual environment. Sex Roles 61, 147–157. doi: 10.1007/s11199-009-9599-3
Gratch, J., Wang, N., Gerten, J., Fast, E., and Duffy, R. (2007). “Creating rapport with virtual agents,” in International Workshop on Intelligent Virtual Agents (Stockholm: Springer), 125–138.
Guadagno, R. E., Blascovich, J., Bailenson, J. N., and McCall, C. (2007). Virtual humans and persuasion: the effects of agency and behavioral realism. Media Psychol. 10, 1–22. doi: 10.108/15213260701300865
Guay, F., Vallerand, R. J., and Blanchard, C. (2000). On the assessment of situational intrinsic and extrinsic motivation: the Situational Motivation Scale (SIMS). Motiv. Emot. 24, 175–213. doi: 10.1023/A:1005614228250
Gulz, A., Haake, M., and Tärning, B. (2007). Visual gender and its motivational and cognitive effects-a user study. Lund Univers. Cogn. Stud. 137, 928–935.
Hart, S. G., and Staveland, L. E. (1988). Development of NASA-TLX (Task Load Index): results of empirical and theoretical research. Adv. Psychol. 52, 139–183. doi: 10.1016/S0166-4115(08)62386-9
Hayes, A. L., Ulinski, A. C., and Hodges, L. F. (2010). “That avatar is looking at me! Social inhibition in virtual worlds,” in International Conference on Intelligent Virtual Agents (Philadelphia, PA: Springer), 454–467.
Jeong, D. C., Feng, D., Krämer, N. C., Miller, L. C., and Marsella, S. (2017). “Negative feedback in your face: examining the effects of proxemics and gender on learning,” in International Conference on Intelligent Virtual Agents (Stockholm: Springer), 170–183.
Johnson, A. M., Ozogul, G., Moreno, R., and Reisslein, M. (2013). Pedagogical agent signaling of multiple visual engineering representations: the case of the young female agent. J. Eng. Educ. 102, 319–337. doi: 10.1002/jee.20009
Kantharaju, R. B., De Franco, D., Pease, A., and Pelachaud, C. (2018). “Is two better than one? Effects of multiple agents on user persuasion,” in Proceedings of the 18th International Conference on Intelligent Virtual Agents (Sydney, NSW), 255–262.
Khashe, S., Lucas, G., Becerik-Gerber, B., and Gratch, J. (2017). Buildings with persona: towards effective building-occupant communication. Comput. Hum. Behav. 75, 607–618. doi: 10.1016/j.chb.2017.05.040
Kim, Y. (2013). Digital peers to help children's text comprehension and perceptions. J. Educ. Technol. Soc. 16, 59–70.
Kim, Y. (2016). The role of agent age and gender for middle-grade girls. Comput. Sch. 33, 59–70. doi: 10.1080/07380569.2016.1143753
Kim, Y., and Baylor, A. L. (2006). A social-cognitive framework for pedagogical agents as learning companions. Educ. Technol. Res. Dev. 54, 569–596. doi: 10.1007/s11423-006-0637-3
Kim, Y., and Baylor, A. L. (2016). Research-based design of pedagogical agent roles: A review, progress, and recommendations. Int. J. Artif. Intell. Educ. 26, 160–169. doi: 10.1007/s40593-015-0055-y
Kim, Y., Baylor, A. L., and Shen, E. (2007). Pedagogical agents as learning companions: the impact of agent emotion and gender. J. Comput. Assist. Learn. 23, 220–234. doi: 10.1111/j.1365-2729.2006.00210.x
Kim, Y., and Lim, J. H. (2013). Gendered socialization with an embodied agent: creating a social and affable mathematics learning environment for middle-grade females. J. Educ. Psychol. 105, 1164. doi: 10.1037/a0031027
Kim, Y., and Wei, Q. (2011). The impact of learner attributes and learner choice in an agent-based environment. Comput. Educ. 56, 505–514. doi: 10.1016/j.compedu.2010.09.016
Krämer, N. C., Karacora, B., Lucas, G., Dehghani, M., Rüther, G., and Gratch, J. (2016). Closing the gender gap in with friendly male instructors? On the effects of rapport behavior and gender of a virtual agent in an instructional interaction. Comput. Educ. 99, 1–13. doi: 10.1016/j.compedu.2016.04.002
Kulms, P., Krämer, N. C., Gratch, J., and Kang, S.-H. (2011). “It's in their eyes: a study on female and male virtual humans' gaze,” in International Workshop on Intelligent Virtual Agents (Reykjavik: Springer), 80–92.
Lee, E.-J. (2003). Effects of “gender” of the computer on informational social influence: the moderating role of task type. Int. J. Hum. Comput. Stud. 58, 347–362. doi: 10.1016/S1071-5819(03)00009-0
Li, J., Kizilcec, R., Bailenson, J., and Ju, W. (2016). Social robots and virtual agents as lecturers for video instruction. Comput. Hum. Behav. 55, 1222–1230. doi: 10.1016/j.chb.2015.04.005
Lindberg, S. M., Hyde, J., Petersen, J., and Linn, M. (2010). Gender similarities characterize math performance. Psychol. Bull. 136:1123–1135. doi: 10.1037/a0021276
Lunardo, R.Bressolles, G., et al. (2016). The interacting effect of virtual agents' gender and dressing style on attractiveness and subsequent consumer online behavior. J. Retail. Consum. Serv. 30, 59–66. doi: 10.1016/j.jretconser.2016.01.006
Makarova, E., Aeschlimann, B., and Herzog, W. (2019). The gender gap in fields: the impact of the gender stereotype of math and science on secondary students' career aspirations. Front. Educ. 4, 60. doi: 10.3389/feduc.2019.00060
Makransky, G., Wismer, P., and Mayer, R. E. (2019). A gender matching effect in learning with pedagogical agents in an immersive virtual reality science simulation. J. Comput. Assist. Learn. 35, 349–358. doi: 10.1111/jcal.12335
McDonnell, R., Jörg, S., Hodgins, J. K., Newell, F., and O'Sullivan, C. (2009). Evaluating the effect of motion and body shape on the perceived sex of virtual characters. ACM Trans. Appl. Percept. 5, 1–14. doi: 10.1145/1462048.1462051
Mell, J., Lucas, G., and Gratch, J. (2017). “Prestige questions, online agents, and gender-driven differences in disclosure,” in International Conference on Intelligent Virtual Agents (Stockholm: Springer), 273–282.
Mohtadi, M. T., Hajami, A., and Allali, H. (2014). “Pedagogical agent for metacognitive scaffolding in interactive learning environments,” in 2014 International Conference on Multimedia Computing and Systems (ICMCS) (Marrakech: IEEE), 652–656.
Moreno, K. N., Person, N. K., Adcock, A. B., Eck, R., Jackson, G. T., and Marineau, J. C. (2002). “Etiquette and efficacy in animated pedagogical agents: the role of stereotypes,” in AAAI Symposium on Personalized Agents (Cape Cod, MA).
Moreno, R., and Flowerday, T. (2006). Students' choice of animated pedagogical agents in science learning: a test of the similarity-attraction hypothesis on gender and ethnicity. Contemp Educ. Psychol. 31, 186–207. doi: 10.1016/j.cedpsych.2005.05.002
Mousas, C., Anastasiou, D., and Spantidi, O. (2018). The effects of appearance and motion of virtual characters on emotional reactivity. Comput. Hum. Behav. 86, 99–108. doi: 10.1016/j.chb.2018.04.036
Nag, P., and Yalçın, Ö. N. (2020). “Gender stereotypes in virtual agents,” in Proceedings of the 20th ACM International Conference on Intelligent Virtual Agents (Glasgow), 1–8.
Nass, C., and Moon, Y. (2000). Machines and mindlessness: social responses to computers. J. Soc. Issues 56, 81–103. doi: 10.1111/0022-4537.00153
Nass, C. I., and Brave, S. (2005). Wired for Speech: How Voice Activates and Advances the Human-Computer Relationship. Cambridge, MA: MIT Press.
Niculescu, A., Hofs, D., Van Dijk, B., and Nijholt, A. (2010). “How the agent's gender influence users' evaluation of a qa sy,” in 2010 International Conference on User Science and Engineering (i-USEr) (Shah Alam, Selangor: IEEE), 16–20.
Niculescu, A., Van Der Sluis, F., and Nijholt, A. (2009). “Feminity, masculinity and androgyny: how humans perceive the gender of anthropomorphic agents,” in Proceedings of 13th International Conference on Human-Computer Interaction (Heidelberg: Springer Verlag), 628–632.
Nunamaker, J. F., Derrick, D. C., Elkins, A. C., Burgoon, J. K., and Patton, M. W. (2011). Embodied conversational agent-based kiosk for automated interviewing. J. Manag. Inf. Syst. 28, 17–48. doi: 10.2753/MIS0742-1222280102
Ozogul, G., Johnson, A. M., Atkinson, R. K., and Reisslein, M. (2013). Investigating the impact of pedagogical agent gender matching and learner choice on learning outcomes and perceptions. Comput. Educ. 67, 36–50. doi: 10.1016/j.compedu.2013.02.006
Pansu, P., Régner, I., Max, S., Colé, P., Nezlek, J. B., and Huguet, P. (2016). A burden for the boys: evidence of stereotype threat in boys' reading performance. J. Exp. Soc. Psychol. 65:26–30. doi: 10.1016/j.jesp.2016.02.008
Payne, J., Szymkowiak, A., Robertson, P., and Johnson, G. (2013). “Gendering the machine: Preferred virtual assistant gender and realism in self-service,” in International Workshop on Intelligent Virtual Agents (Edinburgh: Springer), 106–115.
Pecune, F., Cafaro, A., Ochs, M., and Pelachaud, C. (2016). “Evaluating social attitudes of a virtual tutor,” in International Conference on Intelligent Virtual Agents (Los Angeles, CA: Springer), 245–255.
Pezzullo, L. G., Wiggins, J. B., Frankosky, M. H., Min, W., Boyer, K. E., Mott, B. W., et al. (2017). “Thanks alisha, keep in touch”: gender effects and engagement with virtual learning companions,” in International Conference on Artificial Intelligence in Education (Wuhan: Springer), 299–310.
Pfeifer, A., and Lugrin, B. (2018). “Female robots as role-models? The influence of robot gender and learning materials on learning success,” in International Conference on Artificial Intelligence in Education (London: Springer), 276–280.
Plant, E. A., Baylor, A. L., Doerr, C. E., and Rosenberg-Kima, R. B. (2009). Changing middle-school students' attitudes and performance regarding engineering with computer-based social models. Comput. Educ. 53, 209–215. doi: 10.1016/j.compedu.2009.01.013
Räty, H., Vänskä, J., Kasanen, K., and Kärkkäinen, R. (2002). Parents' explanations of their child's performance in mathematics and reading: a replication and extension of yee and eccles. Sex Roles 46, 121–128. doi: 10.1023/A:1016573627828
Régner, I., Steele, J. R., Ambady, N., Thinus-Blanc, C., and Huguet, P. (2014). Our future scientists: a review of stereotype threat in girls from early elementary school to middle school. Revue Int. Psychol. Soc. 27, 13–51.
Richards, D., Alsharbi, B., and Abdulrahman, A. (2020). “Can I help you? Preferences of young adults for the age, gender and ethnicity of a virtual support person based on individual differences including personality and psychological state,” in Proceedings of the Australasian Computer Science Week Multiconference (Melbourne, VIC), 1–10.
Rosenberg-Kima, R., Baylor, A., Plant, E., and Doerr, C. (2008). Interface agents as social models for female students: the effects of agent visual presence and appearance on female students' attitudes and beliefs. Comput. Hum. Behav. 24, 2741–2756. doi: 10.1016/j.chb.2008.03.017
Rosenberg-Kima, R. B., Plant, E. A., Doerr, C. E., and Baylor, A. L. (2010). The influence of computer-based model's race and gender on female students' attitudes and beliefs towards engineering. J. Eng. Educ. 99, 35–44. doi: 10.1002/j.2168-9830.2010.tb01040.x
Sajjadi, P., Zhao, J., Wallgrün, J. O., Furman, T., La Femina, P. C., Fatemi, A., et al. (2020). “The effect of virtual agent gender and embodiment on the experiences and performance of students in virtual field trips,” in 2020 IEEE International Conference on Teaching, Assessment, and Learning for Engineering (TALE) (Takamatsu: IEEE), 221–228.
Sansone, D. (2019). Teacher characteristics, student beliefs, and the gender gap in STEM fields. Educ. Eval. Policy Anal. 41, 127–144. doi: 10.3102/0162373718819830
Schmader, T., and Johns, M. (2003). Converging evidence that stereotype threat reduces working memory capacity. J. Pers. Soc. Psychol. 85, 440. doi: 10.1037/0022-3514.85.3.440
Schroeder, N. L., Adesope, O. O., and Gilbert, R. B. (2013). How effective are pedagogical agents for learning? A meta-analytic review. J. Educ. Comput. Res. 49, 1–39. doi: 10.2190/EC.49.1.a
Schroeder, N. L., Romine, W. L., and Craig, S. D. (2017). Measuring pedagogical agent persona and the influence of agent persona on learning. Comput. Educ. 109, 176–186. doi: 10.1016/j.compedu.2017.02.015
Sczesny, S., Nater, C., and Eagly, A. H. (2018). “Agency and communion: Their implications for gender stereotypes and gender identities,” in Agency and Communion in Social Psychology (New York, NY: Routledge), 103–116.
Shiban, Y., Schelhorn, I., Jobst, V., Hörnlein, A., Puppe, F., Pauli, P., et al. (2015). The appearance effect: influences of virtual agent features on performance and motivation. Comput. Hum. Behav. 49, 5–11. doi: 10.1016/j.chb.2015.01.077
Silvervarg, A., Haake, M., and Gulz, A. (2013). “Educational potentials in visually androgynous pedagogical agents,” in International Conference on Artificial Intelligence in Education (Memphis, TN: Springer), 599–602.
Spencer, S. J., Steele, C. M., and Quinn, D. M. (1999). Stereotype threat and women's math performance. J. Exp. Soc. Psychol. 35, 4–28. doi: 10.1006/jesp.1998.1373
Spilioto-poulos, D., Makri, E., Vassilakis, C., and Margaris, D. (2020). Multimodal interaction: correlates of learners' metacognitive skill training negotiation experience. Information 11, 381. doi: 10.3390/info11080381
Steele, C. M., and Aronson, J. (1995). Stereotype threat and the intellectual test performance of african americans. J. Pers. Soc. Psychol. 69, 797. doi: 10.1037/0022-3514.69.5.797
Stokes, J., Riger, S., and Sullivan, M. (1995). Measuring perceptions of the working environment for women in corporate settings. Psychol. Women Q. 19, 533–549. doi: 10.1111/j.1471-6402.1995.tb00091.x
Świdrak, J., Pochwatko, G., and Insabato, A. (2021). Does an agent's touch always matter? Study on virtual midas touch, masculinity, social status, and compliance in Polish men. J. Multimodal User Interf. 15, 163–174. doi: 10.1007/s12193-020-00351-x
ter Stal, S., Tabak, M., op den Akker, H., Beinema, T., and Hermens, H. (2020). Who do you prefer? The effect of age, gender and role on users' first impressions of embodied conversational agents in ehealth. Int. J. Hum. Comput. Interact. 36, 881–892. doi: 10.1080/10447318.2019.1699744
Tickle-Degnen, L., and Rosenthal, R. (1990). The nature of rapport and its nonverbal correlates. Psychol. Inq. 1, 285–293. doi: 10.1207/s15327965pli0104_1
van der Lubbe, L. M., and Bosse, T. (2017). “Studying gender bias and social backlash via simulated negotiations with virtual agents,” in International Conference on Intelligent Virtual Agents (Stockholm: Springer), 455–458.
Vilaro, M. J., Wilson-Howard, D. S., Neil, J. M., Tavassoli, F., Zalake, M. S., Lok, B. C., et al. (2021). A subjective culture approach to cancer prevention: rural black and white adults' perceptions of using virtual health assistants to promote colorectal cancer screening. Health Commun. doi: 10.1080/10410236.2021.1910166
Webster, J., and Watson, R. T. (2002). Analyzing the past to prepare for the future: writing a literature review. MIS Q. 26, xiii-xxiii. doi: 10.2307/4132319
West, M., Kraut, R., and Ei Chew, H. (2019). I'd blush if I could: closing gender divides in digital skills through education. Available online at: https://unesdoc.unesco.org/ark:/48223/pf0000367416.page=1
Wirzberger, M., Schmidt, R., Georgi, M., Hardt, W., Brunnett, G., and Rey, G. D. (2019). Effects of system response delays on elderly humans cognitive performance in a virtual training scenario. Scientific Rep. 9, 1–12. doi: 10.1038/s41598-019-44718-x
Yee, D. K., and Eccles, J. S. (1988). Parent perceptions and attributions for children's math achievement. Sex Roles 19, 317–333. doi: 10.1007/BF00289840
Zanbaka, C., Goolkasian, P., and Hodges, L. (2006). “Can a virtual cat persuade you? The role of gender and realism in speaker persuasiveness,” in Proceedings of the SIGCHI Conference on Human Factors in Computing Systems (Montréal, QC), 1153–1162.
Keywords: virtual agent, gender, pedagogical agent, learning environment, gender stereotypes, systematic review
Citation: Armando M, Ochs M and Régner I (2022) The Impact of Pedagogical Agents' Gender on Academic Learning: A Systematic Review. Front. Artif. Intell. 5:862997. doi: 10.3389/frai.2022.862997
Received: 26 January 2022; Accepted: 19 May 2022;
Published: 20 June 2022.
Edited by:
Elaine Harada Teixeira de Oliveira, Federal University of Amazonas, BrazilReviewed by:
Kaoru Sumi, Future University Hakodate, JapanCarol M. Forsyth, Educational Testing Service, United States
Copyright © 2022 Armando, Ochs and Régner. This is an open-access article distributed under the terms of the Creative Commons Attribution License (CC BY). The use, distribution or reproduction in other forums is permitted, provided the original author(s) and the copyright owner(s) are credited and that the original publication in this journal is cited, in accordance with accepted academic practice. No use, distribution or reproduction is permitted which does not comply with these terms.
*Correspondence: Magalie Ochs, magalie.ochs@lis-lab.fr; Isabelle Régner, isabelle.regner@univ-amu.fr